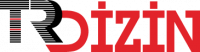
Diyabet tanısının tahminlenmesinde denetimli makine öğrenme algoritmalarının performans karşılaştırması
Yıl: 2022 Cilt: 12 Sayı: 1 Sayfa Aralığı: 211 - 226 Metin Dili: Türkçe DOI: 10.17714/gumusfenbil.820882 İndeks Tarihi: 18-11-2022
Diyabet tanısının tahminlenmesinde denetimli makine öğrenme algoritmalarının performans karşılaştırması
Öz: Hastalık tanısının doğru sınıflandırılmasında, hangi değişkenlerin analize alınacağı ve sonuçların nasıl değerlendirileceği klinik karar verme sürecinin yanı sıra istatistiksel yaklaşımda da doğru bir şekilde tanımlanmalıdır. Bu çalışmada en iyi sınıflandırma performansına sahip algoritmaya iki farklı yaklaşımla karar verilmesi amaçlanmıştır. Kullanılan veri seti, Haziran–Eylül 2013 arasında bir devlet hastanesinin endokrinoloji polikliniğine gelen yaşı 18 ve üstü olan toplam 232 hastadan elde edilmiştir. Diyabet tanısının sınıflandırılması için iki farklı yaklaşım kullanılmıştır. İlk yaklaşımda çokterimli lojistik regresyon yönteminde istatistiksel olarak anlamlı bulunan 18 değişken, ikinci yaklaşımda ise endokrinoloji uzmanı tarafından belirlenen ve klinik olarak önemli bulunan 21 değişkenle modeller kurulmuştur. Diyabet tanısı, denetimli makine öğrenme algoritmalarından Naïve Bayes, Bayes ağları, rastgele orman, karar ağaçları, destek vektör makinaları, k-en yakın komşuluk, yapay sinir ağları ve çokterimli lojistik regresyon yöntemleri ile sınıflandırılmıştır. Model performansları, doğrulukları, Kappa istatistikleri, ortalama mutlak hataları, hata kareler ortalamalarının karekökleri, göreceli mutlak hataları, duyarlılıkları, seçicilikleri, kesinlikleri, F-ölçütleri, Matthews korelasyon katsayıları, ROC eğrileri ve Youden indeksleri kriterlerine göre karşılaştırılmıştır. Model performanslarının test edilmesinde 10-katlı çapraz geçerlilik yöntemi uygulanmış, her algoritmanın çalışma süreleri hesaplanmıştır. Tüm analizler, WEKA 3.8.2 ve R Studio 1.1.383 ile yapılmıştır. Genel anlamda en iyi performansa sahip algoritma, rastgele orman algoritması olarak belirlenmiş, model doğrulukları sırasıyla %84.48 ve %81.90 olarak bulunmuştur. Diyabet hastalığının tanısının konulmasında, doğru sınıflandırma yapabilen modelin seçiminde klinik anlamlılığın yanı sıra istatistiksel anlamlılığa da önem verilmelidir.
Anahtar Kelime: Performance evaluation of supervised machine learning algorithms for predicting diabetes mellitus
Öz: In correct classification of disease diagnosis which variables are analyzed and how results are evaluated should be correctly defined in clinical decision making process as well as in statistical approach. It is aimed to determine the algorithm which has the best classification performance by using two different approaches in this study. The data set was obtained from 232 patients aged≥18 who were admitted to endocrinology outpatient clinic of a public hospital between June-September, 2013. Two different approaches were used to classify diagnosis of diabetes. In the first approach, 18 variables which were found statistically significant in multinomial logistic regression method were utilized; in the second approach, all models were built with 21 clinically significant variables which were determined by expert endocrinologist. Diabetes was classified with supervised machine learning methods; including Naïve Bayes, Bayes network, random forest, decision trees, support vector machine, k-nearest neighbors, artificial neural network and multinomial logistic regression. The performance of models was evaluated with accuracy, Kappa statistics, mean absolute error, root mean squared error, relative absolute error, sensitivity, specificity, precision, F-measure, Matthews correlation coefficient, ROC curve and Youden index. 10-folds cross-validation method was applied to test performance of models; runtimes of each algorithm were calculated. Analyses were performed with WEKA 3.8.2 and R Studio 1.1.383. Generally, random forest algorithm had the best performance with accuracy 84.48% and 81.90%, respectively. Clinical significance should be emphasized as well as statistical significance when choosing correct classification model for diagnosis of diabetes.
Anahtar Kelime: Belge Türü: Makale Makale Türü: Araştırma Makalesi Erişim Türü: Erişime Açık
- Alabi, R.O., Elmusrati, M., Sawazaki-Calone, I., Kowalski, L.P., Haglund, C., Coletta, R.D., Mäkitie, A.A., Salo, T., Almangush, A., & Leivo, I. (2020). Comparison of supervised machine learning classification techniques in prediction of locoregional recurrences in early oral tongue cancer. International Journal of Medical Informatics, 136, 104068. https://doi.org/10.1016/j.ijmedinf.2019.104068
- Ali, M.M., Paul, B.K., Ahmed, K., Bui, F.M., Quinn, J.M.W., & Moni, M.A. (2021). Heart disease prediction using supervised machine learning algorithms: performance analysis and comparison. Computers in Biology and Medicine, 136, 104672. https://doi.org/10.1016/j.compbiomed.2021.104 672
- Alpar, R. (2011). Uygulamalı çok değişkenli istatistiksel yöntemler (3. Baskı). Ankara: Detay. American Diabetes Association. (2014). Standards of medical care in diabetes-2014. Diabetes Care, 37, 14-80. https://doi.org/10.2337/dc14-S014
- Bansal N. (2015). Prediabetes diagnosis and treatment: a review. World Journal of Diabetes, 6(2), 296– 303. https://doi.org/10.4239/wjd.v6.i2.296
- Baratloo, A., Mostafa, H., Ahmed, N., & Gehad, E. A. (2015). Part 1: Simple definition and calculation of accuracy, sensitivity and specificity. Emergency (Tehran, Iran), 3(2), 48–49. https://doi.org/10.22037/emergency.v3i2.8154
- Bilgin, G. (2021). Makine öğrenmesi algoritmaları kullanarak erken dönemde diyabet hastalığı riskinin araştırılması. Journal of Intelligent Systems: Theory and Applications, 4(1), 55-64. https://doi.org/10.38016/jista.877292
- Boughorbel, S., Fethi, J., & Mohammed, E. (2017). Optimal classifier for ımbalanced data using matthews correlation coefficient metric. PloS One, 12(6), e0177678. https://doi.org/10.1371/journal.pone.0177678
- Böhning, D. (1992). Multinomial logistic regression algorithm. Annals of the Institude of Statistical Mathematics, 44(1), 197–200. https://doi.org/10.1007/BF00048682
- Breiman, L. (2001). Random forests. Machine Learning, 45(1), 5–32. https://doi.org/10.1023/A:1010933404324
- Chai, T., & Draxler, R. R. (2014). Root mean square error (RMSE) or mean absolute error (MAE)? – arguments against avoiding rmse in the literature. Geoscientific Model Development, 7, 1247– 1250. https://doi.org/10.5194/gmd-7-1247-2014
- Chen, P., & Pan, C. (2018). Diabetes classification model based on boosting algorithms. BMC Bioinformatics, 19(109). https://doi.org/10.1186/s12859-018-2090-9
- Choudhury A. (2021). Predicting cancer using supervised machine learning: mesothelioma. Technology and Health Care. 29(1), 45-58. https://doi.org/10.3233/THC-202237
- Cihan, P., & Coşkun, H. (2021). Performance comparison of machine learning models for diabetes prediction. 29th Signal Processing and Communications Applications Conference (SIU), (pp. 1-4). İstanbul.
- Cohen, J. (1960). A coefficient of agreement for nominal scales. Educational and Psychological Measurement, 20(1), 37–46. https://doi.org/10.1177/001316446002000104
- Daghistani, T., & Alshammari, R. (2016). Diagnosis of diabetes by applying data mining classification techniques. International Journal of Advanced Computer Science and Applications, 7(7), 329– 332. https://doi.org/10.14569/IJACSA.2016.070747
- Drobatz, K. J. (2009). Measures of accuracy and performance of diagnostic tests. Journal of Veterinary Cardiology, 11, 33–40. https://doi.org/10.1016/j.jvc.2009.03.004
- Egan, A. M., & Dinneen, S. F. (2014). What is diabetes? Medicine (United Kingdom), 42(12), 679–681. https://doi.org/10.1016/j.mpmed.2014.09.005
- Er, M. B., & Işık, İ. (2021). LSTM tabanlı derin ağlar kullanılarak diyabet hastalığı tahmini. Türk Doğa ve Fen Dergisi 10(1), 68-74. https://doi.org/10.46810/tdfd.818528
- Fatima, M., & Pasha, M. (2017). Survey of machine learning algorithms for disease diagnostic. Journal of Intelligent Learning Systems and Applications, 09(1), 1–16. https://doi.org/10.4236/jilsa.2017.91001
- Goldenberg, R., & Punthakee Z. (2013). Definition, classification and diagnosis of diabetes, prediabetes and metabolic syndrome. Canadian Journal of Diabetes, 37(1), 197–212. https://doi.org/10.1016/j.jcjd.2017.10.003
- Goyal, A., & Mehta, R. (2012). Performance comparison of naïve bayes and j48 classification algorithms. International Journal of Applied Engineering Research, 7(11 SUPPL.), 1389– 1393.
- Hajian-Tilaki, K. (2013). Receiver operating characteristic (ROC) curve analysis for medical diagnostic test evaluation. Caspian Journal of Internal Medicine, 4(2), 627–635.
- Hastie, T., Tibshirani, R., & Friedman, J., (2009). The elements of statistical learning: data mining, inference, and prediction (Second Edition). Springer Series in Statistics.
- Heikes, K. E., Eddy, D. M., Arondekar, B., & Schlessinger, L. (2008). Diabetes risk calculator: a simple tool for detecting undiagnosed diabetes and pre-diabetes. Diabetes Care, 31(5),1040– 1045. https://doi.org/10.2337/dc07-1150
- Hripcsak, G., & Rothschild, A. S. (2005). Agreement, the f-measure, and reliability in information retrieval. Journal of the American Medical Informatics Association: JAMIA, 12(3), 296– 298. https://doi.org/10.1197/jamia.M1733
- International Diabetes Federation (2021). IDF diabetes atlas, (10th ed.) Brussels, Belgium: 2021. https://www.diabetesatlas.org.
- Joshi S., & Priyanka Shetty, S. R. (2015). Performance analysis of different classification methods in data mining for diabetes dataset using WEKA tool. International Journal on Recent and Innovation Trends in Computing and Communication, 3(3), 1168-1173. https://doi.org/10.17762/ijritcc2321- 8169.150361
- Kaggle, 2018. (2021, November 16). http://www.kaggle.com/kumargh/pimaindiansdi abetescsv
- Kalsch, J., Bechmann, L.P., Heider, D., Best, J., Manka, P., Kalsch, H., Sowa, J.P., Moebus, S., Slomiany, U., Jockel, K.H., Erbel, R., Gerken, G., & Canbay, A. (2015). Normal liver enzymes are correlated with severity of metabolic syndrome in a large population based cohort. Scientific Reports. 5,13058. https://doi.org/10.1038/srep13058
- Karegowda, A. G., Punya, V., Jayaram, M. A., & Manjunath, A. S. (2012). Rule based classification for diabetic patients using cascaded k-means and decision tree C4. 5th International Journal of Computer Applications, 45(12), 45- 50.
- Kavakiotis, I., Tsave, O., Salifoglou, A., Maglaveras, N., Vlahavas, I., & Chouvarda, I. (2017). Machine learning and data mining methods in diabetes research. Computational and Structural Biotechnology Journal, 15, 104-116. https://doi.org/10.1016/j.csbj.2016.12.005
- Li, H. Xiong, L., Ohno-Machado, L., & Jiang, X. (2014). Privacy preserving rbf kernel support vector machine. BioMed Research International, 2014, 827371. https://doi.org/10.1155/2014/827371
- Maniruzzaman, M., Kumar, N., Menhazul Abedin, M., Islam, M. S., Suri, H. S., El-Baz, A. S., & Suri, J. S. (2017). Comparative approaches for classification of diabetes mellitus data: machine learning paradigm. Computer Methods and Programs in Biomedicine, 152, 23–34. https://doi.org/10.1016/j.cmpb.2017.09.004. Epub 2017 Sep 8
- Muhammad, L.J., Algehyne, E.A., Usman, S.S., Ahmad, A., Chakraborty, C., & Mohammed, I.A. (2021). Supervised machine learning models for prediction of covid-19 infection using epidemiology dataset. SN Computer Science, 2(1),11. https://doi.org/10.1007/s42979-020- 00394-7. Epub 2020 Nov 27
- Neumann, U., Genze, N., & Heider, D. (2017). EFS: an ensemble feature selection tool implemented as R-package and web-application. BioData Mining, 10, 21. https://doi.org/10.1186/s13040- 017-0142-8. eCollection 2017
- Nindrea, R.D., Aryandono, T., Lazuardi, L., & Dwiprahasto, I. (2018). Diagnostic accuracy of different machine learning algorithms for breast cancer risk calculation: a meta-analysis. Asian Pacific Journal of Cancer Prevention, 19(7), 1747-1752. https://doi.org/10.22034/APJCP.2018.19.7.1747.
- Ogurtsova, K., da Rocha Fernandes, J. D., Huang, Y., Linnenkamp, U., Guariguata, L., Cho, N. H., Cavan, D., Shaw, J. E., & Makaroff, L. E. (2017). IDF diabetes atlas: global estimates for the prevalence of diabetes for 2015 and 2040. Diabetes Research and Clinical Practice, 128, 40–50. https://doi.org/10.1016/j.diabres.2017.03.024.
- Olivera, A. R., Roesler, V., Iochpe, C., Schmidt, M. I., Vigo, Á., Barreto, S. M., & Duncan, B. B. (2017). Comparison of machine-learning algorithms to build a predictive model for detecting undiagnosed diabetes- Elsa-Brasil: accuracy study. Sao Paulo Medical Journal, 135(3), 234–246. https://10.1590/1516- 3180.2016.0309010217
- Özlüer Başer, B., Yangın, M., & Sarıdaş, E. S. (2021). Makine öğrenmesi teknikleriyle diyabet hastalığının sınıflandırılması. Süleyman Demirel Üniversitesi Fen Bilimleri Enstitüsü Dergisi, 25(1), 112-120. https://doi.org/10.19113/sdufenbed.842460
- Özmen, Ö., Khdr, A., & Avcı, E. (2018). Sınıflandırıcıların kalp hastalığı verileri üzerine performans karşılaştırması. Fırat Üniversitesi Mühendislik Bilimleri Dergisi, 30(3), 153-159.
- Tiwari, D., Bhati, B.S., Al-Turjman, F., & Nagpal, B. (2021). Pandemic coronavirus disease (Covid- 19): World effects analysis and prediction using machine-learning techniques. Expert Systems. May 11:10.1111/exsy.12714. https://doi.org/10.1111/exsy.12714
- Trevor, H., Tibshirani, R., & Friedman, J. (2011). The elements of statistical learning: data mining, ınference and prediction (2nd ed.). Springer International Publishing.
- Uddin, S., Khan, A., Hossain, M.E., & Moni, M.A. (2019). Comparing different supervised machine learning algorithms for disease prediction. BMC Medical Informatics and Decision Making. 19(1), 281. https://doi.org/10.1186/s12911-019- 1004-8
- Van Harmelen, F., Lifschitz, V., & Porter, B. (2008). Handbook of knowledge representation. Elsevier.
- Walia N., Kumar M., & Kakkar L. (2018). Classification of diabetes patient by using data mining techniques. International Journal for Research in Engineering Application & Management, 4(5), 347-351. https://doi.org/10.18231/2454- 9150.2018.0637
- Worachartcheewan, A., Nantasenamat, C., Prasertsrithong, P., Amranan, J., Monnor, T., Chaisatit, T., Nuchpramool, W., & Prachayasittikul, V. (2013). Machine learning approaches for discerning ıntercorrelation of hematological parameters and glucose level for ıdentification of diabetes mellitus. EXCLI Journal, 12, 885–893. https://doi.org/10.17877/DE290R-7572
- Youden, W. J. (1950). Index for rating diagnostic tests. Cancer, 3(1), 32–35. https://doi.org/10.1002/1097- 0142(1950)3:1<32::aid- cncr2820030106>3.0.co;2-3
- Yu, W., Liu, T., Valdez, R., Gwinn, M., & Khoury, M. J. (2010). Application of support vector machine modeling for prediction of common diseases: the case of diabetes and pre-diabetes. BMC Medical Informatics and Decision Making, 10(1), 16. https://doi.org/10.1186/1472-6947-10-16.
APA | Ozkan Y, sarer yurekli b, SUNER A (2022). Diyabet tanısının tahminlenmesinde denetimli makine öğrenme algoritmalarının performans karşılaştırması. , 211 - 226. 10.17714/gumusfenbil.820882 |
Chicago | Ozkan Yuksel,sarer yurekli banu,SUNER ASLI Diyabet tanısının tahminlenmesinde denetimli makine öğrenme algoritmalarının performans karşılaştırması. (2022): 211 - 226. 10.17714/gumusfenbil.820882 |
MLA | Ozkan Yuksel,sarer yurekli banu,SUNER ASLI Diyabet tanısının tahminlenmesinde denetimli makine öğrenme algoritmalarının performans karşılaştırması. , 2022, ss.211 - 226. 10.17714/gumusfenbil.820882 |
AMA | Ozkan Y,sarer yurekli b,SUNER A Diyabet tanısının tahminlenmesinde denetimli makine öğrenme algoritmalarının performans karşılaştırması. . 2022; 211 - 226. 10.17714/gumusfenbil.820882 |
Vancouver | Ozkan Y,sarer yurekli b,SUNER A Diyabet tanısının tahminlenmesinde denetimli makine öğrenme algoritmalarının performans karşılaştırması. . 2022; 211 - 226. 10.17714/gumusfenbil.820882 |
IEEE | Ozkan Y,sarer yurekli b,SUNER A "Diyabet tanısının tahminlenmesinde denetimli makine öğrenme algoritmalarının performans karşılaştırması." , ss.211 - 226, 2022. 10.17714/gumusfenbil.820882 |
ISNAD | Ozkan, Yuksel vd. "Diyabet tanısının tahminlenmesinde denetimli makine öğrenme algoritmalarının performans karşılaştırması". (2022), 211-226. https://doi.org/10.17714/gumusfenbil.820882 |
APA | Ozkan Y, sarer yurekli b, SUNER A (2022). Diyabet tanısının tahminlenmesinde denetimli makine öğrenme algoritmalarının performans karşılaştırması. Gümüşhane Üniversitesi Fen Bilimleri Dergisi, 12(1), 211 - 226. 10.17714/gumusfenbil.820882 |
Chicago | Ozkan Yuksel,sarer yurekli banu,SUNER ASLI Diyabet tanısının tahminlenmesinde denetimli makine öğrenme algoritmalarının performans karşılaştırması. Gümüşhane Üniversitesi Fen Bilimleri Dergisi 12, no.1 (2022): 211 - 226. 10.17714/gumusfenbil.820882 |
MLA | Ozkan Yuksel,sarer yurekli banu,SUNER ASLI Diyabet tanısının tahminlenmesinde denetimli makine öğrenme algoritmalarının performans karşılaştırması. Gümüşhane Üniversitesi Fen Bilimleri Dergisi, vol.12, no.1, 2022, ss.211 - 226. 10.17714/gumusfenbil.820882 |
AMA | Ozkan Y,sarer yurekli b,SUNER A Diyabet tanısının tahminlenmesinde denetimli makine öğrenme algoritmalarının performans karşılaştırması. Gümüşhane Üniversitesi Fen Bilimleri Dergisi. 2022; 12(1): 211 - 226. 10.17714/gumusfenbil.820882 |
Vancouver | Ozkan Y,sarer yurekli b,SUNER A Diyabet tanısının tahminlenmesinde denetimli makine öğrenme algoritmalarının performans karşılaştırması. Gümüşhane Üniversitesi Fen Bilimleri Dergisi. 2022; 12(1): 211 - 226. 10.17714/gumusfenbil.820882 |
IEEE | Ozkan Y,sarer yurekli b,SUNER A "Diyabet tanısının tahminlenmesinde denetimli makine öğrenme algoritmalarının performans karşılaştırması." Gümüşhane Üniversitesi Fen Bilimleri Dergisi, 12, ss.211 - 226, 2022. 10.17714/gumusfenbil.820882 |
ISNAD | Ozkan, Yuksel vd. "Diyabet tanısının tahminlenmesinde denetimli makine öğrenme algoritmalarının performans karşılaştırması". Gümüşhane Üniversitesi Fen Bilimleri Dergisi 12/1 (2022), 211-226. https://doi.org/10.17714/gumusfenbil.820882 |