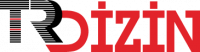
Nadir Hastalıklarda Yapay Zeka Uygulamaları
Yıl: 2022 Cilt: 75 Sayı: 1 Sayfa Aralığı: 63 - 69 Metin Dili: Türkçe DOI: 10.4274/atfm.galenos.2022.35002 İndeks Tarihi: 04-05-2023
Nadir Hastalıklarda Yapay Zeka Uygulamaları
Öz: Günümüzde yaygın olarak görülen hastalıkların tanı tedavi süreçlerinin daha etkili kontrol edilmesiyle nadir hastalıklar öne çıkmaya başlamıştır. Çoğu genetik temelli olan bu hastalık grubunun tanı ve tedavisinde gecikmenin çok daha fazla olduğu ve bazen yıllar sürdüğü, ayrıca ilaç keşfinin daha zorlayıcı olduğu bilinmektedir. Günümüzde genel tıbbın yanı sıra nadir hastalıklar alanında da hızla gelişen yapay zeka teknolojilerinin uygulanmasının bu süreçleri hızlandırabileceği ve hastalıkların yönetiminde faydalı olacağı düşünülmektedir. Buna yönelik çalışmalar son yıllarda sayı ve kalite olarak büyük oranda artmıştır. Bu yazıda hem nadir hastalıklar genelinde hem de birkaç tanesi özelinde mevcut literatür ışığında yapay zekanın kullanımıyla ilgili bilgiler gözden geçirilecektir.
Anahtar Kelime: Artificial Intelligence Applications in Rare Diseases
Öz: Rare diseases have started to come to the fore with the more effective control of the diagnosis and treatment processes of common diseases today. It is known that the delay in diagnosis and treatment of rare diseases, most of which are genetically based, sometimes takes years, and drug discovery is also more challenging. It is thought that the application of rapidly developing artificial intelligence technologies in the field of rare diseases, as well as general medicine, can accelerate these processes and could be beneficial in the management of these diseases. Studies on this subject have increased in number and quality in recent years. In this article, information about the usage of artificial intelligence will be reviewed in the light of the existing literature, both in rare diseases and in a few specific ones.
Anahtar Kelime: Belge Türü: Makale Makale Türü: Derleme Erişim Türü: Erişime Açık
- 1. Wright CF, FitzPatrick DR, Firth HV. Paediatric genomics: diagnosing rare disease in children. Nat Rev Genet. 2018;19:253-268.
- 2. Orphanet INSERM. Orphadata.[2022 Sept 28]. http://www.orphadata.org/ cgi-bin/index.php.
- 3. Nguengang Wakap S, Lambert DM, Olry A, et al. Estimating cumulative point prevalence of rare diseases: analysis of the Orphanet database. Eur J Hum Genet. 2020;28:165-173.
- 4. Black N, Martineau F, Manacorda T. Diagnostic odyssey for rare diseases: exploration of potential indicators . PIRU: Policy Innovation Research Unit. London; 2015. https://piru.lshtm.ac.uk/assets/files/Rare diseases Final report.pdf.
- 5. Kimmel L, Conti RM, Volerman A, et al. Pediatric orphan drug indications: 2010–2018. Pediatrics. 2020;145:2010–8.
- 6. Tuli S, Tuli S, Wander G, et al. Next generation technologies for smart healthcare: challenges, vision, model, trends and future directions. Internet Technology Letters. 2020;3:e145.
- 7. Holzinger A, Langs G, Denk H, et al. Causability and explainability of artificial intelligence in medicine. Wiley Interdisciplinary Reviews: Data Mining and Knowledge Discovery. 2019;9:1–13.
- 8. Zhang Z, Singh J, Gadiraju U, et al. Dissonance between human and machine understanding. Proceedings of the ACM on Human-Computer Interaction. 2019;3(CSCW).
- 9. Baltrusaitis T, Zadeh A, Lim YC, et al. OpenFace 2.0: Facial behavior analysis toolkit. Proceedings - 13th IEEE International Conference on Automatic Face and Gesture Recognition, FG 2018. 2018;59–66.
- 10. Hoeschl HC, Barcellos V. Artificial intelligence and law. IFIP Advances in Information and Communication Technology. 2004;154:25-34.
- 11. Eli-Chukwu NC. Applications of Artificial Intelligence in Agriculture: A Review. Engineering, Technology & Applied Science Research. 2019;9:4377- 4383.
- 12. Bistron M, Piotrowski Z. Artificial intelligence applications in military systems and their influence on sense of security of citizens. Electronics (Switzerland). 2021;10:871.
- 13. PassMark® Software. PassMark CPU Benchmarks - Year on Year Performance. [2022 Apr 13]. https://www.cpubenchmark.net/year-on-year.html.
- 14. Meskó B, Görög M. A short guide for medical professionals in the era of artificial intelligence. NPJ Digit Med. 2020;3:126.
- 15. James KN, Phadke S, Wong TC, et al. Artificial Intelligence in the Genetic Diagnosis of Rare Disease. Advances in Molecular Pathology. 2020;3:143- 155.
- 16. Li Q, Zhao K, Bustamante CD, et al. Xrare: a machine learning method jointly modeling phenotypes and genetic evidence for rare disease diagnosis. Genet Med. 2019;21:2126-2134.
- 17. Hasani N, Farhadi F, Morris MA, et al. Artificial Intelligence in Medical Imaging and its Impact on the Rare Disease Community: Threats, Challenges and Opportunities. PET Clin. 2022;17:13-29.
- 18. Kebir S, Rauschenbach L, Weber M, et al. Machine learning-based differentiation between multiple sclerosis and glioma WHO II°-IV° using O-(2-[18F] fluoroethyl)-L-tyrosine positron emission tomography. J Neurooncol. 2021;152:325-332.
- 19. Liehr T, Acquarola N, Pyle K, et al. Next generation phenotyping in Emanuel and Pallister-Killian syndrome using computer-aided facial dysmorphology analysis of 2D photos. Clin Genet. 2018;93:378-381.
- 20. Cerrolaza JJ, Porras AR, Mansoor A, et al. Identification of dysmorphic syndromes using landmark-specific local texture descriptors. In: 2016 IEEE 13th International Symposium on Biomedical Imaging (ISBI). IEEE; 2016. p. 1080-3.
- 21. Ferry Q, Steinberg J, Webber C, et al. Diagnostically relevant facial gestalt information from ordinary photos. Elife. 2014;3:e02020.
- 22. Kuru K, Niranjan M, Tunca Y, et al. Biomedical visual data analysis to build an intelligent diagnostic decision support system in medical genetics. Artif Intell Med. 2014;62:105-18.
- 23. Dudding-Byth T, Baxter A, Holliday EG, et al. Computer face-matching technology using two-dimensional photographs accurately matches the facial gestalt of unrelated individuals with the same syndromic form of intellectual disability. BMC Biotechnol. 2017;17:90.
- 24. Gurovich Y, Hanani Y, Bar O, et al. Identifying facial phenotypes of genetic disorders using deep learning. Nat Med. 2019;25:60-64.
- 25. Piñol M, Alves R, Teixidó I, et al. Rare Disease Discovery: An Optimized Disease Ranking System. IEEE Transactions on Industrial Informatics. 2017;13:1184-1192.
- 26. Bond WF, Schwartz LM, Weaver KR, et al. Differential diagnosis generators: an evaluation of currently available computer programs. J Gen Intern Med. 2012;27:213-219.
- 27. Long E, Lin H, Liu Z, et al. An artificial intelligence platform for the multihospital collaborative management of congenital cataracts. Nature Biomedical Engineering. 2017;1:1-8.
- 28. Spaccarotella C, Polimeni A, Mancuso C, et al. Assessment of Non-Invasive Measurements of Oxygen Saturation and Heart Rate with an Apple Smartwatch: Comparison with a Standard Pulse Oximeter. J Clin Med. 2022;11:1467.
- 29. Perez MV, Mahaffey KW, Hedlin H, et al. Large-Scale Assessment of a Smartwatch to Identify Atrial Fibrillation. N Engl J Med. 2019;381:1909- 1917.
- 30. Paech C, Kobel M, Michaelis A, et al. Accuracy of the Apple Watch singlelead ECG recordings in pre-term neonates. Cardiol Young. 2022;32:1633- 1637.
- 31. Kobel M, Kalden P, Michaelis A, et al. Accuracy of the Apple Watch iECG in Children With and Without Congenital Heart Disease. Pediatr Cardiol. 2022;43:191-196.
- 32. Spaccarotella CAM, Migliarino S, Mongiardo A, et al. Measurement of the QT interval using the Apple Watch. Sci Rep. 2021;11:10817.
- 33. Newman-Griffis D, Lehman JF, Rosé C, et al. Translational NLP: A New Paradigm and General Principles for Natural Language Processing Research. Proc Conf. 2021;2021:4125-4138.
- 34. Mumcuoğlu E, Öztürk CE, Ozaktas HM, et al. Natural language processing in law: Prediction of outcomes in the higher courts of Turkey. Information Processing and Management. 2021;58:1-16.
- 35. Cronin A, Intepe G, Shearman D, et al. Analysis using natural language processing of feedback data from two mathematics support centres. International Journal of Mathematical Education in Science and Technology. 2019;50:1-17.
- 36. Liang H, Tsui BY, Ni H, et al. Evaluation and accurate diagnoses of pediatric diseases using artificial intelligence. Nat Med. 2019 Mar;25(3):433-438.
- 37. Dong H, Suarez-Paniagua V, Zhang H, et al. Rare Disease Identification from Clinical Notes with Ontologies and Weak Supervision. Annu Int Conf IEEE Eng Med Biol Soc. 2021;2021:2294-2298.
- 38. Martínez-deMiguel C, Segura-Bedmar I, Chacón-Solano E, et al. The RareDis corpus: A corpus annotated with rare diseases, their signs and symptoms. J Biomed Inform. 2022;125:103961.
- 39. Bremer A, Lossius MI, Nakken KO. Dravet syndrome--considerable delay in making the diagnosis. Acta Neurol Scand. 2012;125:359-362.
- 40. Lo Barco T, Kuchenbuch M, Garcelon N, et al. Improving early diagnosis of rare diseases using Natural Language Processing in unstructured medical records: an illustration from Dravet syndrome. Orphanet J Rare Dis. 2021;16:309.
- 41. T.C. Sağlık Bakanlığı. Yenidoğan Metabolik ve Endokrin Hastalık Tarama Programı (NTP). TC. Sağlık Bakanlığı. 2021 [2022 May 7]. https://hsgm. saglik.gov.tr/depo/birimler/cocuk_ergen_db/dokumanlar/KHT_Akis_Semasi. pdf.
- 42. Fanen P, Wohlhuter-Haddad A, Hinzpeter A. Genetics of cystic fibrosis: CFTR mutation classifications toward genotype-based CF therapies. Int J Biochem Cell Biol. 2014;52:94-102.
- 43. Szczesniak RD, Li D, Su W, et al. Phenotypes of Rapid Cystic Fibrosis Lung Disease Progression during Adolescence and Young Adulthood. Am J Respir Crit Care Med. 2017;196:471-478.
- 44. Alaa AM, van der Schaar M. Prognostication and Risk Factors for Cystic Fibrosis via Automated Machine Learning. Sci Rep. 2018;8:11242.
- 45. Cleveland RH, Sawicki GS, Stamoulis C. Similar performance of Brasfield and Wisconsin scoring systems in young children with cystic fibrosis. Pediatr Radiol. 2015;45:1624-1628.
- 46. Zucker EJ, Barnes ZA, Lungren MP, et al. Deep learning to automate Brasfield chest radiographic scoring for cystic fibrosis. J Cyst Fibros. 2020;19:131- 138.
- 47. Lee YS, Krishnan A, Oughtred R, et al. A Computational Framework for Genome-wide Characterization of the Human Disease Landscape. Cell Syst. 2019;8:152-162.e6.
- 48. Ekins S, Puhl AC, Zorn KM, et al. Exploiting machine learning for end-toend drug discovery and development. Nat Mater. 2019;18:435-441.
- 49. Bluemke DA, Moy L, Bredella MA, et al. Assessing Radiology Research on Artificial Intelligence: A Brief Guide for Authors, Reviewers, and Readers- From the Radiology Editorial Board. Radiology. 2020;294:487-489.
- 50. Wang Q, Shen D. A cybernetic eye for rare disease. Nat Biomed Eng. 2017;1:0032.
- 51. T.C. Sağlık Bakanlığı Halk Sağlığı Genel Müdürlüğü Çocuk ve Ergen Sağlığı Dairesi Başkanlığı. Evlilik Öncesi Spinal Musküler Atrofi (SMA) Taşıyıcı Tarama Programı. [2022 Apr 1]. https://hsgm.saglik.gov.tr/tr/cocukergentp- liste.html?view=article&id=6711&catid=880.
APA | Gülşen M, Turan B, Yilmaz A (2022). Nadir Hastalıklarda Yapay Zeka Uygulamaları. , 63 - 69. 10.4274/atfm.galenos.2022.35002 |
Chicago | Gülşen Murat,Turan Bahadır,Yilmaz Asim Egemen Nadir Hastalıklarda Yapay Zeka Uygulamaları. (2022): 63 - 69. 10.4274/atfm.galenos.2022.35002 |
MLA | Gülşen Murat,Turan Bahadır,Yilmaz Asim Egemen Nadir Hastalıklarda Yapay Zeka Uygulamaları. , 2022, ss.63 - 69. 10.4274/atfm.galenos.2022.35002 |
AMA | Gülşen M,Turan B,Yilmaz A Nadir Hastalıklarda Yapay Zeka Uygulamaları. . 2022; 63 - 69. 10.4274/atfm.galenos.2022.35002 |
Vancouver | Gülşen M,Turan B,Yilmaz A Nadir Hastalıklarda Yapay Zeka Uygulamaları. . 2022; 63 - 69. 10.4274/atfm.galenos.2022.35002 |
IEEE | Gülşen M,Turan B,Yilmaz A "Nadir Hastalıklarda Yapay Zeka Uygulamaları." , ss.63 - 69, 2022. 10.4274/atfm.galenos.2022.35002 |
ISNAD | Gülşen, Murat vd. "Nadir Hastalıklarda Yapay Zeka Uygulamaları". (2022), 63-69. https://doi.org/10.4274/atfm.galenos.2022.35002 |
APA | Gülşen M, Turan B, Yilmaz A (2022). Nadir Hastalıklarda Yapay Zeka Uygulamaları. Ankara Üniversitesi Tıp Fakültesi Mecmuası, 75(1), 63 - 69. 10.4274/atfm.galenos.2022.35002 |
Chicago | Gülşen Murat,Turan Bahadır,Yilmaz Asim Egemen Nadir Hastalıklarda Yapay Zeka Uygulamaları. Ankara Üniversitesi Tıp Fakültesi Mecmuası 75, no.1 (2022): 63 - 69. 10.4274/atfm.galenos.2022.35002 |
MLA | Gülşen Murat,Turan Bahadır,Yilmaz Asim Egemen Nadir Hastalıklarda Yapay Zeka Uygulamaları. Ankara Üniversitesi Tıp Fakültesi Mecmuası, vol.75, no.1, 2022, ss.63 - 69. 10.4274/atfm.galenos.2022.35002 |
AMA | Gülşen M,Turan B,Yilmaz A Nadir Hastalıklarda Yapay Zeka Uygulamaları. Ankara Üniversitesi Tıp Fakültesi Mecmuası. 2022; 75(1): 63 - 69. 10.4274/atfm.galenos.2022.35002 |
Vancouver | Gülşen M,Turan B,Yilmaz A Nadir Hastalıklarda Yapay Zeka Uygulamaları. Ankara Üniversitesi Tıp Fakültesi Mecmuası. 2022; 75(1): 63 - 69. 10.4274/atfm.galenos.2022.35002 |
IEEE | Gülşen M,Turan B,Yilmaz A "Nadir Hastalıklarda Yapay Zeka Uygulamaları." Ankara Üniversitesi Tıp Fakültesi Mecmuası, 75, ss.63 - 69, 2022. 10.4274/atfm.galenos.2022.35002 |
ISNAD | Gülşen, Murat vd. "Nadir Hastalıklarda Yapay Zeka Uygulamaları". Ankara Üniversitesi Tıp Fakültesi Mecmuası 75/1 (2022), 63-69. https://doi.org/10.4274/atfm.galenos.2022.35002 |