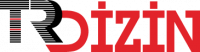
Deep Learning Based Forecasting of Delay on Flights
Yıl: 2022 Cilt: 15 Sayı: 3 Sayfa Aralığı: 239 - 249 Metin Dili: İngilizce DOI: 10.17671/gazibtd.1060646 İndeks Tarihi: 11-10-2022
Deep Learning Based Forecasting of Delay on Flights
Öz: In this study, three different methods from machine learning and deep learning have been implemented for preventing financial and moral losses that may occur as a result of delays in flights and to take necessary precautions by predicting the flight delay in advance, which are a serious problem in the aviation industry. Deep recurrent neural network (DRNN), long-short term memory (LSTM), and random forest (RF) have been extensively tested and compared employing a real data set covering 368 airports across the world with relevancy the success rate of forecasting of delay on flights. The experimental results showed that the LSTM model had a higher success rate of 96.50% at the recall level than the others.
Anahtar Kelime: Derin Öğrenme Tabanlı Havacılık Uçuş Verilerinde Gecikme Durumunun Tahmin Edilmesi
Öz: Bu çalışmada, havacılık endüstrisinde ciddi bir sorun teşkil eden uçuşlarda yaşanan gecikmeler sonucu oluşabilecek maddi-manevi kayıpları önlemek ve uçuş gecikmesinin önceden tahmin edilerek gerekli önlemlerin alınabilmesi amacıyla makine öğrenmesi ve derin öğrenmeden oluşan üç farklı yöntem uygulanmıştır. Deep recurrent neural networks (DRNN), long-short term memory (LSTM) ve random forest (RF) yöntemleri kapsamlı bir şekilde test edilmiş ve dünya genelinde 368 havalimanını kapsayan gerçek bir veri seti kullanılarak uçuşların gecikme durumu tahmin edilmiştir. Deneysel sonuçlar, LSTM modelinin %96.50 recall değeriyle diğer modellere göre daha yüksek başarı oranına sahip olduğunu göstermiştir.
Anahtar Kelime: Belge Türü: Makale Makale Türü: Araştırma Makalesi Erişim Türü: Erişime Açık
- [1] Internet: Bureau of T ransportation Official, Bureau of T ransportation Statistics, https://www.bts.gov/, 15.11.2021
- [2] L. Belcastro, F. Marozzo, D. Talia, and P. Trunfio, “Using Scalable Data Mining for Predicting Flight Delays”, ACM Trans. Intel. syst. Technol., 1, 5-20, 2016. [3]A. Sternberg, J. D. A Soares, D. Carvalho, E. Ogasawara, “ A Review on Flight Delay Prediction.”, arXiv: Computers and Society, 2017.
- [3] A. Sternberg, J. D. A Soares, D. Carvalho, E. Ogasawara, “A Review on Flight Delay Prediction.”, arXiv: Computers and Society, 2017.
- [4] L. Kang, M. Hansen, “Behavioral analysis of airline scheduled block time adjustment”, Transp. pic. Part E: Log. Transp. Rev. 103, 56–68, 2017.
- [5] N. Kenan, A. Jebali, A. Diabat, “The integrated aircraft routing problem with optional flights and delay considerations”, Transp. pic. Part E: Log. Transp. Rev., 118, 355– 375, 2018.
- [6] A. A. Yazdi, P. Dutta, A. B. Steven. “Airline baggage fees and flight delays: a floor wax and dessert topping?”, Transp. pic. Part E: Log. Transp. Rev., 104, 83–96, 2017.
- [7] YJ. Kim, S. Choi, S. Briceno, D. Mavris, “A deep learning approach to flight delay prediction.”, 2016 IEEE/AIAA 35th Digital Avionics Systems Conference , USA, 1-6, 2016.
- [8] S. Choi, YJ. Kim, S. Briceno, D. Mavris, “Prediction of weather induced airline delays based on machine learning algorithms.”, 2016 IEEE/AIAA 35th digital avionics systems conference (DASC); USA, 1-6, 2016.
- [9] L. Belcastro, F. Marozzo, D. Talia, P. Trunfio, “Using scalable data mining for predicting flight delays.”, ACM Trans Intel SystTechnol, 8, 1–20, 2016.
- [10] B. T hiagarajan, L. Srinivasan, A. V. Sharma, D. Sreekanthan, V. Vijayaraghavan, “A machine learning approach for prediction of on time performance of flights.”, In: Proceeding of IEEE/AIAA 35th digital avionics systems conference, USA, 6, 2016
- [11] U. S. Prasad, P. A. Chauhan, L. S. Asha., “Data mining & predictive analysis on airlines performance.”, Int J Pure Appl Math.2018;118:1–12. AIAA 35th digital avionics systems confe re nce (DASC), Sacramento, CA, USA, 6, 1–6, 2016.
- [12] B. Yu, Z. Guo, S. Asian, H. Wang, G. Chen, “Flight delay prediction for commercial air transport: A deep learning approach”, Transportation Research Part E, 125, 203 – 221, 2019.
- [13] S. Manna, S. Biswas, R. Kundu, S. Rakshit, P. Gupta, S. Barman, “ A Statistical Approach to Predict Flight Delay Using Gradient Boosted Decision T ree”, International C onference on Computational Intelligence in Data Science, Chennai, India, 1- 5, 2017.
- [14] H. Idrıs, J. Clarke, R. Bhuva, L. Kang, “Queuing Model for T axi- Out T ime Estimation”, ATC Quarterly,10(1), 1-22, 2002.
- [15] Y. T u, M. Ball, W. Jank, “Estimating Flight Departure Delay Distributions – A Statistical Approach with Long-Term Trend and Short-Term Pattern”, Journal of the American Statistical Association, 103, 112-125, 2008.
- [16] P. Balakrishna, R. Ganesan, L. Sherry, “Accuracy of Reinforcement Learning Algorithms for Predicting Aircraft Taxi- Out T imes: Case-Study of Tampa Bay Departures”, Transportation Research Part C: Emerging Technologies, 18, 950-962, 2012.
- [17] R. Wesonga, F. Nabugoomu, P. Jehopio, “Parameterized Framework for the Analysis of Probabilities of Aircraft Delay at an Airport”, Journal of Air Transport Management, 23, 1-4, 2012.
- [18] M. Arikan, V. Deshpande, M. Sohoni, “Building Reliable Air- T ravel Infrastructure Using Stochastic Models of Airline Networks”, Operations Research, 61 (1), 45-64, 2013.
- [19] S. Khanmohammadi, C. Chou, H. Lewis, D. Elias, (2014), “A Systems Approach for Scheduling Aircraft Landings in JFK Airport”, IEEE International Conference on Fuzzy Systems (IEEE 2014), Beijing, China, 11, 1578-1585, 2014.
- [20] J. Rebollo, H. Balakrishnan, “Characterization and Prediction of Air T raffic Delays,”, Transportation Research Part C: Emerging Technologies, 44, 231-241, 2014.
- [21] O. Lordan, J. Sallan, M. Arroyo, Forecasting of Taxi Times: The Case of Barcelona-El Prat Airport, Journal of Air Transport Management, 56, 118-122, 2016.
- [22] P. Rodriguez, P. Sanchez, E. Gomez, Modelling the Asymmetric Probabilistic Delay of Aircraft Arrival, Journal of Air Transport Management, 62, 90-98, 2017.
- [23] P. Baumgarten, R. Malina, A. Lange, T he Impact of Hubbing Concentration on Flight Delays within Airline Networks: An Empirical Analysis of the US Domestic Market. Transportation Research Part E: Logistics and Transportation Review, 66, 103- 114, 2014.
- [24] N. Pyrgiotis, K.M. Malone, A. Odoni, Modelling Delay Propagation within an Airport Network, Transportation Research Part C, Emerging T echnologies, 27, 60-75, 2013
- [25] P. Fleurquin, J.J. Ramasco, V.M. Eguiluz, Systemic Delay Propagation in the USAirport Network. Nature Scientific Reports, 3, 1-6, 2013. [26] N. Xu, L. Sherry, K. Laskey, Multi-Factor Model for Predicting Delays at U.S. Airports. Transportation Research Record: Journal of the Transportation Research Board, 2052, 62-71, 2008.
- [27] J.T . Wong, S.C. T sai, A Survival Model for Flight Delay Propagation, Journal of Air Transport Management, 23, 5-11, 2012
- [28] B. Schellekens, Stochastic Simulation of Delay Propagation: Improving Schedule Stability at Kenya Airways. Master Thesis, Delft University of Technology,2011.
- [29] Internet: B. Marr, What Is Deep Learning AI? A Simple Guide With 8 Practical Examples, https://www.forbes.com/sites/bernardmarr/2018/10/01/what -is- deep-learning-ai-a-simple-guide-with-8- practical- examples/#3be4ab4c8d4b, 15.11.2021.
- [30] K. Funahashi, Y. Nakamura, “Approximation of dynamical systems by continuous time recurrent neural networks”, Neural networks, 6, 801–806, 1993.
- [31] Internet: A. Amidi, Recurrent Neural Networks Cheatsheet, https://stanford.edu/~shervine/teaching/cs-230/cheatsheet- recurrent-neural-networks, 15.11.2021.
- [32] Y. Lecun, B.E. Boser, J.S. Denker, D. Henderson, R.E. Howard, W.E. Hubbard, LD. Jackel, “Handwritten digit recognition with a backpropagation network”, Advances in Neural Information Processing Systems, 2, 396 –404, 1990.
- [33] M. Valenti, S. Squartini, A. Diment, G. Parascandolo, T. Virtanen, “A convolutional neural network approach for acoustic scene classification”, International Joint Conference on Neural Networks (IJCNN), Anchorage, Alaska, 2017.
- [34] H. Eghbal-Zadeh, B. Lehner, M. Dorfer, G. Widmer, “A hybrid approach using binaural I-vectors and deep convolutional neural networks”, Workshop on Detection and Classification of Acoustic Scenes and Events (DCASE), Budapest, Hungary, 2016.
- [35] Internet: A Review of Recurrent Neuarl Networks: LST M Cells and Network Architectures, https://www.mitpressjournals.org/doi/full/10.1162/neco_a_01199, 15.11.2021.
- [36] Internet: Rectified Linear Units (ReLU) in Deep Learning, https://www.kaggle.com/dansbecker/rectified-linear-units-ReLU- in-deep-learning, 15.11.2021.
- [37] Internet: What is Deep Learning? https://machinelearningmastery.com/what-is-deep-learning/, 15.11.2021.
- [38] K. Funahashi, Y. Nakamura, Approximation of dynamical systems by continuous time recurrent neural networks, Neural networks, 6, 801–806, 1993.
- [39] A. Graves, A. Mohamed, G. Hinton, Speech recognition with deep recurrent neural networks, Acoustics, IEEE International Conference on. IEEE, 6645–6649, 2013.
- [40] R. Pascanu, C. Gulcehre, K. Cho, Y. Bengio, How to construct deep recurrent neural networks, arXiv preprint arXiv, 1312, 6026, 2013.
- [41] S. Karsoliya, “Approximating Number of Hidden layer neurons in Multiple Hidden Layer BPNN Architecture”, International Journal of Engineering Trends and Technology, 6, 714–717, 2012.
APA | Ayaydın A, Akcayol M (2022). Deep Learning Based Forecasting of Delay on Flights. , 239 - 249. 10.17671/gazibtd.1060646 |
Chicago | Ayaydın Anıl,Akcayol M. Ali Deep Learning Based Forecasting of Delay on Flights. (2022): 239 - 249. 10.17671/gazibtd.1060646 |
MLA | Ayaydın Anıl,Akcayol M. Ali Deep Learning Based Forecasting of Delay on Flights. , 2022, ss.239 - 249. 10.17671/gazibtd.1060646 |
AMA | Ayaydın A,Akcayol M Deep Learning Based Forecasting of Delay on Flights. . 2022; 239 - 249. 10.17671/gazibtd.1060646 |
Vancouver | Ayaydın A,Akcayol M Deep Learning Based Forecasting of Delay on Flights. . 2022; 239 - 249. 10.17671/gazibtd.1060646 |
IEEE | Ayaydın A,Akcayol M "Deep Learning Based Forecasting of Delay on Flights." , ss.239 - 249, 2022. 10.17671/gazibtd.1060646 |
ISNAD | Ayaydın, Anıl - Akcayol, M. Ali. "Deep Learning Based Forecasting of Delay on Flights". (2022), 239-249. https://doi.org/10.17671/gazibtd.1060646 |
APA | Ayaydın A, Akcayol M (2022). Deep Learning Based Forecasting of Delay on Flights. Bilişim Teknolojileri Dergisi, 15(3), 239 - 249. 10.17671/gazibtd.1060646 |
Chicago | Ayaydın Anıl,Akcayol M. Ali Deep Learning Based Forecasting of Delay on Flights. Bilişim Teknolojileri Dergisi 15, no.3 (2022): 239 - 249. 10.17671/gazibtd.1060646 |
MLA | Ayaydın Anıl,Akcayol M. Ali Deep Learning Based Forecasting of Delay on Flights. Bilişim Teknolojileri Dergisi, vol.15, no.3, 2022, ss.239 - 249. 10.17671/gazibtd.1060646 |
AMA | Ayaydın A,Akcayol M Deep Learning Based Forecasting of Delay on Flights. Bilişim Teknolojileri Dergisi. 2022; 15(3): 239 - 249. 10.17671/gazibtd.1060646 |
Vancouver | Ayaydın A,Akcayol M Deep Learning Based Forecasting of Delay on Flights. Bilişim Teknolojileri Dergisi. 2022; 15(3): 239 - 249. 10.17671/gazibtd.1060646 |
IEEE | Ayaydın A,Akcayol M "Deep Learning Based Forecasting of Delay on Flights." Bilişim Teknolojileri Dergisi, 15, ss.239 - 249, 2022. 10.17671/gazibtd.1060646 |
ISNAD | Ayaydın, Anıl - Akcayol, M. Ali. "Deep Learning Based Forecasting of Delay on Flights". Bilişim Teknolojileri Dergisi 15/3 (2022), 239-249. https://doi.org/10.17671/gazibtd.1060646 |