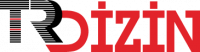
Track density imaging using diffusion tensor imaging data from 1.5 T MRI scanner
Yıl: 2022 Cilt: 30 Sayı: 6 Sayfa Aralığı: 2044 - 2053 Metin Dili: İngilizce DOI: 10.55730/1300-0632.3923 İndeks Tarihi: 08-12-2022
Track density imaging using diffusion tensor imaging data from 1.5 T MRI scanner
Öz: Superresolution track density imaging (TDI) has recently been developed for achieving high resolution track density maps from low-resolution diffusion images acquired at 3 T. But, the utility of the approach is still unclear when applied to diffusion tensor imaging (DTI) data acquired at lower 1.5 T magnetic field strength and thus its advantages or disadvantages awaits for exploration. We implemented an algorithm to generate track density maps of human white matter using streamline tracking and tested its performance with data acquired from two healthy volunteers at 1.5 Tesla. The effects of number of diffusion directions and seed selections on the quality of the reconstructed TDI maps were investigated under a variety of settings. The results were visually evaluated by an anatomist and a radiologist, and statistically characterized using gray level cooccurance Matrices (GLCM). Producing high-quality maps with improved resolution required increasing the number of seeds per voxel. Statistical implications were consistent with visual inspection. Low signal-to-noise ratio in DTI data intrinsically yielded low SNR in the final TD map. Accurately defining the diffusion and thus fiber orientation within a voxel necessitated increasing the number of diffusion encoding directions. Our data suggests that TDI image with DTI data acquired at 1.5 T is possible using right trade-offs in data acquisition and processing and has the capability of delineating the substructures of the brain.
Anahtar Kelime: Belge Türü: Makale Makale Türü: Araştırma Makalesi Erişim Türü: Erişime Açık
- [1] Gramann K, Jung T-P, Ferris DP, Lin C-T, Makeig S. Toward a new cognitive neuroscience: modeling natural brain dynamics. Front Hum Neurosci 2014; 8: 1-3. doi: 10.3389/fnhum.2014.00444
- [2] Bilgen M, Narayana PA. Mohr diagram interpretation of anisotropic diffusion indices in MRI. Magn Reson Imaging 2003; 21: 567-572. doi:10.1016/S0730-725X(03)00071-7
- [3] Gumus K, Keating B, Poser BA, Armstrong B, Chang L et al. Prevention of motion induced signal loss in diffusion weighted echo planar imaging by dynamic restoration of gradient moments. Magn Reson Med 2014; 71: 2006-2013. doi: 10.1002/mrm.24857
- [4] Peng H, Roysam B, Ascoli GA. Automated image computing reshapes computational neuroscience. BMC Bioinfor- matics 2013; 14: 1-5. doi:10.1186/1471-2105-14-293
- [5] Radua J, Romeo M, Mataix-Cols D, Fusar-Poli P. A general approach for combining voxel-based meta-analyses con- ducted in different neuroimaging modalities. Curr Med Chem 2013; 20: 462-466. doi:10.2174/0929867311320030017
- [6] Van Essen DC, Ugurbil K, Auerbach E, Barch D, Behrens TEJ et al. The Human Connectome Project: A data acquisition perspective. NeuroImage 2012; 62: 2222-2231. doi: 10.1016/j.neuroimage.2012.02.018
- [7] Calamante F, Tournier J-D, Jackson GD, Connelly A. Track-density imaging (TDI): Super-resolution white matter imaging using whole-brain track-density mapping. NeuroImage 2010; 53: 1233-1243. doi:10.1016/j.neuroimage.2010.07.024
- [8] Barajas R, Hess C, Phillips J, Von Morze C, Yu J et al. Super-resolution track density imaging of glioblastoma: histopathologic correlation. Am J Neuroradiol 2013; 34: 1319-1325. doi: 10.3174/ajnr.A3400
- [9] Calamante F, Oh SH, Tournier JD, Park SY, Son YD et al. Super resolution track density imaging of thalamic substructures: Comparison with high resolution anatomical magnetic resonance imaging at 7.0 T. Hum Brain Mapp 2013; 34: 2538-2548. doi: 10.1002/hbm.22083
- [10] Calamante F, Tournier J-D, Heidemann RM, Anwander A, Jackson GD et al. Track density imaging (TDI): Validation of super resolution property. NeuroImage 2011; 56: 1259-1266. doi:10.1016/j.neuroimage.2011.02.059
- [11] Calamante F, Tournier J-D, Kurniawan ND, Yang Z, Gyengesi E et al. Super-resolution track-density imaging studies of mouse brain: Comparison to histology. NeuroImage 2012; 59: 286-296. doi:10.1016/j.neuroimage.2011.07.014
- [12] Ellingson BM, Salamon N, Woodworth DC, Holly LT. Correlation between degree of subvoxel spinal cord com- pression measured with super-resolution tract density imaging and neurological impairment in cervical spondylotic myelopathy. J Neurosurg-Spine 2015; 22: 631-638. doi:10.3171/2014.10.SPINE14222
- [13] Kurniawan ND, Richards KL, Yang Z, She D, Ullmann JFP et al. Visualization of mouse barrel cortex using ex-vivo track density imaging. NeuroImage 2014; 87: 465-475. doi:10.1016/j.neuroimage.2013.09.030
- [14] Ullmann JP, Calamante F, Collin S, Reutens D, Kurniawan N. Enhanced characterization of the zebrafish brain as revealed by super-resolution track-density imaging. Brain Struct Funct 2015; 220: 457-468. doi:10.1007/s00429- 013-0667-7
- [15] Ziegler E, Rouillard M, André E, Coolen T, Stender J et al. Mapping track density changes in nigrostriatal and extranigral pathways in Parkinson’s disease. NeuroImage 2014; 99: 498-508. doi:10.1016/j.neuroimage.2014.06.033
- [16] Smith RE, Tournier J-D, Calamante F, Connelly A. Anatomically-constrained tractography: Improved diffusion MRI streamlines tractography through effective use of anatomical information. NeuroImage 2012; 62: 1924-1938. doi: 10.1016/j.neuroimage.2012.06.005
- [17] Smith RE, Tournier J-D, Calamante F, Connelly A. SIFT: Spherical-deconvolution informed filtering of tractograms. NeuroImage 2013; 67: 298-312. doi:10.1016/j.neuroimage.2012.11.049
- [18] Stadlbauer A, Ganslandt O, Salomonowitz E, Buchfelder M, Hammen T et al. Magnetic resonance fiber density mapping of age-related white matter changes. Eur J Radiol 2012; 81: 4005-4012. doi:10.1016/j.ejrad.2012.05.029
- [19] Tench C, Morgan P, Wilson M, Blumhardt L. White matter mapping using diffusion tensor MRI. Magn Reson Med 2002; 47: 967-972. doi:10.1002/mrm.10144
- [20] Jiang H, van Zijl PC, Kim J, Pearlson GD, Mori S. DtiStudio: resource program for diffusion tensor computation and fiber bundle tracking. Comput Meth Prog Bio 2006; 81: 106-116. doi:10.1016/j.cmpb.2005.08.004
- [21] Köşker FB. Difüzyon Tensör Görüntüleme ile Beyin Beyaz Cevher Traktografisi ve Yüksek Çözünürlükte Trak Yoğunluk Görüntülerinin Elde Edilmesi. MSc, Erciyes University, Kayseri, Turkey, 2014.
- [22] Descoteaux M, Deriche R, Knosche TR, Anwander A. Deterministic and Probabilistic Tractography Based on Complex Fibre Orientation Distributions. IEEE T Med Imaging 2009; 28: 269-286. doi:10.1109/TMI.2008.2004424
- [23] Jones DK. Tractography Gone Wild: Probabilistic Fibre Tracking Using the Wild Bootstrap With Diffusion Tensor MRI. IEEE T Med Imaging 2008; 27: 1268-1274. doi:10.1109/TMI.2008.922191
- [24] Zhang M, Sakaie KE, Jones SE. Logical Foundations and Fast Implementation of Probabilistic Tractography. IEEE T Med Imaging 2013; 32: 1397-1410. doi:10.1109/TMI.2013.2257179
- [25] Haralick RM, Shanmugam K, Dinstein IH. Textural features for image classification. IEEE T Syst Man Cyb 1973; 3: 610-621. doi:10.1109/TSMC.1973.4309314
- [26] Tournier J-D, Calamante F, Connelly A. Robust determination of the fibre orientation distribution in diffu- sion MRI: non-negativity constrained super-resolved spherical deconvolution. NeuroImage 2007; 35: 1459-1472. doi:10.1016/j.neuroimage.2007.02.016
- [27] Mori S, Frederiksen K, van Zijl PCM, Stieltjes B, Kraut MA et al. Brain white matter anatomy of tumor patients evaluated with diffusion tensor imaging. Ann Neurol 2002; 51: 377-380. doi:10.1002/ana.10137
- [28] Sundgren PC, Dong Q, Gómez-Hassan D, Mukherji SK, Maly P et al. Diffusion tensor imaging of the brain: review of clinical applications. Neuroradiology 2004; 46: 339-350. doi:10.1007/s00234-003-1114-x
- [29] Yen PS, Teo BT, Chiu CH, Chen SC, Chiu TL et al. White matter tract involvement in brain tumors: a diffusion tensor imaging analysis. Surg Neurol 2009; 72: 464-469. doi:10.1016/j.surneu.2009.05.008
APA | KOSKER F, Gumus K, Tokmakçı M, acer n, senol s, bilgen m (2022). Track density imaging using diffusion tensor imaging data from 1.5 T MRI scanner. , 2044 - 2053. 10.55730/1300-0632.3923 |
Chicago | KOSKER Fatma Betul,Gumus Kazim,Tokmakçı Mahmut,acer niyazi,senol serkan,bilgen mehmet Track density imaging using diffusion tensor imaging data from 1.5 T MRI scanner. (2022): 2044 - 2053. 10.55730/1300-0632.3923 |
MLA | KOSKER Fatma Betul,Gumus Kazim,Tokmakçı Mahmut,acer niyazi,senol serkan,bilgen mehmet Track density imaging using diffusion tensor imaging data from 1.5 T MRI scanner. , 2022, ss.2044 - 2053. 10.55730/1300-0632.3923 |
AMA | KOSKER F,Gumus K,Tokmakçı M,acer n,senol s,bilgen m Track density imaging using diffusion tensor imaging data from 1.5 T MRI scanner. . 2022; 2044 - 2053. 10.55730/1300-0632.3923 |
Vancouver | KOSKER F,Gumus K,Tokmakçı M,acer n,senol s,bilgen m Track density imaging using diffusion tensor imaging data from 1.5 T MRI scanner. . 2022; 2044 - 2053. 10.55730/1300-0632.3923 |
IEEE | KOSKER F,Gumus K,Tokmakçı M,acer n,senol s,bilgen m "Track density imaging using diffusion tensor imaging data from 1.5 T MRI scanner." , ss.2044 - 2053, 2022. 10.55730/1300-0632.3923 |
ISNAD | KOSKER, Fatma Betul vd. "Track density imaging using diffusion tensor imaging data from 1.5 T MRI scanner". (2022), 2044-2053. https://doi.org/10.55730/1300-0632.3923 |
APA | KOSKER F, Gumus K, Tokmakçı M, acer n, senol s, bilgen m (2022). Track density imaging using diffusion tensor imaging data from 1.5 T MRI scanner. Turkish Journal of Electrical Engineering and Computer Sciences, 30(6), 2044 - 2053. 10.55730/1300-0632.3923 |
Chicago | KOSKER Fatma Betul,Gumus Kazim,Tokmakçı Mahmut,acer niyazi,senol serkan,bilgen mehmet Track density imaging using diffusion tensor imaging data from 1.5 T MRI scanner. Turkish Journal of Electrical Engineering and Computer Sciences 30, no.6 (2022): 2044 - 2053. 10.55730/1300-0632.3923 |
MLA | KOSKER Fatma Betul,Gumus Kazim,Tokmakçı Mahmut,acer niyazi,senol serkan,bilgen mehmet Track density imaging using diffusion tensor imaging data from 1.5 T MRI scanner. Turkish Journal of Electrical Engineering and Computer Sciences, vol.30, no.6, 2022, ss.2044 - 2053. 10.55730/1300-0632.3923 |
AMA | KOSKER F,Gumus K,Tokmakçı M,acer n,senol s,bilgen m Track density imaging using diffusion tensor imaging data from 1.5 T MRI scanner. Turkish Journal of Electrical Engineering and Computer Sciences. 2022; 30(6): 2044 - 2053. 10.55730/1300-0632.3923 |
Vancouver | KOSKER F,Gumus K,Tokmakçı M,acer n,senol s,bilgen m Track density imaging using diffusion tensor imaging data from 1.5 T MRI scanner. Turkish Journal of Electrical Engineering and Computer Sciences. 2022; 30(6): 2044 - 2053. 10.55730/1300-0632.3923 |
IEEE | KOSKER F,Gumus K,Tokmakçı M,acer n,senol s,bilgen m "Track density imaging using diffusion tensor imaging data from 1.5 T MRI scanner." Turkish Journal of Electrical Engineering and Computer Sciences, 30, ss.2044 - 2053, 2022. 10.55730/1300-0632.3923 |
ISNAD | KOSKER, Fatma Betul vd. "Track density imaging using diffusion tensor imaging data from 1.5 T MRI scanner". Turkish Journal of Electrical Engineering and Computer Sciences 30/6 (2022), 2044-2053. https://doi.org/10.55730/1300-0632.3923 |