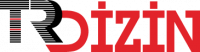
Clinical Assessment of CoLumbo Deep Learning System for Central Canal Stenosis Diagnostics
Yıl: 2023 Cilt: 7 Sayı: 1 Sayfa Aralığı: 47 - 48 Metin Dili: İngilizce DOI: 10.14744/ejmo.2023.59207 İndeks Tarihi: 05-05-2023
Clinical Assessment of CoLumbo Deep Learning System for Central Canal Stenosis Diagnostics
Öz: Objectives: There is a great variability of inter-observer disagreements for central stenosis diagnostics depending on the used classification. This study investigates the level of agreement between lumbar magnetic resonance imaging (MRI) reports created by a deep learning neural network (CoLumbo) and the radiologists’ reading. Methods: A total of 382 (53.4 % females, 46.6 % males and average age 49.52±13.20) prospective consecutive patients in 3 different healthcare centers referred to L-spine MRI for back or leg pain were analyzed by the software CoLumbo for the presence of stenosis on all lumbar levels, by radiologists using it and radiologists not using the dedicated software. In case of disagreement between radiologists, a radiologist-arbiter opinion was used to establish majority opinion. The total number of evaluated levels was 1762. Results: There were 156 debatable cases of disagreements between radiologists using the software, and radiologists, not using CoLumbo, for the presence of central stenosis. In 18 cases, the arbiter opinion has coincided with that of the radiologist not using the software. In 138 cases, the former has coincided with that of the radiologist using the software CoLumbo. Most of the cases of disagreement are borderline cases. The reported sensitivity and specificity of CoLumbo was 92.70% and 99.04%, respectively. Conclusion: The study showed that the radiologist using the CoLumbo software achieved best results. The results of the algorithm were inferior but still better than radiologists not using the software in any published study.
Anahtar Kelime: Belge Türü: Makale Makale Türü: Araştırma Makalesi Erişim Türü: Erişime Açık
- 1. Katz JN, Harris MB. Lumbar spinal stenosis. New Engl J Med 2008;358:818–25.
- 2. Karantanas AH, Zibis AH, Papaliaga M, Georgiou E, Rousogiannis S. Dimensions of the lumbar spinal canal: variations and correlations with somatometric parameters using CT. Eur Radiol 1998;8:1581–5.
- 3. Ravindra VM, Senglaub SS, Rattani A, Dewan MC, Hartl R, Bisson E, et al. Degenerative Lumbar Spine Disease: Estimating Global Incidence and Worldwide Volume. Global Spine J 2018;8:784–94.
- 4. Bae HW, Rajaee SS, Kanim LE. Nationwide Trends in the Surgical Management of Lumbar Spinal Stenosis. Spine 2013;38:916–26.
- 5. Sengupta DK. Dynamic stabilization devices in the treatment of low back pain. Orthop Clin North Am 2004;35:43–56.
- 6. Li AM, Li X, Yang Z. Decompression and coflex interlaminar stabilisation compared with conventional surgical procedures for lumbar spinal stenosis: A systematic review and metaanalysis. Int J Surg 2017;40:60–7.
- 7. Drew B, Bhandari M, Kulkarni AV, Louw D, Reddy K, Dunlop B. Reliability in grading the severity of lumbar spinal stenosis. J Spinal Disord 2000;13:253–8.
- 8. Lewandrowski KU. Retrospective analysis of accuracy and positive predictive value of preoperative lumbar MRI grading after successful outcome following outpatient endoscopic decompression for lumbar foraminal and lateral recess stenosis. Clin Neurol Neurosur 2019;179:74–80.
- 9. Al-Tameemi HN, Al-Essawi S, Shukri M, Naji FK. Using Magnetic Resonance Myelography to Improve Interobserver Agreement in the Evaluation of Lumbar Spinal Canal Stenosis and Root Compression. Asian Spine J 2017;11:198–203.
- 10. Amundsen T, Weber H, Lilleas F, Nordal HJ, Abdelnoor M, Magnaes B. lumbar spinal stenosis - clinical and radiologic features. Spine 1995;20:1178–86.
- 11. Zheng F, Farmer JC, Sandhu HS, O'Leary PF. A novel method for the quantitative evaluation of lumbar spinal stenosis. HSS J 2006;2:136–40.
- 12. Griffith JF, Huang J, Law S-W, Xiao F, Leung JCS, Wang D. Population reference range for developmental lumbar spinal canal size. Quant Imaging Med Surg 2016;6:671–9.
- 13. Amonoo-Kuofi HS. The sagittal diameter of the lumbar vertebral canal in normal adult Nigerians. J Anat 1985;140:69–78.
- 14. Twomey L, Taylor J. Age changes in the lumbar spinal and intervertebral canals. Paraplegia 1988;26:238–49.
- 15. Pesapane F, Codari M, Sardanelli F. Artificial intelligence in medical imaging: threat or opportunity? Radiologists again at the forefront of innovation in medicine. Eur Radiol Exp 2018;2:35.
- 16. Roller BL, Boutin RD, O'Gara TJ, Knio ZO, Jamaludin A, Tan J, et al. Accurate prediction of lumbar microdecompression level with an automated MRI grading system. Skeletal Radiol 2021;50:69–78.
- 17. D'Antoni F, Russo F, Ambrosio L, Bacco L, Vollero L, Vadalà G, et al. Artificial intelligence and computer aided diagnosis in chronic low back pain: A systematic review. Int J Environ Res Public Health 2022;19:5971.
- 18. Georgiev N, Asenov A. Automatic segmentation of lumbar spine MRI using ensemble of 2D algorithms. In: Zheng G, Belavy D, Cai Y, Li S, editors. Computational Methods and Clinical Applications for Spine Imaging. Cham: Springer International Publishing; 2019. p. 154–62.
- 19. Schonstrom N, Lindahl S, Willen J, Hansson T. Dynamic changes in the dimensions of the lumbar spinal canal: an experimental study in vitro. J Orthop Res 1989;7:115–21.
- 20. McHugh ML. Interrater reliability: The kappa statistic. Biochem Med (Zagreb) 2012;22:276–82.
- 21. Yuan S, Zou Y, Li Y, Chen M, Yue Y. A clinically relevant MRI grading system for lumbar central canal stenosis. Clin Imaging 2016;40:1140–5.
- 22. Bhargavan M, Kaye AH, Forman HP, Sunshine JH. Workload of Radiologists in United States in 2006–2007 and Trends Since 1991–1992. Radiology 2009;252:458–67.
- 23. van Rijn JC, Klemetso N, Reitsma JB, Majoie CB, Hulsmans FJ, Peul WC, et al. Observer variation in MRI evaluation of patients suspected of lumbar disk herniation. AJR Am J Roentgenol 2005;184:299–303.
- 24. Speciale AC, Pietrobon R, Urban CW, Richardson WJ, Helms CA, Major N, et al. Observer variability in assessing lumbar spinal stenosis severity on magnetic resonance imaging and its relation to cross-sectional spinal canal area. Spine (Phila Pa 1976) 2002;27:1082–6.
- 25. Lurie JD, Tosteson AN, Tosteson TD, Carragee E, Carrino JA, Kaiser J, et al. Reliability of readings of magnetic resonance imaging features of lumbar spinal stenosis. Spine (Phila Pa 1976) 2008;33:1605–10.
- 26. Schizas C, Theumann N, Burn A, Tansey R, Wardlaw D, Smith FW, et al. Qualitative grading of severity of lumbar spinal stenosis based on the morphology of the dural sac on magnetic resonance images. Spine 2010;35:1919–24.
APA | Georgiev R, Novakova M, Bliznakova K (2023). Clinical Assessment of CoLumbo Deep Learning System for Central Canal Stenosis Diagnostics. , 47 - 48. 10.14744/ejmo.2023.59207 |
Chicago | Georgiev Radoslav,Novakova Marianna,Bliznakova Kristina Clinical Assessment of CoLumbo Deep Learning System for Central Canal Stenosis Diagnostics. (2023): 47 - 48. 10.14744/ejmo.2023.59207 |
MLA | Georgiev Radoslav,Novakova Marianna,Bliznakova Kristina Clinical Assessment of CoLumbo Deep Learning System for Central Canal Stenosis Diagnostics. , 2023, ss.47 - 48. 10.14744/ejmo.2023.59207 |
AMA | Georgiev R,Novakova M,Bliznakova K Clinical Assessment of CoLumbo Deep Learning System for Central Canal Stenosis Diagnostics. . 2023; 47 - 48. 10.14744/ejmo.2023.59207 |
Vancouver | Georgiev R,Novakova M,Bliznakova K Clinical Assessment of CoLumbo Deep Learning System for Central Canal Stenosis Diagnostics. . 2023; 47 - 48. 10.14744/ejmo.2023.59207 |
IEEE | Georgiev R,Novakova M,Bliznakova K "Clinical Assessment of CoLumbo Deep Learning System for Central Canal Stenosis Diagnostics." , ss.47 - 48, 2023. 10.14744/ejmo.2023.59207 |
ISNAD | Georgiev, Radoslav vd. "Clinical Assessment of CoLumbo Deep Learning System for Central Canal Stenosis Diagnostics". (2023), 47-48. https://doi.org/10.14744/ejmo.2023.59207 |
APA | Georgiev R, Novakova M, Bliznakova K (2023). Clinical Assessment of CoLumbo Deep Learning System for Central Canal Stenosis Diagnostics. Eurasian Journal of Medicine and Oncology, 7(1), 47 - 48. 10.14744/ejmo.2023.59207 |
Chicago | Georgiev Radoslav,Novakova Marianna,Bliznakova Kristina Clinical Assessment of CoLumbo Deep Learning System for Central Canal Stenosis Diagnostics. Eurasian Journal of Medicine and Oncology 7, no.1 (2023): 47 - 48. 10.14744/ejmo.2023.59207 |
MLA | Georgiev Radoslav,Novakova Marianna,Bliznakova Kristina Clinical Assessment of CoLumbo Deep Learning System for Central Canal Stenosis Diagnostics. Eurasian Journal of Medicine and Oncology, vol.7, no.1, 2023, ss.47 - 48. 10.14744/ejmo.2023.59207 |
AMA | Georgiev R,Novakova M,Bliznakova K Clinical Assessment of CoLumbo Deep Learning System for Central Canal Stenosis Diagnostics. Eurasian Journal of Medicine and Oncology. 2023; 7(1): 47 - 48. 10.14744/ejmo.2023.59207 |
Vancouver | Georgiev R,Novakova M,Bliznakova K Clinical Assessment of CoLumbo Deep Learning System for Central Canal Stenosis Diagnostics. Eurasian Journal of Medicine and Oncology. 2023; 7(1): 47 - 48. 10.14744/ejmo.2023.59207 |
IEEE | Georgiev R,Novakova M,Bliznakova K "Clinical Assessment of CoLumbo Deep Learning System for Central Canal Stenosis Diagnostics." Eurasian Journal of Medicine and Oncology, 7, ss.47 - 48, 2023. 10.14744/ejmo.2023.59207 |
ISNAD | Georgiev, Radoslav vd. "Clinical Assessment of CoLumbo Deep Learning System for Central Canal Stenosis Diagnostics". Eurasian Journal of Medicine and Oncology 7/1 (2023), 47-48. https://doi.org/10.14744/ejmo.2023.59207 |