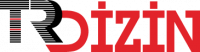
Automatic Detection and Classification of Some Vineyard Diseases with Faster R-CNN Model
Yıl: 2023 Cilt: 11 Sayı: 1 Sayfa Aralığı: 97 - 103 Metin Dili: İngilizce DOI: 10.24925/turjaf.v11i1.97-103.5665 İndeks Tarihi: 14-05-2023
Automatic Detection and Classification of Some Vineyard Diseases with Faster R-CNN Model
Öz: Türkiye is one of the countries with the most important vineyard areas in the world, where the most grape production is made. Vineyard diseases are one of the most important reasons that adversely affect the productivity in viticulture. In this study, some vineyard diseases were detected and classified using the Faster R-CNN deep learning model, which is an artificial intelligence approach. These diseases are powdery mildew, downy mildew, dead arm disease, grapevine leaf roll-associated virus disease (GLRaV) and grapevine fan leaf nepovirus (GFLV) diseases that are common and cause economic problems. The proposed method is trained and tested using 11000 images. At the end of the study, the overall accuracy rate was found to be 92%. The proposed approach gave better results than similar methods in the literature. Therefore, it was concluded that the method can be used reliably in the detection and classification of some vineyard diseases.
Anahtar Kelime: Bazı Bağ Hastalıklarının Faster R-CNN Modeli ile Otomatik Tespit Edilmesi ve Sınıflandırılması
Öz: Türkiye, üzüm üretiminin en çok yapıldığı dünyanın en önemli bağ alanlarına sahip olan ülkelerdendir. Bağcılıkta verimliliği olumsuz etkileyen en önemli sebeplerden birisi bağ hastalıklarıdır. Bu çalışmada, bir yapay zekâ yaklaşımı olan Faster R-CNN derin öğrenme modeli kullanılarak bazı bağ hastalıkları tespit edilmiş ve sınıflandırılmıştır. Bu hastalıklar yaygın olarak görülen ve ekonomik sorun oluşturan külleme, mildiyö, ölü kol hastalığı ile asma yaprak kıvrılma virüs hastalığı (GLRaV) ve asma kısa boğum virüs (GFLV) hastalıklarıdır. Önerilen yöntem 11000 görüntü kullanılarak eğitilmiş ve test edilmiştir. Çalışma sonunda genel doğruluk oranı %92 bulunmuştur. Önerilen yaklaşım, literatürdeki benzer yöntemlerden daha iyi sonuçlar vermiştir. Bu nedenle yöntemin, bazı bağ hastalıklarının tespit edilmesi ve sınıflandırılmasında güvenilir bir şekilde kullanılabileceği sonucuna varılmıştır. Anahtar Kelimeler: Derin Öğrenme Faster R-CNN Bağcılık
Anahtar Kelime: Belge Türü: Makale Makale Türü: Araştırma Makalesi Erişim Türü: Erişime Açık
- Adem K. 2018. Exudate detection for diabetic retinopathy with circular Hough transformation and convolutional neural networks. Expert Systems with Applications, (114):289-295. doi: 10.1016/j.eswa.2018.07.053
- Adem K, Közkurt C. 2019. Defect detection of seals in multilayer aseptic packages using deep learning. Turk J Elec Eng & Comp Sci, 27:4220-4230. doi:10.3906/elk-1903-112
- Adem K, Ozguven MM, Altas Z. 2022. A sugar beet leaf disease classification method based on image processing and deep learning. Multimedia Tools and Applications, https://doi.org/10.1007/s11042-022-13925-6
- Altaş Z, Özgüven MM, Dilmaç M. 2021. Görüntü işleme teknikleri ile bağ yaprak uyuzu hasarının belirlenmesi. Gaziosmanpaşa Bilimsel Araştırma Dergisi (GBAD), Volume 10:3: 77-87
- Ampatzidis Y, De Bellis L, Luvisi A. 2017. iPathology: robotic applications and management of plants and plant diseases. Sustainability, 9(6):1010. https://doi.org/10.3390/su9061010
- Asraf A, Islam M, Haque M. 2020. Deep learning applications to combat novel coronavirus (COVID-19) pandemic. SN Comput Sci, 1(6):1-7
- Ayon SI, Islam MM. 2019. Diabetes prediction: a deep learning approach. Int J Inform Eng Electron Bus, 12(2):21
- Bock CH, Poole GH, Parker PE, Gottwald TR. 2010. Plant disease severity estimated visually, by digital photography and image analysis, and by hyperspectral imaging. CRC Crit Rev Plant Sci, 29(2):59-107
- Chen Y, Wu Q. 2022. Grape leaf disease identification with sparse data via generative adversarial networks and convolutional neural networks. Precision Agriculture, (2022). https://doi.org/10.1007/s11119-022-09941-z
- Cruz AC, Luvisi A, De Bellis L, Ampatzidis Y. 2017. X-FIDO: an effective application for detecting olive quick decline syndrome with deep learning and data fusion. Front Plant Sci, 8:1741. https://doi.org/10.3389/fpls.2017.01741
- FAO, 2022. Food and Agriculture Organization of the United Nations. FAOSTAT, https://www.fao.org/faostat/en/# data/QCL. (Erişim Tarihi: 25.10.2022)
- Gavhale KR, Ujwalla G. 2014. An overview of the research on crop leaves disease detection using image processing techniques. IOSR J Comput Eng, 16(1):10–16
- Gayathri S, Wise DJW, Shamini PB, Muthu kumaran N. 2020. Image analysis and detection of tea leaf disease using deep learning. In: 2020 International Conference on Electronics and Sustainable Communication Systems (ICESC). IEEE, pp 398–403
- Ghoury S, Sungur C, Durdu A. 2019. Real-time diseases detection of grape and grape leaves using Faster R-CNN and SSDMobileNet Architectures. International Conference on Advanced Technologies, Computer Engineering and Science (ICATCES 2019), Apr 26–28, 2019 Alanya, Türkiye
- Girshick R. 2015. Fast R-CNN. Proceedings of the IEEE international conference on computer vision, Santiago, Chile, pp 1440–1448
- Guo W, Feng Q, Li X, Yang S, Yang J. 2022. Grape leaf disease detection based on attention mechanisms. Int J Agric and Biol Eng, 15(5): 205-2012. Doi: 10.25165/j.ijabe.20221505.7548
- Guti ́errez S, Hern ́andez I, Ceballos S, Barrio I, Navajas AMD, Tardaguila J. 2021. Deep learning for the differentiation of downy mildew and spider mite in grapevine under field conditions. Computers and Electronics in Agriculture, 182:105991. https://doi.org/10.1016/j.compag.2021.105991
- Islam M, Haque M, Iqbal H, Hasan M, HasanM, Kabir MN. 2020. Breast cancer prediction: a comparative study using machine learning techniques. SN Comput Sci, 1(5):1-14
- Islam MM, Karray F, Alhajj R, Zeng J. 2021. A review on deep learning techniques for the diagnosis of novel coronavirus (COVID-19). IEEE Access, 9:30551–30572
- Ji M, Wu Z. 2022. Automatic detection and severity analysis of grape black measles disease based on deep learning and fuzzy logic. Computers and Electronics in Agriculture, 193:106718. https://doi.org/10.1016/j.compag.2022.106718
- Jiang H, Learned-Miller E. 2017. Face detection with the Faster R-CNN. In: 2017 12th IEEE international conference on automatic face & gesture recognition (FG 2017), pp 650–657
- Karabat S. 2014. Türkiye ve Dünya Bağcılığı. Apelasyon, ISSN:2149-4908. http://apelasyon.com/Yazi/33-dunya-ve- turkiye-bagciligi (Erişim Tarihi: 26.08.2019).
- Kavala SAPN, Pothuraju R. 2022. Detection of grape leaf disease using transfer learning methods: VGG16&VGG19. Proceedings of the Sixth International Conference on Computing Methodologies and Communication (ICCMC 2022), IEEE Xplore Part Number: CFP22K25-ART; ISBN: 978-1-6654-1028-1
- Kılıçarslan S. 2022. Kuru üzüm tanelerinin sınıflandırılması için hibrit bir yaklaşım. Müh. Bil. ve Araş. Dergisi, 4(1):62-71. https://doi.org/10.46387/bjesr.1084590
- Lauguico S, Concepcion IIR, Tobias RR, Bandala A, Vicerra RR, Dadios E. 2020. Grape leaf multi-disease detection with confidence value using transfer learning integrated to regions with convolutional neural networks. 2020 IEEE Region 10 Conference (Tencon), Osaka, Japan, November 16-19, 2020
- Liu B, Ding Z, Tian L, He D, Li S, Wang H. 2020. Grape leaf disease identification using improved deep convolutional neural networks. Front Plant Sci, 11:1082. https://doi.org/ 10.3389/fpls.2020.01082
- Lu X, Yang R, Zhou J, Jiao J, Liu F, Liu Y, Su B, Gu P. 2022. A hybrid model of ghost-convolution enlightened transformer for effective diagnosis of grape leaf disease and pest. Journal of King Saud University – Computer and Information Sciences, 34(2022): 1755-1767. https://doi.org/10.1016/j. jksuci.2022.03.006
- Ma J, Du K, Zheng F, Zhang L, Gong Z, Sun Z. 2018. A recognition method for cucumber diseases using leaf symptom ımages based on deep convolutional neural network. Comput Electron Agric 154:18–24. https://doi.org/10.1016/j.compag.2018.08.048
- Özgüven MM. 2018. Hassas tarım. Akfon Yayınları, Ankara (in Turkish). ISBN: 978-605-68762-4-0
- Özgüven MM. 2019. Technological concepts and their differences. International Erciyes Agriculture, Animal and Food Sciences Conference 24–27 April 2019- Erciyes University – Kayseri, Turkiye
- Ozguven MM. 2018. The newest agricultural technologies. Curr Investigations Agric Curr Res, 5(1):573–580. https://doi.org/10.32474/CIACR.2018.05.000201
- Ozguven MM, Adem K. 2019. Automatic detection and classification of leaf spot disease in sugar beet using deep learning algorithms. Physica A, 535:122537
- Ozguven MM. 2020. Deep learning algorithms for automatic detection and classification of mildew disease in cucumber. Fresenius Environ Bull, 29:7081–7087
- Ozguven MM, Altas Z. 2022. A new approach to detect mildew disease on cucumber (Pseudoperonospora cubensis) leaves with image processing. J Plant Pathol. https://doi.org/10.1007/s42161-022-01178-z
- Rossi V. 1995. Effect of host resistance in decreasing infection rate of cercospora leaf spot epidemics on sugarbeet. Phytopathol Mediterr, 34:149–156
- Saleem MH, Potgieter J, Arif KM. 2019. Plant disease detection and classification by deep learning. Plants, 8, 468. doi:10.3390/plants8110468
- Song HA, Lee SY. 2013. Hierarchical representation using NMF. International conference on neural information processing, pp 466–473
- TUİK, 2021. Türkiye İstatistik Kurumu. https://data.tuik.gov.tr/ Kategori/GetKategori?p=tarim-111&dil=1. (Erişim Tarihi: 21.12.2021)
- Wang QQiF. 2019. Tomato diseases recognition based on Faster RCNN. IEEE, 10th International Conference on Information Technology in Medicine and Education (ITME), 78-1-7281– 3918–0. https://doi.org/10.1109/ITME.2019.00176
- Xie X, Ma Y, Liu B, He J, Li S, Wang H. 2020. A deep-learning- based real-time detector for grape leaf diseases using improved convolutional neural networks. Front. Plant Sci, 11:751. doi: 10.3389/fpls.2020.00751
- Yadav S, Sengar N, Singh A, Singh A, Dutta MK. 2021. Identification of disease using deep learning and evaluation of bacteriosis in peach leaf. Ecol Inf, 61:101247. https://doi.org/10.1016/j.ecoinf.2021.101247
APA | ALTAŞ Z, ÖZGÜVEN M, Adem K (2023). Automatic Detection and Classification of Some Vineyard Diseases with Faster R-CNN Model. , 97 - 103. 10.24925/turjaf.v11i1.97-103.5665 |
Chicago | ALTAŞ Ziya,ÖZGÜVEN Mehmet Metin,Adem Kemal Automatic Detection and Classification of Some Vineyard Diseases with Faster R-CNN Model. (2023): 97 - 103. 10.24925/turjaf.v11i1.97-103.5665 |
MLA | ALTAŞ Ziya,ÖZGÜVEN Mehmet Metin,Adem Kemal Automatic Detection and Classification of Some Vineyard Diseases with Faster R-CNN Model. , 2023, ss.97 - 103. 10.24925/turjaf.v11i1.97-103.5665 |
AMA | ALTAŞ Z,ÖZGÜVEN M,Adem K Automatic Detection and Classification of Some Vineyard Diseases with Faster R-CNN Model. . 2023; 97 - 103. 10.24925/turjaf.v11i1.97-103.5665 |
Vancouver | ALTAŞ Z,ÖZGÜVEN M,Adem K Automatic Detection and Classification of Some Vineyard Diseases with Faster R-CNN Model. . 2023; 97 - 103. 10.24925/turjaf.v11i1.97-103.5665 |
IEEE | ALTAŞ Z,ÖZGÜVEN M,Adem K "Automatic Detection and Classification of Some Vineyard Diseases with Faster R-CNN Model." , ss.97 - 103, 2023. 10.24925/turjaf.v11i1.97-103.5665 |
ISNAD | ALTAŞ, Ziya vd. "Automatic Detection and Classification of Some Vineyard Diseases with Faster R-CNN Model". (2023), 97-103. https://doi.org/10.24925/turjaf.v11i1.97-103.5665 |
APA | ALTAŞ Z, ÖZGÜVEN M, Adem K (2023). Automatic Detection and Classification of Some Vineyard Diseases with Faster R-CNN Model. Türk Tarım - Gıda Bilim ve Teknoloji dergisi, 11(1), 97 - 103. 10.24925/turjaf.v11i1.97-103.5665 |
Chicago | ALTAŞ Ziya,ÖZGÜVEN Mehmet Metin,Adem Kemal Automatic Detection and Classification of Some Vineyard Diseases with Faster R-CNN Model. Türk Tarım - Gıda Bilim ve Teknoloji dergisi 11, no.1 (2023): 97 - 103. 10.24925/turjaf.v11i1.97-103.5665 |
MLA | ALTAŞ Ziya,ÖZGÜVEN Mehmet Metin,Adem Kemal Automatic Detection and Classification of Some Vineyard Diseases with Faster R-CNN Model. Türk Tarım - Gıda Bilim ve Teknoloji dergisi, vol.11, no.1, 2023, ss.97 - 103. 10.24925/turjaf.v11i1.97-103.5665 |
AMA | ALTAŞ Z,ÖZGÜVEN M,Adem K Automatic Detection and Classification of Some Vineyard Diseases with Faster R-CNN Model. Türk Tarım - Gıda Bilim ve Teknoloji dergisi. 2023; 11(1): 97 - 103. 10.24925/turjaf.v11i1.97-103.5665 |
Vancouver | ALTAŞ Z,ÖZGÜVEN M,Adem K Automatic Detection and Classification of Some Vineyard Diseases with Faster R-CNN Model. Türk Tarım - Gıda Bilim ve Teknoloji dergisi. 2023; 11(1): 97 - 103. 10.24925/turjaf.v11i1.97-103.5665 |
IEEE | ALTAŞ Z,ÖZGÜVEN M,Adem K "Automatic Detection and Classification of Some Vineyard Diseases with Faster R-CNN Model." Türk Tarım - Gıda Bilim ve Teknoloji dergisi, 11, ss.97 - 103, 2023. 10.24925/turjaf.v11i1.97-103.5665 |
ISNAD | ALTAŞ, Ziya vd. "Automatic Detection and Classification of Some Vineyard Diseases with Faster R-CNN Model". Türk Tarım - Gıda Bilim ve Teknoloji dergisi 11/1 (2023), 97-103. https://doi.org/10.24925/turjaf.v11i1.97-103.5665 |