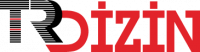
Joint modeling of survival and longitudinal data: Carrico index data example
Yıl: 2023 Cilt: 6 Sayı: 1 Sayfa Aralığı: 24 - 37 Metin Dili: İngilizce DOI: 10.30714/j-ebr.2022.167 İndeks Tarihi: 17-05-2023
Joint modeling of survival and longitudinal data: Carrico index data example
Öz: Aim: When the respiratory system is unable to adequately absorb oxygen or excrete carbon dioxide, acute respiratory failure (ARF) develops. A current area of study is the survival analysis of patients with acute hypercapnic respiratory failure (AHRF) in the field of pulmonary diseases. In the follow-up period, several biochemical markers are repeatedly measured, such as respiration rate and Carrico Index; however, baseline or averaged values are mostly related to treatment failure. Although this approach is not inaccurate, it causes information loss, which leads to biased estimates. This prospective cohort study primarily looked at the relationship between changes in Carrico Index and failure of treatment in AHRF patients. Methods: We included 86 patients from Ankara University School of Medicine Pulmonary Diseases Department. The association between the trajectory of the Carrico Index and failure in AHRF patients was examined using a joint modeling approach for longitudinal and survival data. Results: Results showed that averaged Carrico Index change was inversely and significantly associated with failure (HR: 0.97, 95% CI: -0.05 to 1.97). With hazard ratios of 1.43 and 1.4, chronic health evaluation II (Apache II), and COPD Assesment test (CAT) were positively correlated with failure risk. Conclusions: The present study demonstrate that applying the risk predictors' trajectory through an appropriate statistical method improved accuracy and avoid biased results.
Anahtar Kelime: Belge Türü: Makale Makale Türü: Araştırma Makalesi Erişim Türü: Erişime Açık
- [1]Tschirgi RD, Gerard RW. The carotid- mandibular reflex in acute respiratory failure. Am J Physiol. 1947; 150(2): 358-64.
- [2]Ahmed Y, Adam M, Bakkar LM. Effectiveness of APACHE II and SAPS II scoring models in foreseeing the outcome of critically ill COPD patients. Egypt J Bronchol. 2019; 13(5): 654-59.
- [3]Alaithan AM, Memon JI, Rehmani RS, et al. Chronic obstructive pulmonary disease: hospital and intensive care unit outcomes in the Kingdom of Saudi Arabia. Int J Chron Obstruct Pulmon Dis. 2012; 7:819-23.
- [4]Zheng DW, Wang CZ, Liu RS, et al. The application of improved Glasgow coma scale score of 15 as switching point for invasive noninvasive mechanical ventilation in treatment of severe respiratory failure in chronic obstructive pulmonary disease]. Zhongguo Wei Zhong Bing Ji Jiu Yi Xue. 2011;23(4):224-7.
- [5]Basol M, Goksuluk D, Sipahioglu MH, et al. Effect of serum albumin changes on mortality in patients with peritoneal dialysis: a joint modeling approach and personalized dynamic risk predictions. Biomed Res. Int. 2021; 2021, 6612464.
- [6]Yu M, Taylor JMG, Sandler HM. Individual prediction in prostate cancer studies using a joint longitudinal survival–cure model. J Am Stat Assoc. 2008; 103(481): 178-87.
- [7]Yang L, Yu M, Gao S. Joint models for multiple longitudinal processes and time-to- event outcome. J Stat Comput Simulat. 2016; 86(18): 3682-3700.
- [8]Ibrahim JG, Chu H, Chen LM. Basic concepts and methods for joint models of longitudinal and survival data. J Clin Oncol. 2010; 28(16): 2796.
- [9]Ediebah DE, Galindo-Garre F, Uitdehaag BM, et al. Joint modeling of longitudinal health-related quality of life data and survival. Qual Lif Res. 2015; 24(4), 795-804.
- [10] Lim HJ, Mondal P, Skinner S. Joint modeling of longitudinal and event time data: application to HIV study. J Med Stat Informat. 2013; 1(1): 1.
- [11] Rizopoulos D. Joint models for longitudinal and time-to-event data: With applications in R. CRC press; 2012.
- [12] Fagyas M, Fejes Z, Sütő R, et al. Circulating ACE2 activity predicts mortality and disease severity in hospitalized COVID-19 patients. Int J Infect Dis. 2022; 115: 8-16.
- [13] Konar NM, Karaismailoglu E, Karaagaoglu E. The Use of Joint Modeling Approach in Personalized Medicine, In: Abstracts of the 9th Conference of the Eastern Mediterranean Region and the Italian Region of the nternational Biometric Society. Thessalonoki, Greece, 8-12 May 2017, pp. 91.
- [14] Rizopoulos D, Taylor JM, Van Rosmalen J, et al. Personalized screening intervals for biomarkers using joint models for longitudinal and survival data. Biostatistics. 2016; 17(1): 149-64.
- [15] Çiftci F, Çiledağ A, Erol S, et al. Non-invasive ventilation for acute hypercapnic respiratory failure in older patients. Wiener klinische Wochenschrift. 2017; 129(19): 680-86.
- [16] Therneau T. A package for survival analysis in S. R package version, 2015. 2(7). Available from: https://CRAN.R- project.org/package=survival. 2022/
- [17] Pinheiro J, Bates D, DebRoy S, et al. Linear and nonlinear mixed effects models. R package version, 2007; 3(57): 1-89. R package version 3. pp. 1-157. Available from: https://CRAN.R- project.org/package=nlme/
- [18] Rizopoulos D. JM: An R package for the joint modelling of longitudinal and time-to-event data. J Stat Soft. 2010; 35: 1-33.
- [19] Kleinbaum DG, Klein M. Survival analysis: a self-learning text (Vol. 3). New York: Springer; 2012.
- [20] Auton T Applied survival analysis: regression modelling of time to event data. J Appl Stat. 2000; 27(1): 132-133.
- [21] Klein JP, Moeschberger ML. Survival analysis: techniques for censored and truncated data (Vol. 1230). New York: Springer; 2003.
- [22] Seeley E, McAuley DF, Eisner M, et al. Predictors of mortality in acute lung injury during the era of lung protective ventilation. Thorax. 2008; 63(11): 994-98.
- [23] Satou T, Imamura H, Mochiduki K, et al. Efficacy of protocol based non invasive positive pressure ventilation for acute respiratory distress syndrome: a retrospective observational study. Acute Med Surg. 2020; 7(1): e465.
- [24] Antonelly M, Conti, G, Esquinas A. A multiple-centre survey on the use in clinical practice of noninvasive ventilation as a first- line intervention for acute respiratory distress syndrome. Crit Care Med. 2007; 35(1): 18-25.
- [25] Zhang L, Wang Z, Xu F, et al. The Role of Glucocorticoids in the Treatment of ARDS: A Multicenter Retrospective Study Based on the eICU Collaborative Research Database. Front Med. 2021;8: 678260.
APA | TURGAL E, ERDOĞAN b, Arslan F (2023). Joint modeling of survival and longitudinal data: Carrico index data example. , 24 - 37. 10.30714/j-ebr.2022.167 |
Chicago | TURGAL EBRU,ERDOĞAN beyza,Arslan Fatma Joint modeling of survival and longitudinal data: Carrico index data example. (2023): 24 - 37. 10.30714/j-ebr.2022.167 |
MLA | TURGAL EBRU,ERDOĞAN beyza,Arslan Fatma Joint modeling of survival and longitudinal data: Carrico index data example. , 2023, ss.24 - 37. 10.30714/j-ebr.2022.167 |
AMA | TURGAL E,ERDOĞAN b,Arslan F Joint modeling of survival and longitudinal data: Carrico index data example. . 2023; 24 - 37. 10.30714/j-ebr.2022.167 |
Vancouver | TURGAL E,ERDOĞAN b,Arslan F Joint modeling of survival and longitudinal data: Carrico index data example. . 2023; 24 - 37. 10.30714/j-ebr.2022.167 |
IEEE | TURGAL E,ERDOĞAN b,Arslan F "Joint modeling of survival and longitudinal data: Carrico index data example." , ss.24 - 37, 2023. 10.30714/j-ebr.2022.167 |
ISNAD | TURGAL, EBRU vd. "Joint modeling of survival and longitudinal data: Carrico index data example". (2023), 24-37. https://doi.org/10.30714/j-ebr.2022.167 |
APA | TURGAL E, ERDOĞAN b, Arslan F (2023). Joint modeling of survival and longitudinal data: Carrico index data example. Experimental Biomedical Research, 6(1), 24 - 37. 10.30714/j-ebr.2022.167 |
Chicago | TURGAL EBRU,ERDOĞAN beyza,Arslan Fatma Joint modeling of survival and longitudinal data: Carrico index data example. Experimental Biomedical Research 6, no.1 (2023): 24 - 37. 10.30714/j-ebr.2022.167 |
MLA | TURGAL EBRU,ERDOĞAN beyza,Arslan Fatma Joint modeling of survival and longitudinal data: Carrico index data example. Experimental Biomedical Research, vol.6, no.1, 2023, ss.24 - 37. 10.30714/j-ebr.2022.167 |
AMA | TURGAL E,ERDOĞAN b,Arslan F Joint modeling of survival and longitudinal data: Carrico index data example. Experimental Biomedical Research. 2023; 6(1): 24 - 37. 10.30714/j-ebr.2022.167 |
Vancouver | TURGAL E,ERDOĞAN b,Arslan F Joint modeling of survival and longitudinal data: Carrico index data example. Experimental Biomedical Research. 2023; 6(1): 24 - 37. 10.30714/j-ebr.2022.167 |
IEEE | TURGAL E,ERDOĞAN b,Arslan F "Joint modeling of survival and longitudinal data: Carrico index data example." Experimental Biomedical Research, 6, ss.24 - 37, 2023. 10.30714/j-ebr.2022.167 |
ISNAD | TURGAL, EBRU vd. "Joint modeling of survival and longitudinal data: Carrico index data example". Experimental Biomedical Research 6/1 (2023), 24-37. https://doi.org/10.30714/j-ebr.2022.167 |