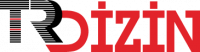
Covid-19 Verileri için Bayes Ağlari ile Makine Öğrenmesi
Yıl: 2023 Cilt: 9 Sayı: 1 Sayfa Aralığı: 127 - 144 Metin Dili: Türkçe DOI: 10.28979/jarnas.1162578 İndeks Tarihi: 24-05-2023
Covid-19 Verileri için Bayes Ağlari ile Makine Öğrenmesi
Öz: Öz − Covid-19 pandemisi, 17 Kasım 2019 tarihinde Çin'in Vuhan Eyaleti'nde ilk defa görülmüştür. Küresel pandemi ilk başta Vuhan’daki deniz mahsülleri ve hayvan satışı yapılan yerlerde görülmüştür. Sonra insanlar arasında da yayılışını devam ettirerek ilk olarak Vuhan ve Çin’in diğer eyaletindeki bölgelere ve dünya üzerinde diğer ülkelere de yayılmıştır. 14 Ağustos 2022 tarihi itibariyle dünyada 590.624.000 vaka meydana gelmiştir ve 6.431.291 hasta ölmüştür. Ülkemizde ve dünya genelinde Covid-19 pandemisinin etkilerini gösteren birçok araştırma ve analiz çalışmaları yapılmıştır. Bu çalışmada dünya genelinde 104 ülkeden oluşan 215.968 adet dünya çapında meydana gelen vaka analiz edilmiştir ve Bayes Ağları (Bayesian Networks) ile makine öğrenimi tekniği kullanılarak hastalar sınıflandırılmaya çalışılmış ve dokuz adet değişkenle Covid-19 virüsüne yakalanan hastaların hayatta kalıp kalmayacağını araştırılmıştır. Böylelikle hangi hastaya öncelik verip tedavi edilmesi gerektiği veya gözlem altında tutulması gerektiği belirlenecektir. Sonuç olarak bu çalışmayla dünya genelindeki Covid-19 pandemisinden kaynaklı ölüm oranlarının düşürülmesi hedeflenmektedir.
Anahtar Kelime: Machine Learning with Bayesian Networks for Covid-19 Data
Öz: Abstract − The Covid-19 pandemic emerged on November 17, 2019, in Wuhan Province of China. The outbreak was initially detected in those found in the seafood and animal market in this region. Later, it spread from person to person and spread to other cities in Hubei province, especially in Wuhan, other provinces of China, and other world countries. Until Aug 14, 2022, 590.624.000 cases occurred globally and 6.266.278 patients died from Covid-19. Many research and analysis studies have been conducted in our country and around the world showing the effects of the Covid-19 pandemic. In this study, 215,968 worldwide cases from 104 countries around the world were analysed and the patients were tried to be classified using Bayesian Networks and machine learning techniques. It was investigated whether the patients who caught the Covid-19 virus would survive using nine variables. In this way, it will be determined which patient should be given priority and treated or kept under observation. Thus, this study aims to reduce the death rates due to the Covid-19 pandemic worldwide.
Anahtar Kelime: Belge Türü: Makale Makale Türü: Araştırma Makalesi Erişim Türü: Erişime Açık
- Adiga, A., Dubhashi, D., Lewis, B. et al (2020). Mathematical Models for COVID-19 Pandemic: A Compara tive Analysis. J Indian Inst Sci 100, 793–807. Doi:https://doi.org/10.1007/s41745-020-00200-6
- Atılgan K.Y. & Ersel D., (2017). Bayesci ağ yapısının öğrenilmesinde grafiksel bir yaklaşım, Journal of Statisticians: Statistics and Actuarial Sciences, 1, 1-10.
- Chen, J. M. (2022). Novel statistics predict the COVID 19 pandemic could terminate in 2022. Journal of Medical Virology, 94(6), 2845-2848.
- Heckerman, D. (2008). A Tutorial on learning with Bayesian networks. Innovations in Bayesian networks, 33- 82. Doi:https://doi.org/10.1007/978-3-540-85066-3_3
- Gemela J. (2001). Financial analysis using Bayesian Networks, Applied Stochastic Models in Business and Industry, 17(1), 57-67. Doi:https://doi.org/10.1002/asmb.422
- Hui D.S. Azhar E.I., Madani, T.A., Ntoumi F., Kock R. Dar O. et al (2020). The continuing COVID-19 epidemic threat of novel coronavirusesto global health — The latest 2019 novel coronavirus outbreak inWuhan, China. International Journal of Infectious Diseases, 91; 264-266. Doi:https://doi.org/10.1016/j.ijid.2020.01.009
- IBM Corp (2016). IBM SPSS Statistics for Windows, Version 23.0. Armonk, New York, U.S.A. Jensen, V. & Nielsen T.D. (2007). Bayesian networks and decision graphs. Vol. 2. New York: Springer. Doi:https://doi.org/10.1007/978-3-540-85066-3_3
- Karacan, H. & Eryılmaz, F. (2021). Covid-19 Detection from Chest X-Ray Images and Hybrid Model Recommendation with Convolutional Neural Networks . Journal of Advanced Research in Natural and Applied Sciences , 7 (4) , 486-503. Doi:https://doi.org/10.28979/jarnas.952700
- Korb, K.B.& Nicholson, A. E. (2011). Bayesian artificial intelligence (Computer science and data analysis series) CRC press. Doi:https://doi.org/10.1201/9780203491294
- Laurìa, E. J. M. & Duchessi, P. J.(2006). A Bayesian Belief Network for IT Implementation Decision Support, Decision Support Systems, 42(3), 738-742, Doi:https://doi.org/10.1016/j.dss.2006.01.003
- Masum, M., Masud, M. A., Adnan, M. I., Shahriar, H., & Kim, S. (2022). Comparative study of a mathematicalepidemic model, statistical modeling, and deep learning for COVID-19 forecasting and management. SocioEconomic Planning Sciences, 80, 101249.
- Maleki, M., Mahmoudi, M. R., Wraith, D., & Pho, K. H. (2020). Time series modelling to forecast the confirmed and recovered cases of COVID-19. Travel medicine and infectious disease, 37, 101742.
- Olmuş, H., & Erbaş, S. (2012). Bayes Ağlarda Kümeleme Metotunu Kullanarak Meme Kanseri Tanısının Modellenmesi. Turkiye Klinikleri Journal of Biostatistics, 4(1),10-19.
- Pearl, J. & Verma, T.S. (1990) Equivalence and Synthesis of Causal Models. Proceedings of the 6th Conference on Uncertainty in Artificial Intelligence, Cambridge, 27-29 July, 220-227.
- Perone, G. (2020) An ARIMA model to forecast the spread and the final size of Covid-2019 epidemic in Italy (first version on SSRN 31 march). SSRN Electron J. Doi:https://doi.org/10.2139/ssrn.3564865
- Spiegelhalter, D.J., Dawid, A.P., Lauritzen, S.L. & Cowell R. G. (1993). Bayesian analysis in expert systems, Statistical Science, 8(3), 219-247. Doi:https://doi.org/10.1214/ss/1177010888
- Scutari, M. (2011). Measures of Variability for Graphical Models. (Yayımlanmamış doktora tezi), Universita degli Studi di Padova, Dipartimento di Scienze Statistiche.
- Şencan, Ş. N. , Şencan, B. , Borazan Çelikbıçak, M. , Arslan, D. , Özkan, E. S. , Gökçen, A. Ş. , Çiftçi, R. B. , Arıkan, İ. , Uğur, B. , Şahin, H. , Coşkun, A. E. , Konşuk Ünlü, H. & Aktaş, S. (2020). Lojistik Büyüme ve Üstel Büyüme Modelleri ile Türkiye’de Covid-19 Modellemesi . Nicel Bilimler Dergisi , 2 (1) , 1-1. https://dergipark.org.tr/tr/pub/nicel/issue/55149/748068
- Tang, S., Wang, C., Nie, J., Kumar, N., Zhang, Y., Xiong, Z. & Barnawi, A. (2021). EDL-COVID: Ensemble Deep Learning for COVID-19 Case Detection From Chest X-Ray Images. IEEE Transactions on Industrial Informatics, 17, 6539-6549. doi: 10.1109/TII.2021.3057683
- Wendler, T. & Sören G. (2016). Data Mining with SPSS Modeler Theory, Exercises and Solutions, Cham Springer International Publishing, Springer. Doi:https://doi.org/10.1007/978-3-319-28709-6
- Yılmaz, H. C. (2022). Covid-19 Verileri İçin Bayes Ağları İle Makine Öğrenmesi, (Yayınlanmamış yüksek lisans tezi), Hacettepe Üniversitesi, Fen Bilimleri Enstitüsü, Ankara, Türkiye.
- Zhou Y, Yang Y, Huang J, Jiang S. & Du L (2019). Advances in MERS-CoV Vaccines and Therapeutics Based on the Receptor-Binding Domain. Viruses. 11(1), 60. https://doi.org/10.3390/v11010060
- Zeroual A., Harrou F., Dairi A. & Sun Y. (2020). Deep Learning Methods for Forecasting Covid-19 Time- Series Data: A comparative study. Chaos, Solit Fractals, 140:110121. Doi:https://doi.org/10.1016/j.chaos.2020.110121
APA | Aktas S, YILMAZ H (2023). Covid-19 Verileri için Bayes Ağlari ile Makine Öğrenmesi. , 127 - 144. 10.28979/jarnas.1162578 |
Chicago | Aktas Serpil,YILMAZ HÜSEYİN CAN Covid-19 Verileri için Bayes Ağlari ile Makine Öğrenmesi. (2023): 127 - 144. 10.28979/jarnas.1162578 |
MLA | Aktas Serpil,YILMAZ HÜSEYİN CAN Covid-19 Verileri için Bayes Ağlari ile Makine Öğrenmesi. , 2023, ss.127 - 144. 10.28979/jarnas.1162578 |
AMA | Aktas S,YILMAZ H Covid-19 Verileri için Bayes Ağlari ile Makine Öğrenmesi. . 2023; 127 - 144. 10.28979/jarnas.1162578 |
Vancouver | Aktas S,YILMAZ H Covid-19 Verileri için Bayes Ağlari ile Makine Öğrenmesi. . 2023; 127 - 144. 10.28979/jarnas.1162578 |
IEEE | Aktas S,YILMAZ H "Covid-19 Verileri için Bayes Ağlari ile Makine Öğrenmesi." , ss.127 - 144, 2023. 10.28979/jarnas.1162578 |
ISNAD | Aktas, Serpil - YILMAZ, HÜSEYİN CAN. "Covid-19 Verileri için Bayes Ağlari ile Makine Öğrenmesi". (2023), 127-144. https://doi.org/10.28979/jarnas.1162578 |
APA | Aktas S, YILMAZ H (2023). Covid-19 Verileri için Bayes Ağlari ile Makine Öğrenmesi. Journal of advanced research in natural and applied sciences (Online), 9(1), 127 - 144. 10.28979/jarnas.1162578 |
Chicago | Aktas Serpil,YILMAZ HÜSEYİN CAN Covid-19 Verileri için Bayes Ağlari ile Makine Öğrenmesi. Journal of advanced research in natural and applied sciences (Online) 9, no.1 (2023): 127 - 144. 10.28979/jarnas.1162578 |
MLA | Aktas Serpil,YILMAZ HÜSEYİN CAN Covid-19 Verileri için Bayes Ağlari ile Makine Öğrenmesi. Journal of advanced research in natural and applied sciences (Online), vol.9, no.1, 2023, ss.127 - 144. 10.28979/jarnas.1162578 |
AMA | Aktas S,YILMAZ H Covid-19 Verileri için Bayes Ağlari ile Makine Öğrenmesi. Journal of advanced research in natural and applied sciences (Online). 2023; 9(1): 127 - 144. 10.28979/jarnas.1162578 |
Vancouver | Aktas S,YILMAZ H Covid-19 Verileri için Bayes Ağlari ile Makine Öğrenmesi. Journal of advanced research in natural and applied sciences (Online). 2023; 9(1): 127 - 144. 10.28979/jarnas.1162578 |
IEEE | Aktas S,YILMAZ H "Covid-19 Verileri için Bayes Ağlari ile Makine Öğrenmesi." Journal of advanced research in natural and applied sciences (Online), 9, ss.127 - 144, 2023. 10.28979/jarnas.1162578 |
ISNAD | Aktas, Serpil - YILMAZ, HÜSEYİN CAN. "Covid-19 Verileri için Bayes Ağlari ile Makine Öğrenmesi". Journal of advanced research in natural and applied sciences (Online) 9/1 (2023), 127-144. https://doi.org/10.28979/jarnas.1162578 |