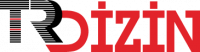
Using the Remote Sensing Method to Simulate the Land Change in the Year 2030
Yıl: 2022 Cilt: 10 Sayı: 12 Sayfa Aralığı: 2453 - 2466 Metin Dili: İngilizce DOI: 10.24925/turjaf.v10i12.2453-2466.5555 İndeks Tarihi: 23-05-2023
Using the Remote Sensing Method to Simulate the Land Change in the Year 2030
Öz: This is study is based with the support of RS-GIS technology on the land use of Samsun Center, as well as the coastal districts of Ilkadım,Atakum,Bafra Plain, through the processing and interpretation of satellite images in the summer months of 2000,2010,2020. Spatial and temporal variability properties of LU/LC were determined using MLC algorithm, controlled classification approach. The predictive values of the LU/LC change that will occur in 2030, calculated with the MLP ANN model based on Machine Learning algorithms and mapped with the QGIS 3.16 program. To determine the accuracy coefficient of the model, 2020 LU/LC simulation performed using the transition potential matrix of 2000 and 2010 LU/LC data. The results of simulation were compared the data of land use land cover with the 2020 to evaluate the accuracy of the simulation model. The model of MLP ANN provided an accuracy of 72% based on the kappa fit index. According to MLP ANN model 2030 results were an increase of 73.33 km² in built up areas, an increase of 56.89 km² in bare areas, and a decrease of 129.66 km² in green areas. It provided a reference basis for future Samsun urban to rural coastline LU planning and management and LU structure optimization. Keywords: Remote Sensing GIS LU/LC Machine Learning MLP ANN
Anahtar Kelime: Belge Türü: Makale Makale Türü: Araştırma Makalesi Erişim Türü: Erişime Açık
- Aburas MM, Ahamad MSS, Omar NQ. 2019. Spatio-temporal simulation and prediction of land-use change using conventional and machine learning models: a review. Environmental monitoring and assessment, 191(4): 1-28.
- Alqadhi S, Mallick J, Balha A, Bindajam A, Singh CK, Hoa PV. 2021. Spatial and decadal prediction of land use/land cover using multi-layer perceptron-neural network (MLP-NN) algorithm for a semi-arid region of Asir, Saudi Arabia. Earth Science Informatics, 14(3): 1547-1562.
- Anand V, Oinam B. 2020. Future land use land cover prediction with special emphasis on urbanization and wetlands. Remote Sensing Letters, 11(3): 225-234.
- Anonim a. (Date of access: 05.06.2021). Provincial and District Areas. Retrieved from https://harita.gov.tr/urun/il-ve-ilce- yuzolcumleri/176.
- Anonim b. (Date of access: 06.06.2021). Samsun Integrated Coastal Areas Management and Planning Project-Spatial Strategy Plan, 208p.
- Anonim c. (Date of access: 02.05.2021). Statistical indicators.Retrieved from https://data.tuik.gov.tr/Bulten/ Index?p=Adrese-Dayali-Nufus-Kayit-Sistemi-Sonuclari- 2020-37210.
- Bağcı H, Bahadır M. 2019. Land Use and Temporal Change in the Kızılırmak Delta (Samsun) (1987-2019). The Journal of Academic Social Science Studies, 34: 295-312.
- Municipality A. 2014). 2015-2019 STRATEGIC PLAN.
- Municipality SB. (Date of access: 27.01.2022). 2020 – 2024 Strategic Plan. Retrieved from https://www.samsun.bel.tr/
- Benediktsson JA, Swain PH, Ersoy OK. 1990. Neural network approaches versus statistical methods in classification of multisource remote sensing data. Vancouver, Canada, July 10-14, 1989) IEEE Transactions on Geoscience and Remote Sensing.
- Blanco-Canqui H, Lal R. 2008. Principles of soil conservation and management: Springer Science & Business Media.
- Bose A, Chowdhury IR. 2020. Monitoring and modeling of spatio-temporal urban expansion and land-use/land-cover change using markov chain model: a case study in Siliguri Metropolitan area, West Bengal, India. Modeling Earth Systems and Environment, 6(4): 2235-2249.
- Bounouh O, Essid H, Farah IR. 2017. Prediction of land use/land cover change methods: A study. Paper presented at the 2017 international conference on advanced technologies for signal and image processing (ATSIP).
- Camacho Olmedo M, Paegelow M, Mas JF. 2013. Interest in intermediate soft-classified maps in land change model validation: suitability versus transition potential. International Journal of Geographical Information Science, 27(12): 2343- 2361.
- Cohen J. 1960. A coefficient of agreement for nominal scales. Educational and psychological measurement, 20(1): 37-46.
- Congedo L. 2016. Semi-automatic classification plugin documentation. Release, 4(0.1): 29.
- Congedo L. 2021. Semi-Automatic Classification Plugin: A Python tool for the download and processing of remote sensing images in QGIS. Journal of Open-Source Software, 6(64): 3172.
- Du J, Fu Q, Fang S, Wu J, He P, Quan Z. 2019. Effects of rapid urbanization on vegetation cover in the metropolises of China over the last four decades. Ecological Indicators, 107: 105458.
- E Silva LP, Xavie, APC, Da Silva RM, Santos CAG. 2020. Modeling land cover change based on an artificial neural network for a semiarid river basin in northeastern Brazil. Global Ecology and Conservation, 21: e00811.
- Fernando HJS, Dimitrova R, Sentic S. 2012. Climate change meets urban environment. In National Security and Human Health Implications of Climate Change (pp. 115-133): Springer.
- Gao J, Li S. 2011. Detecting spatially non-stationery and scale- dependent relationships between urban landscape fragmentation and related factors using Geographically Weighted Regression. Applied Geography, 31(1): 292-302.
- Güler M, Yomralıoğlu T, Reis S. 2007. Using landsat data to determine land use/land cover changes in Samsun, Turkey. Environmental monitoring and assessment, 127(1): 155-167.
- Hakim AMY, Baja S, Rampisela DA, Arif, S. 2021. Modelling land use/land cover changes prediction using multi-layer perceptron neural network (MLPNN): a case study in Makassar City, Indonesia. International Journal of Environmental Studies, 78(2): 301-318.
- Hamad R, Balzter H, Kolo K. 2018. Predicting land use/land cover changes using a CA-Markov model under two different scenarios. Sustainability, 10(10): 3421.
- Islam K, Rahman MF, Jashimuddin M. 2018. Modeling land use change using cellular automata and artificial neural network: the case of Chunati Wildlife Sanctuary, Bangladesh. Ecological Indicators, 88: 439-453.
- Jamali A. 2019. A fit-for-purpose algorithm for environmental monitoring based on maximum likelihood, support vector machine and random forest. Int Arch Photogramm Remote Sens Spatial Inf Sci, 42(3/W7): 25-32.
- Jensen JR. 1986. Introductory digital image processing: a remote sensing perspective. Retrieved from
- Karaçuha Yılmaz E. 2007. Examination of the Coastal Fill Area of Samsun City in terms of Landscape Architecture and Suggestions. In: Ankara University, Department of Landscape Architecture, Master Thesis
- District Government A. 2013. While Changing, Developing Atakum.
- Landis R, Koch GG. 1977. An application of hierarchical kappa- type statistics in the assessment of majority agreement among multiple observers. Biometrics, 363-374.
- Lee SY, Song XY. 2004. Evaluation of the Bayesian and maximum likelihood approaches in analyzing structural equation models with small sample sizes. Multivariate Behavioral Research, 39(4): 653-686.
- Lu Y, Wu P, Ma X, Li X. 2019. Detection and prediction of land use/land cover change using spatiotemporal data fusion and the Cellular Automata–Markov model. Environmental monitoring and assessment, 191(2): 1-19.
- MohanRajan SN, Loganathan A. 2021. Modelling spatial drivers for LU/LC change prediction using hybrid machine learning methods in Javadi Hills, Tamil Nadu, India. Journal of the Indian Society of Remote Sensing, 49(4): 913-934.
- MohanRaja SN, Loganathan A, Manoharan P. 2020. Survey on Land Use/Land Cover (LU/LC) change analysis in remote sensing and GIS environment: Techniques and Challenges. Environmental Science and Pollution Research, 27(24): 29900-29926.
- Mukherjee S, Joshi PK, Mukherjee S, Ghosh A, Garg R, Mukhopadhyay A. 2013. Evaluation of vertical accuracy of open-source Digital Elevation Model (DEM). International Journal of Applied Earth Observation and Geoinformation, 21: 205-217.
- Ozturk D. 2015. Urban growth simulation of Atakum (Samsun, Turkey) using cellular automata-Markov chain and multi- layer perceptron-Markov chain models. Remote Sensing, 7(5): 5918-5950.
- Ozturk D. 2017. Assessment of urban sprawl using Shannon’s entropy and fractal analysis: a case study of Atakum, Ilkadim and Canik (Samsun, Turkey). Journal of environmental engineering and landscape management, 25(3): 264-276.
- Öztürk D, Gündüz U. 2020. Determination of Temporal Changes in Urban Tissue Morphology in Samsun Districts by Fractal Analysis. Dokuz Eylul University Faculty of Engineering Journal of Science and Engineering, 22(64): 81-95.
- Paola JD, Schowengerdt RA. 1995. A review and analysis of backpropagation neural networks for classification of remotely-sensed multi-spectral imagery. International Journal of Remote Sensing, 16(16): 3033-3058.
- Pektezel H. 2015. City and Water Resources. In (pp. 107-132).
- Reddy CS, Singh S, Dadhwal V, Jha C, Rao NR, Diwakar P. 2017. Predictive modelling of the spatial pattern of past and future forest cover changes in India. Journal of Earth System Science, 126(1): 1-16.
- Saleem A, Corner R, Awange J. 2018. On the possibility of using CORONA and Landsat data for evaluating and mapping long- term LULC: Case study of Iraqi Kurdistan. Applied Geography, 90: 145-154.
- Sesli FA. 2010. Mapping and monitoring temporal changes for coastline and coastal area by using aerial data images and digital photogrammetry: A case study from Samsun, Turkey. International Journal of Physical Sciences, 5(10): 1567-1575.
- Turner BL, Skole D, Sanderson S, Fischer G, Fresco L, Leemans R. 1995. Land-use and land-cover change: science/research plan. Global Change Report (Sweden), 43: 669–679.
- Ullah S, Tahir AA, Akbar TA, Hassan QK, Dewan A, Khan AJ. 2019. Remote sensing-based quantification of the relationships between land use land cover changes and surface temperature over the Lower Himalayan Region. Sustainability, 11(19): 5492.
- University Y. 2020. (Erişim tarihi: 01.08.2020). Center for Earth Observation, Landsat. Retrieved from https://yceo.yale.edu/how-convert-landsat-dns-albedo,
- USGS. 2020. (Erişim tarihi: 09.04.2020). Landsat Missions. Retrieved from https://www.usgs.gov/land- resources/nli/landsat
- Uzun A. 1998. Our Black Sea coasts in terms of coastal law. Jeomorfoloji Dergisi, 21: 60-64.
- Wu J. 2014. Urban ecology and sustainability: The state-of-the- science and future directions. Landscape and urban planning, 125: 209-221.
- Yao Z, Wang B, Huang J, Zhang Y, Yang J, Deng R. 2021. Analysis of Land Use Changes and Driving Forces in the Yanhe River Basin from 1980 to 2015. Journal of Sensors, 2021.
- Yirsaw E, Wu W, Shi X, Temesgen H, Bekele B. 2017. Land use/land cover change modeling and the prediction of subsequent changes in ecosystem service values in a coastal area of China, the Su-Xi-Chang Region. Sustainability, 9(7): 1204.
- Zhou W, Pickett ST, Cadenasso ML. 2017. Shifting concepts of urban spatial heterogeneity and their implications for sustainability. Landscape ecology, 32(1): 15-30.
APA | Cevik Degerli B, cetin m (2022). Using the Remote Sensing Method to Simulate the Land Change in the Year 2030. , 2453 - 2466. 10.24925/turjaf.v10i12.2453-2466.5555 |
Chicago | Cevik Degerli Burcu,cetin mehmet Using the Remote Sensing Method to Simulate the Land Change in the Year 2030. (2022): 2453 - 2466. 10.24925/turjaf.v10i12.2453-2466.5555 |
MLA | Cevik Degerli Burcu,cetin mehmet Using the Remote Sensing Method to Simulate the Land Change in the Year 2030. , 2022, ss.2453 - 2466. 10.24925/turjaf.v10i12.2453-2466.5555 |
AMA | Cevik Degerli B,cetin m Using the Remote Sensing Method to Simulate the Land Change in the Year 2030. . 2022; 2453 - 2466. 10.24925/turjaf.v10i12.2453-2466.5555 |
Vancouver | Cevik Degerli B,cetin m Using the Remote Sensing Method to Simulate the Land Change in the Year 2030. . 2022; 2453 - 2466. 10.24925/turjaf.v10i12.2453-2466.5555 |
IEEE | Cevik Degerli B,cetin m "Using the Remote Sensing Method to Simulate the Land Change in the Year 2030." , ss.2453 - 2466, 2022. 10.24925/turjaf.v10i12.2453-2466.5555 |
ISNAD | Cevik Degerli, Burcu - cetin, mehmet. "Using the Remote Sensing Method to Simulate the Land Change in the Year 2030". (2022), 2453-2466. https://doi.org/10.24925/turjaf.v10i12.2453-2466.5555 |
APA | Cevik Degerli B, cetin m (2022). Using the Remote Sensing Method to Simulate the Land Change in the Year 2030. Türk Tarım - Gıda Bilim ve Teknoloji dergisi, 10(12), 2453 - 2466. 10.24925/turjaf.v10i12.2453-2466.5555 |
Chicago | Cevik Degerli Burcu,cetin mehmet Using the Remote Sensing Method to Simulate the Land Change in the Year 2030. Türk Tarım - Gıda Bilim ve Teknoloji dergisi 10, no.12 (2022): 2453 - 2466. 10.24925/turjaf.v10i12.2453-2466.5555 |
MLA | Cevik Degerli Burcu,cetin mehmet Using the Remote Sensing Method to Simulate the Land Change in the Year 2030. Türk Tarım - Gıda Bilim ve Teknoloji dergisi, vol.10, no.12, 2022, ss.2453 - 2466. 10.24925/turjaf.v10i12.2453-2466.5555 |
AMA | Cevik Degerli B,cetin m Using the Remote Sensing Method to Simulate the Land Change in the Year 2030. Türk Tarım - Gıda Bilim ve Teknoloji dergisi. 2022; 10(12): 2453 - 2466. 10.24925/turjaf.v10i12.2453-2466.5555 |
Vancouver | Cevik Degerli B,cetin m Using the Remote Sensing Method to Simulate the Land Change in the Year 2030. Türk Tarım - Gıda Bilim ve Teknoloji dergisi. 2022; 10(12): 2453 - 2466. 10.24925/turjaf.v10i12.2453-2466.5555 |
IEEE | Cevik Degerli B,cetin m "Using the Remote Sensing Method to Simulate the Land Change in the Year 2030." Türk Tarım - Gıda Bilim ve Teknoloji dergisi, 10, ss.2453 - 2466, 2022. 10.24925/turjaf.v10i12.2453-2466.5555 |
ISNAD | Cevik Degerli, Burcu - cetin, mehmet. "Using the Remote Sensing Method to Simulate the Land Change in the Year 2030". Türk Tarım - Gıda Bilim ve Teknoloji dergisi 10/12 (2022), 2453-2466. https://doi.org/10.24925/turjaf.v10i12.2453-2466.5555 |