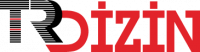
Rüzgâr enerjisi potansiyelini değerlendirirken önemli hususlar
Yıl: 2023 Cilt: 38 Sayı: 2 Sayfa Aralığı: 947 - 962 Metin Dili: Türkçe DOI: 10.17341/gazimmfd.1066351 İndeks Tarihi: 13-03-2023
Rüzgâr enerjisi potansiyelini değerlendirirken önemli hususlar
Öz: Rüzgâr rejimi dağılım modelinin belirlenmesi birkaç nedenden dolayı gereklidir, rüzgâr gücü çıktısını tahmin etmek en önemli konulardan biridir. Bu açıdan rüzgâr hızı dağılımını modellemek için Weibull, Gamma ve Rayleigh dağılımları en yaygın olarak kullanılan dağılımlardır. Ancak, tüm rüzgâr modellerini modellemede üstün olmayabilirler. Sonuç olarak, yerine geçecek dağılım fonksiyonlarının çalışılması gerekmektedir. Bu makale, rüzgâr hızı dağılımını tanımlamak için Weibull, Uç Değer, Ters Gauss, Lojistik, Log-Lojistik, Yarı-Normal, Burr Tipi XII, Genelleştirilmiş Uç Değer, Genelleştirilmiş Pareto ve T Konum-Ölçeği adlı on farklı dağılım fonksiyonlarını kapsamlı bir şekilde sunar. Ayrıca, her dağılımın parametre değerlerini optimize etmek için iki metasezgisel optimizasyon yöntemi olan Genetik Algoritması ve Parçacık Sürü Optimizasyonu kullanılmaktadır. Sunulan dağılımların iyi durumlarını (good-of-fitness) karşılaştırmak için yedi istatistiksel tanımlayıcı ile birlikte altı hata kriteri kullanılmıştır.
Anahtar Kelime: Important considerations while evaluating wind energy potential
Öz: Rayleigh, Gamma, and Weibull distributions are the most widely-used distributions for modeling wind speed distribution. However, they may not be outstanding for modeling all wind patterns. Consequently, substitute distribution functions are required to be studied. This study presents a comprehensive analysis of ten different distributions to represent wind speed patterns: Weibull, Extreme Value, Inverse Gaussian, Logistic, Log-Logistic, Half-Normal, Burr Type XII, Generalized Extreme Value, Generalized Pareto, and T Location-Scale. Additionally, two optimization methods, Genetic Algorithms and Particle Swarm Optimization, are utilized to select the optimal parameter values for each distribution. The good-of-fitness, six error measures, and seven statistical descriptors are employed.
Anahtar Kelime: Belge Türü: Makale Makale Türü: Araştırma Makalesi Erişim Türü: Erişime Açık
- Chang TP. Estimation of wind energy potential using different probability density functions. Applied Energy 2011; 88(5): 1848–1856.
- Wadi M, Elmasry W. Statistical analysis of wind energy potential using different estimation methods for Weibull parameters: a case study. Electrical Engineering 2021: 1–22. doi: https://doi.org/10.1007/s00202-021-01254-0
- Pishgar-Komleh S, Keyhani A, Sefeedpari P. Wind speed and power density analysis based on Weibull and Rayleigh distributions (a case study: Firouzkooh county of Iran). Renewable and sustainable energy reviews 2015; 42: 313–322, 2015.
- Morgan E. C., Lackner M., Vogel R. M., Baise L. G., Probability distributions for offshore wind speeds, Energy Convers. Manage., 52 (1), 15–26, 2011.
- Crutcher H. L., Baer L., Computations from elliptical wind distribution statistics, Journal of Applied Meteorology and Climatology, 1 (4), 522– 530, 1962.
- Dutta S., Genton M.G., A non-Gaussian multivariate distribution with all lower-dimensional Gaussians and related families, J. Multivar. Anal., 132, 82–93, 2014.
- Yuan K., Zhang K., Zheng Y., Li D., Wang Y., Yang Z., Irregular distribution of wind power prediction, J. Mod. Power Syst. Clean Energy, 6 (6), 1172–1180, 2018.
- Garcia A., Torres J., Prieto E., De Francisco A., Fitting wind speed distributions: a case study, Sol. Energy, 62 (2), 139–144, 1998.
- Scerri E., Farrugia R., Wind data evaluation in the Maltese Islands, Renewable energy, 7 (1), 109–114, 1996.
- Ahsanullah M., Alzaatreh A., Some Characterizations of the Log- Logistic Distribution, Stochastics and Quality Control, 33 (1), 23–29, 2018.
- Yilmaz V., Çelik H. E., A statistical approach to estimate the wind speed distribution: the case of Gelibolu region, Doğuş Üniversitesi Dergisi, 9 (1), 122–132, 2011.
- Alavi O., Mohammadi K., Mostafaeipour A., Evaluating the suitability of wind speed probability distribution models: A case of study of east and southeast parts of Iran, Energy Convers. Manage., 119, 101–108, 2016.
- Mert I., Karakuş C., A statistical analysis of wind speed data using Burr, generalized gamma, and Weibull distributions in Antakya, Turkey, Turkish Journal of Electrical Engineering & Computer Sciences, 23 (6), 1571–1586, 2015.
- Rajabi M., Modarres R., Extreme value frequency analysis of wind data from Isfahan, Iran, J. Wind Eng. Ind. Aerodyn., 96 (1), 78–82, 2008.
- El-Shanshoury G. I., Ramadan A., Estimation of extreme value analysis of wind speed in the North-Western coast of Egypt, Arab J Nucl Sci Appl, 45, 265–274, 2012.
- Nagatsuka H., Balakrishnan N., A method for estimating parameters and quantiles of the three-parameter inverse Gaussian distribution based on statistics invariant to unknown location, J. Stat. Comput. Simul., 84 (11), 2361–2377, 2014.
- Alayat M. M., Kassem Y., Çamur H., Assessment of wind energy potential as a power generation source: A case study of eight selected locations in Northern Cyprus, Energies, 11 (10), 2697, 2018.
- Lee D., Baldick R., Probabilistic wind power forecasting based on the laplace distribution and golden search, IEEE/PES Transmission and Distribution Conference and Exposition (T&D), 1–5, 2016.
- Wallner M., A half-normal distribution scheme for generating functions, Eur. J. Comb., 87, 103138, 2020.
- Gómez Y. M., Vidal I., A generalization of the half-normal distribution, Applied Mathematics-A Journal of Chinese Universities, 31 (4), 409– 424, 2016.
- Ayuketang Arreyndip N., Joseph E., Generalized extreme value distribution models for the assessment of seasonal wind energy potential of Debuncha, Cameroon, J. Renewable Energy, 2016, 2016.
- Sarkar A., Deep S., Datta D., Vijaywargiya A., Roy R., Phanikanth V., Weibull and Generalized Extreme Value Distributions for Wind Speed Data Analysis of Some Locations in India, KSCE J. Civ. Eng., 23 (8), 3476–3492, 2019.
- Singh V. P., Guo H., Parameter estimation for 3-parameter generalized Pareto distribution by the principle of maximum entropy (POME), Hydrol. Sci. J., 40 (2), 165–181, 1995.
- D’Amico G., Petroni F., Prattico F., Wind speed prediction for wind farm applications by extreme value theory and copulas, J. Wind Eng. Ind. Aerodyn., 145, 229–236, 2015.
- Zhang J., Wind power fluctuation characteristics of wind farms, Proceedings of the 2015 International Conference on Electrical, Computer Engineering and Electronics, 2015.
- Sohoni V., Gupta S., Nema R., A comparative analysis of wind speed probability distributions for wind power assessment of four sites, Turkish Journal of Electrical Engineering & Computer Sciences, 24 (6), 4724–4735, 2016.
- Wadi M., Kekezoglu B., Baysal M., Tur M. R., Shobole A., Feasibility Study of Wind Energy Potential in Turkey: Case Study of Catalca District in Istanbul, 2nd International Conference on Smart Grid and Renewable Energy (SGRE), 1–6, 2019.
- Gul M., Tai N., Huang W., Nadeem M. H., Yu M., Evaluation of Wind Energy Potential Using an Optimum Approach based on Maximum Distance Metric, Sustainability, 12 (5), 1999, 2020.
- Jung C., Schindler D., Global comparison of the goodness-of-fit of wind speed distributions, Energy Convers. Manage., 133, 216–234, 2017.
- Saxena B. K., Rao K. V. S., Comparison of Weibull parameters computation methods and analytical estimation of wind turbine capacity factor using polynomial power curve model: case study of a wind farm, Renewables: Wind, Water, and Solar, 2 (1), 1–11, 2015.
- Pobočı́ková I., Sedliačková Z., Michalková M., Application of four probability distributions for wind speed modeling, Procedia Eng., 192, 713–718, 2017.
- Drobinski P., Coulais C., Jourdier B., Surface wind-speed statistics modelling: Alternatives to the Weibull distribution and performance evaluation, Boundary Layer Meteorol., 157 (1), 97–123, 2015.
- Abolpour B., Abolpour B., Bakhshi H., Yaghobi M., An Appropriate Extreme Value Distribution for the Annual Extreme Gust Winds Speed, J Fundam Renewable Energy Appl, 7 (223), 2, 2017.
- Quan Y., Wang F., Gu M., A method for estimation of extreme values of wind pressure on buildings based on the generalized extreme-value theory, Math. Probl. Eng., 2014, 2014.
- Zhao X., Zhang Z., Cheng W., Zhang P., A new parameter estimator for the Generalized Pareto distribution under the peaks over threshold framework, Mathematics, 7 (5), 406, 2019.
- An Y., Pandey M., A comparison of methods of extreme wind speed estimation, J. Wind Eng. Ind. Aerodyn., 93 (7), 535–545, 2005.
- Xiao Y., Li Q., Li Z., Chow Y., Li G., Probability distributions of extreme wind speed and its occurrence interval, Eng. Struct., 28 (8), 1173–1181, 2006.
- Krishnamoorthy R., Udhayakumar K., Raju K., Elavarasan R. M., Mihet-Popa L., An Assessment of Onshore and Offshore Wind Energy Potential in India Using Moth Flame Optimization, Energies, 13 (12), 1–41, 2020.
- Zhang L., Li Q., Guo Y., Yang Z., Zhang L., An investigation of wind direction and speed in a featured wind farm using joint probability distribution methods, Sustainability, 10 (12), 4338, 2018.
- Ahsanullah M., Alzaatreh A., Parameter estimation for the log-logistic distribution based on order statistics, REVSTAT Statistical Journal, 16, 429–443, 2018.
- Lin L., Ang A. H., Fan W., Xia D., A probability-based analysis of wind speed distribution and related structural response in southeast China, Struct. Infrastruct. Eng., 15 (1), 14–26, 2019.
- Markose S., Alentorn A., The generalized extreme value distribution, implied tail index, and option pricing, The Journal of Derivatives, 18 (3), 35–60, 2011.
- Kang S., Song J., Parameter and quantile estimation for the generalized Pareto distribution in peaks over threshold framework, J. Korean Stat. Soc., 46, 487–501, 2017.
- Brabson B., Palutikof J., Tests of the generalized Pareto distribution for predicting extreme wind speeds, J. Appl. Meteorol., 39 (9), 1627–1640, 2000.
- Holmes J., Moriarty W., Application of the generalized Pareto distribution to extreme value analysis in wind engineering, J. Wind Eng. Ind. Aerodyn., 83 (1–3), 1–10, 1999.
- Steinkohl C., Davis R. A., Klüppelberg C., Extreme value analysis of multivariate high-frequency wind speed data, J. Stat. Theory Pract. , 7 (1), 73–94, 2013.
- Li K., Kang X., Liu L., Two-stage Optimal Sizing of Hybrid Energy Storage System for Wind Energy Integration in Microgrid, 12th IEEE PES Asia-Pacific Power and Energy Engineering Conference (APPEEC), 1–5, 2020.
- Lin W., Wen J., Cheng S., Lee W.-J., An investigation on the active- power variations of wind farms, IEEE Transactions on Industry Applications, 48 (3), 1087–1094, 2012.
- Elmasry W., Akbulut A., Zaim A. H., Empirical study on multiclass classification-based network intrusion detection, Comput. Intell., 35 (4), 919–954, 2019.
- Elmasry W., Akbulut A., Zaim A. H., Comparative evaluation of different classification techniques for masquerade attack detection, Int. J. Inf. Comput. Secur., 13 (2), 187–209, 2020.
- Wadi M., Elmasry W., Modeling of wind energy potential in Marmara region using different statistical distributions and genetic algorithms, International Conference on Electric Power Engineering–Palestine (ICEPE-P), IEEE, 1–7, 2021.
- Wadi M., Five different distributions and metaheuristics to model wind speed distribution, Journal of Thermal Engineering, 7 (Supp 14), 1898- 1920, 2021.
- Wadi M., Elmasry W., Shobole A, Tur M. R., Bayindir R., Shahinzadeh H., Wind Energy Potential Approximation with Various Metaheuristic Optimization Techniques Deployment, 7th International Conference on Signal Processing and Intelligent Systems (ICSPIS), IEEE, 1-6, 2021.
- Mostafaeipour A., Feasibility study of harnessing wind energy for turbine installation in province of Yazd in Iran, Renewable Sustainable Energy Rev., 14 (1), 93–111, 2010.
- Okorie M. E., Inambao F., Chiguvare Z., Evaluation of wind shear coefficients, surface roughness and energy yields over inland locations in Namibia, Procedia Manuf., 7, 630–638, 2017.
- Gualtieri G., Secci S., Wind shear coefficients, roughness length and energy yield over coastal locations in Southern Italy, Renewable Energy, 36 (3), 1081–1094, 2011.
- Lackner M. A., Rogers A. L., Manwell J. F., McGowan J. G., A new method for improved hub height mean wind speed estimates using short-term hub height data, Renewable Energy, 35 (10), 2340–2347, 2010.
- Laban O. N., Maghanga C. M., Joash K., Determination of the surface roughness parameter and wind shear exponent of Kisii Region from the on-site measurement of wind profiles, J. Energy, 2019, 2019.
- Gualtieri G., Secci S., Comparing methods to calculate atmospheric stability-dependent wind speed profiles: A case study on coastal location, Renewable Energy, 36 (8), 2189–2204, 2011.
- Ağçay M., Attay F., Türkiye’nin Elektrik Enerjisi Arz Talep Dengesinin Tespiti, Üretim Projeksiyonuna Yönelik Rüzgâr Elektrik Santrali Tasarımı RES’in Kurulum Maliyetlerinin ve Üretim Parametrelerinin Analizinin Matlab & Simulink ile Yazılan Programda Yapılması, Lisans Tezi, Yıldız Teknik Üniversitesi, Elektrik-Elektronik Fakültesi, Istanbul, Turkey, 2007.
- Gul M., Tai N., Huang W., Nadeem M. H., Yu M., Assessment of wind power potential and economic analysis at hyderabad in Pakistan: powering to local communities using wind power, Sustainability, 11 (5), 1391, 2019.
- Willmott C. J., Matsuura K., Advantages of the mean absolute error (MAE) over the root mean square error (RMSE) in assessing average model performance, Climate research, 30 (1), 79–82, 2005.
- Hyndman R. J., Koehler A. B., Another look at measures of forecast accuracy, Int. J. Forecasting, 22 (4), 679–688, 2006.
- Daş M., Balpetek N., Kavak Akpınar E., Akpınar S., Investigation of wind energy potential of different provinces found in Turkey and establishment of predictive model using support vector machine regression with the obtained results, Journal of the Faculty of Engineering and Architecture of Gazi University, 34 (4), 2203-2214, 2019.
- Akpınar E.K., Balpetek N., Statistical analysis of wind energy potential of Elazığ province according to Weibull and Rayleigh distributions, Journal of the Faculty of Engineering and Architecture of Gazi University, 34 (1), 569-580, Mar. 2019.
- Papoulis A., Pillai S. U., Probability, random variables, and stochastic processes, Tata McGraw-Hill Education, 2002.
- Soong T. T., Fundamentals of probability and statistics for engineers, John Wiley & Sons, 2004.
- DeCarlo L. T., On the meaning and use of kurtosis, Psychological methods, 2 (3), 292, 1997.
- Irwanto M., Gomesh N., Mamat M., Yusoff Y., Assessment of wind power generation potential in Perlis, Malaysia, Renewable Sustainable Energy Rev., 38, 296–308, 2014.
- Saeidi D., Mirhosseini M., Sedaghat A., Mostafaeipour A., Feasibility study of wind energy potential in two provinces of Iran: North and South Khorasan, Renewable Sustainable Energy Rev., 15 (8), 3558– 3569, 2011.
APA | Wadi M, Elmasry W, Tamyiğit F (2023). Rüzgâr enerjisi potansiyelini değerlendirirken önemli hususlar. , 947 - 962. 10.17341/gazimmfd.1066351 |
Chicago | Wadi Mohammed,Elmasry Wisam,Tamyiğit Furkan Ahmet Rüzgâr enerjisi potansiyelini değerlendirirken önemli hususlar. (2023): 947 - 962. 10.17341/gazimmfd.1066351 |
MLA | Wadi Mohammed,Elmasry Wisam,Tamyiğit Furkan Ahmet Rüzgâr enerjisi potansiyelini değerlendirirken önemli hususlar. , 2023, ss.947 - 962. 10.17341/gazimmfd.1066351 |
AMA | Wadi M,Elmasry W,Tamyiğit F Rüzgâr enerjisi potansiyelini değerlendirirken önemli hususlar. . 2023; 947 - 962. 10.17341/gazimmfd.1066351 |
Vancouver | Wadi M,Elmasry W,Tamyiğit F Rüzgâr enerjisi potansiyelini değerlendirirken önemli hususlar. . 2023; 947 - 962. 10.17341/gazimmfd.1066351 |
IEEE | Wadi M,Elmasry W,Tamyiğit F "Rüzgâr enerjisi potansiyelini değerlendirirken önemli hususlar." , ss.947 - 962, 2023. 10.17341/gazimmfd.1066351 |
ISNAD | Wadi, Mohammed vd. "Rüzgâr enerjisi potansiyelini değerlendirirken önemli hususlar". (2023), 947-962. https://doi.org/10.17341/gazimmfd.1066351 |
APA | Wadi M, Elmasry W, Tamyiğit F (2023). Rüzgâr enerjisi potansiyelini değerlendirirken önemli hususlar. Gazi Üniversitesi Mühendislik Mimarlık Fakültesi Dergisi, 38(2), 947 - 962. 10.17341/gazimmfd.1066351 |
Chicago | Wadi Mohammed,Elmasry Wisam,Tamyiğit Furkan Ahmet Rüzgâr enerjisi potansiyelini değerlendirirken önemli hususlar. Gazi Üniversitesi Mühendislik Mimarlık Fakültesi Dergisi 38, no.2 (2023): 947 - 962. 10.17341/gazimmfd.1066351 |
MLA | Wadi Mohammed,Elmasry Wisam,Tamyiğit Furkan Ahmet Rüzgâr enerjisi potansiyelini değerlendirirken önemli hususlar. Gazi Üniversitesi Mühendislik Mimarlık Fakültesi Dergisi, vol.38, no.2, 2023, ss.947 - 962. 10.17341/gazimmfd.1066351 |
AMA | Wadi M,Elmasry W,Tamyiğit F Rüzgâr enerjisi potansiyelini değerlendirirken önemli hususlar. Gazi Üniversitesi Mühendislik Mimarlık Fakültesi Dergisi. 2023; 38(2): 947 - 962. 10.17341/gazimmfd.1066351 |
Vancouver | Wadi M,Elmasry W,Tamyiğit F Rüzgâr enerjisi potansiyelini değerlendirirken önemli hususlar. Gazi Üniversitesi Mühendislik Mimarlık Fakültesi Dergisi. 2023; 38(2): 947 - 962. 10.17341/gazimmfd.1066351 |
IEEE | Wadi M,Elmasry W,Tamyiğit F "Rüzgâr enerjisi potansiyelini değerlendirirken önemli hususlar." Gazi Üniversitesi Mühendislik Mimarlık Fakültesi Dergisi, 38, ss.947 - 962, 2023. 10.17341/gazimmfd.1066351 |
ISNAD | Wadi, Mohammed vd. "Rüzgâr enerjisi potansiyelini değerlendirirken önemli hususlar". Gazi Üniversitesi Mühendislik Mimarlık Fakültesi Dergisi 38/2 (2023), 947-962. https://doi.org/10.17341/gazimmfd.1066351 |