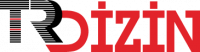
Sahte Haber Tespiti için Derin Bağlamsal Kelime Gömülmeleri ve Sinirsel Ağların Performans Değerlendirmesi
Yıl: 2022 Cilt: 34 Sayı: 2 Sayfa Aralığı: 733 - 742 Metin Dili: Türkçe DOI: 10.35234/fumbd.1126688 İndeks Tarihi: 29-09-2022
Sahte Haber Tespiti için Derin Bağlamsal Kelime Gömülmeleri ve Sinirsel Ağların Performans Değerlendirmesi
Öz: Artan internet ve sosyal medya kullanımı ile sosyal medya ve online haber siteleri bilgi içeriklerini oluşturmada ve yaymada önemli kaynaklar haline gelmişlerdir. Ancak online bilginin miktarı ve üretilme hızından ötürü insan eliyle doğrulanması mümkün olamamaktadır. Dahası rejimler, hükümetler ve etnik kökenler denetimden uzak yalan haberlerin yıkıcı etkisine maruz kalmakta ve bu olumsuz etkilerin minimuma indirgenmesi için yeni teknolojilere ihtiyaç duyulmaktadır. Son yıllarda farklı doğal dil işleme görevlerinde ön-eğitimli modeller başarı ile kullanılmaktadır. Derin sinir ağı ve kavramsal kelime gömülmelerinin birlikte kullanılması durumunda hangi sınıflandırıcının daha verimli olduğu hususu yeterince net değildir. Bu noktada kapsamlı ve karşılaştırmalı çalışmanın eksikliğinden ötürü Global Vektörler (GloVe) ön-eğitimli kelime gömülme katmanının sağladığı bağlamsal temsiller ile dört farklı sınıflandırıcı deneysel sürece dahil edilmiştir. GLoVe katmanından sonra Çok Katmanlı Algılayıcı, Uzun Ömürlü Kısa Dönem Bellek Ağı, Yinelemeli Sinir Ağları ve Evrişimsel Sinir ağları kullanılmıştır. Deneysel çalışmalarda açık erişimli Covid-19 isimli sahte haber tespit veri seti kullanılmış, başarımı çeşitli performans metrikleri ile hesaplanmıştır. En yüksek başarım %91 ile Uzun Ömürlü Kısa Dönem Bellek ağları tarafından rapor edilmiştir. Diğer sonuçlar karşılaştırmalı bir biçimde ilgili tablo ve grafiklerde yer almaktadır. Ön-eğitimli kelime gömülmelerinin farklı sinirsel ağlardan bağımsız olarak yüksek bir hassasiyetle sahte haberlerin tespitinde kullanılabileceğini gösteren umut verici sonuçlar sunulmuştur.
Anahtar Kelime: Performance Evaluation of Deep Contextual Word Embeddings and Neural Networks for Fake News Detection
Öz: With the increasing use of the internet and social media, social media and online news sites have become essential sources in creating and disseminating information content. However, due to the amount and production speed of online information, it is impossible to verify it with human hands. Moreover, regimes, governments, and ethnicities are exposed to the devastating effects of uncontrolled fake news, and new technologies are needed to minimize these harmful effects. Recently, pre-trained models have been used successfully in different natural language processing tasks. However, it is not clear enough which classifier is more efficient when deep neural networks and conceptual word embeddings are used together. At this point, due to the lack of comprehensive and comparative study, Global Vectors (GloVe) were included in the experimental process with four different classifiers with the contextual representations provided by the pre-trained word embedding layer. After the GLoVe layer, Multi-Layer Perceptron (MLP), Long-Short Term Memory Network (LSTM), Recurrent Neural Networks (RNN), and Convolutional Neural networks (CNN) are used. In experimental studies, an open access fake news detection dataset named COVID-19 was used, and its performance was calculated with various performance metrics. LSTM Network reported the highest performance of 91%. Promising results are presented, showing that pre-trained word embeddings can be used to detect fake news with a high sensitivity independent of different neural networks.
Anahtar Kelime: Belge Türü: Makale Makale Türü: Araştırma Makalesi Erişim Türü: Erişime Açık
- [1] Hark C, Karcı A. Karcı summarization: A simple and effective approach for automatic text summarization using Karcı entropy. Information processing & management 2020; vol. 57, no. 3: 102187.
- [2] Türk Dil Kurumu. Türk Dil Kurumu Sözlükleri., Tdk, 2021. https://sozluk.gov.tr/ (accessed Nov. 01, 2021).
- [3] Pan JZ, Pavlova S, Li C, Li N, Li Y, Liu J. Content Based Fake News Detection Using Knowledge Graphs., 2018 669– 683.
- [4] Gravanis G, Vakali A, Diamantaras K, Karadais P. Behind the cues: A benchmarking study for fake news detection. Expert Syst. Appl. 2019; vol. 128 201–213.
- [5] Kaliyar RK, Goswami A, Narang P, Sinha S. FNDNet – A deep convolutional neural network for fake news detection. Cogn. Syst. Res. 2020; vol. 61 32–44.
- [6] ShuKai, SlivaAmy, WangSuhang, TangJiliang, LiuHuan. Fake News Detection on Social Media. ACM SIGKDD Explor. Newsl. 2017; vol. 19, no. 1: 22–36.
- [7] Gilda S. Notice of Violation of IEEE Publication Principles: Evaluating machine learning algorithms for fake news detection.,in 2017 IEEE 15th Student Conference on Research and Development (SCOReD),Dec. 2017,110–115.
- [8] Chollet FDeep Learning with Python. Buzdağı Yayınevi,2021.
- [9] Vaswani, A., Shazeer, N., Parmar, N., Uszkoreit, J., Jones, L., Gomez AN. Attention is all you need. Adv. neural Inf. Process. Syst. 2017; 5998–6008..
- [10] Kaur S, Kumar P, Kumaraguru P. Automating fake news detection system using multi-level voting model. Soft Comput. 2020; vol. 24, no. 12: 9049–9069.
- [11] Verma C, Stoffova V, Illes Z, Tanwar S, Kumar N. Machine Learning-Based Student’s Native Place Identification for Real-Time. IEEE Access 2020; vol. 8 130840–130854.
- [12] Ahmed H, Traore I, Saad S. Detecting opinion spams and fake news using text classification. Secur. Priv. 2018; vol. 1, no. 1: e9.
- [13] Zhang J, Dong B, Yu PS. FakeDetector: Effective Fake News Detection with Deep Diffusive Neural Network.,in 2020 IEEE 36th International Conference on Data Engineering (ICDE),Apr. 2020,1826–1829.
- [14] Bhattacharya P, Patel SB, Gupta R, Tanwar S, Rodrigues JJPC. SaTYa: Trusted Bi-LSTM-Based Fake News Classification Scheme for Smart Community. IEEE Trans. Comput. Soc. Syst. 2021; 1–10.
- [15] Mansouri R, Naderan-Tahan M, Rashti MJ. A Semi-supervised Learning Method for Fake News Detection in Social Media.,in 2020 28th Iranian Conference on Electrical Engineering (ICEE),Aug. 2020,1–5.
- [16] Mahabub A. A robust technique of fake news detection using Ensemble Voting Classifier and comparison with other classifiers. SN Appl. Sci. 2020; vol. 2, no. 4: 525.
- [17] Patwa P et al. Fighting an Infodemic: COVID-19 Fake News Dataset., 2021 21–29.
- [18] Fu X, Liu W, Xu Y, Cui L. Combine HowNet lexicon to train phrase recursive autoencoder for sentence-level sentiment analysis. Neurocomputing 2017; vol. 241 18–27.
- [19] Zhou X, Zafarani R. A Survey of Fake News. ACM Comput. Surv. 2020; vol. 53, no. 5:
- [20] Mikolov T, Chen K, Corrado G, Dean J. Efficient Estimation of Word Representations in Vector Space. 2013; [Online]. Available: http://arxiv.org/abs/1301.3781
- [21] Samadi M, Mousavian M, Momtazi S. Deep contextualized text representation and learning for fake news detection. Information processing & management 2021; vol. 58, no. 6: 102723.
- [22] Aydoğan M, Kocaman V. TRSAv1: A new benchmark dataset for classifying user reviews on Turkish e-commerce websites. J. Inf. Sci. 2022; 016555152210743.
- [23] KARACA YE, ASLAN S. Sentiment Analysis of Covid-19 Tweets by using LSTM Learning Model. Comput. Sci. 2021; no. Special: 366–374.
- [24] Seyyarer E, Uckan T, Hark C, Ayata F, Inan T, Karci A. Applications and Comparisons of Optimization Algorithms Used in Convolutional Neural Networks.,in 2019 International Artificial Intelligence and Data Processing Symposium (IDAP),Sep. 2019,1–6.
- [25] KARACA YE, ASLAN S, HARK C. SEPARATION OF DOMESTIC WASTE WITH DEEP LEARNING TECHNIQUES. Comput. Sci. 2022; vol. 7, no. 1: 10–19.
- [26] SEYYARER E, KARCİ A, ATEŞ A. Stokastik ve deterministik hareketlerin optimizasyon süreçlerindeki etkileri. Gazi Üniversitesi Mühendislik-Mimarlık Fakültesi Derg. 2021; vol. 37, no. 2: 949–966.
- [27] SÖYLEMEZ ÖF, ERGEN B. Farklı Evrişimsel Sinir Ağı Mimarilerinin Yüz İfade Analizi Alanındaki Başarımlarının İncelenmesi. DÜMF Mühendislik Derg. 2020; vol. 11, no. 1: 123–133.
- [28] Tumen V, Soylemez OF, Ergen B. Facial emotion recognition on a dataset using convolutional neural network.,in 2017 International Artificial Intelligence and Data Processing Symposium (IDAP),Sep. 2017,1–5.
APA | hark c (2022). Sahte Haber Tespiti için Derin Bağlamsal Kelime Gömülmeleri ve Sinirsel Ağların Performans Değerlendirmesi. , 733 - 742. 10.35234/fumbd.1126688 |
Chicago | hark cengiz Sahte Haber Tespiti için Derin Bağlamsal Kelime Gömülmeleri ve Sinirsel Ağların Performans Değerlendirmesi. (2022): 733 - 742. 10.35234/fumbd.1126688 |
MLA | hark cengiz Sahte Haber Tespiti için Derin Bağlamsal Kelime Gömülmeleri ve Sinirsel Ağların Performans Değerlendirmesi. , 2022, ss.733 - 742. 10.35234/fumbd.1126688 |
AMA | hark c Sahte Haber Tespiti için Derin Bağlamsal Kelime Gömülmeleri ve Sinirsel Ağların Performans Değerlendirmesi. . 2022; 733 - 742. 10.35234/fumbd.1126688 |
Vancouver | hark c Sahte Haber Tespiti için Derin Bağlamsal Kelime Gömülmeleri ve Sinirsel Ağların Performans Değerlendirmesi. . 2022; 733 - 742. 10.35234/fumbd.1126688 |
IEEE | hark c "Sahte Haber Tespiti için Derin Bağlamsal Kelime Gömülmeleri ve Sinirsel Ağların Performans Değerlendirmesi." , ss.733 - 742, 2022. 10.35234/fumbd.1126688 |
ISNAD | hark, cengiz. "Sahte Haber Tespiti için Derin Bağlamsal Kelime Gömülmeleri ve Sinirsel Ağların Performans Değerlendirmesi". (2022), 733-742. https://doi.org/10.35234/fumbd.1126688 |
APA | hark c (2022). Sahte Haber Tespiti için Derin Bağlamsal Kelime Gömülmeleri ve Sinirsel Ağların Performans Değerlendirmesi. Fırat Üniversitesi Mühendislik Bilimleri Dergisi, 34(2), 733 - 742. 10.35234/fumbd.1126688 |
Chicago | hark cengiz Sahte Haber Tespiti için Derin Bağlamsal Kelime Gömülmeleri ve Sinirsel Ağların Performans Değerlendirmesi. Fırat Üniversitesi Mühendislik Bilimleri Dergisi 34, no.2 (2022): 733 - 742. 10.35234/fumbd.1126688 |
MLA | hark cengiz Sahte Haber Tespiti için Derin Bağlamsal Kelime Gömülmeleri ve Sinirsel Ağların Performans Değerlendirmesi. Fırat Üniversitesi Mühendislik Bilimleri Dergisi, vol.34, no.2, 2022, ss.733 - 742. 10.35234/fumbd.1126688 |
AMA | hark c Sahte Haber Tespiti için Derin Bağlamsal Kelime Gömülmeleri ve Sinirsel Ağların Performans Değerlendirmesi. Fırat Üniversitesi Mühendislik Bilimleri Dergisi. 2022; 34(2): 733 - 742. 10.35234/fumbd.1126688 |
Vancouver | hark c Sahte Haber Tespiti için Derin Bağlamsal Kelime Gömülmeleri ve Sinirsel Ağların Performans Değerlendirmesi. Fırat Üniversitesi Mühendislik Bilimleri Dergisi. 2022; 34(2): 733 - 742. 10.35234/fumbd.1126688 |
IEEE | hark c "Sahte Haber Tespiti için Derin Bağlamsal Kelime Gömülmeleri ve Sinirsel Ağların Performans Değerlendirmesi." Fırat Üniversitesi Mühendislik Bilimleri Dergisi, 34, ss.733 - 742, 2022. 10.35234/fumbd.1126688 |
ISNAD | hark, cengiz. "Sahte Haber Tespiti için Derin Bağlamsal Kelime Gömülmeleri ve Sinirsel Ağların Performans Değerlendirmesi". Fırat Üniversitesi Mühendislik Bilimleri Dergisi 34/2 (2022), 733-742. https://doi.org/10.35234/fumbd.1126688 |