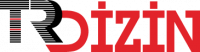
Artificial Intelligence-based Colon Cancer Prediction by Identifying Genomic Biomarkers
Yıl: 2022 Cilt: 4 Sayı: 2 Sayfa Aralığı: 196 - 202 Metin Dili: İngilizce DOI: 10.37990/medr.1077024 İndeks Tarihi: 27-09-2022
Artificial Intelligence-based Colon Cancer Prediction by Identifying Genomic Biomarkers
Öz: Aim: Colon cancer is the third most common type of cancer worldwide. Because of the poor prognosis and unclear preoperative staging, genetic biomarkers have become more important in the diagnosis and treatment of the disease. In this study, we aimed to determine the biomarker candidate genes for colon cancer and to develop a model that can predict colon cancer based on these genes.Material and Methods: In the study, a dataset containing the expression levels of 2000 genes from 62 different samples (22 healthy and 40 tumor tissues) obtained by the Princeton University Gene Expression Project and shared in the figshare database was used. Data were summarized as mean ± standard deviation. Independent Samples T-Test was used for statistical analysis. The SMOTE method was applied before the feature selection to eliminate the class imbalance problem in the dataset. The 13 most important genes that may be associated with colon cancer were selected with the LASSO feature selection method. Random Forest (RF), Decision Tree (DT), and Gaussian Naive Bayes methods were used in the modeling phase.Results: All 13 genes selected by LASSO had a statistically significant difference between normal and tumor samples. In the model created with RF, all the accuracy, specificity, f1-score, sensitivity, negative and positive predictive values were calculated as 1. The RF method offered the highest performance when compared to DT and Gaussian Naive Bayes.Conclusion: In the study, we identified the genomic biomarkers of colon cancer and classified the disease with a high-performance model. According to our results, it can be recommended to use the LASSO+RF approach when modeling high-dimensional microarray data.
Anahtar Kelime: Genomik Biyobelirteçleri Belirleyerek Yapay Zeka Tabanlı Kolon Kanseri Tahmini
Öz: Amaç: Kolon kanseri dünya genelinde en sık görülen üçüncü kanser türüdür. Kötü prognoz ve net olmayan preoperatif evreleme nedeniyle, hastalığın tanı ve tedavisinde genetik biyobelirteçler daha önemli hale gelmiştir. Bu çalışmada kolon kanseri için biyobelirteç adayı genlerin belirlenmesi ve bu genlere dayalı olarak kolon kanserini başarılı bir şekilde tahmin eden bir modelin geliştirilmesi amaçlanmıştır.Materyal ve Metot: Çalışmada, Princeton Üniversitesi Gen Ekspresyon Projesi ile elde edilen ve figshare veri tabanında paylaşılan 62 farklı örnekten (22 sağlıklı ve 40 tümör dokusu) 2000 genin ekspresyon düzeylerini içeren bir veri seti kullanıldı. Veriler ortalama ± standart sapma olarak özetlendi. İstatistiksel analizler için bağımsız örneklerde T-testi kullanıldı. Veri setindeki sınıf dengesizliği sorununu ortadan kaldırmak için öznitelik seçiminden önce SMOTE yöntemi uygulandı. Kolon kanseri ile ilişkili olabilecek en önemli 13 gen, LASSO öznitelik seçim yöntemi ile seçildi. Modelleme aşamasında Rastgele Orman (RF), Karar Ağacı (DT) ve Gauss naive Bayes yöntemleri kullanıldı.Bulgular: LASSO tarafından seçilen 13 genin tümü, normal ve tümör numuneleri arasında istatistiksel olarak anlamlı bir farka sahipti. RF ile oluşturulan modelde doğruluk, seçicilik, f1-skor, duyarlılık, negatif ve pozitif prediktif değerlerinin tümü 1 olarak hesaplanmıştır. DT ve Gaussian Naive Bayes ile karşılaştırıldığında RF yöntemi en yüksek performansı vermiştir.Sonuç: Çalışmada kolon kanserinin genomik biyobelirteçlerini belirledik ve hastalığı yüksek performanslı bir model ile sınıflandırdık. Elde ettiğimiz sonuçlara göre, yüksek boyutlu mikrodizi verilerinin modellenmesinde LASSO+RF yaklaşımının kullanılması önerilebilir.
Anahtar Kelime: Belge Türü: Makale Makale Türü: Araştırma Makalesi Erişim Türü: Erişime Açık
- 1. Globocan W. Estimated cancer incidence, mortality and prevalence worldwide in 2012. Int Agency Res Cancer. 2012.
- 2. Labianca R, Beretta G, Gatta G, et al. Colon cancer. Critical Reviews Oncology Hematology. 2004;51:145-70.
- 3. Loboda A, Nebozhyn MV, Watters JW, et al. EMT is the dominant program in human colon cancer. BMC Med Genomics. 2011;4:1-10.
- 4. Xu C, Meng LB, Duan YC, et al. Screening and identification of biomarkers for systemic sclerosis via microarray technology. Int J Molecular Med. 2019;44:1753-70.
- 5. Ahmad MA, Eckert C, Teredesai A. Interpretable machine learning in healthcare. Proceedings of the 2018 ACM international conference on bioinformatics, Computational Biology Health Informatics. 2018
- 6. Yagin FH, Yagin B, Arslan AK, Çolak C. Comparison of Performances of Associative Classification Methods for Cervical Cancer Prediction: Observational Study. Turkey Clinics J Biostatistics. 2021;13:13:266-72.
- 7. Khaire UM, Dhanalakshmi R. High-dimensional microarray dataset classification using an improved adam optimizer (iAdam). J Ambient Intelligence Humanized Computing. 2020;11:5187-204.
- 8. Hameed SS, Hassan R, Hassan WH, et al. HDG-select: A novel GUI based application for gene selection and classification in high dimensional datasets. PloS One. 2021;16:e0246039.
- 9. Mulla GA, Demir Y, Hassan M. Combination of PCA with SMOTE Oversampling for Classification of High- Dimensional Imbalanced Data. Bitlis Eren University Science and Technology Journal. 2021;10:858-69.
- 10. Güçkiran K, Cantürk İ, Özyilmaz L. DNA microarray gene expression data classification using SVM, MLP, and RF with feature selection methods relief and LASSO. Journal of Suleyman Demirel University Institute of Science and Technology. 2019;23:126-32.
- 11. Akyol K, Bayir Ş, Baha Ş. Importance of Attribute Selection for Parkinson Disease. Academic Platform J Engineering Sci. 2020;8:175-80.
- 12. Yilmaz R, Yagin FH. Early detection of coronary heart disease based on machine learning methods. Med Records. 2022;4:1-6.
- 13. Secgin Y, Oner Z, Turan MK, Oner S. Gender prediction with parameters obtained from pelvis computed tomography images and decision tree algorithm. Med Science. 2021;10:356-61
- 14. Doğan Ş, Türkoğlu İ. Hypothyroidi and hyperthyroidi detection from thyroid hormone parameters by using decision trees. Fırat University Journal of Oriental Studies. 2007;5:163-9.
- 15. Pulat M, Kocakoç ID. Machine Learning and Decision in Turkey. Bibliometric Analysis of Published Theses in the Field of Trees. Journal of Management and Economics. 2021;28:287-308.
- 16. Kamel H, Abdulah D, Al-Tuwaijari JM. Cancer classification using gaussian naive bayes algorithm. 2019 Int Engineering Conference (IEC); 2019:36:165-5.
- 17. Quackenbush J. Microarray analysis and tumor classification. New England J Med. 2006;354:2463-72.
- 18. Jose A. Gene selection by 1-d discrete wavelet transform for classifying cancer samples using dna microarray date. Ph.D. thesis, University of Akron, 2009.
- 19. Yan W, Bai Z, Wang J, et al. ANP32A modulates cell growth by regulating p38 and Akt activity in colorectal cancer. Oncology Reports. 2017;38:1605-12.
- 20. Velmurugan BK, Yeh K-T, Lee C-H, et al. Acidic leucine- rich nuclear phosphoprotein-32A (ANP32A) association with lymph node metastasis predicts poor survival in oral squamous cell carcinoma patients. Oncotarget. 2016;7:10879.
- 21. Liu Q, Tan Y, Huang T, et al. TF-centered downstream gene set enrichment analysis: Inference of causal regulators by integratingTF-DNAinteractionsandproteinpost-translational modifications information. BMC Bioinformatics. 2010;11:1- 17.
- 22. Mora JAM, Ordoñez FM, Bonilla DA. Improvement of k-means clustering algorithm performance in gene expression data analysis through pre-processing with principal component analysis and boosting. 2017;3:53-9.
- 23. Arentz G, Chataway T, Price TJ, et al. Desmin expression in colorectal cancer stroma correlates with advanced stage disease and marks angiogenic microvessels. Clinical Proteomics. 2011;8:1-13.
- 24. Bhunia S, Barbhuiya MA, Gupta S, et al. Epigenetic downregulation of desmin in gall bladder cancer reveals its potential role in disease progression. Indian J Med Research. 2020;151:311.
- 25. Chen H, Xu C, Qing’e Jin ZL. S100 protein family in human cancer. Am J Cancer Res. 2014;4:89.
- 26. Twal WO, Czirok A, Hegedus B, et al. Fibulin-1 suppression of fibronectin-regulated cell adhesion and motility. J Cell Sci. 2001;114:4587-98.
- 27. Xu Z, Chen H, Liu D, Huo J. Fibulin-1 is downregulated through promoter hypermethylation in colorectal cancer: a CONSORT study. Med (Baltimore). 2015;94.e663
- 28. Tong X, Mirzoeva S, Veliceasa D, et al. Chemopreventive apigenin controls UVB-induced cutaneous proliferation and angiogenesis through HuR and thrombospondin-1. Oncotarget. 2014;5:11413.
- 29. Ono C, Sato M, Taka H, et al. Tightly regulated expression of Autographa californica multicapsid nucleopolyhedrovirus immediate early genes emerges from their interactions and possible collective behaviors. Plos One. 2015;10:e0119580.
- 30. Strassburg CP, Kasai Y, Seng BA, et al. Baculovirus recombinant expressing a secreted form of a transmembrane carcinoma-associatedantigen.CancerRes.1992;52:815-21.
- 31. Loging WT, Reisman D. Elevated expression of ribosomal protein genes L37, RPP-1, and S2 in the presence of mutant p53. Cancer Epidemiology and Prevention Biomarkers. 1999;8:1011-6.
- 32. Golob-Schwarzl N, Schweiger C, Koller C, et al. Separation of low and high grade colon and rectum carcinoma by eukaryotic translation initiation factors 1, 5 and 6. Oncotarget. 2017;8:101224.
- 33. Oliveira P, Sanges R, Huntsman D, et al. Characterization of the intronic portion of cadherin superfamily members, common cancer orchestrators. European J Human Genetics. 2012;20:878-83.
- 34. Van Marck V, Stove C, Jacobs K, et al. Pcadherin in adhesion and invasion: Opposite roles in colon and bladder carcinoma. Int J Cancer. 2011;128:1031-44.
- 35. Takahashi K, Sasano H, Fukushima K, et al. 11 beta- hydroxysteroid dehydrogenase type II in human colon: a new marker of fetal development and differentiation in neoplasms. Anticancer Res. 1998;18:3381-8.
- 36. Baba Y, Nosho K, Shima K, et al. Prognostic significance of AMP-activated protein kinase expression and modifying effect of MAPK3/1 in colorectl cancer. British J Cancer. 2010;103:1025-33.
- 37. Esteve-Puig R, Canals F, Colome N, et al. Uncoupling of the LKB1-AMPKα energy sensor pathway by growth factors and oncogenic BRAFV600E. PloS One. 2009;4:e4771.
- 38. Zheng B, Jeong JH, Asara JM, et al. Oncogenic B-RAF negatively regulates the tumor suppressor LKB1 to promote melanoma cell proliferation. Molecular Cell. 2009;33:237-47.
- 39. Kim MJ, Park IJ, Yun H, et al. AMP-activated protein kinase antagonizes pro-apoptotic extracellular signal-regulated kinase activation by inducing dual-specificity protein phosphatases in response to glucose deprivation in HCT116 carcinoma. J Bio Chemistry. 2010;285:14617-27.
- 40. Arowolo MO, Isiaka RM, Abdulsalam SO, et al. A comparative analysis of feature extraction methods for classifying colon cancer microarray data. EAI Endorsed Transactions Scalable Information Systems. 2017;4:1-6.
- 41. Al Rajab M, Lu J, Xu Q. Examining applying high performance genetic data feature selection and classification algorithms for colon cancer diagnosis. Computer Methods Programs Bio Med. 2017;146:11-24.
APA | paksoy n, YAĞIN F (2022). Artificial Intelligence-based Colon Cancer Prediction by Identifying Genomic Biomarkers. , 196 - 202. 10.37990/medr.1077024 |
Chicago | paksoy nur,YAĞIN Fatma Hilal Artificial Intelligence-based Colon Cancer Prediction by Identifying Genomic Biomarkers. (2022): 196 - 202. 10.37990/medr.1077024 |
MLA | paksoy nur,YAĞIN Fatma Hilal Artificial Intelligence-based Colon Cancer Prediction by Identifying Genomic Biomarkers. , 2022, ss.196 - 202. 10.37990/medr.1077024 |
AMA | paksoy n,YAĞIN F Artificial Intelligence-based Colon Cancer Prediction by Identifying Genomic Biomarkers. . 2022; 196 - 202. 10.37990/medr.1077024 |
Vancouver | paksoy n,YAĞIN F Artificial Intelligence-based Colon Cancer Prediction by Identifying Genomic Biomarkers. . 2022; 196 - 202. 10.37990/medr.1077024 |
IEEE | paksoy n,YAĞIN F "Artificial Intelligence-based Colon Cancer Prediction by Identifying Genomic Biomarkers." , ss.196 - 202, 2022. 10.37990/medr.1077024 |
ISNAD | paksoy, nur - YAĞIN, Fatma Hilal. "Artificial Intelligence-based Colon Cancer Prediction by Identifying Genomic Biomarkers". (2022), 196-202. https://doi.org/10.37990/medr.1077024 |
APA | paksoy n, YAĞIN F (2022). Artificial Intelligence-based Colon Cancer Prediction by Identifying Genomic Biomarkers. Medical records-international medical journal (Online), 4(2), 196 - 202. 10.37990/medr.1077024 |
Chicago | paksoy nur,YAĞIN Fatma Hilal Artificial Intelligence-based Colon Cancer Prediction by Identifying Genomic Biomarkers. Medical records-international medical journal (Online) 4, no.2 (2022): 196 - 202. 10.37990/medr.1077024 |
MLA | paksoy nur,YAĞIN Fatma Hilal Artificial Intelligence-based Colon Cancer Prediction by Identifying Genomic Biomarkers. Medical records-international medical journal (Online), vol.4, no.2, 2022, ss.196 - 202. 10.37990/medr.1077024 |
AMA | paksoy n,YAĞIN F Artificial Intelligence-based Colon Cancer Prediction by Identifying Genomic Biomarkers. Medical records-international medical journal (Online). 2022; 4(2): 196 - 202. 10.37990/medr.1077024 |
Vancouver | paksoy n,YAĞIN F Artificial Intelligence-based Colon Cancer Prediction by Identifying Genomic Biomarkers. Medical records-international medical journal (Online). 2022; 4(2): 196 - 202. 10.37990/medr.1077024 |
IEEE | paksoy n,YAĞIN F "Artificial Intelligence-based Colon Cancer Prediction by Identifying Genomic Biomarkers." Medical records-international medical journal (Online), 4, ss.196 - 202, 2022. 10.37990/medr.1077024 |
ISNAD | paksoy, nur - YAĞIN, Fatma Hilal. "Artificial Intelligence-based Colon Cancer Prediction by Identifying Genomic Biomarkers". Medical records-international medical journal (Online) 4/2 (2022), 196-202. https://doi.org/10.37990/medr.1077024 |