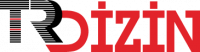
Determining the Short-term Susceptibility of Construction Workers to Occupational Accidents Using Stochastic Gradient Boosting
Yıl: 2023 Cilt: 6 Sayı: 1 Sayfa Aralığı: 5 - 19 Metin Dili: İngilizce DOI: 10.31462/jcemi.2023.01001015 İndeks Tarihi: 15-05-2023
Determining the Short-term Susceptibility of Construction Workers to Occupational Accidents Using Stochastic Gradient Boosting
Öz: Employees working on construction projects have a higher risk of experiencing occupational accidents compared to workers in other sectors. Newly employed workers might face an occupational accident shortly after they start working due to not being able to notice risky environmental conditions. Despite existing studies in construction safety literature focusing on several output variables such as fatality, accident type or accident severity predictions, no studies have examined the short-term susceptibility of construction workers to occupational injuries. This study aims to develop a model to predict construction workers’ susceptibility to short-term occupational accidents using interpretable machine learning (ML) methods. Hence, the primary research objective is to identify construction workers who have high probability of experiencing an occupational accident shortly after their employment. In this respect, a national dataset of occupational accidents encountered in the construction industry in Turkey was collected and subjected to various pre-processing elements (data cleaning, data scaling, and data resampling) to prepare the data for prediction. At the processing step, Stochastic Gradient Boosting (SGB) algorithm was applied for the classification purpose. In the next step, Shapley Additive Explanations (SHAP) was used as an interpretable artificial intelligence algorithm to explain how, to what extent, and in which direction the input variables affect the prediction scheme, which is another distinguishing feature of the present study compared to past studies in the subject matter. Results show that the proposed SGB model is a powerful detector for the classification problem and salary of workers, past accident in the company, and number of workers in the company were the most influencing factors. Overall, this study contributes to practice by improving the safety conditions of the newly employed workers as well as minimizing their accident probability through intensified safety training. Given that contemporary safety management applications demand a new set of data-driven inputs, proposed model is expected to help industry professionals and safety managers apply more robust safety risk mitigation and/or prevention measures.
Anahtar Kelime: Belge Türü: Makale Makale Türü: Araştırma Makalesi Erişim Türü: Erişime Açık
- Laitinen H, Päivärinta K (2010) A new-generation safety contest in the construction industry - A long- term evaluation of a real-life intervention. Safety Science 48(5):680–686.
- Koc K, Gurgun AP (2021) Stakeholder-Associated Life Cycle Risks in Construction Supply Chain. Journal of Management in Engineering 37(1):04020107.
- Tözer KD, Gürcanlı GE, Zalihe Yarkıner (2022) Analysis of workday losses due to falls from scaffoldings in the construction industry. Journal of Construction Engineering, Management & Innovation 5(1):15–27.
- Nnaji C, Gambatese J, Karakhan A, Osei-Kyei R (2020) Development and Application of Safety Technology Adoption Decision-Making Tool. Journal of Construction Engineering and Management 146(4):04020028.
- Yılmaz F (2021) Analysis of the interaction between safety measures and workers’ safety awareness from the construction workers’ perspective. Eng Constr Archit Manag. doi: 10.1108/ECAM-07-2021-0564.
- Mostofi F, Toğan V, Ayözen YE, Tokdemir OB (2022) Construction Safety Risk Model with Construction Accident Network: A Graph Convolutional Network Approach. Sustainability 14(23):15906.
- Koc K, Ekmekcioğlu Ö, Gurgun AP (2022) Accident prediction in construction using hybrid wavelet-machine learning. Automation in Construction 133(February 2021):103987.
- Toğan V, Mostofi F, Ayözen Y, Tokdemir OB (2022) Customized AutoML: An Automated Machine Learning System for Predicting Severity of Construction Accidents. Buildings 12(11):1933.
- Ayhan BU, Tokdemir OB (2020) Accident Analysis for Construction Safety Using Latent Class Clustering and Artificial Neural Networks. Journal of Construction Engineering and Management 146(3):04019114.
- Choi J, Gu B, Chin S, Lee JS (2020) Machine learning predictive model based on national data for fatal accidents of construction workers. Automation in Construction 110(September 2019):102974.
- Goh YM, Ubeynarayana CU (2017) Construction accident narrative classification: An evaluation of text mining techniques. Accident Analysis and Prevention 108(May):122–130.
- Koc K, Gurgun AP (2022) Scenario-based automated data preprocessing to predict severity of construction accidents. Automation in Construction 140(May):104351.
- Ayhan BU, Tokdemir OB (2019) Predicting the outcome of construction incidents. Safety Science 113(November 2018):91–104.
- Baker H, Hallowell MR, Tixier AJ (2020) AI-based prediction of independent construction safety outcomes from universal attributes. Automation in Construction 118(June):103146.
- Gondia A, Ezzeldin M, El-Dakhakhni W (2022) Machine Learning–Based Decision Support Framework for Construction Injury Severity Prediction and Risk Mitigation. ASCE-ASME Journal of Risk and Uncertainty in Engineering Systems, Part A: Civil Engineering 8(3):1–17.
- Ajayi A, Oyedele L, Owolabi H, Akinade O, Bilal M, Davila Delgado JM, Akanbi L (2020) Deep Learning Models for Health and Safety Risk Prediction in Power Infrastructure Projects. Risk Analysis 40(10):2019–2039.
- Koc K, Ekmekcioğlu Ö, Gurgun AP (2022) Prediction of construction accident outcomes based on an imbalanced dataset through integrated resampling techniques and machine learning methods. Eng Constr Archit Manag. doi: 10.1108/ECAM-04-2022-0305.
- Goh YM, Ubeynarayana CU, Wong KLX, Guo BHW (2018) Factors influencing unsafe behaviors: A supervised learning approach. Accident Analysis and Prevention 118(June):77–85.
- Koc K, Ekmekcioğlu Ö, Gurgun AP (2023) Developing a national data-driven construction safety management framework with interpretable fatal accident prediction. Journal of Construction Engineering and Management 149(4):04.
- Kang K, Ryu H (2019) Predicting types of occupational accidents at construction sites in Korea using random forest model. Safety Science 120:226–236.
- Mistikoglu G, Gerek IH, Erdis E, Mumtaz Usmen PE, Cakan H, Kazan EE (2015) Decision tree analysis of construction fall accidents involving roofers. Expert Systems with Applications 42(4):2256–2263.
- Chakraborty D, Elhegazy H, Elzarka H, Gutierrez L (2020) A novel construction cost prediction model using hybrid natural and light gradient boosting. Advanced Engineering Informatics 46(April):101201.
- Pan Y, Zhang L (2021) Roles of artificial intelligence in construction engineering and management: A critical review and future trends. Automation in Construction 122(August 2020):103517.
- Baker H, Hallowell MR, Tixier AJ-P (2020) AI- based prediction of independent construction safety outcomes from universal attributes. Automation in Construction 118:103146.
- Zhang F, Fleyeh H, Wang X, Lu M (2019) Construction site accident analysis using text mining and natural language processing techniques. Automation in Construction 99(December 2018):238–248.
- Kang K, Ryu H (2019) Predicting types of occupational accidents at construction sites in Korea using random forest model. Safety Science 120:226–236.
- Lee JY, Yoon YG, Oh TK, Park S, Ryu SI (2020) A study on data pre-processing and accident prediction modelling for occupational accident analysis in the construction industry. Applied Sciences (Switzerland) 10(21):1–23.
- Ekmekcioğlu Ö, Koc K (2022) Explainable step- wise binary classification for the susceptibility assessment of geo-hydrological hazards. Catena 216(February):106379.
- LuoM,XieJ,YanY,KeZ,YuP,WangZ,Zhang J (2020) Comparing machine learning algorithms in predicting thermal sensation using ASHRAE Comfort Database II. Energy and Buildings 210:109776.
- Rahmati O, Pourghasemi HR (2017) Identification of Critical Flood Prone Areas in Data-Scarce and Ungauged Regions: A Comparison of Three Data Mining Models. Water Resources Management 31(5):1473–1487.
- Koc K, Ekmekcioğlu Ö, Gurgun AP (2021) Integrating feature engineering, genetic algorithm and tree-based machine learning methods to predict the post-accident disability status of construction workers. Automation in Construction 131:103896.
- Ekmekcioğlu Ö, Koc K, Özger M, Işık Z (2022) Exploring the additional value of class imbalance distributions on interpretable flash flood susceptibility prediction in the Black Warrior River basin, Alabama, United States. Journal of Hydrology 610(March):127877.
- Friedman JH (2002) Stochastic gradient boosting. Computational Statistics & Data Analysis 38(4):367–378.
- Ke G, Meng Q, Finley T, Wang T, Chen W, Ma W, Ye Q, Liu TY (2017) LightGBM: A highly efficient gradient boosting decision tree. Advances in Neural Information Processing Systems 2017- Decem(Nips):3147–3155.
- Bentéjac C, Csörgő A, Martínez-Muñoz G (2021) A comparative analysis of gradient boosting algorithms. Artificial Intelligence Review 54(3):1937–1967.
- Ayhan BU, Tokdemir OB (2019) Safety assessment in megaprojects using artificial intelligence. Safety Science 118(March):273–287.
- Ayhan BU, Tokdemir OB (2019) Predicting the outcome of construction incidents. Safety Science 113(November 2018):91–104.
- Poh CQX, Ubeynarayana CU, Goh YM (2018) Safety leading indicators for construction sites: A machine learning approach. Automation in Construction 93(October 2017):375–386.
- Canbek G, Taskaya Temizel T, Sagiroglu S (2021) BenchMetrics: a systematic benchmarking method for binary classification performance metrics. Neural Computing and Applications 33(21):14623–14650.
- Zhu R, Hu X, Hou J, Li X (2021) Application of machine learning techniques for predicting the consequences of construction accidents in China. Process Safety and Environmental Protection 145:293–302.
- Fryer D, Strumke I, Nguyen H (2021) Shapley Values for Feature Selection: The Good, the Bad, and the Axioms. IEEE Access 9:144352–144360.
- Naser MZ (2021) An engineer’s guide to eXplainable Artificial Intelligence and Interpretable Machine Learning: Navigating causality, forced goodness, and the false perception of inference. Automation in Construction 129(July):103821.
- Wang R, Kim JH, Li MH (2021) Predicting stream water quality under different urban development pattern scenarios with an interpretable machine learning approach. Science of the Total Environment 761:144057.
- Wang R, Asghari V, Cheung CM, Hsu SC, Lee CJ (2022) Assessing effects of economic factors on construction cost estimation using deep neural networks. Automation in Construction 134(December 2021):104080.
- Dikshit A, Pradhan B (2021) Interpretable and explainable AI (XAI) model for spatial drought prediction. Science of the Total Environment 801:149797.
- Alizadeh SS, Mortazavi SB, Sepehri MM (2015) Assessment of accident severity in the construction industry using the Bayesian theorem. International Journal of Occupational Safety and Ergonomics 21(4):551–557.
- Hidaka A, Miyake A (2015) Selection of Suitable Safety Activities and Experience of Safety Condition Establishment. Open Journal of Safety Science and Technology 05(01):1–9.
- Ustabaş İ, Sarı H, Fandaklı S (2021) The effect of occupational choice on job satisfaction and occupational accidents in the construction sector: A case study on Rize and Çorum in Turkey. Journal of Construction Engineering, Management & Innovation 4(4):198–209.
APA | Koc K (2023). Determining the Short-term Susceptibility of Construction Workers to Occupational Accidents Using Stochastic Gradient Boosting. , 5 - 19. 10.31462/jcemi.2023.01001015 |
Chicago | Koc Kerim Determining the Short-term Susceptibility of Construction Workers to Occupational Accidents Using Stochastic Gradient Boosting. (2023): 5 - 19. 10.31462/jcemi.2023.01001015 |
MLA | Koc Kerim Determining the Short-term Susceptibility of Construction Workers to Occupational Accidents Using Stochastic Gradient Boosting. , 2023, ss.5 - 19. 10.31462/jcemi.2023.01001015 |
AMA | Koc K Determining the Short-term Susceptibility of Construction Workers to Occupational Accidents Using Stochastic Gradient Boosting. . 2023; 5 - 19. 10.31462/jcemi.2023.01001015 |
Vancouver | Koc K Determining the Short-term Susceptibility of Construction Workers to Occupational Accidents Using Stochastic Gradient Boosting. . 2023; 5 - 19. 10.31462/jcemi.2023.01001015 |
IEEE | Koc K "Determining the Short-term Susceptibility of Construction Workers to Occupational Accidents Using Stochastic Gradient Boosting." , ss.5 - 19, 2023. 10.31462/jcemi.2023.01001015 |
ISNAD | Koc, Kerim. "Determining the Short-term Susceptibility of Construction Workers to Occupational Accidents Using Stochastic Gradient Boosting". (2023), 5-19. https://doi.org/10.31462/jcemi.2023.01001015 |
APA | Koc K (2023). Determining the Short-term Susceptibility of Construction Workers to Occupational Accidents Using Stochastic Gradient Boosting. Journal of Construction Engineering, Management & Innovation (Online), 6(1), 5 - 19. 10.31462/jcemi.2023.01001015 |
Chicago | Koc Kerim Determining the Short-term Susceptibility of Construction Workers to Occupational Accidents Using Stochastic Gradient Boosting. Journal of Construction Engineering, Management & Innovation (Online) 6, no.1 (2023): 5 - 19. 10.31462/jcemi.2023.01001015 |
MLA | Koc Kerim Determining the Short-term Susceptibility of Construction Workers to Occupational Accidents Using Stochastic Gradient Boosting. Journal of Construction Engineering, Management & Innovation (Online), vol.6, no.1, 2023, ss.5 - 19. 10.31462/jcemi.2023.01001015 |
AMA | Koc K Determining the Short-term Susceptibility of Construction Workers to Occupational Accidents Using Stochastic Gradient Boosting. Journal of Construction Engineering, Management & Innovation (Online). 2023; 6(1): 5 - 19. 10.31462/jcemi.2023.01001015 |
Vancouver | Koc K Determining the Short-term Susceptibility of Construction Workers to Occupational Accidents Using Stochastic Gradient Boosting. Journal of Construction Engineering, Management & Innovation (Online). 2023; 6(1): 5 - 19. 10.31462/jcemi.2023.01001015 |
IEEE | Koc K "Determining the Short-term Susceptibility of Construction Workers to Occupational Accidents Using Stochastic Gradient Boosting." Journal of Construction Engineering, Management & Innovation (Online), 6, ss.5 - 19, 2023. 10.31462/jcemi.2023.01001015 |
ISNAD | Koc, Kerim. "Determining the Short-term Susceptibility of Construction Workers to Occupational Accidents Using Stochastic Gradient Boosting". Journal of Construction Engineering, Management & Innovation (Online) 6/1 (2023), 5-19. https://doi.org/10.31462/jcemi.2023.01001015 |