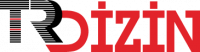
Detection of Some Grape Varieties with Deep Learning Techniques
Yıl: 2023 Cilt: 11 Sayı: 1 Sayfa Aralığı: 125 - 130 Metin Dili: İngilizce DOI: 10.24925/turjaf.v11i1.125-130.5722 İndeks Tarihi: 14-05-2023
Detection of Some Grape Varieties with Deep Learning Techniques
Öz: While determining grape varieties in viticulture, characterization features of shoots, leaves, clusters and fruits are used. These characterization features, in order to form an international method union, with a working team formed on behalf of the "International Board for Plant Genetic Resources" and the "Office International de la Vigne et du Vin-OIV" and "International Board for Plant Genetic Resources". It has been published in a norm called 'Grape Descriptors', developed in collaboration with the international union for the Protection of New Varietes of Plants-UPOV. The ampelographic characteristics of grape varieties are determined according to the characterization features in this norm. Each grape variety has ampelographic characteristics specific to its shoot, leaf, inflorescence and fruit. After these features are determined according to the grape descriptor norm, they are expressed numerically or verbally. In this study, using ampelographic features, Corint, Merlot, Tayfi, Michele palieri, Narince grape varieties were classified using deep learning techniques. The aim is to determine which grape variety it is by using the ampelographic characteristics of grape varieties with deep learning techniques. A new CNN model consisting of 15 layers was created for the study A total of 1028 images of 227×227×3 dimensions of the clusters and fruits of five grape varieties were used in the data set with five classes. 80% of the images are reserved for training and 20% for validation. In the MATLAB program, 96.10% classification success rate was achieved with the new and originally developed CNN model. It was concluded that the CNN model created as a result of the analyzes was successful and could be used in the classification of grape varieties.
Anahtar Kelime: Derin Öğrenme Teknikleri ile Bazı Üzüm Çeşitlerinin Tespiti
Öz: Bağcılıkta üzüm çeşitleri belirlenirken, sürgün, yaprak, salkım ve meyveye ait karakterizasyon özellikler kullanılmaktadır. Bu karakterizasyon özellikler uluslararası yöntem birliğinin oluşması için “Uluslararası Bitki Gen Kaynakları Merkezi” (International Board for Plant Genetic Resources) adına oluşturulmuş bir çalışma ekibi ile “Bağcılık ve Şarapçılık Ofisi” (Office Internatıonal de la Vigne et du Vin-OIV) ve “Uluslararası Yeni Bitki Çeşitlerinin Korunması Birliği” (International union for the Protection of New Varietes of Plants- UPOV) ile işbirliği içinde yapılan çalışmalarla geliştirilmiş ‘Üzüm Tanımlayıcıları’ (Grape Descriptors) adıyla bir normda yayınlanmıştır. Üzüm çeşitlerinin sahip olduğu ampelografik özellikler bu normdaki karakterizasyon özelliklere göre belirlenerek ortaya çıkarılmaktadır. Her üzüm çeşidinin sürgününe, yaprağına, salkımına ve meyvesine özgü ampelografik özellikleri bulunmaktadır. Bu özellikler ‘Üzüm Tanımlayıcıları’ normuna göre belirlendikten sonra sayısal veya sözel olarak ifade edilmektedir. Bu çalışmada ampelografik özellikler kullanılarak Corint, Merlot, Tayfi, Michele palieri, Narince üzüm çeşitlerinin derin öğrenme teknikleri ile sınıflandırılması yapılmıştır. Amaç üzüm çeşitlerinin ampelografik özelliklerini kullanarak derin öğrenme teknikleri ile hangi üzüm çeşidi olduğunu belirlemektir. Yapılan çalışma için 15 katmandan meydana gelen yeni bir CNN modeli oluşturulmuştur. Beş sınıf bulunan veri setinde beş adet üzüm çeşidinin salkım ve meyvelerine ait 227x227x3 boyutunda toplam 1028 adet görüntü kullanılmıştır. Görüntülerin %80’i eğitim için %20’si ise doğrulama için ayrılmıştır. MATLAB programında, yeni ve özgün olarak geliştirilen CNN modeli ile %96,10 sınıflandırma başarım oranı elde edilmiştir. Yapılan analizler neticesinde geliştirilen CNN modelinin başarılı olduğu ve üzüm çeşitlerinin sınıflandırılmasında kullanılabileceği sonucuna varılmıştır.
Anahtar Kelime: Belge Türü: Makale Makale Türü: Araştırma Makalesi Erişim Türü: Erişime Açık
- Adem K. 2018. Exudate detection for diabetic retinopathy with circular Hough transformation and convolutional neural networks. Expert Systems with Applications 2018; (114): 289-295. doi: 10.1016/j.eswa.2018.07.053
- Adem K, Közkurt C. 2019. Defect detection of seals in multilayer aseptic packages using deep learning. Turkish Journal of Electrical Engineering and Computer Sciences: Vol. 27: No. 6, Article 13. https://doi.org/10.3906/elk-1903-112
- Ağaoğlu YS. 1999. Bilimsel ve uygulamalı bağcılık. Cilt-I Asma Biyolojisi. Kavaklıdere Eğitim Yay. No.1, 205 s
- Alleweldt G. 1997. Genetics of grapvine breeding. Progress in Botany, 58:441-454
- Altas Z, Ozguven MM, Yanar Y. 2018. Determination of sugar best leaf spot disease level (cercospora beticola sacc.) with image processing technique by using drone. Current Investigations in Agriculture and Current Research, 5(3):621- 631
- Altuntaş Y, Kocamaz AF, Yeroğlu C. 2019. Identification of apricot varieties using leaf characteristics and KNN classifier. In: 2019 International Artificial Intelligence and Data Processing Symposium (IDAP), pp 1-6
- Anonymous 1997. Descriptors for grapevine (vitis spp.). International Plant Genetic Resources Institute, ISBN 92- 9043-352-3
- Atik İ. 2022. Derin öğrenme yöntemlerini kullanarak bitki yaprağındaki hastalıkların sınıflandırılması. Kahramanmaraş Sütçü İmam Üniversitesi Mühendislik Bilimleri Dergisi, 25(2):126-137
- Bengio Y, Courville A, Vincent P. 2013. Represantation learning: a review and new perspectives, IEEE Trans. Pattern Anal. Mach. Intell., vol. 35, no. 8, pp 1798-1828
- Çelik H, Ağaoğlu YS, Fidan Y, Marasalı B, Söylemezoğlu G. 1998. Genel bağcılık. Sun Fidan Mesleki Kitaplar Serisi-1, 253 s
- Çelik H, Karanis C. 1998. Amasya’da yetiştirilen bazı üzüm çeşitlerinin ampelografik özelliklerinin saptanması üzerine bir araştırma. 4. Bağcılık Sempozyumu, 357-361, Yalova.
- Deng L, Yu D. 2014. Deep learning: methods and applications. Found. Trends Signal Process, vol. 7, no.3-4, pp 197-387, 2014
- Diaz YP, Tomczak JM, Bhulai S. 2021. Deep learning for white cabbege seedling prediction. Computers and Electronics in Agriculture, Volume 184. doi.org/10.1016/j.compag.2021. 106059
- Divakar S, Bhattacharjee A, Priyadarshini R. 2021. Smote-DL: a deep learning-based plant disease detection method. 2021 6th International Conference for Convergence in Technology (I2CT), IEEE. doi: 10.1109/I2CT51068.2021.9417920
- FAO, 2021. Food and Agriculture Organization of the United Nations. http://www.fao.org/faostat/en/#home (Erişim Tarihi: 29.10.2022)
- Karabat S. 2014. Dünya ve Türkiye bağcılığı. Apelasyon e-Dergi, Ocak 2014, Sayı: 2. http:// www.apelasyon.com/Yazi/33- dunya-ve-turkiye-bagciligi. Erişim: 22.09.2022
- Lim TK. 2013. Edible medicinal and non-medicinal plants. Springer Science and Business Media Dordrecht, Volume 6, Fruits, Vitaceae: 450-482 pp
- Mohameth F, Bingcai C, Sada KA. 2020. Plant disease detection with deep learning and feature extraction using plant village. Journal of Computer and Communications, Vol.8:10-22. doi: 10.4236/jcc.2020.86002
- Nasiri A, Omid M, Garavand AT, Jafari A. 2022.Deep learning- based precision agriculture through weed recognition in sugar beet fields. Sustainable Computing: Informatics and Systems. doi.org/10.1016/j.suscom.2022.100759
- Ozguven MM. 2020. Deep learning algorithms for automatic detection and classification of mildew disease in cucumber. Fresenius Environmental Bulletin, 29(08/2020):7081-7087
- Ozguven MM, Altas Z. 2022. A new approach to detect mildew disease on cucumber (Pseudoperonospora cubensis) leaves with image processing. J Plant Pathol. https://doi.org/10.1007/s42161-022-01178-z
- Pınar H, Çöçen E, Yaman M, Uzun A, Sarıtepe Y. 2018. Türk Çekirdekli beyaz dutlarının (morus alba 1) fenolojik ve pomolojik özellikleri. Bahçe 47 (Özel Sayı 2: Uluslararası Tarım Kongresi (UTAK 2018):6-12
- Raei E, Asanjan AA, Nikoo MR, Sadegh M, Pourshahabi S, Aamowski JF. 2022. A deep learning image segmentation model for agriculture irrigation system classification. Computers and Electronics in Agriculture, Volume 198. doi.org/10.1016/j.compag.2022.106977
- Sağlam H, Sağlam Çalkan Ö. 2018. Türkiye bağcılığına tarihsel bir bakış; asma genetik kaynaklarının önemi. Selçuk Tarım ve Gıda Bilimleri Dergisi, 32 (3):601-606
- Song HA, Lee SY. 2013. Hierarchical represantation using NMF. In International Conference on Neural Information Processing, 2013, pp 466-473
- Türkoğlu M, Hanbay D. 2019. Plant disease and pest detection using deep learning- based features. Turkish Journal of Electrical Engineering and Computer Sciences, (2019) 27: 1636-1651. doi:10.3906/elk-1809-181
- Ünal MS. 2020. Şırnak yöresel üzüm çeşitlerinin bazı özelliklerinin belirlenmesi. ÇOMÜ Zir. Fak. Derg. (COMU J. Agric. Fac.), 8(2):277-287. doi: 10.33202/comuagri.740162
- Yamamoto K, Togami T, Yamaguchi N. 2017. Super-resolution of plant disease images for the acceleration of image-based phenotyping and vigor diagnosis in agriculture. Sensors, 17 (11):2557. doi.org/10.3390/s17112557
- Xia EQ, Deng GF, Guo YJ, Li HB. 2010. Biological activities of polyphenols from grapes. International Journal of Molecular Science, pp 622-646
APA | TERZİ İ, ÖZGÜVEN M, YAGCI A (2023). Detection of Some Grape Varieties with Deep Learning Techniques. , 125 - 130. 10.24925/turjaf.v11i1.125-130.5722 |
Chicago | TERZİ İsmail,ÖZGÜVEN Mehmet Metin,YAGCI ADEM Detection of Some Grape Varieties with Deep Learning Techniques. (2023): 125 - 130. 10.24925/turjaf.v11i1.125-130.5722 |
MLA | TERZİ İsmail,ÖZGÜVEN Mehmet Metin,YAGCI ADEM Detection of Some Grape Varieties with Deep Learning Techniques. , 2023, ss.125 - 130. 10.24925/turjaf.v11i1.125-130.5722 |
AMA | TERZİ İ,ÖZGÜVEN M,YAGCI A Detection of Some Grape Varieties with Deep Learning Techniques. . 2023; 125 - 130. 10.24925/turjaf.v11i1.125-130.5722 |
Vancouver | TERZİ İ,ÖZGÜVEN M,YAGCI A Detection of Some Grape Varieties with Deep Learning Techniques. . 2023; 125 - 130. 10.24925/turjaf.v11i1.125-130.5722 |
IEEE | TERZİ İ,ÖZGÜVEN M,YAGCI A "Detection of Some Grape Varieties with Deep Learning Techniques." , ss.125 - 130, 2023. 10.24925/turjaf.v11i1.125-130.5722 |
ISNAD | TERZİ, İsmail vd. "Detection of Some Grape Varieties with Deep Learning Techniques". (2023), 125-130. https://doi.org/10.24925/turjaf.v11i1.125-130.5722 |
APA | TERZİ İ, ÖZGÜVEN M, YAGCI A (2023). Detection of Some Grape Varieties with Deep Learning Techniques. Türk Tarım - Gıda Bilim ve Teknoloji dergisi, 11(1), 125 - 130. 10.24925/turjaf.v11i1.125-130.5722 |
Chicago | TERZİ İsmail,ÖZGÜVEN Mehmet Metin,YAGCI ADEM Detection of Some Grape Varieties with Deep Learning Techniques. Türk Tarım - Gıda Bilim ve Teknoloji dergisi 11, no.1 (2023): 125 - 130. 10.24925/turjaf.v11i1.125-130.5722 |
MLA | TERZİ İsmail,ÖZGÜVEN Mehmet Metin,YAGCI ADEM Detection of Some Grape Varieties with Deep Learning Techniques. Türk Tarım - Gıda Bilim ve Teknoloji dergisi, vol.11, no.1, 2023, ss.125 - 130. 10.24925/turjaf.v11i1.125-130.5722 |
AMA | TERZİ İ,ÖZGÜVEN M,YAGCI A Detection of Some Grape Varieties with Deep Learning Techniques. Türk Tarım - Gıda Bilim ve Teknoloji dergisi. 2023; 11(1): 125 - 130. 10.24925/turjaf.v11i1.125-130.5722 |
Vancouver | TERZİ İ,ÖZGÜVEN M,YAGCI A Detection of Some Grape Varieties with Deep Learning Techniques. Türk Tarım - Gıda Bilim ve Teknoloji dergisi. 2023; 11(1): 125 - 130. 10.24925/turjaf.v11i1.125-130.5722 |
IEEE | TERZİ İ,ÖZGÜVEN M,YAGCI A "Detection of Some Grape Varieties with Deep Learning Techniques." Türk Tarım - Gıda Bilim ve Teknoloji dergisi, 11, ss.125 - 130, 2023. 10.24925/turjaf.v11i1.125-130.5722 |
ISNAD | TERZİ, İsmail vd. "Detection of Some Grape Varieties with Deep Learning Techniques". Türk Tarım - Gıda Bilim ve Teknoloji dergisi 11/1 (2023), 125-130. https://doi.org/10.24925/turjaf.v11i1.125-130.5722 |