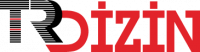
Tahıl Emtia Fiyat Oynaklığının Markov Değişim Asimetrik Garch Modelleriyle İncelenmesi
Yıl: 2023 Cilt: 15 Sayı: 1 Sayfa Aralığı: 500 - 513 Metin Dili: Türkçe DOI: 10.20491/isarder.2023.1600 İndeks Tarihi: 20-05-2023
Tahıl Emtia Fiyat Oynaklığının Markov Değişim Asimetrik Garch Modelleriyle İncelenmesi
Öz: Amaç – Finansal piyasa oynaklığı hem ekonomik performans hem de finansal istikrar göstergesi olarak önemli rol oynamaktadır. Alternatif GARCH tipi zaman serisi modelleri, bu amacın gerçekleştirilmesinde en çok tercih edilen yaklaşımlardan olmuşlardır. Finansal zaman serilerinde meydana gelen yapısal değişiklikleri modellemede de MS-GARCH yaklaşımı kullanımı ortaya çıkmıştır. Bu çalışma vadeli tahıl emtia piyasalarında işlem gören dört ana tahıl emtia (buğday, yulaf, mısır ve soya fasulyesi) getirilerinin oynaklığı asimetrik MS-GARCH yapılarıyla modelleyerek ampirik literatüre katkıda bulunmayı amaçlamaktadır. Yöntem – Araştırmanın amacı doğrultusunda, asimetrik MS-GARCH modelleri kullanılarak dört tahıl emtiası için oynaklık analizleri gerçekleştirilmiştir. Araştırmada kullanılan veri setleri investing.com veri tabanından günlük olarak elde edilmiş olup, 2014-2022 dönemini kapsamaktadır. Araştırmanın analizlerinde RStudio 2022.07.2 programı kullanılmıştır. Bulgular – Çalışmada gerçekleştirilen asimetrik MS-GARCH modellerinden elde edilen sonuçlara göre, buğday, yulaf, mısır ve soya fasulyesi emtialarıda ısrarcı rejim yapılarının varlığı belirlenmiştir. Emtia getirilerinin düşük oynaklık dönemindeyken tekrar düşük oynaklık döneminde veya yüksek oynaklık dönemindeyken tekrar yüksek oynaklık döneminde kaldığı sonucuna ulaşılmıştır. Ele alınan bütün emtiaların düşük oynaklık rejimindeyken meydana gelen negatif şokların, pozitif şoklara göre oynaklık üzerinde daha fazla etki ortaya çıkardığı tespit edilmiştir. Tahıl emtia getirilerinde kaldıraç etkisinin söz konusu olduğu belirlenmiştir. Buğday getirilerinin %25, mısır getirilerinin %21, soya fasulyesi getirilerinin %1,2 ve yulaf getirilerinin %12 yüksek oynaklık döneminde kaldığı görülmüştür. Tartışma – Fiyat oynaklıklarının farklı rejim yapılarına göre analiz edilmesini sağlayan MS-GARCH tipi modellerin kullanılması yüksek belirsizlik ortamında daha kesin öngörüler yapılmasını sağlayarak, meydana gelebilecek risklerin azaltılmasına sebep olacaktır.
Anahtar Kelime: Investigation of Grain Commodity Price Volatility Using Markov Switching Asymmetric Garch Models
Öz: Purpose – Financial market volatility plays an important role as an indicator of both economic performance and financial stability. Alternative GARCH type time series models have been one of the most preferred approaches to achieve this goal. The use of the MS-GARCH approach has also emerged in modeling the structural changes in financial time series. This study aims to contribute to the empirical literature by modeling the volatility of the returns of the four main grain commodities (wheat, oats, corn and soybeans) traded in the futures grain commodity markets with asymmetric MS-GARCH structures. Design/methodology/approach – For the purpose of the research, volatility analyzes were performed for four grain commodities using asymmetric MS-GARCH models. The data sets used in the research were obtained from the investing.com database on a daily basis and cover the period of 2014-2022. RStudio 2022.07.2 program was used in the analysis of the research. Findings – According to the results obtained from the asymmetric MS-GARCH models performed in the study, the existence of persistent regime structures was determined in wheat, oat, corn and soybean commodities. It was concluded that commodity returns remained in the low volatility period when they were in the low volatility period or again in the high volatility period when they were in the high volatility period. It has been determined that negative shocks that occur when all commodities are in the low volatility regime have more impact on volatility than positive shocks. It has been determined that there is a leverage effect on the grain commodity returns. It was observed that wheat yields were 25%, corn yields 21%, soybean yields 1.2% and oat yields 12% in high volatility period. Discussion – The use of MS-GARCH type models, which enable the analysis of price volatility according to different regime structures, will enable more precise predictions to be made in an environment of high uncertainty, and reduce the risks that may occur.
Anahtar Kelime: Belge Türü: Makale Makale Türü: Araştırma Makalesi Erişim Türü: Erişime Açık
- Anderluh, J., & Borovkova, S. (2008). Commodity volatility modelling and option pricing with a potential function approach. European journal of Finance, 14(2), 91-113.
- Ang, A., Bekaert, G., & Liu, J. (2005). Why stocks may disappoint. Journal of Financial Economics, 76(3), 471-508.
- Ardia, D. (2008). Financial risk management with Bayesian estimation of GARCH models (Vol. 612). Heidelberg: Springer.
- Ardia, D., Bluteau, K., & Rüede, M. (2019). Regime changes in Bitcoin GARCH volatility dynamics. Finance Research Letters, 29, 266-271.
- Ardia, D., Bluteau, K., Boudt, K., & Catania, L. (2018). Forecasting risk with Markov-switching GARCH models: A large-scale performance study. International Journal of Forecasting, 34(4), 733-747.
- Ardia, D., Bluteau, K., Boudt, K., Catania, L., & Trottier, D. A. (2019). Markov-switching GARCH models in R: The MSGARCH package. Journal of Statistical Software, 91(4).
- Augustyniak, M. (2014). Maximum likelihood estimation of the Markov-switching GARCH model. Computational Statistics & Data Analysis, 76, 61-75.
- Bauwens, L., De Backer, B., & Dufays, A. (2014). A Bayesian method of change-point estimation with recurrent regimes: Application to GARCH models. Journal of Empirical Finance, 29, 207-229.
- Bellemare, M. F., Barrett, C. B., & Just, D. R. (2013). The welfare impacts of commodity price volatility: evidence from rural Ethiopia. American Journal of Agricultural Economics, 95(4), 877-899.
- Black, F. (1976). Studies of stock market volatility changes. 1976 Proceedings of the American statistical association bisiness and economic statistics section.
- Bollerslev, T. (1986). Generalized autoregressive conditional heteroskedasticity. Journal of econometrics, 31(3), 307-327.
- Bouët, A., & Laborde Debucquet, D. (2012). Food crisis and export taxation: the cost of non-cooperative trade policies. Review of World Economics, 148(1), 209-233.
- Brooks, C., & Persand, G. (2001). The trading profitability of forecasts of the gilt–equity yield ratio. International journal of forecasting, 17(1), 11-29.
- Cai, J. (1994). A Markov model of switching-regime ARCH. Journal of Business & Economic Statistics, 12(3), 309- 316.
- Caporale, G. M., & Zekokh, T. (2019). Modelling volatility of cryptocurrencies using Markov-Switching GARCH models. Research in International Business and Finance, 48, 143-155.
- Cifter, A. (2013). Forecasting electricity price volatility with the Markov-switching GARCH model: Evidence from the Nordic electric power market. Electric Power Systems Research, 102, 61-67.
- De la Torre-Torres, O.V.; Galeana-Figueroa, E.; Álvarez-García, J. (2019). A test of using markov-switching GARCH models in oil and natural gas trading. Energies, 13, 129.
- De la Torre-Torres, D., Oscar, V., Aguilasocho-Montoya, D., Álvarez-García, J., & Simonetti, B. (2020). Using Markov-switching models with Markov chain Monte Carlo inference methods in agricultural commodities trading. Soft Computing, 24(18), 13823-13836.
- Demeke, M., Spinelli, A., Croce, S., Pernechele, V., Stefanelli, E., Jafari, A., ... & Roux, C. (2014). Food and agriculture policy decisions: trends, emerging issues and policy alignments since the 2007/08 food security crisis. Food and Agriculture Organization of the United Nations (FAO).
- Dueker, M. J. (1997). Markov switching in GARCH processes and mean-reverting stock-market volatility. Journal of Business & Economic Statistics, 15(1), 26-34.
- Egelkraut, T. M., & Garcia, P. (2006). Intermediate volatility forecasts using implied forward volatility: The performance of selected agricultural commodity options. Journal of Agricultural and Resource Economics, 508-528.
- Elder, J., & Jin, H. J. (2007). Long memory in commodity futures volatility: A wavelet perspective. Journal of Futures Markets: Futures, Options, and Other Derivative Products, 27(5), 411-437.
- Enders, W. (2015). Applied econometric time series fourth edition. New York (US): University of Alabama.
- Frömmel, M. (2010). Volatility regimes in Central and Eastern European countries’ exchange rates. Czech Journal of Economics and Finance, 60(1), 2–21.
- Glosten, L. R., Jagannathan, R., & Runkle, D. E. (1993). On the relation between the expected value and the volatility of the nominal excess return on stocks. The journal of finance, 48(5), 1779-1801.
- Gray, S. F. (1996). Modeling the conditional distribution of interest rates as a regime-switching process. Journal of Financial Economics, 42(1), 27-62.
- Haas, M., Mittnik, S., & Paolella, M. S. (2004). A new approach to Markov-switching GARCH models. Journal of financial Econometrics, 2(4), 493-530.
- Hamida, H. B. H., & Scalera, F. (2019). Threshold Mean Reversion and Regime Changes of Cryptocurrencies using SETAR-MSGARCH Models. International Journal of Academic Research in Accounting, Finance and Management Sciences, 9(3), 221-229.
- Hamilton, J. D., & Susmel, R. (1994). Autoregressive conditional heteroskedasticity and changes in regime. Journal of econometrics, 64(1-2), 307-333.
- Hauptmann, J., Hoppenkamps, A., Min, A., Ramsauer, F., & Zagst, R. (2014). Forecasting market turbulence using regime-switching models. Financial Markets and Portfolio Management, 28(2), 139-164.
- Hu, L., & Shin, Y. (2008). Optimal test for Markov switching GARCH models. Studies in Nonlinear Dynamics & Econometrics, 12(3).
- Klaassen, F. (2002). Improving GARCH volatility forecasts with regime-switching GARCH. In Advances in Markov-switching models (pp. 223-254). Physica, Heidelberg.
- Korkpoe, C. H., & Howard, N. (2019). Volatility model choice for sub-Saharan frontier equity markets-A Markov regime switching Bayesian approach. EMAJ: Emerging Markets Journal, 9(1), 69-79.
- Kristjanpoller, W., & Michell, K. (2018). A stock market risk forecasting model through integration of switching regime, ANFIS and GARCH techniques. Applied soft computing, 67, 106-116.
- Lamoureux, C. G., & Lastrapes, W. D. (1990). Persistence in variance, structural change, and the GARCH model. Journal of Business & Economic Statistics, 8(2), 225-234.
- Li, N., Ker, A., Sam, A. G., & Aradhyula, S. (2017). Modeling regime dependent agricultural commodity price volatilities. Agricultural economics, 48(6), 683-691.
- Lolea, I. C., & Vilcu, L. C. (2018, May). Measures of volatility for the Romanian Stock Exchange: a regime switching approach. In Proceedings of the International Conference on Business Excellence (Vol. 12, No. 1, pp. 544-556).
- Marcucci, J. (2005). Forecasting stock market volatility with regime-switching GARCH models. Studies in Nonlinear Dynamics & Econometrics, 9(4).
- Mason, N. M., & Myers, R. J. (2013). The effects of the Food Reserve Agency on maize market prices in Zambia. Agricultural Economics, 44(2), 203-216.
- Moore, T., & Wang, P. (2007). Volatility in stock returns for new EU member states: Markov regime switching model. International Review of Financial Analysis, 16(3), 282-292.
- Naeem, M., Tiwari, A. K., Mubashra, S., & Shahbaz, M. (2019). Modeling volatility of precious metals markets by using regime-switching GARCH models. Resources Policy, 64, 101497.
- Nelson, D. B. (1991). Conditional heteroskedasticity in asset returns: A new approach. Econometrica: Journal of the econometric society, 347-370.
- Ng, S., & Perron, P. (2001). Lag length selection and the construction of unit root tests with good size and power. Econometrica, 69(6), 1519-1554.
- Pyo, D. J. (2021). The COVID-19 and stock return volatility: Evidence from South Korea. East Asian Economic Review, 25(2), 205-230.
- Romero-Aguilar, R. S. (2015). Essays on the world food crisis: A quantitative economics assessment of policy options. The Ohio State University.
- Sema,G., Konte, M. A., & Diongue, A. K. (2021). Forecasting Value-at-Risk using Markov Regime-Switching asymmetric GARCH model with Stable distribution in the context of the COVID-19 pandemic. African Journal of Applied Statistics, 8(1), 1049-1071.
- Triantafyllou, A., Dotsis, G., & Sarris, A. H. (2015). Volatility forecasting and time varying variance risk premiums in grains commodity markets. Journal of Agricultural Economics, 66(2), 329-357.
- Visković, J., Arnerić, J., & Rozga, A. (2014). Volatility switching between two regimes. International Journal of Economics and Management Engineering, 8(3), 699-703.
- Wang, P., & Theobald, M. (2008). Regime-switching volatility of six East Asian emerging markets. Research in International Business and Finance, 22(3), 267-283.
- World Bank Group. 2022. Commodity Markets Outlook: Pandemic, war, recession: Drivers of aluminum and copper prices, October 2022. World Bank, Washington, DC.
- Zakoian, J.M., Threshold Heteroscedastic Models, Journal of Economic Dynamics and Control, 18, 1994, 931-955.
- Zhang, Y. J., Yao, T., He, L. Y., & Ripple, R. (2019). Volatility forecasting of crude oil market: Can the regime switching GARCH model beat the single-regime GARCH models?. International Review of Economics & Finance, 59, 302-317.
APA | Özer H, YARBAŞI İ (2023). Tahıl Emtia Fiyat Oynaklığının Markov Değişim Asimetrik Garch Modelleriyle İncelenmesi. , 500 - 513. 10.20491/isarder.2023.1600 |
Chicago | Özer Hüseyin,YARBAŞI İkram Yusuf Tahıl Emtia Fiyat Oynaklığının Markov Değişim Asimetrik Garch Modelleriyle İncelenmesi. (2023): 500 - 513. 10.20491/isarder.2023.1600 |
MLA | Özer Hüseyin,YARBAŞI İkram Yusuf Tahıl Emtia Fiyat Oynaklığının Markov Değişim Asimetrik Garch Modelleriyle İncelenmesi. , 2023, ss.500 - 513. 10.20491/isarder.2023.1600 |
AMA | Özer H,YARBAŞI İ Tahıl Emtia Fiyat Oynaklığının Markov Değişim Asimetrik Garch Modelleriyle İncelenmesi. . 2023; 500 - 513. 10.20491/isarder.2023.1600 |
Vancouver | Özer H,YARBAŞI İ Tahıl Emtia Fiyat Oynaklığının Markov Değişim Asimetrik Garch Modelleriyle İncelenmesi. . 2023; 500 - 513. 10.20491/isarder.2023.1600 |
IEEE | Özer H,YARBAŞI İ "Tahıl Emtia Fiyat Oynaklığının Markov Değişim Asimetrik Garch Modelleriyle İncelenmesi." , ss.500 - 513, 2023. 10.20491/isarder.2023.1600 |
ISNAD | Özer, Hüseyin - YARBAŞI, İkram Yusuf. "Tahıl Emtia Fiyat Oynaklığının Markov Değişim Asimetrik Garch Modelleriyle İncelenmesi". (2023), 500-513. https://doi.org/10.20491/isarder.2023.1600 |
APA | Özer H, YARBAŞI İ (2023). Tahıl Emtia Fiyat Oynaklığının Markov Değişim Asimetrik Garch Modelleriyle İncelenmesi. İşletme Araştırmaları Dergisi, 15(1), 500 - 513. 10.20491/isarder.2023.1600 |
Chicago | Özer Hüseyin,YARBAŞI İkram Yusuf Tahıl Emtia Fiyat Oynaklığının Markov Değişim Asimetrik Garch Modelleriyle İncelenmesi. İşletme Araştırmaları Dergisi 15, no.1 (2023): 500 - 513. 10.20491/isarder.2023.1600 |
MLA | Özer Hüseyin,YARBAŞI İkram Yusuf Tahıl Emtia Fiyat Oynaklığının Markov Değişim Asimetrik Garch Modelleriyle İncelenmesi. İşletme Araştırmaları Dergisi, vol.15, no.1, 2023, ss.500 - 513. 10.20491/isarder.2023.1600 |
AMA | Özer H,YARBAŞI İ Tahıl Emtia Fiyat Oynaklığının Markov Değişim Asimetrik Garch Modelleriyle İncelenmesi. İşletme Araştırmaları Dergisi. 2023; 15(1): 500 - 513. 10.20491/isarder.2023.1600 |
Vancouver | Özer H,YARBAŞI İ Tahıl Emtia Fiyat Oynaklığının Markov Değişim Asimetrik Garch Modelleriyle İncelenmesi. İşletme Araştırmaları Dergisi. 2023; 15(1): 500 - 513. 10.20491/isarder.2023.1600 |
IEEE | Özer H,YARBAŞI İ "Tahıl Emtia Fiyat Oynaklığının Markov Değişim Asimetrik Garch Modelleriyle İncelenmesi." İşletme Araştırmaları Dergisi, 15, ss.500 - 513, 2023. 10.20491/isarder.2023.1600 |
ISNAD | Özer, Hüseyin - YARBAŞI, İkram Yusuf. "Tahıl Emtia Fiyat Oynaklığının Markov Değişim Asimetrik Garch Modelleriyle İncelenmesi". İşletme Araştırmaları Dergisi 15/1 (2023), 500-513. https://doi.org/10.20491/isarder.2023.1600 |