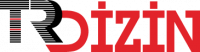
Early Detection of Coronary Heart Disease Based on Machine Learning Methods
Yıl: 2022 Cilt: 4 Sayı: 1 Sayfa Aralığı: 1 - 6 Metin Dili: İngilizce DOI: 10.37990/medr.1011924 İndeks Tarihi: 27-09-2022
Early Detection of Coronary Heart Disease Based on Machine Learning Methods
Öz: Aim: Heart disease detection using machine learning methods has been an outstanding research topic as heart diseases continue to be a burden on healthcare systems around the world. Therefore, in this study, the performances of machine learning methods for predictive classification of coronary heart disease were compared.Material and Method: In the study, three different models were created with Random Forest (RF), Logistic Regression (LR), and Support Vector Machine (SVM) algorithms for the classification of coronary heart disease. For hyper parameter optimization, 3-repeats 10-fold repeated cross validation method was used. The performance of the models was evaluated based on Accuracy, F1 Score, Specificity, Sensitivity, Positive Predictive Value, Negative Predictive Value, and Confusion Matrix (Classification matrix).Results: RF 0.929, SVM 0.897 and LR 0.861 classified coronary heart disease with accuracy. Specificity, Sensitivity, F1-score, Negative predictive and Positive predictive values of the RF model were calculated as 0.929, 0.928, 0.928, 0.929 and 0.928, respectively. The Sensitivity value of the SVM model was higher compared to the RF. Conclusion: Considering the accurate classification rates of Coronary Heart disease, the RF model outperformed the SVM and LR models. Also, the RF model had the highest sensitivity value. We think that this result, which has a high sensitivity criterion in order to minimize overlooked heart patients, is clinically very important.
Anahtar Kelime: Makine Öğrenme Yöntemlerine Dayali Kroner Kalp Hastaliğinin Erken Tespiti
Öz: Amaç: Kalp hastalıklarının dünya çapında sağlık sistemleri üzerinde bir yük olmaya devam etmesi nedeniyle, makine öğrenme yöntemlerini kullanarak kalp hastalığı tespiti olağanüstü bir araştırma konusu olmuştur. Bu nedenle, bu çalışmada, koroner kalp hastalığının tahmin edici sınıflandırması için makine öğrenme yöntemlerinin performansları karşılaştırılmıştır. Materyal ve Metot: Çalışmada koroner kalp hastalığının sınıflandırılması için Rasgele Orman (RF), Lojistik Regresyon (LR) ve Destek Vektör Makinesi (SVM) algoritmaları ile üç farklı model oluşturulmuştur. Hiperparametre optimizasyonu için 3 tekrarlı 10 katlı tekrarlı çapraz doğrulama yöntemi kullanıldı. Modellerin performansı Doğruluk, F1 Skoru, Seçicilik, Duyarlılık, Pozitif Tahmin Değeri, Negatif Tahmin Değeri ve Karışıklık Matrisi (Sınıflandırma matrisi) temel alınarak değerlendirilmiştir. Bulgular: Koroner kalp hastalığını RF 0.929, SVM 0.897 ve LR 0.861 doğrulukla sınıflandırdı. RF modelinin seçicilik, duyarlılık, F1-skor, negatif tahmin ve pozitif tahmin değerleri sırasıyla 0.929, 0.928, 0.928, 0.929 ve 0.928 olarak hesaplanmıştır. Ek olarak SVM modelinin duyarlılık değeri RF’ye göre daha yüksek çıkmıştır. Sonuç: Koroner Kalp hastalığının doğru sınıflandırma oranları göz önüne alındığında, RF modeli SVM ve LR modellerinden daha iyi performans göstermiştir. Ayrıca RF modeli en yüksek duyarlılık değerine sahipti. Gözden kaçırılan kalp hastalarını en aza indirmek için yüksek bir duyarlılık kriterine sahip olan bu sonucun klinik açıdan oldukça önemli olduğunu düşünmekteyiz.
Anahtar Kelime: Belge Türü: Makale Makale Türü: Araştırma Makalesi Erişim Türü: Erişime Açık
- 1. Watanabe T, Ando K, Daidoji H, et al. A randomized controlled trial of eicosapentaenoic acid in patients with coronary heart disease on statins. J Cardiol. 2017;70:537-44.
- 2. Paynter NP, Balasubramanian R, Giulianini F, et al. Metabolic predictors of incident coronary heart disease in women. Circulation. 2018;137:841-53.
- 3. Wolters FJ, Segufa RA, Darweesh SK, et al. Coronary heart disease, heart failure, and the risk of dementia: a systematic review and meta-analysis. Alzheimer’s & Dementia. 2018;14:1493-504.
- 4. Dogan MV, Grumbach IM, Michaelson JJ, et al. Integrated genetic and epigenetic prediction of coronary heart disease in the Framingham Heart Study. PloS One. 2018;13:0190549.
- 5. KayaÖÜMO.Performance evaluation ofmultilayer perceptron artificial neural network model in the classification of heart failure. J Cognitive Systems. 2021;6:35-8.
- 6. Shameer K, Johnson KW, Glicksberg BS, et al. Machine learning in cardiovascular medicine: are we there yet? Heart. 2018;104:1156-64.
- 7. Birjandi SM, Khasteh SH. A survey on data mining techniques used in medicine. J Diabetes Metabol Disorders. 2021:1-17.
- 8. Kucukakcali Z, Balikçi Çiçek İ, Güldoğan E, et al. Assessment of associative classification approach for predicting mortality by heart failure. J Cognitive Systems. 2020;5:41-5.
- 9. Balikçi Çiçek İ, Küçükakçali Z, Çolak C. Associative classification approach can predict prostate cancer based on the extracted association rules. J Cognitive Systems. 2020;5:51-4.
- 10. Balikçi Çiçek İ, Küçükakçali Z. Classification Of Hypothyroid Disease With Extreme Learning Machine Model. The Journal of Cognitive Systems. 2020;5:64-8.
- 11. Küçükakçali Z, Balikçi Çiçek İ. Performance evaluation of the ensemble learning models in the classification of chronic kidney failure. J Cognitive Systems. 2020;5:55-9.
- 12. Arslan AK, Kucukakcali Z, Balikçi Çiçek İ, et al. A novel interpretable web-based tool on the associative classification methods: an application on breast cancer dataset. J Cognitive Systems. 2020;5:33-40.
- 13. Balikçi Çiçek İ, Küçükakçali Z. Classification of prostate cancer and determination of related factors with different artificial neural network. Middle Black Sea J Health Sci. 2020;6:325-32.
- 14. Küçükakçali Z, Balikçi Çiçek İ, Güldoğan E. Performance evaluation of the deep learning models in the classification of heart attack and determination of related factors. J Cognitive Systems. 2020;5:99-103.
- 15. Siddhartha M. Heart Disease Dataset (Comprehensive). Kaggle Inc. 2019.
- 16. Shah K, Patel H, Sanghvi D, et al. A comparative analysis of logistic regression, random forest and KNN models for the text classification. Augmented Human Res. 2020;5:1-16.
- 17. Kirasich K, Smith T, Sadler B. Random forest vs logistic regression: binary classification for heterogeneous datasets. SMU Data Science Review. 2018;1:9.
- 18. Pisner DA, Schnyer DM. Support vector machine. Machine Learning: Elsevier; 2020. p.101-21.
- 19. Probst P, Wright MN, Boulesteix AL. Hyperparameters and tuning strategies for random forest. wiley interdisciplinary reviews: data mining and knowledge discovery. 2019;9:1301.
- 20. Iwendi C, Bashir AK, Peshkar A, et al. COVID-19 patient health prediction using boosted random forest algorithm. Frontiers in Public Health. 2020;8:357.
- 21. Shah D, Patel S, Bharti SK. Heart disease prediction using machine learning techniques. SN Computer Science. 2020;1:1-6.
- 22. Kondababu A, Siddhartha V, Kumar BB, et al. A comparative study on machine learning based heart disease prediction. Materials Today: Proceedings. 2021.
- 23. Bahrami B, Shirvani MH. Prediction and diagnosis of heart disease by data mining techniques. JMultidisciplinary Engineering Sci Technol (JMEST). 2015;2:164-8.
- 24. Ashari A, Paryudi I, Tjoa AM. Performance comparison between Naïve Bayes, decision tree and k-nearest neighbor in searching alternative design in an energy simulation tool. International Journal of Advanced Computer Science and Applications (IJACSA). 2013;4:33-9.
- 25. Islam H, Elgendy Y, Segal R, et al. Risk prediction model for in-hospital mortality in women with ST-elevation myocardial infarction: A machine learning approach. J Heart & Lung. 2017:1-7.
- 26. Buettner R, Schunter M, editors. Efficient machine learning based detection of heart disease. 2019 IEEE International Conference on E-health Networking, Application & Services (HealthCom); 2019: IEEE.
APA | Yilmaz R, YAĞIN F (2022). Early Detection of Coronary Heart Disease Based on Machine Learning Methods. , 1 - 6. 10.37990/medr.1011924 |
Chicago | Yilmaz Rustem,YAĞIN Fatma Hilal Early Detection of Coronary Heart Disease Based on Machine Learning Methods. (2022): 1 - 6. 10.37990/medr.1011924 |
MLA | Yilmaz Rustem,YAĞIN Fatma Hilal Early Detection of Coronary Heart Disease Based on Machine Learning Methods. , 2022, ss.1 - 6. 10.37990/medr.1011924 |
AMA | Yilmaz R,YAĞIN F Early Detection of Coronary Heart Disease Based on Machine Learning Methods. . 2022; 1 - 6. 10.37990/medr.1011924 |
Vancouver | Yilmaz R,YAĞIN F Early Detection of Coronary Heart Disease Based on Machine Learning Methods. . 2022; 1 - 6. 10.37990/medr.1011924 |
IEEE | Yilmaz R,YAĞIN F "Early Detection of Coronary Heart Disease Based on Machine Learning Methods." , ss.1 - 6, 2022. 10.37990/medr.1011924 |
ISNAD | Yilmaz, Rustem - YAĞIN, Fatma Hilal. "Early Detection of Coronary Heart Disease Based on Machine Learning Methods". (2022), 1-6. https://doi.org/10.37990/medr.1011924 |
APA | Yilmaz R, YAĞIN F (2022). Early Detection of Coronary Heart Disease Based on Machine Learning Methods. Medical records-international medical journal (Online), 4(1), 1 - 6. 10.37990/medr.1011924 |
Chicago | Yilmaz Rustem,YAĞIN Fatma Hilal Early Detection of Coronary Heart Disease Based on Machine Learning Methods. Medical records-international medical journal (Online) 4, no.1 (2022): 1 - 6. 10.37990/medr.1011924 |
MLA | Yilmaz Rustem,YAĞIN Fatma Hilal Early Detection of Coronary Heart Disease Based on Machine Learning Methods. Medical records-international medical journal (Online), vol.4, no.1, 2022, ss.1 - 6. 10.37990/medr.1011924 |
AMA | Yilmaz R,YAĞIN F Early Detection of Coronary Heart Disease Based on Machine Learning Methods. Medical records-international medical journal (Online). 2022; 4(1): 1 - 6. 10.37990/medr.1011924 |
Vancouver | Yilmaz R,YAĞIN F Early Detection of Coronary Heart Disease Based on Machine Learning Methods. Medical records-international medical journal (Online). 2022; 4(1): 1 - 6. 10.37990/medr.1011924 |
IEEE | Yilmaz R,YAĞIN F "Early Detection of Coronary Heart Disease Based on Machine Learning Methods." Medical records-international medical journal (Online), 4, ss.1 - 6, 2022. 10.37990/medr.1011924 |
ISNAD | Yilmaz, Rustem - YAĞIN, Fatma Hilal. "Early Detection of Coronary Heart Disease Based on Machine Learning Methods". Medical records-international medical journal (Online) 4/1 (2022), 1-6. https://doi.org/10.37990/medr.1011924 |