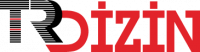
Prediction of COVID-19 Based on Genomic Biomarkers of Metagenomic Next-Generation Sequencing Data Using Artificial Intelligence Technology
Yıl: 2022 Cilt: 44 Sayı: 6 Sayfa Aralığı: 544 - 548 Metin Dili: İngilizce DOI: 10.14744/etd.2022.00868 İndeks Tarihi: 20-05-2023
Prediction of COVID-19 Based on Genomic Biomarkers of Metagenomic Next-Generation Sequencing Data Using Artificial Intelligence Technology
Öz: Objective: The primary aim of this study was to use metagenomic next-generation sequencing (mNGS) data to identify coronavirus 2019 (COVID-19)-related biomarker genes and to construct a machine learning model that could successfully differentiate patients with COVID-19 from healthy controls. Materials and Methods: The mNGS dataset used in the study demonstrated expression of 15,979 genes in the upper air- way in 234 patients who were COVID-19 negative and COVID-19 positive. The Boruta method was used to select qualitative biomarker genes associated with COVID-19. Random forest (RF), gradient boosting tree (GBT), and multi-layer perceptron (MLP) models were used to predict COVID-19 based on the selected biomarker genes. Results: The MLP (0.936) model outperformed the GBT (0.851), and RF (0.809) models in predicting COVID-19. The three most important biomarker candidate genes associated with COVID-19 were IFI27, TPTI, and FAM83A. Conclusion: The proposed model (MLP) was able to predict COVID-19 successfully. The results showed that the generated model and selected biomarker candidate genes can be used as diagnostic models for clinical testing or potential therapeutic targets and vaccine design.
Anahtar Kelime: Belge Türü: Makale Makale Türü: Araştırma Makalesi Erişim Türü: Erişime Açık
- 1. Sahin TT, Akbulut S, Yilmaz S. COVID-19 pandemic: Its impact on liver disease and liver transplantation. World J Gastroenterol 2020; 26(22): 2987–99.
- 2. Myoung J. Two years of COVID-19 pandemic: where are we now?. J Microbiol 2022; 60(3): 235–7.
- 3. Huang C, Wang Y, Li X, Ren L, Zhao J, Hu Y, et al. Clinical features of patients infected with 2019 novel coronavirus in Wuhan, China. The lancet 2020; 395(10223): 497–506.
- 4. Yan L, Zhang HT, Goncalves J, Xiao Y, Wang M, Guo Y, et al. An in- terpretable mortality prediction model for COVID-19 patients. Nature machine intelligence 2020; 2(5): 283–8.
- 5. Yagin FH, Güldoğan E, Ucuzal H, Colak C. A computer-assisted di- agnosis tool for classifying COVID-19 based on Chest X-Ray images. Konuralp Med J 2021; 13(S1): 438–45.
- 6. Arentz M, Yim E, Klaff L, Lokhandwala S, Riedo FX, Chong M, et al. Characteristics and outcomes of 21 critically ill patients with COVID-19 in Washington State. JAMA 2020; 323(16): 1612–4.
- 7. Shi F, Wang J, Shi J, Wu Z, Wang Q, Tang Z, et al. Review of artificial intelligence techniques in imaging data acquisition, segmentation, and diagnosis for COVID-19. IEEE Rev Biomed Eng 2021; 14: 4–15.
- 8. Hoque MN, Rahman MS, Ahmed R, Hossain MS, Islam MS, Islam T, et al. Diversity and genomic determinants of the microbiomes associ- ated with COVID-19 and non-COVID respiratory diseases. Gene Rep 2021; 23: 101200.
- 9. Zhang Y, Pan Y, Zhao X, Shi W, Chen Z, Zhang S, et al. Genomic characterization of SARS-CoV-2 identified in a reemerging COVID-19 outbreak in Beijing’s Xinfadi market in 2020. Biosaf Health 2020; 2(4): 202–5.
- 10. Ding W, Nayak J, Swapnarekha H, Abraham A, Naik B, Pelusi D. Fusion of intelligent learning for COVID-19: A state-of-the-art re- view and analysis on real medical data. Neurocomputing 2021; 457: 40–66.
- 11. Mostafa HH, Fissel JA, Fanelli B, Bergman Y, Gniazdowski V, Dadlani M, et al. metagenomic next-generation sequencing of nasopharyngeal specimens collected from confirmed and suspect COVID-19 patients. mBio 2020; 11(6): e01969–20.
- 12. Perçin İ, Yağin FH, Güldoğan E, Yoloğlu S. ARM: An interactive web software for association rules mining and an application in medicine. 2019 International Artificial Intelligence and Data Processing Sympo- sium (IDAP). IEEE; 2019. pp.1–5.
- 13. Bellini V, Valente M, Bertorelli G, Pifferi B, Craca M, Mordonini M, et al. Machine learning in perioperative medicine: a systematic review. J Anesthesia, Analgesia and Critical Care 2022; 2(1): 1–13.
- 14. Yagin FH, Yagin B, Arslan AK, Colak C. Comparison of performances of associative classification methods for cervical cancer prediction: Ob- servational study. Turkiye Klinikleri J Biostatistics 2021; 13(3): 266– 72.
- 15. Shiri I, Salimi Y, Pakbin M, Hajianfar G, Avval AH, Sanaat A, et al. COVID-19 prognostic modeling using CT radiomic features and ma- chine learning algorithms: Analysis of a multi-institutional dataset of 14,339 patients. Comput Biol Med 2022; 145: 105467.
- 16. Abubaker Bagabir S, Ibrahim NK, Abubaker Bagabir H, Hashem Ateeq R. Covid-19 and Artificial Intelligence: Genome sequencing, drug de- velopment and vaccine discovery. J Infect Public Health 2022; 15(2): 289–96.
- 17. Suvarna K, Biswas D, Pai MGJ, Acharjee A, Bankar R, Palanivel V, et al. Proteomics and machine learning approaches reveal a set of prog- nostic markers for COVID-19 severity with drug repurposing potential. Front Physiol 2021; 12: 652799.
- 18. Mick E, Kamm J, Pisco AO, Ratnasiri K, Babik JM, Castañeda Get al. Upper airway gene expression reveals suppressed immune responses to SARS-CoV-2 compared with other respiratory viruses. Nat Com- mun 2020; 11(1): 5854.
- 19. Li Z, Guo W, Ding S, Feng K, Lu L, Huang T, et al. Detecting blood methylation signatures in response to childhood cancer radiotherapy via machine learning methods. Biology (Basel) 2022; 11(4): 607.
- 20. He S, Wu J, Wang D, He X. Predictive modeling of groundwater ni- trate pollution and evaluating its main impact factors using random forest. Chemosphere 2022; 290: 133388.
- 21. Palimkar P, Shaw RN, Ghosh A. Machine learning technique to prog- nosis diabetes disease: random forest classifier approach. Advanced Computing and Intelligent Technologies. Springer; 2022.p.219–44.
- 22. Larestani A, Mousavi SP, Hadavimoghaddam F, Hemmati-Sarapardeh A. Predicting formation damage of oil fields due to mineral scaling during water-flooding operations: Gradient boosting decision tree and cascade-forward back-propagation network. J Petroleum Scien Engi- neer 2022; 208(A): 109315.
- 23. Kruse R, Mostaghim S, Borgelt C, Braune C, Steinbrecher M. Multi- layer perceptrons. Computational intelligence. Springer; 2022.p.53– 124.
- 24. Xu Y, Li F, Asgari A. Prediction and optimization of heating and cool- ing loads in a residential building based on multi-layer perceptron neu- ral network and different optimization algorithms. Energy 2022; 240: 122692.
- 25. Cohen J. The world is round (p<. 05). American Psychologist 1994; 49(12): 997–1003.
- 26. Shojaei M, Shamshirian A, Monkman J, Grice L, Tran M, Tan CW, et al. IFI27 transcription is an early predictor for COVID-19 out- comes; a multi-cohort observational study. medRxiv 2021. Avail- able from: URL: https://www.medrxiv.org/content/10.1101/ 2021.10.29.21265555v1.
- 27. Hasankhani A, Bahrami A, Sheybani N, Aria B, Hemati B, Fatehi F, et al. Differential co-expression network analysis reveals key hub-high traffic genes as potential therapeutic targets for COVID-19 pandemic. Front Immunol 2021; 12: 789317.
- 28. Zhang YH, Li H, Zeng T, Chen L, Li Z, Huang T, et al. Identifying transcriptomic signatures and rules for SARS-CoV-2 infection. Front Cell Dev Biol 2021; 8: 627302.
APA | Akbulut S, YAĞIN F, ÇOLAK C (2022). Prediction of COVID-19 Based on Genomic Biomarkers of Metagenomic Next-Generation Sequencing Data Using Artificial Intelligence Technology. , 544 - 548. 10.14744/etd.2022.00868 |
Chicago | Akbulut Sami,YAĞIN Fatma Hilal,ÇOLAK Cemil Prediction of COVID-19 Based on Genomic Biomarkers of Metagenomic Next-Generation Sequencing Data Using Artificial Intelligence Technology. (2022): 544 - 548. 10.14744/etd.2022.00868 |
MLA | Akbulut Sami,YAĞIN Fatma Hilal,ÇOLAK Cemil Prediction of COVID-19 Based on Genomic Biomarkers of Metagenomic Next-Generation Sequencing Data Using Artificial Intelligence Technology. , 2022, ss.544 - 548. 10.14744/etd.2022.00868 |
AMA | Akbulut S,YAĞIN F,ÇOLAK C Prediction of COVID-19 Based on Genomic Biomarkers of Metagenomic Next-Generation Sequencing Data Using Artificial Intelligence Technology. . 2022; 544 - 548. 10.14744/etd.2022.00868 |
Vancouver | Akbulut S,YAĞIN F,ÇOLAK C Prediction of COVID-19 Based on Genomic Biomarkers of Metagenomic Next-Generation Sequencing Data Using Artificial Intelligence Technology. . 2022; 544 - 548. 10.14744/etd.2022.00868 |
IEEE | Akbulut S,YAĞIN F,ÇOLAK C "Prediction of COVID-19 Based on Genomic Biomarkers of Metagenomic Next-Generation Sequencing Data Using Artificial Intelligence Technology." , ss.544 - 548, 2022. 10.14744/etd.2022.00868 |
ISNAD | Akbulut, Sami vd. "Prediction of COVID-19 Based on Genomic Biomarkers of Metagenomic Next-Generation Sequencing Data Using Artificial Intelligence Technology". (2022), 544-548. https://doi.org/10.14744/etd.2022.00868 |
APA | Akbulut S, YAĞIN F, ÇOLAK C (2022). Prediction of COVID-19 Based on Genomic Biomarkers of Metagenomic Next-Generation Sequencing Data Using Artificial Intelligence Technology. Erciyes Medical Journal, 44(6), 544 - 548. 10.14744/etd.2022.00868 |
Chicago | Akbulut Sami,YAĞIN Fatma Hilal,ÇOLAK Cemil Prediction of COVID-19 Based on Genomic Biomarkers of Metagenomic Next-Generation Sequencing Data Using Artificial Intelligence Technology. Erciyes Medical Journal 44, no.6 (2022): 544 - 548. 10.14744/etd.2022.00868 |
MLA | Akbulut Sami,YAĞIN Fatma Hilal,ÇOLAK Cemil Prediction of COVID-19 Based on Genomic Biomarkers of Metagenomic Next-Generation Sequencing Data Using Artificial Intelligence Technology. Erciyes Medical Journal, vol.44, no.6, 2022, ss.544 - 548. 10.14744/etd.2022.00868 |
AMA | Akbulut S,YAĞIN F,ÇOLAK C Prediction of COVID-19 Based on Genomic Biomarkers of Metagenomic Next-Generation Sequencing Data Using Artificial Intelligence Technology. Erciyes Medical Journal. 2022; 44(6): 544 - 548. 10.14744/etd.2022.00868 |
Vancouver | Akbulut S,YAĞIN F,ÇOLAK C Prediction of COVID-19 Based on Genomic Biomarkers of Metagenomic Next-Generation Sequencing Data Using Artificial Intelligence Technology. Erciyes Medical Journal. 2022; 44(6): 544 - 548. 10.14744/etd.2022.00868 |
IEEE | Akbulut S,YAĞIN F,ÇOLAK C "Prediction of COVID-19 Based on Genomic Biomarkers of Metagenomic Next-Generation Sequencing Data Using Artificial Intelligence Technology." Erciyes Medical Journal, 44, ss.544 - 548, 2022. 10.14744/etd.2022.00868 |
ISNAD | Akbulut, Sami vd. "Prediction of COVID-19 Based on Genomic Biomarkers of Metagenomic Next-Generation Sequencing Data Using Artificial Intelligence Technology". Erciyes Medical Journal 44/6 (2022), 544-548. https://doi.org/10.14744/etd.2022.00868 |