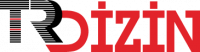
Unsupervised Learning Approach for Detection and Localization of Structural Damage using Output-only Measurements
Yıl: 2022 Cilt: 10 Sayı: 3 Sayfa Aralığı: 149 - 156 Metin Dili: İngilizce DOI: 10.21541/apjess.1100238 İndeks Tarihi: 21-09-2022
Unsupervised Learning Approach for Detection and Localization of Structural Damage using Output-only Measurements
Öz: Interrogation of the vibration data collected from the sensors embedded throughout the structure without relying on a finite element model of the system for monitoring the health of structural systems has received significant attention in the recent years especially with the current advancements in sensor technology. The data-driven methods explored within this context falls into the realm of statistical pattern recognition field requiring extraction of damage detection features and a statistical decision- making process for identification of damage. Machine learning algorithms provide statistical means for making such decisions. In this study, an unsupervised machine learning approach, one-class support vector machine (OC-SVM), requiring training data only from the undamaged state of the structure is explored for damage detection purposes. The coefficients of the autoregressive (AR) model are extracted as damage sensitive features and used as the required training data. The trained classifier is then used with the data obtained from the same structure at different damage states for classification. Damage detection in the form of recognizing outliers or anomalies not belonging to the target class, is followed by damage localization within the given sensor resolution using statistical means. To this end, Itakura distance measuring the distance between two sets of linear predictor coefficients of the AR processes, is utilized as damage location indicator. Numerical simulations are performed on a truss and a beam structure with several damage scenarios including realistic levels of measurement noise and modeling error. Results show that the proposed approach can successfully detect existence of damage and the statistical measure shows promising performance for further localization of the damaged region.
Anahtar Kelime: Belge Türü: Makale Makale Türü: Araştırma Makalesi Erişim Türü: Erişime Açık
- [1] A. Rytter, Vibrational Based Inspection of Civil Engineering Structures. University of Aalborg, 1993.
- [2] R. J. Barthorpe, On model-and data-based approaches to structural health monitoring (Doctoral dissertation), University of Sheffield, 2010.
- [3] H. Sohn, C. R. Farrar, H. F. Hunter, K. Worden, Applying the LANL Statistical Pattern Recognition Paradigm for Structural Health Monitoring to Data from a Surface-Effect Fast Petrol Boat. Los Alamos National Laboratory Report LA-13761-MS. Los Alamos National Laboratory, Los Alamos, NM, 2001.
- [4] H. Sohn and C. R. Farrar, “Damage diagnosis using time series analysis of vibration signals.” Smart Materials and Structures, vol. 10, no. 3, pp. 446-451, 2001, doi: 10.1088/0964-1726/10/3/304.
- [5] J. N. Wandji, “A nonparametric goodness-of-fit test for a class of parametric autoregressive models.” Journal of Statistical Planning and Inference, vol. 71, no. 1, pp. 57-74, 1998, doi: 10.1016/s0378-3758(98)00010-x.
- [6] S. G. Mattson and S. M. Pandit, “Statistical moments of autoregressive model residuals for damage localisation.” Mechanical Systems and Signal Processing, vol. 20, no. 3, pp. 627-645, 2006, doi: 10.1016/j.ymssp.2004.08.005.
- [7] K. K. Nair, A. S. Kiremidjian, and K. H. Law, “Time series-based damage detection and localization algorithm with application to the ASCE benchmark structure.” Journal of Sound and Vibration, vol. 291, no. 1, pp. 349-368, 2006, doi: 10.1016/j.jsv.2005.06.016.
- [8] C. Kar and A. Mohanty, “Monitoring gear vibrations through motor current signature analysis and wavelet transform.” Mechanical Systems and Signal Processing, vol. 20, no. 1, pp. 158-187, 2006, doi: 10.1016/j.ymssp.2004.07.006.
- [9] H. Zheng and A. Mita, “Two-stage damage diagnosis based on the distance between ARMA models and pre- whitening filters.” Smart Materials and Structures, vol. 16, no. 5, pp. 1829-1836, 2007, doi: 10.1088/0964- 1726/16/5/038.
- [10] K. Krishnan Nair and A. S. Kiremidjian, “Time Series Based Structural Damage Detection Algorithm Using Gaussian Mixtures Modeling.” Journal of Dynamic Systems, Measurement, and Control, vol. 129, no. 3, pp. 285-293, 2006, doi: 10.1115/1.2718241.
- [11] M. Gul and F. N. Catbas, “Damage Assessment with Ambient Vibration Data Using a Novel Time Series Analysis Methodology.” Journal of Structural Engineering, vol. 137, no. 12, pp. 1518-1526, 2011, doi: 10.1061/(asce)st.1943-541x.0000366.
- [12] K. Roy, B. Bhattacharya, and S. Ray-Chaudhuri, “ARX model-based damage sensitive features for structural damage localization using output-only measurements.” Journal of Sound and Vibration, vol. 349, pp. 99-122, 2015, doi: 10.1016/j.jsv.2015.03.038.
- [13] A. Entezami and H. Shariatmadar, “An unsupervised learning approach by novel damage indices in structural health monitoring for damage localization and quantification.” Structural Health Monitoring, vol. 17, no. 2, pp. 325-345, 2017, doi: 10.1177/1475921717693572.
- [14] K. Worder, G. Manson, and N. Fieller, “Damage detection using outlier analysis.” Journal of Sound and Vibration, vol. 229, no. 3, pp. 647-667, 2000, doi: 10.1006/jsvi.1999.2514.
- [15] M. Flah, I. Nunez, W. Ben Chaabene, and M. L. Nehdi, “Machine Learning Algorithms in Civil Structural Health Monitoring: A Systematic Review.” Archives of Computational Methods in Engineering, vol. 28, no. 4, pp. 2621-2643, 2020, doi: 10.1007/s11831-020-09471- 9.
- [16] O. Avci, O. Abdeljaber, S. Kiranyaz, M. Hussein, M. Gabbouj, and D. J. Inman, “A review of vibration- based damage detection in civil structures: From traditional methods to Machine Learning and Deep Learning applications.” Mechanical Systems and Signal Processing, vol. 147, p. 107077, 2021, doi: 10.1016/j.ymssp.2020.107077.
- [17] F. Itakura, “Minimum prediction residual principle applied to speech recognition.” IEEE Transactions on Acoustics, Speech, and Signal Processing, vol. 23, no. 1, pp. 67-72, 1975, doi: 10.1109/tassp.1975.1162641.
- [18] G. E. Box, G. M. Jenkins, G. C. Reinsel, Time series analysis: Forecasting and control, Prentice-Hall, Upper Saddle River, NJ, 1994.
- [19] L. Ljung, L. System identification: Theory for the user, Prentice-Hall, Upper Saddle River, NJ, 1999.
- [20] B. Schölkopf, R. C. Williamson, A. Smola, A., J. Shawe-Taylor, and J. Platt, “Support vector method for novelty detection.” Advances in neural information processing systems, vol. 12, pp. 582–588, 1999.
- [21] B. Schölkopf, A. J. Smola, and F. Bach, Learning with kernels: support vector machines, regularization, optimization, and beyond. MIT press, 2002.
- [22] Q. Zhang, “Statistical damage identification for bridges using ambient vibration data.” Computers & Structures, vol. 85, no. 7, pp. 476-485, 2007, doi: 10.1016/j.compstruc.2006.08.071.
- [23] M. Gul and F. N. Catbas, “Structural health monitoring and damage assessment using a novel time series analysis methodology with sensor clustering.” Journal of Sound and Vibration, vol. 330, no. 6, pp. 1196-1210, 2011, doi: 10.1016/j.jsv.2010.09.024..
- [24] F. N. Catbas, H. B. Gokce, and M. Gul, “Nonparametric analysis of structural health monitoring data for identification and localization of changes: Concept, lab, and real-life studies.” Structural Health Monitoring, vol. 11, no. 5, pp. 613-626, 2012, doi: 10.1177/1475921712451955.
- [25] A. Mosavi, D. Dickey, R. Seracino, and S. Rizkalla, “Identifying damage locations under ambient vibrations utilizing vector autoregressive models and Mahalanobis distances.” Mechanical Systems and Signal Processing, vol. 26, pp. 254-267, 2012, doi: 10.1016/j.ymssp.2011.06.009.
- [26] H. Zheng and A. Mita, “Localized Damage Detection of Structures Subject to Multiple Ambient Excitations Using Two Distance Measures for Autoregressive Models.” Structural Health Monitoring, vol. 8, no. 3, pp. 207-222, 2009, doi: 10.1177/1475921708102145.
APA | Gunes B (2022). Unsupervised Learning Approach for Detection and Localization of Structural Damage using Output-only Measurements. , 149 - 156. 10.21541/apjess.1100238 |
Chicago | Gunes Burcu Unsupervised Learning Approach for Detection and Localization of Structural Damage using Output-only Measurements. (2022): 149 - 156. 10.21541/apjess.1100238 |
MLA | Gunes Burcu Unsupervised Learning Approach for Detection and Localization of Structural Damage using Output-only Measurements. , 2022, ss.149 - 156. 10.21541/apjess.1100238 |
AMA | Gunes B Unsupervised Learning Approach for Detection and Localization of Structural Damage using Output-only Measurements. . 2022; 149 - 156. 10.21541/apjess.1100238 |
Vancouver | Gunes B Unsupervised Learning Approach for Detection and Localization of Structural Damage using Output-only Measurements. . 2022; 149 - 156. 10.21541/apjess.1100238 |
IEEE | Gunes B "Unsupervised Learning Approach for Detection and Localization of Structural Damage using Output-only Measurements." , ss.149 - 156, 2022. 10.21541/apjess.1100238 |
ISNAD | Gunes, Burcu. "Unsupervised Learning Approach for Detection and Localization of Structural Damage using Output-only Measurements". (2022), 149-156. https://doi.org/10.21541/apjess.1100238 |
APA | Gunes B (2022). Unsupervised Learning Approach for Detection and Localization of Structural Damage using Output-only Measurements. Academic Platform journal of engineering and smart systems (Online), 10(3), 149 - 156. 10.21541/apjess.1100238 |
Chicago | Gunes Burcu Unsupervised Learning Approach for Detection and Localization of Structural Damage using Output-only Measurements. Academic Platform journal of engineering and smart systems (Online) 10, no.3 (2022): 149 - 156. 10.21541/apjess.1100238 |
MLA | Gunes Burcu Unsupervised Learning Approach for Detection and Localization of Structural Damage using Output-only Measurements. Academic Platform journal of engineering and smart systems (Online), vol.10, no.3, 2022, ss.149 - 156. 10.21541/apjess.1100238 |
AMA | Gunes B Unsupervised Learning Approach for Detection and Localization of Structural Damage using Output-only Measurements. Academic Platform journal of engineering and smart systems (Online). 2022; 10(3): 149 - 156. 10.21541/apjess.1100238 |
Vancouver | Gunes B Unsupervised Learning Approach for Detection and Localization of Structural Damage using Output-only Measurements. Academic Platform journal of engineering and smart systems (Online). 2022; 10(3): 149 - 156. 10.21541/apjess.1100238 |
IEEE | Gunes B "Unsupervised Learning Approach for Detection and Localization of Structural Damage using Output-only Measurements." Academic Platform journal of engineering and smart systems (Online), 10, ss.149 - 156, 2022. 10.21541/apjess.1100238 |
ISNAD | Gunes, Burcu. "Unsupervised Learning Approach for Detection and Localization of Structural Damage using Output-only Measurements". Academic Platform journal of engineering and smart systems (Online) 10/3 (2022), 149-156. https://doi.org/10.21541/apjess.1100238 |