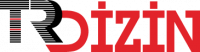
Twitter iletileri duygu değerleri ve Bist 30 endeksi günlük değer değişimlerinin Pearson korelasyonu ve Granger nedensellik analizi
Yıl: 2021 Cilt: 36 Sayı: 3 Sayfa Aralığı: 1687 - 1701 Metin Dili: Türkçe DOI: 10.17341/gazimmfd.660018 İndeks Tarihi: 11-11-2022
Twitter iletileri duygu değerleri ve Bist 30 endeksi günlük değer değişimlerinin Pearson korelasyonu ve Granger nedensellik analizi
Öz: Müşteri odaklı bir anlayışın egemen olduğu çağımızda, yatırımcılar ile etkin bir iletişim ağının oluşturulabilmesi adına finansal işletmeler sosyal medya platformlarına önem vermeye başlamıştır. Bu çalışmada, iki farklı zaman dilimini kapsayacak şekilde, yatırımcıların etkin bir sosyal medya platformu olan Twitter’da paylaşmış oldukları kısa iletilerden elde edilen farklı duygu polarite değerleriyle, Bist30 endeksi günlük değer değişimleri arasındaki ilişkiyi incelemek için Pearson korelasyon analizi ve Granger nedensellik analizi metotları uygulanmıştır. Analizler sonucunda, duygu polarite değerleri ve Bist30 endeksi günlük değer değişimleri arasında anlamlı korelasyon ilişkileri tespit edilmiştir. Ayrıca, özelikle olağan dışı olayların gerçekleştiği bir dönemde duygu polarite değerlerinden Bist30 değer değişimlerine doğru bir Granger nedensellik ilişkisinin ortaya çıktığı görülmüştür. Türkçe finansal iletiler ile çalışan ve duygu analizi aşamasında Doc2Vec metodu kullanılırken yeni bir öneriyi içeren bu çalışma, korelasyon analizi ve Granger nedensellik analizi aşamalarını barındıran, analizleri gerçekleştirmek için gerekli olan istatistiksel ve ekonometrik tüm testleri uygulayan en kapsamlı çalışmalardandır.
Anahtar Kelime: Pearson correlation and Granger causality analysis of Twitter sentiments and the daily changes in Bist30 index returns
Öz: In our age, where a customer-oriented approach is dominant, financial enterprises have started to focus on social media platforms in order to create an active communication network with their investors. In this study, encompassing two different time periods, Pearson correlation analysis and Granger causality analysis are conducted in order to identify the relation between the investors’ varying sentimental polarity values in their posts they share on Twitter, which is an effective social media platform, and daily changes in Bist30 index returns. As a result, significant correlations are found between the polarity values of tweets and the daily changes in Bist30 index returns. In addition to this result, especially in the period of unusual events, it is seen that there is a Granger causality from the polarity values of tweets towards the daily changes in Bist30 index returns. This study that works with the financial Turkish tweets and proposes a new method while conducting Doc2Vec algorithm at the level of sentiment analysis, is one of the most comprehensive studies since it not only contains correlation analysis and Granger causality analysis steps, but also applies all the statistical and econometric tests, which are necessary to carry out analysis.
Anahtar Kelime: Belge Türü: Makale Makale Türü: Araştırma Makalesi Erişim Türü: Erişime Açık
- 1. Symantech. Social Media Protection Flash Poll Global Results. http://www.symantec.com/about/news/release/article.js p?prid=20110721_01. Yayin tarihi Temmuz 21, 2011. Erisim tarihi Mart, 20, 2019.
- 2. Liu, L. X., Ann E. S., Yong Z., The long-run role of the media: Evidence from initial public offerings, Management Science, 60 (8), 1945-1964, 2014.
- 3. Atan, S., Çinar, Y. Borsa Istanbul’da finansal haberler ile piyasa degeri iliskisinin metin madenciligi ve duygu (sentiment) analizi ile incelenmesi, Ankara Üniversitesi Sbf Dergisi,74 (1),1-34, 2019.
- 4. Teti, E., Dallocchio, M., Aniasi, A. The relationship between twitter and stock prices. Evidence from the US technology industry, Technological Forecasting and Social Change, 149 (119747), 1-9, 2019.
- 5. Sprenger T. O., Tumasjan A., Sandner P. G., Welpe, I. M., Tweets and trades: The information content of stock microblogs, European Financial Management, 20 (5), 926-957, 2014.
- 6. Eliaçik A. B., Erdogan N., Mikro Bloglardaki Finans Topluluklari için Kullanici Agirliklandirilmis Duygu Analizi Yöntemi, Ulusal Yazılım Mühendisliği Sempozyumu, İzmir, 782-793, 2015.
- 7. Yildirim M., Yüksel C. A., Sosyal Medya ile Hisse Senedi Fiyatinin Günlük Hareket Yönü Arasindaki Iliskinin Incelenmesi: Duygu Analizi Uygulamasi. Uluslararasi Iktisadi ve Idari Incelemeler Dergisi, 22, 33-44, 2017.
- 8. Nielsen F., A new anew: evaluation of a word list for sentiment analysis in microblogs, In The ESQ2011 Workshop on Making Sense of Microposts, Heraklion, Crete, 93-98, 2011.
- 9. Wilson T., Wiebe J., Hofimann P., Recognizing contextual polarity in phrase-level sentiment analysis, in: Proceedings of the Conference on Human Language Technology and Empirical Methods in Natural Language Processing-HLT, 347–354, 2005.
- 10. Baccianella, S., Esuli, A., Sebastiani, F., Sentiwordnet 3.0: an enhanced lexical resource for sentiment analysis and opinion mining, In LREC’10, Valetta, 2200-2204, 2010.
- 11. Dehkharghani, R., Saygin, Y., Yanikoglu, B., Oflazer, K., SentiTurkNet: a Turkish polarity lexicon for sentiment analysis. Language Resources and Evaluation, 50 (3), 667-685, 2016.
- 12. Loughran, T. I. M., McDonald, B., When is a Liability not a Liability? Textual Analysis, Dictionaries, and 10- Ks. Journal of Finance, 66 (1), 35-65, 2011.
- 13. Le Q., Mikolov T., Distributed representations of sentences and documents, In Proceedings of the 31st International Conference on Machine Learning, Pekin- Çin, 1188–1196, 21-22 Haziran, 2014.
- 14. Granger, C. W. J., Investigating causal relations by econometric models and cross-spectral methods, Econometrica, 37 (3), 424–438, 1969.
- 15. Bollen J., Mao H., Zeng X., Twitter mood predicts the stock market, Journal of computational science, 2 (1), 1- 8, 2011.
- 16. Mittal A., Goel A., Stock prediction using twitter sentiment analysis, Working Paper Standford University, CS 229, 1-5, 2012.
- 17. Smailovic, J., Grcar, M., Lavrac, N., Znidarsic, M. (2014), Stream-based active learning for sentiment analysis in the financial domain, Information Sciences, 285 (32), 181–203, 2014.
- 18. Risius, M., Akolk, F., Beck, R., Differential emotions and the stock market—The case of company-specific trading, European Conference on Trading on Twitter Information Systems, Germany, Paper 147, 2015.
- 19. Sul, H.K., Dennis, A.R., Yuan, L., Trading on twitter: using social media sentiment to predict stock returns, Decision Sciences, 48 (3), 454–488, 2017.
- 20. Tabari, N., Biswas, P., Praneeth, B., Seyeditabari, A., Hadzikadic, M., Zadrozny, W., Causality analysis of twitter sentiments and stock market returns. In Proceedings of the First Workshop on Economics and Natural Language Processing, 11-19, 2018.
- 21. Ranco G., Aleksovski D., Caldarelli G., Grcar M., Mozetic I., The effects of Twitter sentiment on stock price returns, PloS one, 10 (9), 1-21, 2015.
- 22. Pagolu V. S., Reddy K. N., Panda G., Majhi B, Sentiment analysis of Twitter data for predicting stock market movements, In 2016 international conference on signal processing, communication, power and embedded system (SCOPES), Paralakhemundi- Hindistan, 1345-1350, 3-5 Ekim, 2016.
- 23. Zhang, W., Li, X., Shen, D., Teglio, A., Daily happiness and stock returns: some international evidence. Phys. A Stat. Mech. Its Appl. 460, 201–209, 2016.
- 24. Deng S., Huang Z. J., Sinha A. P., Zhao H., The Interaction between Microblog Sentiment and Stock Return: An Empirical Examination, MIS quarterly, 42 (3), 895-918, 2018.
- 25. Zhao R., Quantifying the correlation and prediction of daily happiness sentiment and stock return: The Case of Singapore, Physica A: Statistical Mechanics and its Applications, 533, 1-9, 2019.
- 26. You, W., Guo, Y., Peng, C., Twitter’s daily happiness sentiment and the predictability of stock returns, Financ. Res. Lett. 23, 58–64, 2017.
- 27. Li, X., Shen, D., Xue, M., Zhang, W., Daily happiness and stock returns: the case of Chinese company listed in the United States, Econ. Model. 64, 496–501, 2017.
- 28. Hu, M., Liu., B., Mining and summarizing customer reviews, Proceedings of the tenth ACM SIGKDD international conference on Knowledge discovery and data mining, 168–177, 2004.
- 29. Snow, R., O Connor, B., Jurafsky, D., Ng, A., Labs, D., Capp St., Cheap and Fast But is it Good? Evaluating NonExpert Annotations for Natural Language Tasks, 254–263, 2008.
- 30. Githup. https://github.com/SentimentAnalysisofBist30 , Erişim tarihi: Aralık, 27, 2020.
- 31. Güven Z.A., Diri B., Çakaloğlu T., Comparison of n- stage Latent Dirichlet Allocation versus other topic modeling methods for emotion analysis, Journal of the Faculty of Engineering and Architecture of Gazi University, 35 (4), 2135-2146, 2020.
- 32. Mikolov T., Chen K., Corrado G., Dean J., Efficient estimation of word representations in vector space, Proceedings of Workshop at ICLR, Arizona-Amerika, 1-12, 2-4 Mayıs, 2013.
- 33. Bonett D. G., Wright T. A., Sample size requirements for estimating Pearson, Kendall and Spearman correlations, Psychometrika, 65 (1), 23-28, 2000.
- 34. Genceli M., Kolmogorov-Smirnov, Lilliefors and Shaphiro-Wilk tests for normality, Sigma Journal of Engineering and Natural Sciences, 25 (4), 306-328, 2007.
- 35. IBM SPPS yazılımı. https://www.ibm.com/tr- tr/analytics/spss-statistics-software. Erişim tarihi: Haziran, 1, 2019.
- 36. Cheung Y. W., Lai K. S., Lag order and critical values of the augmented Dickey–Fuller test, Journal of Business & Economic Statistics, 13 (3), 277-280, 1995.
- 37. Işık G., Artuner H., Turkish dialect recognition in terms of prosodic by long short-term memory neural networks, Journal of the Faculty of Engineering and Architecture of Gazi University 35 (1), 213-224, 2020.
- 38. Kilimci Z.H., Financial sentiment analysis with Deep Ensemble Models (DEMs) for stock market prediction, Journal of the
APA | Ateş E, GÜRAN A (2021). Twitter iletileri duygu değerleri ve Bist 30 endeksi günlük değer değişimlerinin Pearson korelasyonu ve Granger nedensellik analizi. , 1687 - 1701. 10.17341/gazimmfd.660018 |
Chicago | Ateş Emine,GÜRAN Aysun Twitter iletileri duygu değerleri ve Bist 30 endeksi günlük değer değişimlerinin Pearson korelasyonu ve Granger nedensellik analizi. (2021): 1687 - 1701. 10.17341/gazimmfd.660018 |
MLA | Ateş Emine,GÜRAN Aysun Twitter iletileri duygu değerleri ve Bist 30 endeksi günlük değer değişimlerinin Pearson korelasyonu ve Granger nedensellik analizi. , 2021, ss.1687 - 1701. 10.17341/gazimmfd.660018 |
AMA | Ateş E,GÜRAN A Twitter iletileri duygu değerleri ve Bist 30 endeksi günlük değer değişimlerinin Pearson korelasyonu ve Granger nedensellik analizi. . 2021; 1687 - 1701. 10.17341/gazimmfd.660018 |
Vancouver | Ateş E,GÜRAN A Twitter iletileri duygu değerleri ve Bist 30 endeksi günlük değer değişimlerinin Pearson korelasyonu ve Granger nedensellik analizi. . 2021; 1687 - 1701. 10.17341/gazimmfd.660018 |
IEEE | Ateş E,GÜRAN A "Twitter iletileri duygu değerleri ve Bist 30 endeksi günlük değer değişimlerinin Pearson korelasyonu ve Granger nedensellik analizi." , ss.1687 - 1701, 2021. 10.17341/gazimmfd.660018 |
ISNAD | Ateş, Emine - GÜRAN, Aysun. "Twitter iletileri duygu değerleri ve Bist 30 endeksi günlük değer değişimlerinin Pearson korelasyonu ve Granger nedensellik analizi". (2021), 1687-1701. https://doi.org/10.17341/gazimmfd.660018 |
APA | Ateş E, GÜRAN A (2021). Twitter iletileri duygu değerleri ve Bist 30 endeksi günlük değer değişimlerinin Pearson korelasyonu ve Granger nedensellik analizi. Gazi Üniversitesi Mühendislik Mimarlık Fakültesi Dergisi, 36(3), 1687 - 1701. 10.17341/gazimmfd.660018 |
Chicago | Ateş Emine,GÜRAN Aysun Twitter iletileri duygu değerleri ve Bist 30 endeksi günlük değer değişimlerinin Pearson korelasyonu ve Granger nedensellik analizi. Gazi Üniversitesi Mühendislik Mimarlık Fakültesi Dergisi 36, no.3 (2021): 1687 - 1701. 10.17341/gazimmfd.660018 |
MLA | Ateş Emine,GÜRAN Aysun Twitter iletileri duygu değerleri ve Bist 30 endeksi günlük değer değişimlerinin Pearson korelasyonu ve Granger nedensellik analizi. Gazi Üniversitesi Mühendislik Mimarlık Fakültesi Dergisi, vol.36, no.3, 2021, ss.1687 - 1701. 10.17341/gazimmfd.660018 |
AMA | Ateş E,GÜRAN A Twitter iletileri duygu değerleri ve Bist 30 endeksi günlük değer değişimlerinin Pearson korelasyonu ve Granger nedensellik analizi. Gazi Üniversitesi Mühendislik Mimarlık Fakültesi Dergisi. 2021; 36(3): 1687 - 1701. 10.17341/gazimmfd.660018 |
Vancouver | Ateş E,GÜRAN A Twitter iletileri duygu değerleri ve Bist 30 endeksi günlük değer değişimlerinin Pearson korelasyonu ve Granger nedensellik analizi. Gazi Üniversitesi Mühendislik Mimarlık Fakültesi Dergisi. 2021; 36(3): 1687 - 1701. 10.17341/gazimmfd.660018 |
IEEE | Ateş E,GÜRAN A "Twitter iletileri duygu değerleri ve Bist 30 endeksi günlük değer değişimlerinin Pearson korelasyonu ve Granger nedensellik analizi." Gazi Üniversitesi Mühendislik Mimarlık Fakültesi Dergisi, 36, ss.1687 - 1701, 2021. 10.17341/gazimmfd.660018 |
ISNAD | Ateş, Emine - GÜRAN, Aysun. "Twitter iletileri duygu değerleri ve Bist 30 endeksi günlük değer değişimlerinin Pearson korelasyonu ve Granger nedensellik analizi". Gazi Üniversitesi Mühendislik Mimarlık Fakültesi Dergisi 36/3 (2021), 1687-1701. https://doi.org/10.17341/gazimmfd.660018 |