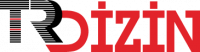
Predicting the response to bDMARD treatment in RA: Then what?
Yıl: 2022 Cilt: 14 Sayı: 2 Sayfa Aralığı: 87 - 93 Metin Dili: İngilizce DOI: 10.4274/raed.galenos.2022.69188 İndeks Tarihi: 19-09-2022
Predicting the response to bDMARD treatment in RA: Then what?
Öz: Objective: Biologic disease-modifying antirheumatic drugs (bDMARDs) offer promising results for rheumatoid arthritis (RA) patients in general, but a substantial percentage of patients do not respond to them. It is important to predict the response before the treatment so that unnecessary adversities for the patients and costs for the healthcare system can be avoided. This study aims to develop a machine learning (ML) model that works with readily-available demographic and clinical factors for prediction of response to bDMARDs, and discusses additional non-pharmacological practices. Methods: Several ML models were tested in 190 RA patients from Turkey, and the logistic regression model was found to be superior. The relation between long-term and short-term responses were also analyzed. Results: Predictors of the logistic regression model were age, sex, coronary artery disease, spine surgery, steroid treatment, sulfasalazine treatment and baseline health assesment questionnaire score. The model displayed 79.5% accuracy and an area under receiver operating characteristic curve of 0.82. 87% of the patients who were goodresponders in six-month follow-up were also good responders in oneyear follow-up. Among non-responders in six-month follow-up, 75% were also non-responders in one-year follow-up. Conclusion: Making the prediction at an early stage is crucial for the patients as well as the healthcare system. However, it is equally important to determine how to proceed with the patients who are unlikely to respond to bDMARDs. Current literature does not adequately answer this question. Additional treatment options and multiple evaluation criteria for these options should be considered; multiple criteria models can provide useful decision support for this purpose.
Anahtar Kelime: Romatoid artritte bDMARD yanıtını öngörmek: Peki sonrasında?
Öz: Amaç: Biyolojik hastalık modifiye edici antiromatizmal ilaçlar (bDMARD’lar) genel olarak romatoid artrit (RA) hastaları için umut verici sonuçlar sunar; ancak hastaların önemli bir yüzdesi bunlara yanıt vermez. Yan etkilerin azaltılması ve sağlık sistemi için maliyetlerden kaçınılabilmesi için yanıtın tedavi öncesi tahmin edilmesi önemlidir. Bu çalışmada, bDMARD’lara yanıtı tahmin etmek için demografik ve klinik faktörlerle çalışan bir makine öğrenimi (ML) modeli geliştirme ve ek farmakolojik olmayan uygulamaların tartışılması amaçlanmıştır. Yöntem: Yüz doksan Türk RA hastasında birkaç ML modeli test edilmiştir ve lojistik regresyon modelinin üstün olduğu bulunmuştur. Uzun ve kısa vadeli sonuçlar arasındaki ilişki de analiz edilmiştir. Bulgular: Lojistik regresyon modelinde, cinsiyet, koroner arter hastalığı, omurga cerrahisi, steroid tedavisi, sülfasalazin tedavisi ve başlangıç sağlık değerlendirme anketi skoru prediktör olarak saptanmıştır. Model, %79,5 doğruluk ve 0,82’lik bir alıcı işletim karakteristiği eğrisi altında kalan alan sergilemiştir. Altı aylık takipte iyi yanıt veren hastaların %87’sinin, bir yıllık takipte de iyi yanıt verdiği gözlemlenmiştir. Altı aylık takipte yanıt vermeyenlerin %75’inin bir yıllık takipte yanıt vermediği gözlemlenmiştir. Sonuç: Tedavi yanıtlarının erken aşamada öngörülmesi hastalar için olduğu kadar sağlık sistemi için de çok önemlidir. Bununla birlikte, bDMARD’lara yanıt verme olasılığı düşük olan hastalarda nasıl bir yol izleneceğini belirlemek de aynı derecede önemlidir. Mevcut literatür bu soruya yeterince cevap vermemektedir. Ek tedavi seçenekleri ve çoklu değerlendirme kriterleri göz önünde bulundurulmalıdır; çok kriterli modeller bu amaç için faydalı karar desteği sağlayabilir.
Anahtar Kelime: Belge Türü: Makale Makale Türü: Araştırma Makalesi Erişim Türü: Erişime Açık
- 1. Li H, Luo M, Zheng J, et al. An artificial neural network prediction model of congenital heart disease based on risk factors: a hospitalbased case-control study. Medicine (Baltimore) 2017;96:e6090.
- 2. Weng SF, Reps J, Kai J, Garibaldi JM, Quereshi N. Can machinelearning improve cardiovascular risk prediction using routine clinical data. PLoS One 2017;12:e0174944.
- 3. Dagliati A, Marini S, Sacchi L, et al. Machine learning methods to predict diabetes complications. J Diabetes Sci Technol 2018;12:295-302.
- 4. Lee H, Troschel FM, Tajmir S, et al. Pixel-level deep segmentation: artificial intelligence quantifies muscle on computed tomography for body morphometric analysis. J Digit Imaging 2017;30:487-98.
- 5. Becker A. Artificial intelligence in medicine: What is it doing for us today? Health Policy and Technology 2019;8:198-205.
- 6. Kłak A, Paradowska-Gorycka A, Kwiatkowska B, Raciborski F. Personalized medicine in rheumatology. Reumatologia 2016;54:177-86.
- 7. Singh S, Kumar A, Panneerselvam K, Vennila JJ. Diagnosis of arthritis through fuzzy inference system. J Med Syst 2012;36:1459-68.
- 8. Alshawwa IA, Elkahlout M, El-Mashharawi HQ, Abu-Naser SS. An Expert System for Depression Diagnosis. IJAHMR 2019;3:20-7.
- 9. Yoo J, Lim MK, Ihm C, Choi ES, Kang MS. A study on prediction of rheumatoid arthritis using machine learning. International Journal of Applied Engineering Research 2017;12:9858-62.
- 10. Shanmugam S, Preethi J. Improved feature selection and classification for rheumatoid arthritis disease using weighted decision tree approach (REACT). The Journal of Supercomputing 2019;75:5507-19.
- 11. Alder H, Michel BA, Marx C, et al. Computer-based diagnostic expert systems in rheumatology: Where do we stand in 2014? Int J Rheumatol 2014;672714.
- 12. Huizinga TWJ. Personalied medicine in rheumatoid arthritis: is the glass half full or half empty? J Intern Med 2015;277:178-87.
- 13. Anderson JJ, Wells G, Verhoeven AC, Felson DT. Factors predicting response to treatment in rheumatoid arthritis: the importance of disease duration. Arthritis Rheum 2000;43:22-9.
- 14. Ma MHY, Ibrahim F, Walker D, et al. Remission in early rheumatoid arthritis: predicting treatment response. J Rheumatol 2012;39:470-5.
- 15. Romão VC, Canhão H, Fonseca JE. Old drugs, old problems: Where do we stand in prediction of rheumatoid arthritis responsiveness to methotrexate and other synthetic DMARDs? BMC Med 2013;11:17.
- 16. Takeuchi T. Biomarkers as a treatment guide in rheumatoid arthritis. Clinical Immunology 2018; 186:59-62.
- 17. Maciejewski M, Sands C, Nair N, et al. Prediction of response of methotrexate in patients with rheumatoid arthritis using serum lipidomics. Scientific Reports 2021;11:7266.
- 18. Conaghan PG. Predicting outcomes in rheumatoid arthritis. Clin Rheumatol 2011;30:41-7.
- 19. Hashimoto T, Yoshida K, Hashimoto N, et al. Circulating cell free DNA: a marker to predict the therapeutic response for biological DMARDs in rheumatoid arthritis. Int J Rheum Dis 2017;20:722-30.
- 20. Wijbrandts CA, Tak PP. Prediction of Response to Targeted Treatment in Rheumatoid Arthritis. Mayo Clin Proc 2017;92:1129-43.
- 21. Hyrich KL, Watson KD, Silman AJ, Symmons DPM, British Society for Rheumatology Biologics Register. Predictors of response to anti-TNF-alpha therapy among patients with rheumatoid arthritis: results from the British Society for Rheumatology Biologics Register. Rheumatology (Oxford) 2006;45:1558-65.
- 22. Atzeni F, Antivalle M, Pallavicini FB, et al. Predicting response to anti-TNF treatment in rheumatoid arthritis patients. Autoimmun Rev 2009;8:431-7.
- 23. Koo BS, Eun S, Shin K, et al. Machine learning model for identifying important clinical features for predicting remission in patients with rheumatoid arthritis treated with biologics. Arthritis Res Ther 2021;23:178.
- 24. Verstappen SMM, Jacobs JWG, Huisman A-M, van Rijthoven AWAM, Sokka T. Functional Health Assessment Questionnaire (HAQ) and Psychological HAQ Are Associated with and Predicted by Different Factors in Rheumatoid Arthritis. J Rheumatol 2007;34:1837-40.
- 25. Maska L, Anderson J, Michaud K. Measures of functional status and quality of life in rheumatoid arthritis: Health Assessment Questionnaire Disability Index (HAQ), Modified Health Assessment Questionnaire (MHAQ), Multidimensional Health Assessment Questionnaire (MDHAQ), Health Assessment Questionnaire II (HAQ-II), Improved Health Assessment Questionnaire (Improved HAQ), and Rheumatoid Arthritis Quality of Life (RAQoL). Arthritis Care Res (Hoboken) 2011;63 (Suppl 11):S4-13.
- 26. Behrens F, Koehm M, Schwaneck EC, et al. Use of a “critical difference” statistical criterion improves the predictive utility of the Health Assessment Questionnaire-Disability Index score in patients with rheumatoid arthritis. BMC Rheumatol 2019;3:51.
- 27. Roodenrijs NMT, Hamar A, Kedves M, et al. Pharmacological and non-pharmacological therapeutic strategies in difficult-totreat rheumatoid arthritis: a systematic literature review informing the EULAR recommendations for the management of difficultto- treat rheumatoid arthritis. RMD Open 2021;7:e001512.
- 28. Adunin G, Diaby V, Xiao H. Application of multicriteria decision analysis in health care: a systematic review and bibliometric analysis. Health Expect 2015;18:1894-905.
APA | Tuncer Sakar C, KARAKAYA G, Bilgin E, Kılıç L, Kalyoncu U (2022). Predicting the response to bDMARD treatment in RA: Then what?. , 87 - 93. 10.4274/raed.galenos.2022.69188 |
Chicago | Tuncer Sakar Ceren,KARAKAYA GULSAH,Bilgin Emre,Kılıç Levent,Kalyoncu Umut Predicting the response to bDMARD treatment in RA: Then what?. (2022): 87 - 93. 10.4274/raed.galenos.2022.69188 |
MLA | Tuncer Sakar Ceren,KARAKAYA GULSAH,Bilgin Emre,Kılıç Levent,Kalyoncu Umut Predicting the response to bDMARD treatment in RA: Then what?. , 2022, ss.87 - 93. 10.4274/raed.galenos.2022.69188 |
AMA | Tuncer Sakar C,KARAKAYA G,Bilgin E,Kılıç L,Kalyoncu U Predicting the response to bDMARD treatment in RA: Then what?. . 2022; 87 - 93. 10.4274/raed.galenos.2022.69188 |
Vancouver | Tuncer Sakar C,KARAKAYA G,Bilgin E,Kılıç L,Kalyoncu U Predicting the response to bDMARD treatment in RA: Then what?. . 2022; 87 - 93. 10.4274/raed.galenos.2022.69188 |
IEEE | Tuncer Sakar C,KARAKAYA G,Bilgin E,Kılıç L,Kalyoncu U "Predicting the response to bDMARD treatment in RA: Then what?." , ss.87 - 93, 2022. 10.4274/raed.galenos.2022.69188 |
ISNAD | Tuncer Sakar, Ceren vd. "Predicting the response to bDMARD treatment in RA: Then what?". (2022), 87-93. https://doi.org/10.4274/raed.galenos.2022.69188 |
APA | Tuncer Sakar C, KARAKAYA G, Bilgin E, Kılıç L, Kalyoncu U (2022). Predicting the response to bDMARD treatment in RA: Then what?. Ulusal Romatoloji Dergisi, 14(2), 87 - 93. 10.4274/raed.galenos.2022.69188 |
Chicago | Tuncer Sakar Ceren,KARAKAYA GULSAH,Bilgin Emre,Kılıç Levent,Kalyoncu Umut Predicting the response to bDMARD treatment in RA: Then what?. Ulusal Romatoloji Dergisi 14, no.2 (2022): 87 - 93. 10.4274/raed.galenos.2022.69188 |
MLA | Tuncer Sakar Ceren,KARAKAYA GULSAH,Bilgin Emre,Kılıç Levent,Kalyoncu Umut Predicting the response to bDMARD treatment in RA: Then what?. Ulusal Romatoloji Dergisi, vol.14, no.2, 2022, ss.87 - 93. 10.4274/raed.galenos.2022.69188 |
AMA | Tuncer Sakar C,KARAKAYA G,Bilgin E,Kılıç L,Kalyoncu U Predicting the response to bDMARD treatment in RA: Then what?. Ulusal Romatoloji Dergisi. 2022; 14(2): 87 - 93. 10.4274/raed.galenos.2022.69188 |
Vancouver | Tuncer Sakar C,KARAKAYA G,Bilgin E,Kılıç L,Kalyoncu U Predicting the response to bDMARD treatment in RA: Then what?. Ulusal Romatoloji Dergisi. 2022; 14(2): 87 - 93. 10.4274/raed.galenos.2022.69188 |
IEEE | Tuncer Sakar C,KARAKAYA G,Bilgin E,Kılıç L,Kalyoncu U "Predicting the response to bDMARD treatment in RA: Then what?." Ulusal Romatoloji Dergisi, 14, ss.87 - 93, 2022. 10.4274/raed.galenos.2022.69188 |
ISNAD | Tuncer Sakar, Ceren vd. "Predicting the response to bDMARD treatment in RA: Then what?". Ulusal Romatoloji Dergisi 14/2 (2022), 87-93. https://doi.org/10.4274/raed.galenos.2022.69188 |