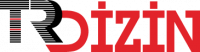
Görüntü-Metre ile görüntü işleme tabanlı mesafe ölçümü
Yıl: 2023 Cilt: 38 Sayı: 2 Sayfa Aralığı: 1129 - 1140 Metin Dili: Türkçe DOI: 10.17341/gazimmfd.979121 İndeks Tarihi: 13-03-2023
Görüntü-Metre ile görüntü işleme tabanlı mesafe ölçümü
Öz: Günümüzde görüntü sensörleri (kameralar), görüntü analizi (sınıflandırma, segmentasyon vb.) ve sentezi (nesne tespit, takip, mesafe tespiti vb.) için yaygın olarak kullanılmaktadır. Çalışmada lazer-metre, lidar-metre, radar ve benzeri endüstriyel amaçlar için kullanılabilecek, görüntü işleme tabanlı bir ölçüm cihazının (Image-meter) geliştirilmesi için teorik temellerin atılması amaçlanmaktadır. Bu amaçla literatürdeki görüntü işleme tabanlı mesafe tespit yöntemleri incelenmiştir. Bu yöntemlerin başarımını olumsuz etkileyen temel etkenler tespit edilmiş, bu etkenlerden etkilenmeyen yeni bir yöntem geliştirilmiştir. Geliştirilmesi planlanan ölçüm cihazı teorik temellere oturtulmuştur. Bu teorik temellerin işletilmesi donanımsal ve yazılımsal bileşenlere dayandırılmıştır. Çalışmada bu teorik temeller verilmiş, donanımsal ve yazılımsal bileşenlerin tasarımları gerçekleştirilmiştir. 1-1000m için yapılan hesaplamalar sonucunda %0.2’nin altında başarı oranına ulaşılabileceği belirlenmiştir. Donanımsal ve yazılımsal bileşenlerin bu hata oranını artıracağı aşikardır. Bu hatalar standart ve random hatalardan oluşacaktır. Çalışmada bu hatalar öngörülmüş ve mesafe ölçüm denklemine ilave edilmiştir. Öngörülen hataların tespiti donanımsal prototipin ve yazılım bileşenlerin geliştirilmesi ile gelecek çalışmada belirlenecektir.
Anahtar Kelime: Image processing based distance measurement with Image Meter
Öz: Currently, image sensors (cameras), are widely used for image analysis (classification, segmentation, etc.) and synthesis (object detection, tracking, distance detection, etc.). The aim of the study is to lay the theoretical foundations for the development of an image meter based measurement device (image meter) that can be used for laser-meter, lidar-meter, radar and similar industrial purposes. For this purpose, distance detection methods based on image processing in the literature have been studied. The main factors that negatively affect the performance of these methods have been identified, and a new method has been developed that is not affected by these factors. The measuring device, which is planned to be developed, is based on theoretical foundations. The operation of these theoretical foundations is based on hardware and software components. In the study, these theoretical foundations were given and designs of hardware and software components were realized. In study, as a result of calculations made for 1-1000m, it was determined that a success rate below 0.2% could be achieved. The study consists of an electro-mechanical component have hardware and software projects. It is obvious that errors caused by hardware and software components will reduce the success rate. These errors will consist of standard and random errors. In the study, these errors are foreseen and added to the distance measurement equation. Detection of predicted errors will be determined in the future study with the development of the hardware prototype and software components.
Anahtar Kelime: Belge Türü: Makale Makale Türü: Araştırma Makalesi Erişim Türü: Erişime Açık
- Vyas, A., Yu, S., & Paik, J., Fundamentals of digital image processing, In Multiscale Transforms with Application to Image Processing, 3-11, Singapore, 2018.
- Kurnaz Ç., Gül B.K., Determination of the relationship between sodium ring width on iris and cholesterol level, Journal of the Faculty of Engineering and Architecture of Gazi University, 33 (4), 1557-1568, 2018.
- Kılınç M., Gözde H., Detection of buried anti-personnel mines in thermal images by circular hough transformation supported active thermography method, Journal of the Faculty of Engineering and Architecture of Gazi University, 35 (2), 697–707, 2020.
- Ülkir O., Ertuǧrul I., Akkuş N., Characterization of electrothermal micro-actuator using image processing algorithm, Journal of the Faculty of Engineering and Architecture of Gazi University, 36 (2), 1159–1170, 2021.
- Yanik, H., Turan, B., Mesafe Ölçümünde Image -Metre: Görüntü İşleme ile Mesafe Tespit Yöntemlerinde Eksik Ne ?, International Conference on Data Science and Applications, 4, 480-486, 2021.
- Selçuk T., Çolakoğlu A.S., Alkan A., Bread texture analysis and development of user interface using image processing techniques, Journal of the Faculty of Engineering and Architecture of Gazi University, 33 (1), 31–41, 2018.
- Takatsuka, M, West, G. A. W., Venkatesh, S., Caelli, T. M., Low-cost interactive active range finder, IEEE Computer Society Conference on Computer Vision and Pattern Recognition 14 (3), 139–144, 2003.
- Phelawan, J.,Kittisut, P., Pornsuwancharoen, N., A new technique for distance measurement of between vehicles to vehicles by plate car using image processing, Procedia Eng., 32, 348–353, 2012.
- Seshadrinathan, K., Nestares, O., Wu, Y., Corporation, I., Clara, S., Accurate measurement of point to point distances in 3D camera images, International Symposium on Electronic Imaging, Digital Photography and Mobile Imaging XIII, 20–25, 2017.
- Hassan, M.F.A., Hussain, A., Saad, M.M.H., Win, K., 3D distance measurement accuracy on low-cost stereo camera, Science International, 29 (3), 599-605, 2017.
- Shortis, M., Calibration techniques for accurate measurements by underwater camera systems, Sensors, 15 (12), 30810–30827, 2015.
- Katada, K., Chen, S., Zhang, L., The triangulation of toe-in style stereo camera, In The 2nd International Conference on Intelligent Systems and Image Processing, 18–21, 2014.
- Oh, J. H., Park, J., Lee, S. H., Lee, B. H., & Park, J. I. Error modeling of depth measurement using FIR stereo camera systems, 2013.
- Ashoori, M. E., & Mahlouji, M., Measuring the Distance between the Two Vehicles Using Stereo Vision with Optical Axes Cross. Modern Applied Science, 12 (165), 10-5539, 2017.
- Yamaguti, N., Oe, S., Terada, K., A method of distance measurement by using monocular camera. In Proceedings of the 36th SICE annual conference. IEEE, International session papers, 1255-1260, 1997.
- Mahammed, M. A., Melhum, A. I., & Kochery, F. A., Object distance measurement by stereo vision. International Journal of Science and Applied Information Technology (IJSAIT), 2 (2), 05-08, 2013.
- Theodosis, P., Wilson, L., & Cheng, S., EE368 Final Project: Road Sign Detection and Distance Estimation in Autonomous Car Application, 2013.
- Gan, Y., Xu, X., Sun, W., & Lin, L., Monocular depth estimation with affinity, vertical pooling, and label enhancement. In Proceedings of the European Conference on Computer Vision, 224-239, 2018.
- Liang, Z., Feng, Y., Guo, Y., Liu, H., Chen, W., Qiao, L., Zhang, J., Learning for disparity estimation through feature constancy, In Proceedings of the IEEE conference on computer vision and pattern recognition, 2811-2820, 2018.
- Zeglazi, O., Rziza, M., Amine, A., & Demonceaux, C., Efficient Dense Disparity Map Reconstruction using Sparse Measurements. In 13th International Joint Conference on Computer Vision, Imaging and Computer Graphics Theory and Applications (VISAPP 2018)., 2018.
- Mayer, N., Ilg, E., Hausser, P., Fischer, P., Cremers, D., Dosovitskiy, A., Brox, T., A large dataset to train convolutional networks for disparity, optical flow, and scene flow estimation, In Proceedings of the IEEE conference on computer vision and pattern recognition, 4040- 4048, 2016.
- Fan, R., Ai, X., Dahnoun, N., Road surface 3D reconstruction based on dense subpixel disparity map estimation. IEEE Transactions on Image Processing, 27 (6), 3025-3035.2018.
- Hantao, L., Distance determination from pairs of ımages from low cost cameras, The University of Edinburgh School of Engineering and Electronics MSc in Signal Processing and Communication MSc Project Mission Statement, 1–95, 2005.
- Solak, S., Bolat, E. D., Distance estimation using stereo vision for indoor mobile robot applications, In 2015 9th International Conference on Electrical and Electronics Engineering IEEE, (ELECO), 685-688, 2016. Nagar, S., Verma, J. Distance measurement using stereo vision, International Journal of Electrical and Electronics Engineers, 7 (01).2015.
- Lai, X. B., Wang, H. S., & Xu, Y. H., A real-time range finding system with binocular stereo vision, International Journal of Advanced Robotic Systems, 9 (1), 26, 2012.
- Ummenhofer, B., Zhou, H., Uhrig, J., Mayer, N., Ilg, E., Dosovitskiy, A., Brox, T., Demon: Depth and motion network for learning monocular stereo, In Proceedings of the IEEE conference on computer vision and pattern recognition, 5038-5047, 2017.
- Vedula, S., Rander, P., Collins, R., Kanade, T., Three-dimensional scene flow, IEEE transactions on pattern analysis and machine intelligence, 27 (3), 475-480, 2005.
- Budiharto, W., Santoso, A., Purwanto, D., Jazidie, A., Multiple moving obstacles avoidance of service robot using stereo vision, TELKOMNIKA (Telecommunication Computing Electronics and Control), 9 (3), 433-444, 2011.
- Montiel-Ross, O., Sepúlveda, R., Castillo, O., Quiñones, J., Efficient stereoscopic video matching and map reconstruction for a wheeled mobile robot, International Journal of Advanced Robotic Systems, 9 (4), 120, 2012.
- Stein, G. P., Gdalyahu, Y., Shashua, A., Stereo-assist: Top-down stereo for driver assistance systems, In 2010 IEEE Intelligent Vehicles Symposium, IEEE, 723-730, 2010.
- Campbell, N. D., Vogiatzis, G., Hernández, C., Cipolla, R., Using multiple hypotheses to improve depth-maps for multi-view stereo, In European Conference on Computer Vision, Springer, Berlin, Heidelberg, 766-779, 2008.
- Hsu, T. S., Wang, T. C., An improvement stereo vision images processing for object distance measurement. International Journal of Automation and Smart Technology, 5(2), 85-90, 2015.
- Robert, L., Deriche, R., Dense depth map reconstruction: A minimization and regularization approach which preserves discontinuities. In European Conference on Computer Vision, Springer, Berlin, Heidelberg, 439-451, 1996.
- Mancini, M., Costante, G., Valigi, P., Ciarfuglia, T. A., Delmerico, J., Scaramuzza, D., Toward domain independence for learning-based monocular depth estimation, IEEE Robotics and Automation Letters, 2 (3), 1778-1785, 2017.
- Tao, M. W., Hadap, S., Malik, J., Ramamoorthi, R., Depth from combining defocus and correspondence using light-field cameras. In Proceedings of the IEEE International Conference on Computer Vision, 673-680, 2013.
- Kytö, M., Nuutinen, M., Oittinen, P., Method for measuring stereo camera depth accuracy based on stereoscopic vision, In Three- Dimensional Imaging, Interaction, and Measurement, 7864, 168-176, 2011.
- He, Y., Liang, B., Zou, Y., He, J., & Yang, J., Depth errors analysis and correction for time-of-flight (ToF) cameras. Sensors, 17 (1), 92, 2017.
- Holzmann, C., Hochgatterer, M., Measuring distance with mobile phones using single-camera stereo vision, In 2012 32nd International conference on distributed computing systems workshops IEEE., 88-93, 2012.
- Lee, S., Lee, J., Hayes, M.H., Katsaggelos, A.K., Paik, J., Single camera-based full depth map estimation using color shifting property of a multiple color-filter aperture, In 2012 IEEE International Conference on Acoustics, Speech and Signal Processing IEEE. (ICASSP), 801-804, 2012.
- Wedel, U. Franke, J. Klappstein, T. Brox, and D. Cremers, Realtime depth estimation and obstacle detection from monocular video, Pattern Recognit, 475–484, 2006.
- Jiao, J., Cao, Y., Song, Y., Lau, R., Look deeper into depth: Monocular depth estimation with semantic booster and attention-driven loss. In Proceedings of the European conference on computer vision (ECCV), 53-69, 2018.
- Soyaslan, M., Object distance detection through disparity map in stereo camera system, Sakarya University Journal of Science, 20 (2), 111-119, 2016.
- Reddy Mopuri, K., Krishna Uppala, P., Venkatesh Babu, R., Ask, acquire, and attack: Data-free uap generation using class impressions. arXiv e-prints, arXiv-1808, 2018.
- Srijha, R., Methodology for distance measurement : A Comparative Study, International Journal of Electronics, Electrical and Computational System IJEECS, 6 (8), 451–457, 2017.
- Chen, K. Y., Tsung, P. K., Lin, P. C., Yang, H. J., Chen, L. G., Hybrid motion/depth-oriented inpainting for virtual view synthesis in multiview applications, In 2010 3DTV-Conference: The True Vision- Capture, Transmission and Display of 3D Video IEEE., 1-4, 2010.
- Bai, M., Luo, W., Kundu, K., Urtasun, R., Exploiting semantic information and deep matching for optical flow. In European Conference on Computer Vision Springer, Cham, 154-170, 2016.
- Murphey, Y. L., Chen, J., Crossman, J., Zhang, J., Richardson, P., Sieh, L., DepthFinder, a real-time depth detection system for aided driving. In Proceedings of the IEEE Intelligent Vehicles Symposium IEEE., 122- 127, 2000.
- Rahman, A., Salam, A., Islam, M., Sarker, P., An image based approach to compute object distance. International Journal of Computational Intelligence Systems, 1 (4), 304-312, 2008.
- Uslu, O., Özkan, E.., Ip CCTV Sistemlerinde pixel (ppm) hesaplaması ve doğru çözünürlük tespiti, https://www.emo.org.tr/ekler/a48e0098ca1a33f_ek.pdf. yayın tarihi 2016, Erişim tarihi 01.08.2020.
APA | YANIK H, turan b (2023). Görüntü-Metre ile görüntü işleme tabanlı mesafe ölçümü. , 1129 - 1140. 10.17341/gazimmfd.979121 |
Chicago | YANIK Haydar,turan bülent Görüntü-Metre ile görüntü işleme tabanlı mesafe ölçümü. (2023): 1129 - 1140. 10.17341/gazimmfd.979121 |
MLA | YANIK Haydar,turan bülent Görüntü-Metre ile görüntü işleme tabanlı mesafe ölçümü. , 2023, ss.1129 - 1140. 10.17341/gazimmfd.979121 |
AMA | YANIK H,turan b Görüntü-Metre ile görüntü işleme tabanlı mesafe ölçümü. . 2023; 1129 - 1140. 10.17341/gazimmfd.979121 |
Vancouver | YANIK H,turan b Görüntü-Metre ile görüntü işleme tabanlı mesafe ölçümü. . 2023; 1129 - 1140. 10.17341/gazimmfd.979121 |
IEEE | YANIK H,turan b "Görüntü-Metre ile görüntü işleme tabanlı mesafe ölçümü." , ss.1129 - 1140, 2023. 10.17341/gazimmfd.979121 |
ISNAD | YANIK, Haydar - turan, bülent. "Görüntü-Metre ile görüntü işleme tabanlı mesafe ölçümü". (2023), 1129-1140. https://doi.org/10.17341/gazimmfd.979121 |
APA | YANIK H, turan b (2023). Görüntü-Metre ile görüntü işleme tabanlı mesafe ölçümü. Gazi Üniversitesi Mühendislik Mimarlık Fakültesi Dergisi, 38(2), 1129 - 1140. 10.17341/gazimmfd.979121 |
Chicago | YANIK Haydar,turan bülent Görüntü-Metre ile görüntü işleme tabanlı mesafe ölçümü. Gazi Üniversitesi Mühendislik Mimarlık Fakültesi Dergisi 38, no.2 (2023): 1129 - 1140. 10.17341/gazimmfd.979121 |
MLA | YANIK Haydar,turan bülent Görüntü-Metre ile görüntü işleme tabanlı mesafe ölçümü. Gazi Üniversitesi Mühendislik Mimarlık Fakültesi Dergisi, vol.38, no.2, 2023, ss.1129 - 1140. 10.17341/gazimmfd.979121 |
AMA | YANIK H,turan b Görüntü-Metre ile görüntü işleme tabanlı mesafe ölçümü. Gazi Üniversitesi Mühendislik Mimarlık Fakültesi Dergisi. 2023; 38(2): 1129 - 1140. 10.17341/gazimmfd.979121 |
Vancouver | YANIK H,turan b Görüntü-Metre ile görüntü işleme tabanlı mesafe ölçümü. Gazi Üniversitesi Mühendislik Mimarlık Fakültesi Dergisi. 2023; 38(2): 1129 - 1140. 10.17341/gazimmfd.979121 |
IEEE | YANIK H,turan b "Görüntü-Metre ile görüntü işleme tabanlı mesafe ölçümü." Gazi Üniversitesi Mühendislik Mimarlık Fakültesi Dergisi, 38, ss.1129 - 1140, 2023. 10.17341/gazimmfd.979121 |
ISNAD | YANIK, Haydar - turan, bülent. "Görüntü-Metre ile görüntü işleme tabanlı mesafe ölçümü". Gazi Üniversitesi Mühendislik Mimarlık Fakültesi Dergisi 38/2 (2023), 1129-1140. https://doi.org/10.17341/gazimmfd.979121 |