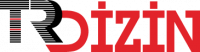
A type-2 fuzzy rule-based model for diagnosis of COVID-19
Yıl: 2023 Cilt: 31 Sayı: 1 Sayfa Aralığı: 39 - 52 Metin Dili: İngilizce DOI: 10.55730/1300-0632.3970 İndeks Tarihi: 16-05-2023
A type-2 fuzzy rule-based model for diagnosis of COVID-19
Öz: In this study, a type-2 fuzzy logic-based decision support system comprising clinical examination and blood test results that health professionals can use in addition to existing methods in the diagnosis of COVID-19 has been developed. The developed system consists of three fuzzy units. The first fuzzy unit produces COVID-19 positivity as a percentage according to the respiratory rate, loss of smell, and body temperature values, and the second fuzzy unit according to the C-reactive protein, lymphocyte, and D-dimer values obtained as a result of the blood tests. In the third fuzzy unit, the COVID-19 positivity risks according to the clinical examination and blood analysis results, which are the outputs of the first and second fuzzy units, are evaluated together and the result is obtained. As a result of the evaluation of the trials with 60 different scenarios by physicians, it has been revealed that the system can detect COVID-19 risk with 86.6% accuracy.
Anahtar Kelime: Belge Türü: Makale Makale Türü: Araştırma Makalesi Erişim Türü: Erişime Açık
- [1] World Health Organization, Laboratory testing for 2019 novel coronavirus (2019-nCoV) in suspected human cases, Interim guidance, 2020.
- [2] Yang Y, Yang M, Shen C, Wang F, Yuan J et al. Diagnosis and Monitoring the Viral Shedding of SARS-CoV-2 Infection. Innovation 2020; 1 (3):100061. https://doi.org/10.1016/j.xinn.2020.100061
- [3] Ai T, Yang Z, Hou H, Zhan C, Chen C et al. Correlation of Chest CT and RT-PCR Testing for Coro- navirus Disease 2019 (COVID-19) in China: A Report of 1014 Cases. Radiology 2020; 296 (2):32-40. https://doi.org/10.1148/radiol.2020200642
- [4] Wang L, Wong A. COVID-net: a tailored deep convolutional neural network design for detection of COVID-19 cases from chest radiography images. Scientific Reports 2020; 10:19549. https://doi.org/10.1038/s41598-020-76550-z
- [5] Khan AI, Shah, Bhat MM. A deep neural network for detection and diagnosis of covid-19 from chest X-ray images. Computer Methods and Programs in Biomedicine 2020; 196:105581. https://doi.org/10.1016/j.cmpb.2020.105581
- [6] Afshar P, Heidarian S, Naderkhani F, Oikonomou A, Plataniotis KN et al. COVID-CAPS: A capsule network-based framework for identification of COVID-19 cases from X-ray images. Pattern Recognition Letters 2020; 138:638-643. https://doi.org/10.1016/j.patrec.2020.09.010
- [7] Dorr F, Chaves H, Serra MM, Ramirez A, Costa ME et al. COVID-19 pneumonia accurately de- tected on chest radiographs with artificial intelligence. Intelligence Based Medicine 2020; 3:100014. https://doi.org/10.1016/j.ibmed.2020.100014
- [8] Shibly KH, Dey SK, Islam MTU, Rahman MM. COVID faster R-CNN: a novel framework to diagnosis novel coronavirus disease (COVID-19) in X-ray images. Informatics in Medical Unlocked 2020; 20:100405. https://doi.org/10.1016/j.imu.2020.100405
- [9] Singh KK, Singh A. Diagnosis of COVID-19 from chest X-ray images using wavelets-based depthwise convolution network. Big Data Mining and Analytics 2021; 4 (2):84-93. https://doi.org/10.26599/BDMA.2020.9020012
- [10] Laghi A. Cautions about radiologic diagnosis of COVID-19 infection driven by artificial intelligence. The Lancet Digital Health 2020; 2 (5):e225. https://doi.org/10.1016/S2589-7500(20)30079-0
- [11] Daioku T, Kobayashi M, Oishi F. Development of Visual Educational Materials for Radia- tion Protection in Computed Tomography. Journal of Radiology Nursing 2021; 40 (3):268-274. https://doi.org/10.1016/j.jradnu.2021.04.006
- [12] Bao J, Li C, Zhang K, Kang H, Chen W. Comparative analysis of laboratory indexes of severe and non-severe patients infected with COVID-19. Clinica Chimica Acta 2020; 509:180-194. https://doi.org/10.1016/j.cca.2020.06.009
- [13] Fan BE, Chong VCL, Chan SSW, Lim GH, Lim KGE et al. Hematologic parameters in patients with covid-19 infection. American Journal of Hematology 2020; 95 (6):131-134. https://doi.org/10.1002/ajh.25774
- [14] Ferrari D, Motta A, Strollo M, Banfi G, Locatelli M. Routine blood tests as a potential diagnostic tool for COVID-19. Clinical Chemistry and Laboratory Medicine 2020; 58 (7):1095-1099. https://doi.org/10.1515/cclm-2020-0398
- [15] Khartabil TA, Russcher H, Van der Ven A, de Rijke YB. A summary of the diagnostic and prognostic value of hemocytometry markers in COVID-19 patients. Critical Reviews in Clinical Laboratory Sciences 2020; 57 (6):415- 431. https://doi.org/10.1080/10408363.2020.1774736
- [16] Kermali M, Khalsa RK, Pillai K, Ismail Z, Harky A. The role of biomarkers in diagnosis of COVID-19 - A systematic review. Life Sciences 2020; 254:117788. https://doi.org/10.1016/j.lfs.2020.117788
- [17] Shaban WM, Rabie AH, Saleh AI, Abo-Elsoud MA. Detecting COVID-19 patients based on fuzzy inference engine and Deep Neural Network. Applied Soft Computing 2021; 99:106906. https://doi.org/10.1016/j.asoc.2020.106906
- [18] Batista AFM, Miraglia JL, Donato THR, Filho ADPC. COVID-19 diagnosis prediction in emergency care patients: a machine learning approach. medRxiv 2020. https://doi.org/10.1101/2020.04.04.20052092
- [19] Brinati D, Campagner A, Ferrari D, Locatelli M, Banfi G et al. Detection of COVID-19 Infection from Rou- tine Blood Exams with Machine Learning: A Feasibility Study. Journal of Medical Systems 2020; 44 (8):135. https://doi.org/10.1007/s10916-020-01597-4
- [20] AlJame M, Ahmad I, Imtiaz A, Mohammed A. Ensemble learning model for diagnosing COVID-19 from routine blood tests. Informatics in Medicine Unlocked 2020; 21:100449. https://doi.org/10.1016/j.imu.2020.100449
- [21] Jiang X, Coffee M, Bari A, Wang J, Jiang X et al. Towards an artificial intelligence framework for data- driven prediction of coronavirus clinical severity. Computers, Materials and Continua 2020; 63 (1): 537-551. https://doi.org/10.32604/cmc.2020.010691
- [22] Abdulkareem KH, Mohammed MA, Salim A, Arif M, Geman O et al. Realizing an Effective COVID-19 Diagnosis System Based on Machine Learning and IoT in Smart Hospital Environment. IEEE Internet Things Journal 2021; 8 (21):15919-15928. https://doi.org/10.1109/JIOT.2021.3050775
- [23] Alakus TA, Turkoglu I, Comparison of deep learning approaches to predict COVID-19 infection, Chaos, Solitons and Fractals 2020; 140:110120. https://doi.org/10.1016/j.chaos.2020.110120
- [24] Ahamad M, Aktar S, Mahfuz R, Uddin S, Liò P et al. A machine learning model to identify early stage symptoms of SARS-Cov-2 infected patients. Expert Systems with Applications 2020; 160:11366. https://doi.org/10.1016/j.eswa.2020.113661.
- [25] Arpaci S, Huang M, Al-Emran M, Al-Kabi MN, Peng M. Predicting the covid-19 infection with fourteen clinical features using machine learning classification algorithms. Multimedia Tools and Applications 2020; 80:11943–11957. https://doi.org/10.1007/s11042-020-10340-7
- [26] Wu G, Zhou S, Wang Y. A prediction model of outcome of SARS-CoV-2 pneumonia based on laboratory findings. Scientific Reports 2020; 10:14042. https://doi.org/10.1038/s41598-020-71114-7
- [27] Shatnawi M, Shatnawi A, AlShara Z, Husari G. Symptoms-Based Fuzzy-Logic Approach for COVID- 19 Diagnosis. International Journal of Advanced Computer Science and Applications 2021; 12 (4). http://dx.doi.org/10.14569/IJACSA.2021.0120457
- [28] Liang Q, Mendel J. Interval type-2 fuzzy logic systems: Theory and design. IEEE Transactions on Fuzzy Systems 2000; 8 (5):535-550. https://doi.org/10.1109/91.873577
- [29] Karnik NN, Mendel JM. Introduction to type-2 fuzzy logic systems. In: IEEE International Conference on Fuzzy Systems; Alaska, USA; 1998. pp. 915-920.
- [30] Liang Q, Mendel JM. Interval type-2 fuzzy logic systems. In: 9. IEEE International Conference on Fuzzy Systems; Texas, USA; 2000. pp. 328-333.
- [31] Maftouni M, Turksen IB, Zarandi MHF, Roshani F. Type-2 fuzzy rule-based expert system for Ankylosing spondylitis diagnosis. In: North American Fuzzy Information Processing Society; Washington, USA; 2015. pp. 1-5.
- [32] Ontiveros E, Melin P, Castillo. Comparative study of interval Type-2 and general Type-2 fuzzy systems in medical diagnosis. Information Sciences 2020; 525:37-53. https://doi.org/10.1016/j.ins.2020.03.059
- [33] Zarandi MHF, Zarinbal M, Izadi M. Systematic image processing for diagnosing brain tumors: A Type-II fuzzy expert system approach. Applied Soft Computing 2011; 11 (1):285–294. https://doi.org/10.1016/j.asoc.2009.11.019
- [34] Zarandi MHF, Soltanzadeh S, Mohammadi A, Castillo O. Designing a general type-2 fuzzy expert system for diagnosis of depression. Applied Soft Computing 2019; 80:329-341. https://doi.org/10.1016/j.asoc.2019.03.027
- [35] Liu X, Wang Z, Zhang S, Garg H. Novel correlation coefficient between hesitant fuzzy sets with application to medical diagnosis. Expert Systems with Applications 2021; 183:115393. https://doi.org/10.1016/j.eswa.2021.115393
- [36] Rocha EM, Junior WB, Lucas KE, Júnior CTC, Júnior JGC et al. A fuzzy type-2 fault detection methodology to minimize false alarm rate in induction motor monitoring applications. Applied Soft Computing 2020; 93:106373. https://doi.org/10.1016/j.asoc.2020.106373
- [37] Montazeri-Gh M, Yazdani S. Application of interval type-2 fuzzy logic systems to gas turbine fault diagnosis. Applied Soft Computing 2020; 96:106703. https://doi.org/10.1016/j.asoc.2020.106703
- [38] Camci E, Kayacan E. Game of drones: UAV pursuit-evasion game with type-2 fuzzy logic controllers tuned by reinforcement learning. In: IEEE International Conference on Fuzzy Systems; Vancouver, Canada; 2016. pp. 618- 625.
- [39] Taskin A, Kumbasar T. An Open Source Matlab/Simulink Toolbox for Interval Type-2 Fuzzy Logic Systems. In: IEEE Symposium Series on Computational Intelligence; Cape Town, South Africa; 2015. pp.1561-1568.
- [40] Mastrangelo A, Bonato M, Cinque P. Smell and taste disorders in COVID-19: From pathogenesis to clinical features and outcomes. Neuroscience Letters 2021; 748:135694. https://doi.org/10.1016/j.neulet.2021.135694
- [41] Zhou Z, Yang Z, Ou J, Zhang H, Zhang Q et al. Temperature dependence of the SARS-CoV-2 affinity to human ACE2 determines COVID-19 progression and clinical outcome. Computational and Structural Biotechnology Journal 2021; 19:161-167. https://doi.org/10.1016/j.csbj.2020.12.005
- [42] Ozen M, Yilmaz A, Cakmak V, Beyoglu R, Oskay A et al. D-Dimer as a potential biomarker for disease severity in COVID-19. The American Journal of Emergency Medicine 2021; 40:55-59. https://doi.org/10.1016/j.ajem.2020.12.023
- [43] Jing LJ, Liu J, Chen Y, Ye B, Li N etc. Characteristics of laboratory findings of COVID-19 pa- tients with comorbid diabetes mellitus. Diabetes Research and Clinical Practice 2020; 167:108351. https://doi.org/10.1016/j.diabres.2020.108351.
- [44] Wang G, Wu C, Zhang Q, Wu F, Yu B etc. C-Reactive Protein Level May Predict the Risk of COVID-19 Aggravation. Open Forum Infectious Diseases 2020; 7 (5): 153. https://doi.org/10.1093/ofid/ofaa153
- [45] Cillóniz C, Torres A, Garcia-Vidal C, Moreno-Garcia E, Amaro R etc. The Value of C-Reactive Protein-to- Lymphocyte Ratio in Predicting the Severity of SARS-CoV-2 Pneumonia. Archivos de Bronconeumología 2021; 57:79-82. https://doi.org/10.1016/j.arbres.2020.07.038
- [46] Keskin S, Keskin DD. One Year Analysis of Hematological and Inflammatory Parameters to Predict the Severity of COVID-19 Infection in Pregnant Women. Middle Black Sea Journal of Health Science 2022; 8 (1):146-157. https://doi.org/10.19127/mbsjohs.1064479
APA | ŞAHİN İ, AKDOGAN E, Aktan M (2023). A type-2 fuzzy rule-based model for diagnosis of COVID-19. , 39 - 52. 10.55730/1300-0632.3970 |
Chicago | ŞAHİN İHSAN,AKDOGAN ERHAN,Aktan Mehmet Emin A type-2 fuzzy rule-based model for diagnosis of COVID-19. (2023): 39 - 52. 10.55730/1300-0632.3970 |
MLA | ŞAHİN İHSAN,AKDOGAN ERHAN,Aktan Mehmet Emin A type-2 fuzzy rule-based model for diagnosis of COVID-19. , 2023, ss.39 - 52. 10.55730/1300-0632.3970 |
AMA | ŞAHİN İ,AKDOGAN E,Aktan M A type-2 fuzzy rule-based model for diagnosis of COVID-19. . 2023; 39 - 52. 10.55730/1300-0632.3970 |
Vancouver | ŞAHİN İ,AKDOGAN E,Aktan M A type-2 fuzzy rule-based model for diagnosis of COVID-19. . 2023; 39 - 52. 10.55730/1300-0632.3970 |
IEEE | ŞAHİN İ,AKDOGAN E,Aktan M "A type-2 fuzzy rule-based model for diagnosis of COVID-19." , ss.39 - 52, 2023. 10.55730/1300-0632.3970 |
ISNAD | ŞAHİN, İHSAN vd. "A type-2 fuzzy rule-based model for diagnosis of COVID-19". (2023), 39-52. https://doi.org/10.55730/1300-0632.3970 |
APA | ŞAHİN İ, AKDOGAN E, Aktan M (2023). A type-2 fuzzy rule-based model for diagnosis of COVID-19. Turkish Journal of Electrical Engineering and Computer Sciences, 31(1), 39 - 52. 10.55730/1300-0632.3970 |
Chicago | ŞAHİN İHSAN,AKDOGAN ERHAN,Aktan Mehmet Emin A type-2 fuzzy rule-based model for diagnosis of COVID-19. Turkish Journal of Electrical Engineering and Computer Sciences 31, no.1 (2023): 39 - 52. 10.55730/1300-0632.3970 |
MLA | ŞAHİN İHSAN,AKDOGAN ERHAN,Aktan Mehmet Emin A type-2 fuzzy rule-based model for diagnosis of COVID-19. Turkish Journal of Electrical Engineering and Computer Sciences, vol.31, no.1, 2023, ss.39 - 52. 10.55730/1300-0632.3970 |
AMA | ŞAHİN İ,AKDOGAN E,Aktan M A type-2 fuzzy rule-based model for diagnosis of COVID-19. Turkish Journal of Electrical Engineering and Computer Sciences. 2023; 31(1): 39 - 52. 10.55730/1300-0632.3970 |
Vancouver | ŞAHİN İ,AKDOGAN E,Aktan M A type-2 fuzzy rule-based model for diagnosis of COVID-19. Turkish Journal of Electrical Engineering and Computer Sciences. 2023; 31(1): 39 - 52. 10.55730/1300-0632.3970 |
IEEE | ŞAHİN İ,AKDOGAN E,Aktan M "A type-2 fuzzy rule-based model for diagnosis of COVID-19." Turkish Journal of Electrical Engineering and Computer Sciences, 31, ss.39 - 52, 2023. 10.55730/1300-0632.3970 |
ISNAD | ŞAHİN, İHSAN vd. "A type-2 fuzzy rule-based model for diagnosis of COVID-19". Turkish Journal of Electrical Engineering and Computer Sciences 31/1 (2023), 39-52. https://doi.org/10.55730/1300-0632.3970 |