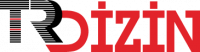
Prediction of Breast Cancer Distant Metastasis by Artificial Intelligence Methods from an Epidemiological Perspective
Yıl: 2022 Cilt: 23 Sayı: 3 Sayfa Aralığı: 210 - 215 Metin Dili: İngilizce DOI: 10.4274/imj.galenos.2022.62443 İndeks Tarihi: 22-09-2022
Prediction of Breast Cancer Distant Metastasis by Artificial Intelligence Methods from an Epidemiological Perspective
Öz: Introduction: Despite significant advances in breast cancer (BC) management, the prognosis for most patients with distant metastasis remains poor. We predicted distant metastasis in BC patients with artificial intelligence (AI) methods based on genomic biomarkers. Methods: The dataset used in the study included 97 patients with BC, of whom 46 (47%) developed distant metastases, and 51 (53%) did not develop distant metastases, and the expression level of 24,481 genes of these patients. An approach combining Boruta + LASSO methods was applied to identify biomarker genes associated with BC distant metastasis. Mann-Whitney U test was used to examine the difference between groups in terms of gene expression levels in statistical analyses, and Cohen d effect sizes and odds ratios were calculated. AdaBoost and XGBoost algorithms, which are tree-based methods, were used for BC distant metastasis prediction, and the results were compared by evaluating comprehensive performance criteria. Results: After Boruta + LASSO methods, 14 biomarker candidate genes were identified. These predictive genes were PIB5PA, SSX2, OR1F1, ALDH4A1, FGF18, WISP1, PRAME, CEGP1, AL080059, NMU, ATP5E, SMARCE1, FGD6, and SLC37A1. In effect size results; in particular, show that the AL080059 (Cohen’s D: 1.318) gene is clinically predictive of BC Metastasis. The accuracy, F1-score, positive predictive value, sensitivity, and area under the ROC Curve (AUC) values obtained with the AdaBoost algorithm for BC metastasis prediction was 95%, 96.3%, 100%, 92.6%, and 98.8%, respectively. The model created with the XGBoost algorithm, on the other hand, obtained 90%, 92.9%, 92.9%, 92.9%, 97.6% accuracy, F1-score, positive predictive value, sensitivity, and AUC values, respectively. Conclusion: Identifying genes that successfully predict BC distant metastasis with AI methods in the study may be decisive for future therapeutic targets and help clinicians better adapt adjuvant chemotherapy to their patients. Additionally, the AdaBoost prediction model created can discriminate patients at risk of BC distant metastases.
Anahtar Kelime: Belge Türü: Makale Makale Türü: Araştırma Makalesi Erişim Türü: Erişime Açık
- 1. Sung H, Ferlay J, Siegel RL, Laversanne M, Soerjomataram I, Jemal A, et al. Global Cancer Statistics 2020: GLOBOCAN Estimates of Incidence and Mortality Worldwide for 36 Cancers in 185 Countries. CA Cancer J Clin 2021; 71: 209-49.
- 2. Rojas K, Stuckey A. Breast Cancer Epidemiology and Risk Factors. Clin Obstet Gynecol 2016; 59: 651-72.
- 3. Momenimovahed Z, Salehiniya H. Epidemiological characteristics of and risk factors for breast cancer in the world. Breast Cancer (Dove Med Press) 2019; 11: 151-64.
- 4. Sun YS, Zhao Z, Yang ZN, Xu F, Lu HJ, Zhu ZY, et al. Risk Factors and Preventions of Breast Cancer. Int J Biol Sci 2017; 13: 1387-97.
- 5. Kwong A, Chen JW, Shin VY. A new paradigm of genetic testing for hereditary breast/ovarian cancers. Hong Kong Med J 2016; 22: 171-7.
- 6. Tevaarwerk AJ, Gray RJ, Schneider BP, Smith ML, Wagner LI, Fetting JH, et al. Survival in patients with metastatic recurrent breast cancer after adjuvant chemotherapy: little evidence of improvement over the past 30 years. Cancer 2013; 119: 1140-8.
- 7. Yates LR, Knappskog S, Wedge D, Farmery JHR, Gonzalez S, Martincorena I, et al. Genomic Evolution of Breast Cancer Metastasis and Relapse. Cancer Cell 2017; 32: 169-84.
- 8. Holleczek B, Stegmaier C, Radosa JC, Solomayer EF, Brenner H. Risk of loco- regional recurrence and distant metastases of patients with invasive breast cancer up to ten years after diagnosis - results from a registry-based study from Germany. BMC Cancer 2019; 19: 520.
- 9. Belkacemi Y, Hanna NE, Besnard C, Majdoul S, Gligorov J. Local and Regional Breast Cancer Recurrences: Salvage Therapy Options in the New Era of Molecular Subtypes. Front Oncol 2018; 8: 112.
- 10. Anwar SL, Avanti WS, Nugroho AC, Choridah L, Dwianingsih EK, Harahap WA, et al. Risk factors of distant metastasis after surgery among different breast cancer subtypes: a hospital-based study in Indonesia. World J Surg Oncol 2020; 18: 117.
- 11. Harris LN, Ismaila N, McShane LM, Andre F, Collyar DE, Gonzalez-Angulo AM, et al. Use of Biomarkers to Guide Decisions on Adjuvant Systemic Therapy for Women With Early-Stage Invasive Breast Cancer: American Society of Clinical Oncology Clinical Practice Guideline. J Clin Oncol 2016; 34: 1134-50.
- 12. De Mattos-Arruda L, Weigelt B, Cortes J, Won HH, Ng CKY, Nuciforo P, et al. Capturing intra-tumor genetic heterogeneity by de novo mutation profiling of circulating cell-free tumor DNA: a proof-of-principle. Ann Oncol 2014; 25: 1729-35.
- 13. Savas P, Teo ZL, Lefevre C, Flensburg C, Caramia F, Alsop K, et al. The Subclonal Architecture of Metastatic Breast Cancer: Results from a Prospective Community-Based Rapid Autopsy Program “CASCADE”. PLoS Med 2016; 13: e1002204.
- 14. Yue W, Wang Z, Chen H, Payne A, Liu X. Machine learning with applications in breast cancer diagnosis and prognosis. Designs 2018; 2: 13.
- 15. Vaka AR, Soni B, Reddy S. Breast cancer detection by leveraging Machine Learning. ICT Express 2020; 6: 320-4.
- 16. van ‘t Veer LJ, Dai H, van de Vijver MJ, He YD, Hart AA, Mao M, et al. Gene expression profiling predicts clinical outcome of breast cancer. Nature 2002; 415: 530-6.
- 17. Cevallos M, Egger M. STROBE (STrengthening the Reporting of OBservational studies in Epidemiology). Guidelines for reporting health research: a user’s manual; 2014. pp. 169-79.
- 18. Ed. Cohen J. “The earth is round (p<.05),” What if there were no significance tests? 2016; 69-82.
- 19. Obi N, Werner S, Thelen F, Becher H, Pantel K. Metastatic Breast Cancer Recurrence after Bone Fractures. Cancers (Basel) 2022; 14: 601.
- 20. Greve KB, Lindgreen JN, Terp MG, Pedersen CB, Schmidt S, Mollenhauer J, et al. Ectopic expression of cancer/testis antigen SSX2 induces DNA damage and promotes genomic instability. Mol Oncol 2015; 9: 437-49.
- 21. Liu Y, Baglia M, Zheng Y, Blot W, Bao PP, Cai H, et al. ALDH1A1 mRNA expression in association with prognosis of triple-negative breast cancer. Oncotarget 2015; 6: 41360-9.
- 22. Marcato P, Dean CA, Pan D, Araslanova R, Gillis M, Joshi M, et al. Aldehyde dehydrogenase activity of breast cancer stem cells is primarily due to isoform ALDH1A3 and its expression is predictive of metastasis. Stem Cells 2011; 29: 32-45.
- 23. Song N, Zhong J, Hu Q, Gu T, Yang B, Zhang J, et al. FGF18 enhances migration and the epithelial-mesenchymal transition in breast cancer by regulating Akt/ GSK3β/β-catenin signaling. Cell Physiol Biochem 2018; 49: 1019-32.
- 24. Chen J, Yin J, Li X, Wang Y, Zheng Y, Qian C, et al. WISP1 polymorphisms contribute to platinum-based chemotherapy toxicity in lung cancer patients. Int J Mol Sci 2014; 15: 21011-27.
- 25. Lin YH, Hsiao YH, Yang SF, Liu YF, Hsu CF, Wang PH. Association between genetic polymorphisms of WNT1 inducible signaling pathway protein 1 and uterine cervical cancer. Reprod Sci 2018; 25: 1549-56.
- 26. Chen CT, Lee HL, Chiou HL, Chou CH, Wang PH, Yang SF, et al. Impacts of WNT1-inducible signaling pathway protein 1 polymorphism on hepatocellular carcinoma development. PLoS One 2018; 13: e0198967.
- 27. Wang Y, Yang SH, Hsu PW, Chien SY, Wang CQ, Su CM, et al. Impact of WNT1- inducible signaling pathway protein-1 (WISP-1) genetic polymorphisms and clinical aspects of breast cancer. Medicine (Baltimore) 2019; 98:e17854.
- 28. Sokol ES, Feng YX, Jin DX, Tizabi MD, Miller DH, Cohen MA, et al. SMARCE1 is required for the invasive progression of in situ cancers. Proc Natl Acad Sci U S A 2017; 114: 4153-8.
- 29. Epping MT, Hart AA, Glas AM, Krijgsman O, Bernards R. PRAME expression and clinical outcome of breast cancer. Br J Cancer 2008; 99: 398-403.
- 30. Lu Y, Tong Y, Huang J, Lin L, Wu J, Fei X, et al. Diverse Distribution and Gene Expression on the 21-Gene Recurrence Assay in Breast Cancer Patients with Locoregional Recurrence Versus Distant Metastasis. Cancer Manag Res. 2021; 13: 6279-89.
- 31. Song Q, Jing H, Wu H, Zou B, Zhou G, Kambara H. Comparative Gene Expression Analysis of Breast Cancer-Related Genes by Multiplex Pyrosequencing Coupled with Sequence Barcodes. Advances and Clinical Practice in Pyrosequencing. Springer; 2016. pp. 315-25.
- 32. Galber C, Acosta MJ, Minervini G, Giorgio V. The role of mitochondrial ATP synthase in cancer. Biol Chem 2020; 401: 1199-214.
- 33. Jones JB, Song JJ, Hempen PM, Parmigiani G, Hruban RH, Kern SE. Detection of mitochondrial DNA mutations in pancreatic cancer offers a “mass”-ive advantage over detection of nuclear DNA mutations. Cancer Res 2001; 61: 1299-304.
- 34. Máximo V, Soares P, Lima J, Cameselle-Teijeiro J, Sobrinho-Simoes M. Mitochondrial DNA somatic mutations (point mutations and large deletions) and mitochondrial DNA variants in human thyroid pathology: a study with emphasis on Hürthle cell tumors. Am J Pathol 2002; 160: 1857-65.
- 35. Jiménez-Morales S, Pérez-Amado CJ, Langley E, Hidalgo-Miranda A. Overview of mitochondrial germline variants and mutations in human disease: Focus on breast cancer (Review) Int J Oncol 2018; 53: 923-36.
- 36. Wu S, Akhtari M, Alachkar H. Characterization of mutations in the mitochondrial encoded electron transport chain complexes in acute myeloid leukemia. Sci Rep 2018; 8: 13301.
- 37. Grzybowska-Szatkowska L, Slaska B, Rzymowska J, Brzozowska A, Floriańczyk B. Novel mitochondrial mutations in the ATP6 and ATP8 genes in patients with breast cancer. Mol Med Rep 2014; 10: 1772-8.
- 38. Garczyk S, Klotz N, Szczepanski S, Denecke B, Antonopoulos W, von Stillfried S, et al. Oncogenic features of neuromedin U in breast cancer are associated with NMUR2 expression involving crosstalk with members of the WNT signaling pathway. Oncotarget 2017; 8: 36246-65.
- 39. Zeng J, Li M, Shi H, Guo J. Upregulation of FGD6 predicts poor prognosis in gastric cancer. Front Med (Lausanne) 2021; 8: 672595.
- 40. Hameed SS, Hassan R, Hassan WH, Muhammadsharif FF, Latiff LA. HDG-select: A novel GUI based application for gene selection and classification in high dimensional datasets. PloS One 2021; 16: e0246039.
- 41. Zemmour C, Bertucci F, Finetti P, Chetrit B, Birnbaum D, Filleron T, et al. Prediction of early breast cancer metastasis from DNA microarray data using high-dimensional cox regression models. Cancer Inform 2015; 14:(Suppl 2): 129-38.
APA | Akbulut S, YAĞIN F, ÇOLAK C (2022). Prediction of Breast Cancer Distant Metastasis by Artificial Intelligence Methods from an Epidemiological Perspective. , 210 - 215. 10.4274/imj.galenos.2022.62443 |
Chicago | Akbulut Sami,YAĞIN Fatma Hilal,ÇOLAK Cemil Prediction of Breast Cancer Distant Metastasis by Artificial Intelligence Methods from an Epidemiological Perspective. (2022): 210 - 215. 10.4274/imj.galenos.2022.62443 |
MLA | Akbulut Sami,YAĞIN Fatma Hilal,ÇOLAK Cemil Prediction of Breast Cancer Distant Metastasis by Artificial Intelligence Methods from an Epidemiological Perspective. , 2022, ss.210 - 215. 10.4274/imj.galenos.2022.62443 |
AMA | Akbulut S,YAĞIN F,ÇOLAK C Prediction of Breast Cancer Distant Metastasis by Artificial Intelligence Methods from an Epidemiological Perspective. . 2022; 210 - 215. 10.4274/imj.galenos.2022.62443 |
Vancouver | Akbulut S,YAĞIN F,ÇOLAK C Prediction of Breast Cancer Distant Metastasis by Artificial Intelligence Methods from an Epidemiological Perspective. . 2022; 210 - 215. 10.4274/imj.galenos.2022.62443 |
IEEE | Akbulut S,YAĞIN F,ÇOLAK C "Prediction of Breast Cancer Distant Metastasis by Artificial Intelligence Methods from an Epidemiological Perspective." , ss.210 - 215, 2022. 10.4274/imj.galenos.2022.62443 |
ISNAD | Akbulut, Sami vd. "Prediction of Breast Cancer Distant Metastasis by Artificial Intelligence Methods from an Epidemiological Perspective". (2022), 210-215. https://doi.org/10.4274/imj.galenos.2022.62443 |
APA | Akbulut S, YAĞIN F, ÇOLAK C (2022). Prediction of Breast Cancer Distant Metastasis by Artificial Intelligence Methods from an Epidemiological Perspective. İstanbul Medical Journal, 23(3), 210 - 215. 10.4274/imj.galenos.2022.62443 |
Chicago | Akbulut Sami,YAĞIN Fatma Hilal,ÇOLAK Cemil Prediction of Breast Cancer Distant Metastasis by Artificial Intelligence Methods from an Epidemiological Perspective. İstanbul Medical Journal 23, no.3 (2022): 210 - 215. 10.4274/imj.galenos.2022.62443 |
MLA | Akbulut Sami,YAĞIN Fatma Hilal,ÇOLAK Cemil Prediction of Breast Cancer Distant Metastasis by Artificial Intelligence Methods from an Epidemiological Perspective. İstanbul Medical Journal, vol.23, no.3, 2022, ss.210 - 215. 10.4274/imj.galenos.2022.62443 |
AMA | Akbulut S,YAĞIN F,ÇOLAK C Prediction of Breast Cancer Distant Metastasis by Artificial Intelligence Methods from an Epidemiological Perspective. İstanbul Medical Journal. 2022; 23(3): 210 - 215. 10.4274/imj.galenos.2022.62443 |
Vancouver | Akbulut S,YAĞIN F,ÇOLAK C Prediction of Breast Cancer Distant Metastasis by Artificial Intelligence Methods from an Epidemiological Perspective. İstanbul Medical Journal. 2022; 23(3): 210 - 215. 10.4274/imj.galenos.2022.62443 |
IEEE | Akbulut S,YAĞIN F,ÇOLAK C "Prediction of Breast Cancer Distant Metastasis by Artificial Intelligence Methods from an Epidemiological Perspective." İstanbul Medical Journal, 23, ss.210 - 215, 2022. 10.4274/imj.galenos.2022.62443 |
ISNAD | Akbulut, Sami vd. "Prediction of Breast Cancer Distant Metastasis by Artificial Intelligence Methods from an Epidemiological Perspective". İstanbul Medical Journal 23/3 (2022), 210-215. https://doi.org/10.4274/imj.galenos.2022.62443 |