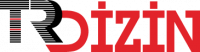
Comparison of AR parametric methods with subspace-based methods for EMG signal classification using stand-alone and merged neural network models
Yıl: 2016 Cilt: 24 Sayı: 3 Sayfa Aralığı: 1547 - 1559 Metin Dili: İngilizce İndeks Tarihi: 29-07-2022
Comparison of AR parametric methods with subspace-based methods for EMG signal classification using stand-alone and merged neural network models
Öz: This research introduces an electromyogram (EMG) pattern classification of individual motor unit action potentials (MUPs) from intramuscular electromyographic signals. The presented technique automatically classifies EMG patterns into healthy, myopathic, or neurogenic categories. To extract a feature vector from the EMG signal, we use different autoregressive (AR) parametric methods and subspace-based methods. The proposal was validated using EMG recordings composed of 1200 EMG patterns obtained from 7 healthy, 7 myopathic, and 13 neurogenic-disordered people. A feedforward error backpropagation artificial neural network (FEBANN) and combined neural network (CNN) were used for classification, where the success rate was slightly higher in CNN. Among the different AR and subspace methods used in this study, the highest performance was obtained with the eigenvector method. The following rates were the results achieved by using the CNN. The correct classification rate for EMG patterns was 97% for healthy, 93% for myopathic, and 92% for neurogenic patterns. The obtained accuracy for EMG signal classification is approximately 94% for CNN. The rates for FEBANN were as follows: 97% for healthy patterns, 92% for myopathic patterns, and 91% for neurogenic patterns. The obtained accuracy was 93.3%. By directly using raw EMG signals, EMG classifications of healthy, myopathic, or neurogenic classes are automatically addressed.
Anahtar Kelime: Konular:
Belge Türü: Makale Makale Türü: Araştırma Makalesi Erişim Türü: Erişime Açık
- [1] Henneberg K. Principles of electromyography. In: Bronzino JD, editor. The Biomedical Engineering Handbook. Boca Raton, FL, USA: CRC Press, 2000. pp. 242251.
- [2] Katsis CD, Goletsis Y, Likas A, Fotiadis DI, Sarmas I. A novel method for automated EMG decomposition and MUAP classification. Artif Intell Med 2006; 37: 5564.
- [3] McGill KC. Optimal resolution of superimposed action potentials. IEEE T Biomed Eng 2002; 49: 640650.
- [4] Krarup C. Pitfalls in electrodiagnosis. J Neurol 1999; 246: 11151126.
- [5] Richfield EK, Cohen BA, Albers JW. Review of quantitative and automated needle electromyographic analyses. IEEE T Biomed Eng 1981; 28: 506514.
- [6] Pattichis CS, Elia AG. Autoregressive and cepstral analyses of motor unit action potentials. Med Eng Phys 1999; 21: 405419.
- [7] Pattichis CS, Schizas CN, Middleton LT. Neural network models in EMG diagnosis. IEEE T Biomed Eng 1995; 42: 486496.
- [8] Loudon GH, Jones NB, Sehmi AS. New signal processing techniques for the decomposition of EMG signals. Med Biol Eng Comput 1992; 30: 591599.
- [9] Hassoun MH, Wang C, Spitzer AR. NNERVE: Neural network extraction of repetitive vectors for electromyographyPart I: Algorithm. IEEE T Biomed Eng 1994; 41: 10391052.
- [10] Hassoun MH, Wang C, Spitzer AR. NNERVE: Neural network extraction of repetitive vectors for electromyographyPart II: Performance analysis. IEEE T Biomed Eng 1994; 41: 10531061.
- [11] Christodoulou CI, Pattichis CS. Unsupervised pattern recognition for the classification of EMG signals. IEEE T Biomed Eng 1999; 46: 169178.
- [12] Subasi A, Kiymik MK. Muscle fatigue detection in EMG using timefrequency methods, ICA and neural networks. J Med Syst 2010; 34: 777785.
- [13] Diab MO, El-Merhie A, El-Halabi N, Khoder L. Classification of uterine EMG signals using supervised classification method. J Biomed Sci Eng 2013; 3: 837842.
- [14] Subasi A. Classification of EMG signals using PSO optimized SVM for diagnosis of neuromuscular disorders. Comput Biol Med 2013; 43: 576586.
- [15] Sueaseenak D, Chanwimalueang T, Pintavirooj C, Sangworasil M. An accurate forearm EMG signal classification method using two-channel electrode. IEEJ T Electr Electr 2013; 8: 328338.
- [16] Young AJ, Smith LH, Rouse EJ, Hargrove LJ. Classification of simultaneous movements using surface EMG pattern recognition. IEEE T Biomed Eng 2013; 60: 12501258.
- [17] Ibrahimy MI, Ahsan R, Khalifa OO. Design and optimization of Levenberg-Marquardt based neural network classifier for EMG signals to identify hand motions. Meas Sci Rev 2013; 13: 142151.
- [18] Muthuswamy J, Thakor NV. Spectral analysis methods for neurological signals. J Neurosci Meth 1998; 83: 114.
- [19] Isaksson A, Wennberg A, Zetterberg LH. Computer analysis of EEG signals with parametric models. P IEEE 1981; 69: 451461.
- [20] Swindlehurst AL, Kailath T. A performance analysis of subspace-based methods in the presence of model errors Part I: The MUSIC algorithm. IEEE T Signal Proces 1992; 40: 17581774.
- [21] Swindlehurst AL, Kailath T. A performance analysis of subspace-based methods in the presence of model errors Part II: Multidimensional algorithms. IEEE T Signal Proces 1993; 41: 28822890.
- [22] Friedlander B, Weiss AJ. Effects of model errors on waveform estimation using the MUSIC algorithm. IEEE T Signal Proces 1994; 42: 147155.
- [23] Friedlander B. A sensitivity analysis of the MUSIC algorithm. IEEE T Acoust Speech 1990; 38: 17401751.
- [24] Porat B, Friedlander B. Analysis of the asymptotic relative efficiency of the MUSIC algorithm. IEEE T Acoust Speech 1998; 36: 532544.
- [25] Proakis JG, Manolakis DG. Digital Signal Processing: Principles, Algorithms, and Applications. 1st ed. Upper Saddle River, NJ, USA: Prentice Hall, 1996.
- [26] Kay SM, Marple SL. Spectrum analysis-a modern perspective. P IEEE 1981; 69: 13801419.
- [27] Kay SM. Modern Spectral Estimation: Theory and Application. Upper Saddle River, NJ, USA: Prentice Hall, 1999.
- [28] Stoica P, Moses R. Introduction to Spectral Analysis. Upper Saddle River, NJ, USA: Prentice Hall, 1997.
- [29] Subasi A, Er¸celebi E, Alkan A, Koklukaya E. Comparison of subspace-based methods with AR parametric methods in epileptic seizure detection. Comput Biol Med 2006; 36: 195208.
- [30] Subasi A, Yilmaz M, Ozcalik HR. Classification of EMG signals using wavelet neural network. J Neurosci Meth 2006; 156: 360367.
- [31] Subasi A. Classi?cation of EMG signals using combined features and soft computing techniques. Appl Soft Comp 2012; 12: 21882198.
- [32] Akaike H. A new look at the statistical model identification. IEEE T Automat Contr 1974; 19: 716723.
- [33] Schmidt RO. Multiple emitter location and signal parameter estimation. IEEE T Antenn Propag 1986; 34: 276280.
- [34] Wax M, Kailath T. Detection of signals by information theoretic criteria. IEEE T Acoust Speech 1985; 33: 387392.
- [35] Micheli-Tzanakou E. Neural networks in biomedical signal processing. In: Bronzino JD, editor. The Biomedical Engineering Handbook. Boca Raton, FL, USA: CRC Press, 2000. pp. 713.
- [36] Haykin S. Neural Networks: A Comprehensive Foundation. New York, NY, USA: Macmillan, 1994.
- [37] Fausett L. Fundamentals of Neural Networks: Architectures, Algorithms, and Applications. Englewood Cliffs, NJ, USA: Prentice Hall, 1994.
- [38] G¨uler I, Ubeyli ED. ECG beat classifier designed by combined neural network model. Pattern Recogn 2005; 38: ¨ 199208.
- [39] Hansen JV, Nelson RD. Data mining of time series using stacked generalizers. Neurocomputing 2002; 43: 173184.
- [40] Ting KM, Witten IH. Issues in stacked generalization. J Artif Intell Res 1999; 10: 271289.
- [41] Wolpert DH. Stacked generalization. Neural Networks 1992; 5: 241259.
- [42] Zhang R, McAllister G, Scotney B, McClean S, Houston G. Combining wavelet analysis and Bayesian networks for the classification of auditory brainstem response. IEEE T Inf Technol B 2006; 10: 458467.
APA | BOZKURT M, SUBAŞI A, KÖKLÜKAYA E, Yılmaz M (2016). Comparison of AR parametric methods with subspace-based methods for EMG signal classification using stand-alone and merged neural network models. , 1547 - 1559. |
Chicago | BOZKURT MEHMET RECEP,SUBAŞI Abdulhamit,KÖKLÜKAYA Etem,Yılmaz Mustafa Comparison of AR parametric methods with subspace-based methods for EMG signal classification using stand-alone and merged neural network models. (2016): 1547 - 1559. |
MLA | BOZKURT MEHMET RECEP,SUBAŞI Abdulhamit,KÖKLÜKAYA Etem,Yılmaz Mustafa Comparison of AR parametric methods with subspace-based methods for EMG signal classification using stand-alone and merged neural network models. , 2016, ss.1547 - 1559. |
AMA | BOZKURT M,SUBAŞI A,KÖKLÜKAYA E,Yılmaz M Comparison of AR parametric methods with subspace-based methods for EMG signal classification using stand-alone and merged neural network models. . 2016; 1547 - 1559. |
Vancouver | BOZKURT M,SUBAŞI A,KÖKLÜKAYA E,Yılmaz M Comparison of AR parametric methods with subspace-based methods for EMG signal classification using stand-alone and merged neural network models. . 2016; 1547 - 1559. |
IEEE | BOZKURT M,SUBAŞI A,KÖKLÜKAYA E,Yılmaz M "Comparison of AR parametric methods with subspace-based methods for EMG signal classification using stand-alone and merged neural network models." , ss.1547 - 1559, 2016. |
ISNAD | BOZKURT, MEHMET RECEP vd. "Comparison of AR parametric methods with subspace-based methods for EMG signal classification using stand-alone and merged neural network models". (2016), 1547-1559. |
APA | BOZKURT M, SUBAŞI A, KÖKLÜKAYA E, Yılmaz M (2016). Comparison of AR parametric methods with subspace-based methods for EMG signal classification using stand-alone and merged neural network models. Turkish Journal of Electrical Engineering and Computer Sciences, 24(3), 1547 - 1559. |
Chicago | BOZKURT MEHMET RECEP,SUBAŞI Abdulhamit,KÖKLÜKAYA Etem,Yılmaz Mustafa Comparison of AR parametric methods with subspace-based methods for EMG signal classification using stand-alone and merged neural network models. Turkish Journal of Electrical Engineering and Computer Sciences 24, no.3 (2016): 1547 - 1559. |
MLA | BOZKURT MEHMET RECEP,SUBAŞI Abdulhamit,KÖKLÜKAYA Etem,Yılmaz Mustafa Comparison of AR parametric methods with subspace-based methods for EMG signal classification using stand-alone and merged neural network models. Turkish Journal of Electrical Engineering and Computer Sciences, vol.24, no.3, 2016, ss.1547 - 1559. |
AMA | BOZKURT M,SUBAŞI A,KÖKLÜKAYA E,Yılmaz M Comparison of AR parametric methods with subspace-based methods for EMG signal classification using stand-alone and merged neural network models. Turkish Journal of Electrical Engineering and Computer Sciences. 2016; 24(3): 1547 - 1559. |
Vancouver | BOZKURT M,SUBAŞI A,KÖKLÜKAYA E,Yılmaz M Comparison of AR parametric methods with subspace-based methods for EMG signal classification using stand-alone and merged neural network models. Turkish Journal of Electrical Engineering and Computer Sciences. 2016; 24(3): 1547 - 1559. |
IEEE | BOZKURT M,SUBAŞI A,KÖKLÜKAYA E,Yılmaz M "Comparison of AR parametric methods with subspace-based methods for EMG signal classification using stand-alone and merged neural network models." Turkish Journal of Electrical Engineering and Computer Sciences, 24, ss.1547 - 1559, 2016. |
ISNAD | BOZKURT, MEHMET RECEP vd. "Comparison of AR parametric methods with subspace-based methods for EMG signal classification using stand-alone and merged neural network models". Turkish Journal of Electrical Engineering and Computer Sciences 24/3 (2016), 1547-1559. |