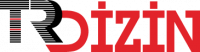
Assessing the importance of features for detection of hard exudates in retinal images
Yıl: 2017 Cilt: 25 Sayı: 2 Sayfa Aralığı: 1223 - 1237 Metin Dili: İngilizce İndeks Tarihi: 29-07-2022
Assessing the importance of features for detection of hard exudates in retinal images
Öz: Diabetes disrupts the operation of the eye and leads to vision loss, affecting particularly the nerve layer and capillary vessels in this layer by changes in the blood vessels of the retina. Suddenly loss and blurred vision problems occur in the image, depending on the phase of the disease, called diabetic retinopathy. Hard exudates are one of the primary signs of diabetic retinopathy. Automatic recognition of hard exudates in retinal images can contribute to detection of the disease. We present an automatic screening system for the detection of hard exudates. This system consists of two main steps. Firstly, the features were extracted from patch images consisting of hard exudate and normal regions using the DAISY algorithm based on the histogram of oriented gradients. After, we utilized the recursive feature elimination (RFE) method, using logistic regression (LR) and support vector classi er (SVC) estimators on the raw dataset. Therefore, we obtained two datasets containing the most important features. The number of important features in each dataset created with LR and SVC was 126 and 259, respectively. Afterward, we observed different classi er algorithms' performances by using 5-fold cross validation on these important features' dataset and it was observed that the random forest (RF) classi er is the best classi er. Secondly, we obtained important features from the feature vector that corresponds with the region of interest in accordance with the keypoint information in a new retinal fundus image. Then we performed detection of hard exudate regions on the retinal fundus image by using the RF classi er.
Anahtar Kelime: Konular:
Belge Türü: Makale Makale Türü: Araştırma Makalesi Erişim Türü: Erişime Açık
- [1] Mohamed Q, Gillies MC, Wong TY. Management of diabetic retinopathy: a systematic review. Jama-J Am Med Assoc 2007; 298: 902-916.
- [2] Venkatesan R, Chandakkar P, Li B, Li HK. Classi cation of diabetic retinopathy images using multi-class multiple- instance learning based on color correlogram features. In: IEEE 2012 International Conference on Engineering in Medicine and Biology Society (EMBC); 28 Aug{1 Sep 2012; San Diego, CA, USA: IEEE. pp. 1462-1465.
- [3] Kauppi T, Kalesnykiene V, Kamarainen JK, Lensu L, Sorri I, Raninen A, Voutilainen R, Uusitalo H, Kalviainen H, Pietila J. Diaretdb1 diabetic retinopathy database and evaluation protocol. In: Proceedings of the British Machine Vision Conference; 10{13 Sep 2007; University of Warwick, UK: pp. 252-261.
- [4] Bay H, Ess A, Tuytelaars T, Gool LV. SURF: Speeded-up robust features. Comput Vis Image Und 2008; 110: 346-359.
- [5] Calonder M, Lepetit V, Strecha C, Fua P. BRIEF: Binary robust independent elementary features. Lect Notes Comput Sc 2010; 6314: 778-792.
- [6] Rosten E, Drummond T. Machine learning for high-speed corner detection. Lect Notes Comput Sc 2006; 3951: 430-443.
- [7] Chen X, Bu W, Wu X, Dai B, Teng Y. A novel method for automatic hard exudates detection in color retinal images. In: IEEE 2012 International Conference on Machine Learning and Cybernetics (ICMLC); 15{17 Jul 2012; Xian, China: IEEE. pp. 1175-1181.
- [8] Garcia M, Valverde C, Lopez MI, Poza J, Hornero R. Comparison of logistic regression and neural network classi ers in the detection of hard exudates in retinal images. In: IEEE 2013 International Conference on Engineering in Medicine and Biology Society; 3{7 Jul 2013; Osaka, Japan: IEEE. pp. 5891-5894.
- [9] Kayal D, Banerjee S. A new dynamic thresholding based technique for detection of hard exudates in digital retinal fundus image. In: IEEE 2014 International Conference on Signal Processing and Integrated Networks (SPIN); 20{21 Feb 2014; Noida, India: IEEE. pp. 141-144.
- [10] Mishra AM, Singh PK, Chawla KS. An information geometry based scheme for hard exudate detection in fundus images. In: IEEE 2011 India Conference (INDICON); 16{18 Dec 2011; Hyderabad, India: IEEE. pp. 1-4.
- [11] Welfer D, Scharcanski J, Marinho DR. A coarse-to- ne strategy for automatically detecting exudates in color eye fundus images. Comput Med Imag Grap 2010; 34: 228-235.
- [12] Sanchez CI, Niemeijer M, Suttorp Schulten MSA, Abramoff M, Van Ginneken B. Improving hard exudate detec- tion in retinal images through a combination of local and contextual information. In: IEEE 2010 International Symposium on Biomedical Imaging: From Nano to Macro; 14{17 Apr 2010; Rotterdam, Netherlands: IEEE. pp. 5-8.
- [13] Tjandrasa H, Putra RE, Wijaya AY, Arieshanti I. Classi cation of non-proliferative diabetic retinopathy based on hard exudates using soft margin SVM. In: IEEE 2013 International Conference on Control System, Computing and Engineering (ICCSCE); 29 Nov{1 Dec 2013; Mindeb: IEEE. pp. 376-380.
- [14] Xu L, Luo S. Support vector machine based method for identifying hard exudates in retinal images. In: IEEE 2009 Youth Conference on Information Computing and Telecommunication; 20{21 Sep 2009; Beijing, China: IEEE. pp. 138-141.
- [15] Osareh A. Mirmehdi M, Thomas B, Markham R. Automated identi cation of diabetic retinal exudates in digital colour images. Br J Ophthalmol 2003; 870: 1220-1223.
- [16] Zhang X, Chutatape O. Top-down and bottom-up strategies in lesion detection of background diabetic retinopathy. Proc CVPR IEEE 2005; 2: 422-428.
- [17] Sanchez CI, Hornero R, Lopez MI, Aboy M, Poza J, Abasolo D. A novel automatic image processing algorithm for detection of hard exudates based on retinal image analysis. Med Eng Phys 2008; 30: 350-357.
- [18] Jaya T, Dheeba J, Singh NA. Detection of hard exudates in colour fundus images using Fuzzy Support Vector Machine-based expert system. J Digit Imaging 2015; 28: 761-768.
- [19] Lin P, Bing-Kun Z. An effective approach to detect hard exudates in color retinal image. In: Qian Z, Cao L, Su W, Wang T, Yang H, editors. Recent Advances in Computer Science and Information Engineering. Berlin, Germany: Springer, 2012. pp. 541-546.
- [20] JayaKumari C, Maruthi R. Detection of hard exudates in color fundus images of the human retina. Procedia Engineering 2012; 30: 297-302.
- [21] Xu L, Luo S. Support vector machine based method for identifying hard exudates in retinal images. In: IEEE 2009 Youth Conference on Information, Computing and Telecommunication; 20{21 Sep 2009; Beijing, China: IEEE. pp. 138-141.
- [22] Yang J, Jiang YG, Hauptmann AG, Ngo CW. Evaluating bag-of-visual-words representations in scene classi cation. In: International Multimedia Conference; 24 Sep 2007; ACM, New York, NY, USA: pp. 197-206.
- [23] Mikolajczyk K, Schmid C. A performance evaluation of local descriptors. IEEE T Pattern Anal 2005; 27: 1615-1630.
- [24] Jiang YG, Ngo CW, Yang J. Towards optimal bag-of-features for object categorization and semantic video retrieval. In: Conference on Image and Video Retrieval; 09 Jun 2007; ACM, New York, NY, USA: pp. 494-501.
- [25] Lazebnik S, Schmid C, Ponce J. Beyond bags of features: spatial pyramid matching for recognizing natural scene categories. Proc CVPR IEEE 2006; 2: 2169-2178.
- [26] Fei-Fei L, Perona P. A bayesian hierarchical model for learning natural scene categories. Proc CVPR IEEE 2006; 2: 524-531.
- [27] Sivic J, Zisserman A. Video google: A text retrieval approach to object matching in videos. In: IEEE 2003 International Conference on Computer Vision; 13{16 Oct 2003; Nice, France: IEEE. pp. 1470-1477.
- [28] Rublee E, Rabaud V, Konolige K, Bradski G. ORB: an efficient alternative to SIFT or SURF. In: IEEE International Conference on Computer Vision (ICCV); 6{13 Nov 2011; Barcelona, Spain: IEEE. pp. 2564-2571.
- [29] Tola E, Lepetit V, Fua P. DAISY: An efficient dense descriptor applied to wide-baseline stereo. IEEE T Pattern Anal 2010; 32: 815-830.
- [30] Guyon I, Weston J, Barnhill S, Vapnik V. Gene selection for cancer classi cation using support vector machines. Mach Learn 2002; 46: 389-422.
- [31] Aggarwal CC. Data classi cation algorithms and applications. In: Kumar V, editor. Data Mining and Knowledge Discovery Series. Boca Raton, FL, USA: CRC Press 2014. pp. 11-16, 493-495.
- [32] Breiman L. Random forests. Mach Learn 2001; 45: 5-32.
- [33] Parikh R, Mathai A, Parikh S, Sekhar GC, Thomas R. Understanding and using sensitivity, speci city and predictive values. Indian J Ophthalmol 2008; 56: 45-50.
- [34] Wong HB, Lim GH. Measures of diagnostic accuracy: sensitivity, speci city, PPV and NPV. Proceedings of Singapore Healthcare 2011; 20: 316-318.
- [35] Shaikh SA. Measures derived from a 2x2 table for an accuracy of a diagnostic test. J Biom Biostat 2011; 2: 128.
- [36] Kuncheva LI. Combining Pattern Classi ers: Methods and Algorithms. Hoboken, NJ, USA: Wiley, 2004.
- [37] Sasi NM, Jayasree VK. Contrast limited adaptive histogram equalization for qualitative enhancement of myocardial perfusion images. Engineering 2013; 5: 326-331.
APA | AKYOL K, ŞEN B, BAYIR Ş, ÇAKMAK H (2017). Assessing the importance of features for detection of hard exudates in retinal images. , 1223 - 1237. |
Chicago | AKYOL KEMAL,ŞEN BAHA,BAYIR Şafak,ÇAKMAK Hasan Basri Assessing the importance of features for detection of hard exudates in retinal images. (2017): 1223 - 1237. |
MLA | AKYOL KEMAL,ŞEN BAHA,BAYIR Şafak,ÇAKMAK Hasan Basri Assessing the importance of features for detection of hard exudates in retinal images. , 2017, ss.1223 - 1237. |
AMA | AKYOL K,ŞEN B,BAYIR Ş,ÇAKMAK H Assessing the importance of features for detection of hard exudates in retinal images. . 2017; 1223 - 1237. |
Vancouver | AKYOL K,ŞEN B,BAYIR Ş,ÇAKMAK H Assessing the importance of features for detection of hard exudates in retinal images. . 2017; 1223 - 1237. |
IEEE | AKYOL K,ŞEN B,BAYIR Ş,ÇAKMAK H "Assessing the importance of features for detection of hard exudates in retinal images." , ss.1223 - 1237, 2017. |
ISNAD | AKYOL, KEMAL vd. "Assessing the importance of features for detection of hard exudates in retinal images". (2017), 1223-1237. |
APA | AKYOL K, ŞEN B, BAYIR Ş, ÇAKMAK H (2017). Assessing the importance of features for detection of hard exudates in retinal images. Turkish Journal of Electrical Engineering and Computer Sciences, 25(2), 1223 - 1237. |
Chicago | AKYOL KEMAL,ŞEN BAHA,BAYIR Şafak,ÇAKMAK Hasan Basri Assessing the importance of features for detection of hard exudates in retinal images. Turkish Journal of Electrical Engineering and Computer Sciences 25, no.2 (2017): 1223 - 1237. |
MLA | AKYOL KEMAL,ŞEN BAHA,BAYIR Şafak,ÇAKMAK Hasan Basri Assessing the importance of features for detection of hard exudates in retinal images. Turkish Journal of Electrical Engineering and Computer Sciences, vol.25, no.2, 2017, ss.1223 - 1237. |
AMA | AKYOL K,ŞEN B,BAYIR Ş,ÇAKMAK H Assessing the importance of features for detection of hard exudates in retinal images. Turkish Journal of Electrical Engineering and Computer Sciences. 2017; 25(2): 1223 - 1237. |
Vancouver | AKYOL K,ŞEN B,BAYIR Ş,ÇAKMAK H Assessing the importance of features for detection of hard exudates in retinal images. Turkish Journal of Electrical Engineering and Computer Sciences. 2017; 25(2): 1223 - 1237. |
IEEE | AKYOL K,ŞEN B,BAYIR Ş,ÇAKMAK H "Assessing the importance of features for detection of hard exudates in retinal images." Turkish Journal of Electrical Engineering and Computer Sciences, 25, ss.1223 - 1237, 2017. |
ISNAD | AKYOL, KEMAL vd. "Assessing the importance of features for detection of hard exudates in retinal images". Turkish Journal of Electrical Engineering and Computer Sciences 25/2 (2017), 1223-1237. |