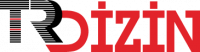
Enlightening the molecular mechanisms of type 2 diabetes with a novel pathway clustering and pathway subnetwork approach
Yıl: 2022 Cilt: 46 Sayı: 4 Sayfa Aralığı: 318 - 341 Metin Dili: İngilizce DOI: 10.55730/1300-0152.2620 İndeks Tarihi: 05-12-2022
Enlightening the molecular mechanisms of type 2 diabetes with a novel pathway clustering and pathway subnetwork approach
Öz: Type 2 diabetes mellitus (T2D) constitutes 90% of the diabetes cases, and it is a complex multifactorial disease. In the last decade, genome-wide association studies (GWASs) for T2D successfully pinpointed the genetic variants (typically single nucleotide polymorphisms, SNPs) that associate with disease risk. In order to diminish the burden of multiple testing in GWAS, researchers attempted to evaluate the collective effects of interesting variants. In this regard, pathway-based analyses of GWAS became popular to discover novel multigenic functional associations. Still, to reveal the unaccounted 85 to 90% of T2D variation, which lies hidden in GWAS datasets, new post-GWAS strategies need to be developed. In this respect, here we reanalyze three metaanalysis data of GWAS in T2D, using the methodology that we have developed to identify disease-associated pathways by combining nominally significant evidence of genetic association with the known biochemical pathways, protein-protein interaction (PPI) networks, and the functional information of selected SNPs. In this research effort, to enlighten the molecular mechanisms underlying T2D development and progress, we integrated different in silico approaches that proceed in top-down manner and bottom-up manner, and presented a comprehensive analysis at protein subnetwork, pathway, and pathway subnetwork levels. Using the mutual information based on the shared genes, the identified protein subnetworks and the affected pathways of each dataset were compared. While most of the identified pathways recapitulate the pathophysiology of T2D, our results show that incorporating SNP functional properties, PPI networks into GWAS can dissect leading molecular pathways, and it could offer improvement over traditional enrichment strategies.
Anahtar Kelime: Belge Türü: Makale Makale Türü: Araştırma Makalesi Erişim Türü: Erişime Açık
- Adeyemo AA, Tekola-Ayele F, Doumatey AP, Bentley AR, Chen G et al. (2015). Evaluation of Genome Wide Association Study Associated Type 2 Diabetes Susceptibility Loci in Sub Saharan Africans. Frontiers in Genetics 6: 335. doi: 10.3389/ fgene.2015.00335
- Akhtar S, Benter IF (2013. The role of epidermal growth factor receptor in diabetes-induced cardiac dysfunction. BioImpacts doi: 10.5681/bi.2013.008
- Akhtar S, Chandrasekhar B, Attur S, Dhaunsi GS, Yousif MHM et al. (2015). Transactivation of ErbB Family of Receptor Tyrosine Kinases Is Inhibited by Angiotensin-(1-7) via Its Mas Receptor.
- PLOS ONE 10: e0141657. doi: 10.1371/journal.pone.0141657 Bader GD, Hogue CWV (2003). An automated method for finding molecular complexes in large protein interaction networks. BMC Bioinformatics 4: 2. doi: 10.1186/1471-2105-4-2
- Bakir-Gungor B, Bakir-Gungor B, Sezerman OU (2012). Identification of SNP Targeted Pathways From Genome-wide Association Study (GWAS) Data. Protocol Exchange doi: 10.1038/protex.2012.019
- Bakir-Gungor B, Baykan B, İseri SU, Tuncer FN, Sezerman OU (2013). Identifying SNP targeted pathways in partial epilepsies with genome-wide association study data. Epilepsy Research 105: 92–102. doi: 10.1016/j.eplepsyres.2013.02.008
- Bakir-Gungor B, Egemen E, Sezerman OU (2014). PANOGA: A web server for identification of SNP-targeted pathways from genome-wide association study data. Bioinformatics 30: 1287– 1289. doi: 10.1093/bioinformatics/btt743
- Bakir-Gungor B, Remmers EF, Meguro A, Mizuki N, Kastner DL et al. (2015a). Identification of possible pathogenic pathways in Behçet’s disease using genome-wide association study data from two different populations. European Journal of Human Genetics: EJHG 23: 678–687. doi: 10.1038/ejhg.2014.158
- Bakir-Gungor B, Remmers EF, Meguro A, Mizuki N, Kastner DL et al. (2015b). Identification of possible pathogenic pathways in Behçet’s disease using genome-wide association study data from two different populations. European Journal of Human Genetics: EJHG, 23 (5): 678–687. doi:10.1038/ejhg.2014.158
- Bakir-Gungor B, Sezerman OU (2011). A New Methodology to Associate SNPs with Human Diseases According to Their Pathway Related Context. PLoS ONE 6: e26277. doi: 10.1371/ journal.pone.0026277
- Bakir-Gungor B, Sezerman OU (2013). The Identification of Pathway Markers in Intracranial Aneurysm Using Genome-Wide Association Data from Two Different Populations. PLoS ONE 8: e57022. doi: 10.1371/journal.pone.0057022
- Barabási A-L, Gulbahce N, Loscalzo J (2011). Network medicine: a network-based approach to human disease. Nature Reviews Genetics 12: 56–68. doi: 10.1038/nrg2918
- Baranzini SE, Galwey NW, Wang J, Khankhanian P, Lindberg R et al. (2009). Pathway and network-based analysis of genome-wide association studies in multiple sclerosis. Human Molecular Genetics doi: 10.1093/hmg/ddp120
- Berntorp K, Frid A, Alm R, Fredrikson G, Sjöberg K et al. (2013). Antibodies against gonadotropin-releasing hormone (GnRH) in patients with diabetes mellitus is associated with lower body weight and autonomic neuropathy. BMC Research Notes 6: 329. doi: 10.1186/1756-0500-6-329
- Bindea G, Mlecnik B, Hackl H, Charoentong P, Tosolini M et al. (2009). ClueGO: a Cytoscape plug-in to decipher functionally grouped gene ontology and pathway annotation networks. Bioinformatics (Oxford, England) 25: 1091–1093. doi: 10.1093/ bioinformatics/btp101
- Bonàs-Guarch S, Guindo-Martínez M, Miguel-Escalada I, Grarup N, Sebastian D et al. (2018). Re-analysis of public genetic data reveals a rare X-chromosomal variant associated with type 2 diabetes. Nature Communications 9: 321. doi: 10.1038/s41467- 017-02380-9
- Bonnefond A, Froguel P (2015). Rare and Common Genetic Events in Type 2 Diabetes: What Should Biologists Know? Cell Metabolism 21: 357–368. doi: 10.1016/j.cmet.2014.12.020
- Boström P, Andersson L, Vind B, Håversen L, Rutberg M et al. (2010). The SNARE protein SNAP23 and the SNARE-interacting protein Munc18c in human skeletal muscle are implicated in insulin resistance/type 2 diabetes. Diabetes doi: 10.2337/db09- 1503
- Brocca G, Ferraresso S, Zamboni C, Martinez-Merlo EM, Ferro S et al. (2019). Array Comparative Genomic Hybridization Analysis Reveals Significantly Enriched Pathways in Canine Oral Melanoma. Frontiers in Oncology 9: 1397.
- Brubaker D, Liu Y, Wang J, Tan H, Zhang G et al. (2016). Finding lost genes in GWAS via integrative—omics analysis reveals novel sub-networks associated with preterm birth. Human Molecular Genetics 25: 5254–5264. doi: 10.1093/hmg/ddw325
- Carter H, Douville C, Stenson PD, Cooper DN, Karchin R (2013). Identifying Mendelian disease genes with the Variant Effect Scoring Tool. BMC Genomics 14: S3. doi: 10.1186/1471-2164- 14-S3-S3
- Chang X, Lima L de A, Liu Y, Li J, Li Q et al. (2018). Common and Rare Genetic Risk Factors Converge in Protein Interaction Networks Underlying Schizophrenia. Frontiers in Genetics 9: 434.
- Cirillo E, Kutmon M, Gonzalez Hernandez M, Hooimeijer T, Adriaens ME et al. (2018). From SNPs to pathways: Biological interpretation of type 2 diabetes (T2DM) genome wide association study (GWAS) results. PLOS ONE 13: e0193515. doi: 10.1371/journal.pone.0193515
- Claussnitzer M, Cho JH, Collins R, Cox NJ, Dermitzakis ET et al. (2020). A brief history of human disease genetics. Nature 577: 179–189. doi: 10.1038/s41586-019-1879-7
- Costantini S, Prandini P, Corradi M, Pasquali A, Contreas G et al. (2011). A novel synonymous substitution in the GCK gene causes aberrant splicing in an Italian patient with GCK-MODY phenotype. Diabetes Research and Clinical Practice 92: e23– e26. doi: 10.1016/j.diabres.2011.01.014
- DeFronzo RA, Ferrannini E, Groop L, Henry RR, Herman WH et al. (2015). Type 2 diabetes mellitus. Nature Reviews Disease Primers 1: 15019. doi: 10.1038/nrdp.2015.19
- Dissanayake WC, Sorrenson B, Shepherd PR (2018). The role of adherens junction proteins in the regulation of insulin secretion. Bioscience Reports 38. doi: 10.1042/BSR20170989
- Dlamini Z, Mokoena F, Hull R (2017). Abnormalities in alternative splicing in diabetes: therapeutic targets. Journal of Molecular Endocrinology 59: R93–R107. doi: 10.1530/JME-17-0049
- Douville C, Masica DL, Stenson PD, Cooper DN, Gygax DM et al. (2016). Assessing the Pathogenicity of Insertion and Deletion Variants with the Variant Effect Scoring Tool (VEST-Indel). Human mutation 37: 28–35. doi: 10.1002/humu.22911
- Elmansy D, Koyutürk M (2019). Cross-population analysis for functional characterization of type II diabetes variants. BMC Bioinformatics 20: 320. doi: 10.1186/s12859-019-2835-0
- Erdmann J, Zeller T (eds.). (2019). From GWAS Hits to Treatment Targets. Frontiers Media SA doi: 10.3389/978-2-88945-982-7
- Farber CR, Mesner LD (2016). A Systems-Level Understanding of Cardiovascular Disease through Networks. In: Rodriguez- Oquendo ABT-TCGM ed. Translational Cardiometabolic Genomic Medicine. Boston: Elsevier 59–81. doi: 10.1016/ B978-0-12-799961-6.00003-2
- Fernández-Tajes J, Gaulton KJ, van de Bunt M, Torres J, Thurner M et al. (2019). Developing a network view of type 2 diabetes risk pathways through integration of genetic, genomic and functional data. Genome Medicine 11: 19. doi: 10.1186/ s13073-019-0628-8
- Fisher RA (1934). Statistical methods for research workers. Florez JC, Udler MS, Hanson RL (2021). Genetics of Type 2 Diabetes, In: Diabetes in America. 3rd edition, Review from National Institute of Diabetes and Digestive and Kidney Diseases (US), Bethesda (MD).
- Freeman JS (2013). Review of Insulin-Dependent and Insulin- Independent Agents for Treating Patients With Type 2 Diabetes Mellitus and Potential Role for Sodium-Glucose Co- Transporter 2 Inhibitors. Postgraduate Medicine 125: 214–226. doi: 10.3810/pgm.2013.05.2672
- Fuchsberger C, Flannick J, Teslovich TM, Mahajan A, Agarwala V et al. (2016). The genetic architecture of type 2 diabetes. Nature 536: 41–47. doi: 10.1038/nature18642
- Gallagher MD, Chen-Plotkin AS (2018). The post-GWAS era: from association to function. The American Journal of Human Genetics 102: 717–730.
- García-Campos MA, Espinal-Enríquez J, Hernández-Lemus E (2015). Pathway Analysis: State of the Art. Frontiers in Physiology 6: 383. doi: 10.3389/fphys.2015.00383
- Ghiassian SD, Menche J, Barabási AL (2015). A DIseAse MOdule Detection (DIAMOnD) Algorithm Derived from a Systematic Analysis of Connectivity Patterns of Disease Proteins in the Human Interactome. PLoS Computational Biology 11: 1–21. doi: 10.1371/journal.pcbi.1004120
- Grotz AK, Gloyn AL, Thomsen SK (2017). Prioritising Causal Genes at Type 2 Diabetes Risk Loci. Current Diabetes Reports 17: 76. doi: 10.1007/s11892-017-0907-y
- Herder C, Brunner EJ, Rathmann W, Strassburger K, Tabak AG et al. (2009). Elevated Levels of the Anti-Inflammatory Interleukin-1 Receptor Antagonist Precede the Onset of Type 2 Diabetes: The Whitehall II Study. Diabetes Care 32: 421–423. doi: 10.2337/ dc08-1161
- Houtz J, Borden P, Ceasrine A, Minichiello L, Kuruvilla R (2016). Neurotrophin Signaling Is Required for Glucose-Induced Insulin Secretion. Developmental Cell 39: 329–345. doi: 10.1016/j.devcel.2016.10.003
- Huang X, Liu G, Guo J, Su Z (2018). The PI3K/AKT pathway in obesity and type 2 diabetes. International Journal of Biological Sciences 14: 1483–1496. doi: 10.7150/ijbs.27173
- Huang DW, Sherman BT, Lempicki RA (2009a). Bioinformatics enrichment tools: paths toward the comprehensive functional analysis of large gene lists. Nucleic Acids Research 37: 1–13. doi: 10.1093/nar/gkn923
- Huang DW, Sherman BT, Lempicki RA (2009b). Systematic and integrative analysis of large gene lists using DAVID bioinformatics resources. Nature Protocols 4: 44–57. doi: 10.1038/nprot.2008.211
- Ideker T, Ozier O, Schwikowski B, Siegel AF (2002). Discovering regulatory and signalling circuits in molecular interaction networks. Bioinformatics 18: 233–240. doi: 10.1093/ bioinformatics/18.suppl1.S233
- International Diabetes Federation. IDF Diabetes Atlas, 10th edn. Brussels, Belgium: International Diabetes Federation, 2021.
- Kajdaniuk D, Marek B, Borgiel-Marek H, Kos-Kudła B (2013). Transforming growth factor beta1 (TGFbeta1) in physiology and pathology. Endokrynologia Polska 64: 384–396. doi: 10.5603/EP.2013.0022
- Kao PYP, Leung KH, Chan LWC, Yip SP, Yap MKH (2017). Pathway analysis of complex diseases for GWAS, extending to consider rare variants, multi-omics and interactions. Biochimica et Biophysica Acta (BBA) - General Subjects 1861: 335–353. doi: 10.1016/j.bbagen.2016.11.030
- Lamparter D, Marbach D, Rueedi R, Kutalik Z, Bergmann S (2016). Fast and Rigorous Computation of Gene and Pathway Scores from SNP-Based Summary Statistics. PLOS Computational Biology 12: e1004714.
- Lee I, Blom UM, Wang PI, Shim JE, Marcotte EM (2011). Prioritizing candidate disease genes by network-based boosting of genome- wide association data. Genome Research 21: 1109–1121. doi: 10.1101/gr.118992.110
- Leng S, Zhang W, Zheng Y, Liberman Z, Rhodes CJ et al. (2010). Glycogen synthase kinase 3 beta mediates high glucose- induced ubiquitination and proteasome degradation of insulin receptor substrate 1. The Journal of endocrinology 206: 171– 181. doi: 10.1677/JOE-09-0456
- Li M-X, Gui H-S, Kwan JSH, Sham PC (2011). GATES: a rapid and powerful gene-based association test using extended Simes procedure. American journal of human genetics 88: 283–293. doi: 10.1016/j.ajhg.2011.01.019
- Lin J-R, Jaroslawicz D, Cai Y, Zhang Q, Wang Z et al. (2017). PGA: post-GWAS analysis for disease gene identification. Bioinformatics 34: 1786–1788.
- Liu JZ, McRae AF, Nyholt DR, Medland SE, Wray NR et al. (2010). A versatile gene-based test for genome-wide association studies. American journal of human genetics 87: 139–145. doi: 10.1016/j.ajhg.2010.06.009
- Liu Y, Zhao J, Jiang T, Yu M, Jiang G et al. (2017). A pathway analysis of genome-wide association study highlights novel type 2 diabetes risk pathways. Scientific Reports 7: 12546. doi: 10.1038/s41598-017-12873-8
- López de Maturana E, Rodríguez JA, Alonso L, Lao O, Molina- Montes E et al. (2020). A multilayered post-GWAS assessment on genetic susceptibility to pancreatic cancer. bioRxiv:2020.02.11.941351. doi: 10.1101/2020.02.11.941351
- Luk CT, Shi SY, Cai EP, Sivasubramaniyam T, Krishnamurthy M et al. (2017). FAK signalling controls insulin sensitivity through regulation of adipocyte survival. Nature Communications 8: 14360. doi: 10.1038/ncomms14360
- Maffei A, Lembo G, Carnevale D (2018). PI3Kinases in Diabetes Mellitus and Its Related Complications. International Journal of Molecular Sciences 19: 4098. doi: 10.3390/ijms19124098
- Mahajan A, Taliun D, Thurner M, Robertson NR, Torres JM et al. (2018a). Fine-mapping type 2 diabetes loci to single-variant resolution using high-density imputation and islet-specific epigenome maps. Nature Genetics 50: 1505–1513. doi: 10.1038/ s41588-018-0241-6
- Mahajan A, Wessel J, Willems SM, Zhao W, Robertson NR et al. (2018b). Refining the accuracy of validated target identification through coding variant fine-mapping in type 2 diabetes article. Nature Genetics doi: 10.1038/s41588-018-0084-1
- Mameishvili E, Serafimidis I, Iwaszkiewicz S, Lesche M, Reinhardt S et al. (2019). Aldh1b1 expression defines progenitor cells in the adult pancreas and is required for Kras-induced pancreatic cancer. Proceedings of the National Academy of Sciences 116: 20679 LP – 20688. doi: 10.1073/pnas.1901075116
- McHugh ML 2012. Interrater reliability: the kappa statistic. Biochemia Medica 22: 276–82.
- Mercader JM, Florez JC (2017). The Genetic Basis of Type 2 Diabetes in Hispanics and Latin Americans: Challenges and Opportunities. Frontiers in Public Health 5. doi: 10.3389/ fpubh.2017.00329
- Meyre D (2017). Give GWAS a Chance. Diabetes 66: 2741–2742. doi: 10.2337/dbi17-0026
- Mlecnik B, Galon J, Bindea G (2019). Automated exploration of gene ontology term and pathway networks with ClueGO-REST. Bioinformatics 35: 3864–3866. doi: 10.1093/bioinformatics/ btz163
- Nguyen T-M, Shafi A, Nguyen T, Draghici S (2019). Identifying significantly impacted pathways: a comprehensive review and assessment. Genome Biology 20: 203. doi: 10.1186/s13059- 019-1790-4
- Ono H, Katagiri H, Funaki M, Anai M, Inukai K et al. (2001). Regulation of Phosphoinositide Metabolism, Akt Phosphorylation, and Glucose Transport by PTEN (Phosphatase and Tensin Homolog Deleted on Chromosome 10) in 3T3-L1 Adipocytes. Molecular Endocrinology 15: 1411– 1422. doi: 10.1210/mend.15.8.0684
- Perry JRB, McCarthy MI, Hattersley AT, Zeggini E, Weedon MN et al. (2009). Interrogating Type 2 Diabetes Genome-Wide Association Data Using a Biological Pathway-Based Approach. Diabetes 58: 1463–1467. doi: 10.2337/db08-1378
- Pers TH, Karjalainen JM, Chan Y, Westra H-J, Wood AR et al. (2015). Biological interpretation of genome-wide association studies using predicted gene functions. Nature Communications 6: 5890. doi: 10.1038/ncomms6890
- Piko P, Werissa NA, Fiatal S, Sandor J, Adany R (2021). Impact of Genetic Factors on the Age of Onset for Type 2 Diabetes Mellitus in Addition to the Conventional Risk Factors. Journal of Personalized Medicine 11 (1): 6. doi: 10.3390/jpm11010006
- Piñero J, Ramírez-Anguita JM, Saüch-Pitarch J, Ronzano F, Centeno E et al. (2019). The DisGeNET knowledge platform for disease genomics: 2019 update. Nucleic Acids Research 48: D845– D855. doi: 10.1093/nar/gkz1021
- Saccone SF, Saccone NL, Swan GE, Madden PAF, Goate AM et al. (2008). Systematic biological prioritization after a genome- wide association study: an application to nicotine dependence. Bioinformatics (Oxford, England) 24: 1805–1811. doi: 10.1093/ bioinformatics/btn315
- Schierding W, O’Sullivan JM (2015). Connecting SNPs in Diabetes: A Spatial Analysis of Meta-GWAS Loci. Frontiers in Endocrinology 6: 102. doi: 10.3389/fendo.2015.00102
- Schmid D, Stolzlechner M, Sorgner A, Bentele C, Assinger A et al. (2012). An abundant, truncated human sulfonylurea receptor 1 splice variant has prodiabetic properties and impairs sulfonylurea action. Cellular and Molecular Life Sciences 69: 129–148. doi: 10.1007/s00018-011-0739-x
- Scott RA, Scott LJ, Mägi R, Marullo L, Gaulton KJ et al. (2017). An expanded genome-wide association study of type 2 diabetes in Europeans. Diabetes 66: 2888–2902.
- Segrè A V, Consortium D, investigators M, Groop L, Mootha VK et al. (2010). Common inherited variation in mitochondrial genes is not enriched for associations with type 2 diabetes or related glycemic traits. PLoS genetics 6: e1001058. doi: 10.1371/journal.pgen.1001058.
- Sonawane AR, Weiss ST, Glass K, Sharma A (2019). Network Medicine in the Age of Biomedical Big Data. Frontiers in Genetics 10: 294.
- De Souza A, Irfan K, Masud F, Saif MW (2016). Diabetes Type 2 and Pancreatic Cancer: A History Unfolding. JOP: Journal of the Pancreas 17: 144–148.
- Thrash A, Tang JD, DeOrnellis M, Peterson DG, Warburton ML (2019). Pathway Association Studies Tool. bioRxiv:691964. doi: 10.1101/691964.
- Tripathi S, Moutari S, Dehmer M, Emmert-Streib F (2016). Comparison of module detection algorithms in protein networks and investigation of the biological meaning of predicted modules. BMC Bioinformatics 17: 129. doi: 10.1186/ s12859-016-0979-8
- Ulgen E, Ozisik O, Sezerman OU (2019). pathfindR: An R Package for Comprehensive Identification of Enriched Pathways in Omics Data Through Active Subnetworks. Frontiers in Genetics 10: 858.
- Vinh NX, Epps J, Bailey J (2010). Information theoretic measures for clusterings comparison: Variants, properties, normalization and correction for chance. Journal of Machine Learning Research 11: 2837–2854.
- Visscher PM, Wray NR, Zhang Q, Sklar P, McCarthy MI et al. (2017). 10 years of GWAS discovery: biology, function, and translation. The American Journal of Human Genetics 101: 5–22.
- Wang L, Jia P, Wolfinger RD, Chen X, Grayson BL et al. (2011). An efficient hierarchical generalized linear mixed model for pathway analysis of genome-wide association studies. Bioinformatics (Oxford, England) 27: 686–692. doi: 10.1093/ bioinformatics/btq728
- White MJ, Yaspan BL, Veatch OJ, Goddard P, Risse-Adams OS et al. (2019). Strategies for Pathway Analysis Using GWAS and WGS Data. Current Protocols in Human Genetics. doi: 10.1002/ cphg.79
- Wong CK, Wade-Vallance AK, Luciani DS, Brindle PK, Lynn FC et al. (2018). The p300 and CBP Transcriptional Coactivators Are Required for β-Cell and α-Cell Proliferation. Diabetes 67: 412 LP – 422. doi: 10.2337/db17-0237
- Wood AR, Esko T, Yang J, Vedantam S, Pers TH et al. (2014). Defining the role of common variation in the genomic and biological architecture of adult human height. Nature Genetics 46: 1173–1186. doi: 10.1038/ng.3097
- Xiong Q-Y, Yu C, Zhang Y, Ling L, Wang L et al. (2017). Key proteins involved in insulin vesicle exocytosis and secretion. Biomedical Reports 6: 134–139. doi: 10.3892/br.2017.839
- Xuan Vinh N, Epps J, Bailey J (2010). Information Theoretic Measures for Clusterings Comparison: Variants, Properties, Normalization and Correction for Chance.
- Xue A, Wu Y, Zhu Z, Zhang F, Kemper KE et al. (2018). Genome- wide association analyses identify 143 risk variants and putative regulatory mechanisms for type 2 diabetes. Nature Communications 9: 2941. doi: 10.1038/s41467-018-04951-w
- Yang H, Wang K (2015). Genomic variant annotation and prioritization with ANNOVAR and wANNOVAR. Nature Protocols 10: 1556–1566.
- Yoon S, Nguyen HCT, Yoo YJ, Kim J, Baik B et al. (2018). Efficient pathway enrichment and network analysis of GWAS summary data using GSA-SNP2. Nucleic Acids Research 46: e60–e60. doi: 10.1093/nar/gky175
- Zeng Z, Bromberg Y (2019). Predicting Functional Effects of Synonymous Variants: A Systematic Review and Perspectives. Frontiers in Genetics 10: 914.
- Zhao W, Rasheed A, Tikkanen E, Lee J-J, Butterworth AS et al. (2017). Identification of new susceptibility loci for type 2 diabetes and shared etiological pathways with coronary heart disease. Nature Genetics 49: 1450–1457. doi: 10.1038/ng.3943
- Zheng Y, Ley SH, Hu FB (2018). Global aetiology and epidemiology of type 2 diabetes mellitus and its complications. Nature Reviews Endocrinology 14: 88–98. doi: 10.1038/nrendo.2017.151
- Zhong H, Yang X, Kaplan LM, Molony C, Schadt EE (2010). Integrating Pathway Analysis and Genetics of Gene Expression for Genome-wide Association Studies. American Journal of Human Genetics doi: 10.1016/j.ajhg.2010.02.020
APA | Bakir-Gungor B, YAZICI M, Goy G, Temiz M (2022). Enlightening the molecular mechanisms of type 2 diabetes with a novel pathway clustering and pathway subnetwork approach. , 318 - 341. 10.55730/1300-0152.2620 |
Chicago | Bakir-Gungor Burcu,YAZICI Miray ÜNLÜ,Goy Gokhan,Temiz Mustafa Enlightening the molecular mechanisms of type 2 diabetes with a novel pathway clustering and pathway subnetwork approach. (2022): 318 - 341. 10.55730/1300-0152.2620 |
MLA | Bakir-Gungor Burcu,YAZICI Miray ÜNLÜ,Goy Gokhan,Temiz Mustafa Enlightening the molecular mechanisms of type 2 diabetes with a novel pathway clustering and pathway subnetwork approach. , 2022, ss.318 - 341. 10.55730/1300-0152.2620 |
AMA | Bakir-Gungor B,YAZICI M,Goy G,Temiz M Enlightening the molecular mechanisms of type 2 diabetes with a novel pathway clustering and pathway subnetwork approach. . 2022; 318 - 341. 10.55730/1300-0152.2620 |
Vancouver | Bakir-Gungor B,YAZICI M,Goy G,Temiz M Enlightening the molecular mechanisms of type 2 diabetes with a novel pathway clustering and pathway subnetwork approach. . 2022; 318 - 341. 10.55730/1300-0152.2620 |
IEEE | Bakir-Gungor B,YAZICI M,Goy G,Temiz M "Enlightening the molecular mechanisms of type 2 diabetes with a novel pathway clustering and pathway subnetwork approach." , ss.318 - 341, 2022. 10.55730/1300-0152.2620 |
ISNAD | Bakir-Gungor, Burcu vd. "Enlightening the molecular mechanisms of type 2 diabetes with a novel pathway clustering and pathway subnetwork approach". (2022), 318-341. https://doi.org/10.55730/1300-0152.2620 |
APA | Bakir-Gungor B, YAZICI M, Goy G, Temiz M (2022). Enlightening the molecular mechanisms of type 2 diabetes with a novel pathway clustering and pathway subnetwork approach. Turkish Journal of Biology, 46(4), 318 - 341. 10.55730/1300-0152.2620 |
Chicago | Bakir-Gungor Burcu,YAZICI Miray ÜNLÜ,Goy Gokhan,Temiz Mustafa Enlightening the molecular mechanisms of type 2 diabetes with a novel pathway clustering and pathway subnetwork approach. Turkish Journal of Biology 46, no.4 (2022): 318 - 341. 10.55730/1300-0152.2620 |
MLA | Bakir-Gungor Burcu,YAZICI Miray ÜNLÜ,Goy Gokhan,Temiz Mustafa Enlightening the molecular mechanisms of type 2 diabetes with a novel pathway clustering and pathway subnetwork approach. Turkish Journal of Biology, vol.46, no.4, 2022, ss.318 - 341. 10.55730/1300-0152.2620 |
AMA | Bakir-Gungor B,YAZICI M,Goy G,Temiz M Enlightening the molecular mechanisms of type 2 diabetes with a novel pathway clustering and pathway subnetwork approach. Turkish Journal of Biology. 2022; 46(4): 318 - 341. 10.55730/1300-0152.2620 |
Vancouver | Bakir-Gungor B,YAZICI M,Goy G,Temiz M Enlightening the molecular mechanisms of type 2 diabetes with a novel pathway clustering and pathway subnetwork approach. Turkish Journal of Biology. 2022; 46(4): 318 - 341. 10.55730/1300-0152.2620 |
IEEE | Bakir-Gungor B,YAZICI M,Goy G,Temiz M "Enlightening the molecular mechanisms of type 2 diabetes with a novel pathway clustering and pathway subnetwork approach." Turkish Journal of Biology, 46, ss.318 - 341, 2022. 10.55730/1300-0152.2620 |
ISNAD | Bakir-Gungor, Burcu vd. "Enlightening the molecular mechanisms of type 2 diabetes with a novel pathway clustering and pathway subnetwork approach". Turkish Journal of Biology 46/4 (2022), 318-341. https://doi.org/10.55730/1300-0152.2620 |