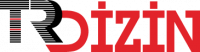
DIAGNOSING DISEASES FROM FINGERNAIL IMAGES
Yıl: 2022 Cilt: 30 Sayı: 3 Sayfa Aralığı: 464 - 470 Metin Dili: İngilizce DOI: 10.31796/ogummf.1111749 İndeks Tarihi: 05-01-2023
DIAGNOSING DISEASES FROM FINGERNAIL IMAGES
Öz: This paper investigates how people's finger and nail appearance helps diagnose various diseases, such as Darier's disease, Muehrcke's lines, alopecia areata, beau's lines, bluish nails, and clubbing, by image processing and deep learning techniques. We used a public dataset consisting of 17 different classes with 655 samples. We divided the dataset into three folds based on a widely used rule, the 0.7:0.2:0.1, for training, validation, and testing purposes. We tested the EfficientNet-B2 model for performance evaluation purposes by using Noisy-Student weights by setting the batch size and epochs as 32 and 1000. The model achieves a 72% accuracy score and 91% AUC score for test samples to detect fingernail diseases. The empirical findings in this study provide a new understanding that the EfficientNet-B2 model can categorize nail disease types through numerous classes.
Anahtar Kelime: TIRNAK GÖRÜNTÜLERİNDEN HASTALIK TEŞHİSİ
Öz: Bu makale, insanların parmak ve tırnak görünümünün Darier hastalığı, Muehrcke çizgileri, alopesi areata, beau çizgileri, mavimsi tırnaklar ve çomaklaşma gibi çeşitli hastalıkların görüntü işleme ve derin öğrenme teknikleriyle teşhis edilmesine nasıl yardımcı olduğunu araştırıyor. 655 örnekle 17 farklı sınıftan oluşan genel bir veri seti kullandık. Eğitim, doğrulama ve test amaçları için yaygın olarak kullanılan bir kural olan 0.7:0.2:0.1'e dayanarak veri setini üç kata böldük. Yığın boyutu ve devirleri 32 ve 1000 olarak ayarlayarak Gürültülü-Öğrenci ağırlıklarını kullanarak EfficientNet-B2 modelini performans değerlendirme amacıyla test ettik. Model, tırnak hastalıklarını algılamak için test numunelerinden %72 doğruluk puanı ve %91 AUC puanı elde ediyor. Bu çalışmadaki deneysel bulgular, EfficientNet-B2 modelinin tırnak hastalığı tiplerini çok sayıda sınıf aracılığıyla kategorize edebileceğine dair yeni bir anlayışı doğrulamaktadır.
Anahtar Kelime: Belge Türü: Makale Makale Türü: Araştırma Makalesi Erişim Türü: Erişime Açık
- Abdulhadi, J., Al-Dujaili, A., Humaidi, A. J., & Fadhel, M. A.- R. (2021). Human Nail Dıseases Classifıcation based on transfer learning.
- Atila, Ü., Uçar, M., Akyol, K., & Uçar, E. (2021). Plant leaf disease classification using EfficientNet deep learning model. Ecological Informatics, 61, 101182.
- Azman, K. A., & Kairuddin, W. N. H. W. (2022). Disease Classification by Human Fingernail Colour Image Processing. Evolution in Electrical and Electronic Engineering, 3(1), 180–190.
- Cammarasana, S., Nicolardi, P., & Patanè, G. (2022). Real- time denoising of ultrasound images based on deep learning. Medical & Biological Engineering & Computing, 1–16.
- Esteva, A., Kuprel, B., Novoa, R. A., Ko, J., Swetter, S. M., Blau, H. M., & Thrun, S. (2017). Dermatologist-level classification of skin cancer with deep neural networks. Nature, 542(7639), 115–118.
- Fangyu, L. I., & Hua, H. E. (2018). Assessing the accuracy of diagnostic tests. Shanghai Archives of Psychiatry, 30(3), 207.
- Farman, H., Ahmad, J., Jan, B., Shahzad, Y., Abdullah, M., & Ullah, A. (2022). EfficientNet-Based Robust Recognition of Peach Plant Diseases in Field Images. CMC-COMPUTERS MATERIALS & CONTINUA, 71(1), 2073–2089.
- Gómez-de-Mariscal, E., Garcia-López-de-Haro, C., Ouyang, W., Donati, L., Lundberg, E., Unser, M., ... Sage, D. (2021). DeepImageJ: A user-friendly environment to run deep learning models in ImageJ. Nature Methods, 18(10), 1192–1195.
- Gustisyaf, A. I., & Sinaga, A. (2021). Implementation of Convolutional Neural Network to Classification Gender based on Fingerprint. International Journal of Modern Education & Computer Science, 13(4).
- Hanh, B. T., Van Manh, H., & Nguyen, N.-V. (2022). Enhancing the performance of transferred efficientnet models in leaf image-based plant disease classification. Journal of Plant Diseases and Protection, 129(3), 623–634.
- Indi, T. S., & Patil, D. D. (2019). Nail Feature Analysis and Classification Techniques for Disease Detection. Int. J. Comput. Sci. Eng, 7(5), 1376–1383.
- Iqbal, A., Sharif, M., Yasmin, M., Raza, M., & Aftab, S. (2022). Generative adversarial networks and its applications in the biomedical image segmentation: a comprehensive survey. International Journal of Multimedia Information Retrieval, 1–36.
- Izadi, V., Morovati, M., Ranjbaran, G., & Homayounmajd, S. (2021). An Empirical Study of Nail Fungus Classification Using Deep and Traditional Machine Learning Methods. Journal of Bioengineering Research, 3(3), 16–23.
- Kaggle. (2022). Nail Dataset. Retrieved from https://www.kaggle.com/datasets/reubenindust rustech/nail-dataset-new
- Kovalev, V. A., Liauchuk, V. A., Voynov, D. M., & Tuzikov, A. V. (2021). Biomedical Image Recognition in Pulmonology and Oncology with the Use of Deep Learning. Pattern Recognition and Image Analysis, 31(1), 144–162.
- Li, B., Liu, B., Li, S., & Liu, H. (2022). An Improved EfficientNet for Rice Germ Integrity Classification and Recognition. Agriculture, 12(6), 863.
- Lundervold, A. S., & Lundervold, A. (2019). An overview of deep learning in medical imaging focusing on MRI. Zeitschrift Für Medizinische Physik, 29(2), 102–127.
- Maniyan, P., & Shivakumar, B. L. (2018). Early Disease Detection Through Nail Image Processing Based On Ensemble Of KNN Classifier And Image Features. IOSR Journal of Computer Engineering (IOSR-JCE), 20(3).
- Mansour, R. F., Althobaiti, M. M., & Ashour, A. A. (2021). Internet of things and synergic deep learning based biomedical tongue color image analysis for disease diagnosis and classification. IEEE Access, 9, 94769–94779.
- Marques, G., Ferreras, A., & de la Torre-Diez, I. (2022). An ensemble-based approach for automated medical diagnosis of malaria using EfficientNet. Multimedia Tools and Applications, 1–18.
- Mehra, M., D’Costa, S., D’Mello, R., George, J., & Kalbande, D. R. (2021). Leveraging Deep Learning for Nail Disease Diagnostic. 2021 4th Biennial International Conference on Nascent Technologies in Engineering (ICNTE), 1–5.
- Meijering, E. (2020). A bird’s-eye view of deep learning in bioimage analysis. Computational and Structural Biotechnology Journal, 18, 2312–2325.
- Nayak, D. R., Padhy, N., Mallick, P. K., Zymbler, M., & Kumar, S. (2022). Brain Tumor Classification Using Dense Efficient-Net. Axioms, 11(1), 34.
- Ravi, V., Acharya, V., & Alazab, M. (2022). A multichannel EfficientNet deep learning-based stacking ensemble approach for lung disease detection using chest X-ray images. Cluster Computing, 1–23.
- Safira, L., Irawan, B., & Setianingsih, C. (2019). K-Nearest Neighbour Classification and Feature Extraction GLCM for Identification of Terry’s Nail. 2019 IEEE International Conference on Industry 4.0, Artificial Intelligence, and Communications Technology (IAICT), 98–104.
- Sharma, S., Gupta, S., Gupta, D., Rashid, J., Juneja, S., Kim, J., & Elarabawy, M. M. (2022). Performance evaluation of the deep learning based convolutional neural network approach for the recognition of chest X-ray images. Frontiers in Oncology, 12.
- Tan, M., & Le, Q. V. (2019). EfficientNet: improving accuracy and efficiency through AutoML and model scaling. Google AI Blog.
- Thahira Banu, V., & Devi, M. R. (2021). Hybrid Classifier to Classify The Finger Nail Abnormalities. Information Technology In Industry, 9(1), 549–555.
- Thanikachalam, V., Shanthi, S., Kalirajan, K., Abdel- Khalek, S., Omri, M., & Ladhar, L. M. (2022). Intelligent Deep Learning Based Disease Diagnosis Using Biomedical Tongue Images. CMC-Computers Materials & Continua, 70(3), 5667–5681.
- Tsutsumi, K., Goshtasbi, K., Risbud, A., Khosravi, P., Pang, J. C., Lin, H. W., ... Abouzari, M. (2021). A web-based deep learning model for automated diagnosis of otoscopic images. Otology & Neurotology, 42(9), e1382--e1388.
- Venugopal, V., Joseph, J., Das, M. V., & Nath, M. K. (2022). An EfficientNet-based modified sigmoid transform for enhancing dermatological macro-images of melanoma and nevi skin lesions. Computer Methods and Programs in Biomedicine, 106935.
- Wang, J., Liu, Q., Xie, H., Yang, Z., & Zhou, H. (2021). Boosted efficientnet: Detection of lymph node metastases in breast cancer using convolutional neural networks. Cancers, 13(4), 661.
- Watson, C., Cooper, N., Palacio, D. N., Moran, K., & Poshyvanyk, D. (2022). A Systematic Literature Review on the Use of Deep Learning in Software Engineering Research. ACM Transactions on Software Engineering and Methodology (TOSEM), 31(2), 1–58.
- Yang, X., Hu, Q., & Li, S. (2020). Recognition and classification of damaged fingerprint based on deep learning fuzzy theory. Journal of Intelligent & Fuzzy Systems, 38(4), 3529–3537.
- Yani, M., & others. (2019). Application of transfer learning using convolutional neural network method for early detection of terry’s nail. Journal of Physics: Conference Series, 1201(1), 12052.
- Zhu, S., Lu, B., Wang, C., Wu, M., Zheng, B., Jiang, Q., ... Yang, W. (2022). Screening of common retinal diseases using six-category models based on EfficientNet. Frontiers in Medicine, 130.
APA | Can Z, isik s (2022). DIAGNOSING DISEASES FROM FINGERNAIL IMAGES. , 464 - 470. 10.31796/ogummf.1111749 |
Chicago | Can Zuhal,isik sahin DIAGNOSING DISEASES FROM FINGERNAIL IMAGES. (2022): 464 - 470. 10.31796/ogummf.1111749 |
MLA | Can Zuhal,isik sahin DIAGNOSING DISEASES FROM FINGERNAIL IMAGES. , 2022, ss.464 - 470. 10.31796/ogummf.1111749 |
AMA | Can Z,isik s DIAGNOSING DISEASES FROM FINGERNAIL IMAGES. . 2022; 464 - 470. 10.31796/ogummf.1111749 |
Vancouver | Can Z,isik s DIAGNOSING DISEASES FROM FINGERNAIL IMAGES. . 2022; 464 - 470. 10.31796/ogummf.1111749 |
IEEE | Can Z,isik s "DIAGNOSING DISEASES FROM FINGERNAIL IMAGES." , ss.464 - 470, 2022. 10.31796/ogummf.1111749 |
ISNAD | Can, Zuhal - isik, sahin. "DIAGNOSING DISEASES FROM FINGERNAIL IMAGES". (2022), 464-470. https://doi.org/10.31796/ogummf.1111749 |
APA | Can Z, isik s (2022). DIAGNOSING DISEASES FROM FINGERNAIL IMAGES. Eskişehir Osmangazi Üniversitesi mühendislik ve mimarlık fakültesi dergisi (online), 30(3), 464 - 470. 10.31796/ogummf.1111749 |
Chicago | Can Zuhal,isik sahin DIAGNOSING DISEASES FROM FINGERNAIL IMAGES. Eskişehir Osmangazi Üniversitesi mühendislik ve mimarlık fakültesi dergisi (online) 30, no.3 (2022): 464 - 470. 10.31796/ogummf.1111749 |
MLA | Can Zuhal,isik sahin DIAGNOSING DISEASES FROM FINGERNAIL IMAGES. Eskişehir Osmangazi Üniversitesi mühendislik ve mimarlık fakültesi dergisi (online), vol.30, no.3, 2022, ss.464 - 470. 10.31796/ogummf.1111749 |
AMA | Can Z,isik s DIAGNOSING DISEASES FROM FINGERNAIL IMAGES. Eskişehir Osmangazi Üniversitesi mühendislik ve mimarlık fakültesi dergisi (online). 2022; 30(3): 464 - 470. 10.31796/ogummf.1111749 |
Vancouver | Can Z,isik s DIAGNOSING DISEASES FROM FINGERNAIL IMAGES. Eskişehir Osmangazi Üniversitesi mühendislik ve mimarlık fakültesi dergisi (online). 2022; 30(3): 464 - 470. 10.31796/ogummf.1111749 |
IEEE | Can Z,isik s "DIAGNOSING DISEASES FROM FINGERNAIL IMAGES." Eskişehir Osmangazi Üniversitesi mühendislik ve mimarlık fakültesi dergisi (online), 30, ss.464 - 470, 2022. 10.31796/ogummf.1111749 |
ISNAD | Can, Zuhal - isik, sahin. "DIAGNOSING DISEASES FROM FINGERNAIL IMAGES". Eskişehir Osmangazi Üniversitesi mühendislik ve mimarlık fakültesi dergisi (online) 30/3 (2022), 464-470. https://doi.org/10.31796/ogummf.1111749 |