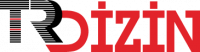
Using electrooculography with visual stimulus tracking test in diagnosing of ADHD: findings from machine learning algorithms
Yıl: 2022 Cilt: 52 Sayı: 5 Sayfa Aralığı: 1616 - 1626 Metin Dili: İngilizce DOI: 10.55730/1300-0144.5502 İndeks Tarihi: 27-12-2022
Using electrooculography with visual stimulus tracking test in diagnosing of ADHD: findings from machine learning algorithms
Öz: Background/aim: Attention deficit hyperactivity disorder (ADHD), one of the most common neurodevelopmental disorders in childhood, is diagnosed clinically by assessing the symptoms of inattention, hyperactivity, and impulsivity. Also, there are limited objective assessment tools to support the diagnosis. Thus, in this study, a new electrooculography (EOG) based on visual stimulus tracking to support the diagnosis of ADHD was proposed. Materials and methods: Reference stimulus one-to-one tracking numbers (RSOT) and colour game detection (CGD) were applied to 53 medication-free children with ADHD and 36 healthy controls (HCs). Also, the test was applied six months after the treatment to children with ADHD. Parameters obtained during the visual stimulus tracking test were analyzed and Higuchi fractal dimension (HFD) and Hjorth parameters were calculated for all EOG records. Results: The average test success rate was higher in HCs than in children with ADHD. Based on machine learning algorithms, the proposed system can distinguish drug-free ADHD patients from HCs with an 89.13% classification performance and also distinguish drug-free children from treated children with an 80.47% classification performance. Conclusion: The findings showed that the proposed system could be helpful to support the diagnosis of ADHD and the follow-up of the treatment.
Anahtar Kelime: Belge Türü: Makale Makale Türü: Araştırma Makalesi Erişim Türü: Erişime Açık
- 1. American Psychiatric Association. Attention-deficit and disruptive behavior disorders. In: Diagnostic and Statistical Manual of Mental Disorders. 5th ed. Arlington, VA: American Psychiatric Association; 2013.
- 2. Willcutt EG. The Prevalence of DSM-IV Attention- Deficit/Hyperactivity Disorder: A MetaAnalytic Review. Neurotherapeutics 2012; 9: 490-499. https://doi.org/10.1007/ s13311-012-0135-8
- 3. Polanczyk GV, Willcutt EG, Salum GA, Kieling C, Rohde LA. ADHD prevalence estimates across three decades: an updated systematic review and meta-regression analysis. International Journal of Epidemiology 2014; 43: 434-442. https://doi. org/10.1093/ije/dyt261
- 4. Fayyad J, Sampson NA, Hwang I, Adamowski T, Aguilar- Gaxiola S et al. The descriptive epidemiology of DSM-IV Adult ADHD in the World Health Organization World Mental Health Surveys. ADHD Attention Deficit and Hyperactivity Disorders 2017; 9: 47-65. https://doi.org/10.1007/s12402-016- 0208-3
- 5. Kieling R, Rohde LA. ADHD in Children and Adults: Diagnosis and Prognosis. In: Stanford C, Tannock R. (editors) Behavioral Neuroscience of Attention Deficit Hyperactivity Disorder and Its Treatment. Current Topics in Behavioral Neurosciences, vol 9. Springer, Berlin: Heidelberg; 2010. pp. 1-16. https://doi. org/10.1007/7854_2010_115
- 6. Conners CK, Sitarenios G, Parker JDA, Epstein JN. The Revised Conners Parent Rating Scale (CPRS-R): Factor structure, reliability, and criterion validity. Journal of Abnormal Child Psychology 1998; 26(4): 257-268. https://doi. org/10.1023/A:1022602400621
- 7. Conners CK, Sitarenios G, Parker JDA, Epstein JN. Revision and restandardization of the Conners Teacher Rating Scale (CTRS-R): Factor structure, reliability, and criterion validity. Journal of Abnormal Child Psychology 1998; 26(4): 279-291. https://doi.org/10.1023/A:1022606501530
- 8. Guy W. Clinical Global Impressions: In ECDEU Assessment Manual for Psychopharmacology, Revised DHEWPub. (ADM). Rockville, MD: National Institute for Mental Health; 1976. pp. 218-222.
- 9. Kedem B. Spectral analysis and discrimination by zero- crossings. Proceedings of the IEEE 1986; 74(11): 1477-1493.
- 10. Pliszka S, AACAP Work Group on Quality Issues. Practice parameter for the assessment and treatment of children and adolescents with attention-deficit/hyperactivity disorder. Journal of the American Academy of Child and Adolescent Psychiatry 2007; 46: 894-921. https://doi.org/10.1097/ chi.0b013e318054e724
- 11. Liu CC, Chaovalitwongse WA, Pardalos PM, Seref O, Xanthopoulos P et al. Quantitative analysis on electrooculography (EOG) for neurodegenerative disease. Systems Analysis and Optimization in Biomedicine 2007;953(1):246-253. https://doi.org/10.1063/1.2817347
- 12. Barea R, Boquete L, Mazo M, Lopez E. Wheelchair guidance strategies using EOG. Journal of Intelligent and Robotic Systems 2002; 34: 279-299. https://doi.org/10.1023/A:1016359503796
- 13. Al-Rahayfeh A, Faezipour M. Enhanced Frame Rate for Real- Time Eye Tracking Using Circular Hough Transform. In: Systems Applications and Technology Conference (LISAT): IEEE; 2013. pp. 1-6.
- 14. Kashani MAA, Arani MM, Fini MRR. Eye detection and tracking in images with using bag of pixels. In: 2011 IEEE 3rd International Conference on Communication Software and Networks (ICCSN); 2011. pp. 64-68.
- 15. Liang Z, Tan F, Chi Z. Video-based biometric identification using eye-tracking technique. In: 2012 IEEE International Conference on Signal Processing, Communication and Computing (ICSPCC 2012); 2012. pp. 728-733.
- 16. Hansen DW, Hammoud R. An Improved Likelihood Model for Eye Tracking. Computer Vision and Image Understanding 2007; 106(2): 220-230. https://doi.org/10.1016/j.cviu.2006.06.012
- 17. Lee YJ, Lee S, Chang M, Kwak HW. Saccadic movement deficiencies in adults with ADHD tendencies. ADHD Attention Deficit Hyperactivity Disorders 2015; 7: 271-280. https://doi. org/10.1007/s12402-015-0174-1
- 18. Tsang V, Chu PC. Comparing Eye-tracking Data of Children with High-functioning ASD, Comorbid ADHD, and of a Control Watching Social Videos. Journal of Visualized Experiments 2018; (142):e58694.
- 19. Marotta A, Pasini A, Menotti E, Pasquini A, Pitzianti MB et al. Controlling attention to gaze and arrows in attention deficit hyperactivity disorder. Psychiatry Research 2017; 251: 148-154. https://doi.org/10.1016/j.psychres.2017.01.094
- 20. Rojas-Líbano D, Wainstein G, Carrasco X, Aboitiz F, Crossley N et al. A pupil size, eye-tracking and neuropsychological dataset from ADHD children during a cognitive task. Scientific Data 2019; 6(1): 25. https://doi.org/10.1038/s41597-019-0037-2
- 21. Esas MY, Latifoğlu F, Demirci E, Altıntop ÇG. Determination of Attention Deficit Hyperactivity Disorder by Electrooculogram Test. In: 2017 Medical Technologies National Congress (TIPTEKNO), Trabzon, Turkey; 2017. pp. 1-4.
- 22. Latifoğlu F, Esas M, Demirci E. Diagnosis of attention- deficit hyperactivity disorder using EOG signals: a new approach. Biomedical Engineering / Biomedizinische Technik 2020; 65(2): 149-164. https://doi.org/10.1515/bmt-2019-0027
- 23. Gökler B, Ünal F, Pehlivantürk B, Kültür EÇ, Akdemir D et al. Reliability and validity of schedule for affective disorders and schizophrenia for school-age children-present and lifetime version-Turkish version (K-SADS-PL-T). Turkish Journal of Child and Adolescent Mental Health 2004;11(3): 109-116.
- 24. Hassani H. Singular spectrum analysis: methodology and comparison. Journal of Data Science 2007; 5: 239-257.
- 25. Mert C, Milnikov A. Singular spectrum analysis method as a universal filter. Application of Information and Communication Technologies, 2011. pp. 1-5.
- 26. Kesic S, Spasic SZ. Application of Higuchi’s fractal dimension from basic to clinical neurophysiology: A review. Computer Methods and Programs in Biomedicine 2016; 133: 55-70. https://doi.org/10.1016/j.cmpb.2016.05.014
- 27. Gomez C, Mediavilla A, Hornero R, Abasolo D, Fernandez A. Use of the Higuchi’s fractal dimension for the analysis of MEG recordings from Alzheimer’s disease patients. Medical Engineering and Physics 2009; 31(3): 306-313. https://doi. org/10.1016/j.medengphy.2008.06.010
- 28. Amady M, Horwat F. Evaluation of Hjorth parameters in forearm surface EMG analysis during an occupational repetitive task. Electroencephalography and Clinical Neurophysiology 1996; 101: 181-183. https://doi.org/10.1016/0924-980X(96)00316-5
- 29. Mohammed M, Khan MB, Bashier EBM. Machine learning: algorithms and applications, CRC Press; 2016. https://doi. org/10.1201/9781315371658
APA | Latifoğlu F, ESAS M, ILERI R, ÖZMEN s, Demirci E (2022). Using electrooculography with visual stimulus tracking test in diagnosing of ADHD: findings from machine learning algorithms. , 1616 - 1626. 10.55730/1300-0144.5502 |
Chicago | Latifoğlu Fatma,ESAS Mustafa Yasin,ILERI Ramis,ÖZMEN sevgi,Demirci Esra Using electrooculography with visual stimulus tracking test in diagnosing of ADHD: findings from machine learning algorithms. (2022): 1616 - 1626. 10.55730/1300-0144.5502 |
MLA | Latifoğlu Fatma,ESAS Mustafa Yasin,ILERI Ramis,ÖZMEN sevgi,Demirci Esra Using electrooculography with visual stimulus tracking test in diagnosing of ADHD: findings from machine learning algorithms. , 2022, ss.1616 - 1626. 10.55730/1300-0144.5502 |
AMA | Latifoğlu F,ESAS M,ILERI R,ÖZMEN s,Demirci E Using electrooculography with visual stimulus tracking test in diagnosing of ADHD: findings from machine learning algorithms. . 2022; 1616 - 1626. 10.55730/1300-0144.5502 |
Vancouver | Latifoğlu F,ESAS M,ILERI R,ÖZMEN s,Demirci E Using electrooculography with visual stimulus tracking test in diagnosing of ADHD: findings from machine learning algorithms. . 2022; 1616 - 1626. 10.55730/1300-0144.5502 |
IEEE | Latifoğlu F,ESAS M,ILERI R,ÖZMEN s,Demirci E "Using electrooculography with visual stimulus tracking test in diagnosing of ADHD: findings from machine learning algorithms." , ss.1616 - 1626, 2022. 10.55730/1300-0144.5502 |
ISNAD | Latifoğlu, Fatma vd. "Using electrooculography with visual stimulus tracking test in diagnosing of ADHD: findings from machine learning algorithms". (2022), 1616-1626. https://doi.org/10.55730/1300-0144.5502 |
APA | Latifoğlu F, ESAS M, ILERI R, ÖZMEN s, Demirci E (2022). Using electrooculography with visual stimulus tracking test in diagnosing of ADHD: findings from machine learning algorithms. Turkish Journal of Medical Sciences, 52(5), 1616 - 1626. 10.55730/1300-0144.5502 |
Chicago | Latifoğlu Fatma,ESAS Mustafa Yasin,ILERI Ramis,ÖZMEN sevgi,Demirci Esra Using electrooculography with visual stimulus tracking test in diagnosing of ADHD: findings from machine learning algorithms. Turkish Journal of Medical Sciences 52, no.5 (2022): 1616 - 1626. 10.55730/1300-0144.5502 |
MLA | Latifoğlu Fatma,ESAS Mustafa Yasin,ILERI Ramis,ÖZMEN sevgi,Demirci Esra Using electrooculography with visual stimulus tracking test in diagnosing of ADHD: findings from machine learning algorithms. Turkish Journal of Medical Sciences, vol.52, no.5, 2022, ss.1616 - 1626. 10.55730/1300-0144.5502 |
AMA | Latifoğlu F,ESAS M,ILERI R,ÖZMEN s,Demirci E Using electrooculography with visual stimulus tracking test in diagnosing of ADHD: findings from machine learning algorithms. Turkish Journal of Medical Sciences. 2022; 52(5): 1616 - 1626. 10.55730/1300-0144.5502 |
Vancouver | Latifoğlu F,ESAS M,ILERI R,ÖZMEN s,Demirci E Using electrooculography with visual stimulus tracking test in diagnosing of ADHD: findings from machine learning algorithms. Turkish Journal of Medical Sciences. 2022; 52(5): 1616 - 1626. 10.55730/1300-0144.5502 |
IEEE | Latifoğlu F,ESAS M,ILERI R,ÖZMEN s,Demirci E "Using electrooculography with visual stimulus tracking test in diagnosing of ADHD: findings from machine learning algorithms." Turkish Journal of Medical Sciences, 52, ss.1616 - 1626, 2022. 10.55730/1300-0144.5502 |
ISNAD | Latifoğlu, Fatma vd. "Using electrooculography with visual stimulus tracking test in diagnosing of ADHD: findings from machine learning algorithms". Turkish Journal of Medical Sciences 52/5 (2022), 1616-1626. https://doi.org/10.55730/1300-0144.5502 |