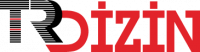
Machine learning-based ovarian cancer prediction with XGboost and stochastic gradient boosting models
Yıl: 2023 Cilt: 12 Sayı: 1 Sayfa Aralığı: 231 - 237 Metin Dili: İngilizce DOI: 10.5455/medscience.2022.09.207 İndeks Tarihi: 29-05-2023
Machine learning-based ovarian cancer prediction with XGboost and stochastic gradient boosting models
Öz: Ovarian cancer is one of the most common types of gynecological malignancies with its high mortality rate, silent and occult tumor growth, late onset of symptoms and diagnosis in advanced stages. Therefore, the need to develop new diagnostic techniques to predict the course of the disease and the prognosis of this malignancy has increased. In this study, ovarian cancer and benign ovarian tumor samples will be classified to create an accurate diagnostic predictive model using the machine learning method XGBoost and Stochastic Gradient Boosting and disease-related risk factors will be determined. This current study considered the open- access ovarian cancer and benign ovarian tumor samples data set. For this purpose, data from 349 patients were included. The data set was divided as 80:20 as a training and test dataset. XGBoost and Stochastic Gradient Boosting were constructed for the classification via five-fold cross-validation. Accuracy, balanced accuracy, sensitivity, specificity, positive predictive value, and negative predictive value performance metrics were evaluated for model performance. Among the performance criteria in the test stage obtained from the XGBoost model that has the best classification result; accuracy, balanced accuracy, sensitivity, specificity, positive predictive value, negative predictive value, and F1 score were obtained as 89.5%, 88.7%, 85.7%, 91.7%, 85.7%, 91.7%, and 85.7%, respectively. According to the variable importance obtained as a result of the model, the variables most associated with the diagnosis were CA72-4, HE4, LYM%, ALB, EO%, BUN, RBC, NEU, and MCV, respectively. The applied machine learning model successfully classified ovarian cancer and created a highly accurate diagnostic prediction model. The results from the study revealed effective parameters that can diagnose ovarian cancer with high accuracy. With the parameters determined as a result of the modeling, the clinician will be able to simplify and facilitate the decision-making process for the diagnosis of ovarian cancer.
Anahtar Kelime: Belge Türü: Makale Makale Türü: Araştırma Makalesi Erişim Türü: Erişime Açık
- 1. Momenimovahed Z, Ghoncheh M, Pakzad R, et al. Incidence and mortality of uterine cancer and relationship with Human Development Index in the world. Cukurova Med J. 2017;42:233-40.
- 2. Bray F, Ferlay J, Soerjomataram I, et al. Global cancer statistics 2018: GLOBOCAN estimates of incidence and mortality worldwide for 36 cancers in 185 countries. CA: a cancer journal for clinicians. 2018;68:394- 424.
- 3. Coburn S, Bray F, Sherman M, Trabert B. International patterns and trends in ovarian cancer incidence, overall and by histologic subtype. International journal of cancer. 2017;140:2451-60.
- 4. Yoneda A, Lendorf ME, Couchman JR, Multhaupt HA. Breast and ovarian cancers: a survey and possible roles for the cell surface heparan sulfate proteoglycans. Journal of Histochemistry & Cytochemistry. 2012;60:9- 21.
- 5. Badgwell D, Bast Jr RC. Early detection of ovarian cancer. Disease markers. 2007;23:397-410.
- 6. Lowe KA, Chia VM, Taylor A, et al. An international assessment of ovarian cancer incidence and mortality. Gynecologic oncology. 2013;130:107-14.
- 7. Chornokur G, Amankwah EK, Schildkraut JM, Phelan CM. Global ovarian cancer health disparities. Gynecologic oncology. 2013;129:258- 64.
- 8. Momenimovahed Z, Tiznobaik A, Taheri S, Salehiniya H. Ovarian cancer in the world: epidemiology and risk factors. International journal of women's health. 2019;11:287-99.
- 9. Lu M, Fan Z, Xu B, et al. Using machine learning to predict ovarian cancer. International Journal of Medical Informatics. 2020;141:104195.
- 10. Polikar R. Ensemble learning. Ensemble machine learning: Springer; 2012. p. 1-34.
- 11. Akman M, Genç Y, Ankarali H. [Random Forests Methods and an Application in Health Science]. Turkiye Klinikleri J Biostat. 2011;3:36.
- 12. Lu M, Fan Z, Xu B, et al. Using machine learning to predict ovarian cancer. International journal of medical informatics. 2020;141:104195.
- 13. Wang J, Li P, Ran R, et al. A short-term photovoltaic power prediction model based on the gradient boost decision tree. Appl Sci. 2018;8:689.
- 14. Dikker J. Boosted tree learning for balanced item recommendation in online retail 2017.
- 15. Salam Patrous Z. Evaluating XGBoost for user classification by using behavioral features extracted from smartphone sensors [Master Thesis]: KTH Royal Institute of Technology; 2018.
- 16. Friedman JH. Stochastic gradient boosting. Computational statistics & data analysis. 2002;38:367-78.
- 17. Lawrence R, Bunn A, Powell S, Zambon M. Classification of remotely sensed imagery using stochastic gradient boosting as a refinement of classification tree analysis. Remote sensing of environment. 2004;90:331- 6.
- 18. Rojas V, Hirshfield KM, Ganesan S, Rodriguez-Rodriguez L. Molecular characterization of epithelial ovarian cancer: implications for diagnosis and treatment. International journal of molecular sciences. 2016;17:2113.
- 19. Bhatt P, Vhora I, Patil S, et al. Role of antibodies in diagnosis and treatment of ovarian cancer: Basic approach and clinical status. Journal of controlled release. 2016;226:148-67.
- 20. Chandra A, Pius C, Nabeel M, et al. Ovarian cancer: Current status and strategies for improving therapeutic outcomes. Cancer medicine. 2019;8:7018-31.
- 21. Zeppernick F, Meinhold-Heerlein I. The new FIGO staging system for ovarian, fallopian tube, and primary peritoneal cancer. Archives of gynecology and obstetrics. 2014;290:839-42.
- 22. Schiavone MB, Herzog TJ, Lewin SN, et al. Natural history and outcome of mucinous carcinoma of the ovary. American journal of obstetrics and gynecology. 2011;205:480. e1-. e8.
- 23. Howlader N, Krapcho M, Miller D, et al. SEER Cancer Statistics Review, 1975-2014, based on November 2016 SEER data submission, posted to the SEER web site. Bethesda, MD, National Cancer Institute. 2017.
- 24. Davenport T, Kalakota R. The potential for artificial intelligence in healthcare. Future healthcare journal. 2019;6:94.
- 25. Yu K-H, Beam AL, Kohane IS. Artificial intelligence in healthcare. Nature biomedical engineering. 2018;2:719-31.
- 26. Anastasi E, Manganaro L, Granato T, et al. Is CA72-4 a Useful Biomarker in Differential Diagnosis between Ovarian Endometrioma and Epithelial Ovarian Cancer? Disease Markers. 2013;35:984641.
- 27. Kobayashi H. [The clinical usefulness of serum CA72-4 analysis in patients with ovarian cancer]. Nihon Sanka Fujinka Gakkai Zasshi. 1989;41:585-9.
- 28. Drapkin R, Von Horsten HH, Lin Y, et al. Human epididymis protein 4 (HE4) is a secreted glycoprotein that is overexpressed by serous and endometrioid ovarian carcinomas. Cancer research. 2005;65:2162-9.
- 29. Anastasi E, Giovanna Marchei G, Viggiani V, et al. HE4: a new potential early biomarker for the recurrence of ovarian cancer. Tumor Biology. 2010;31:113-9.
- 30. Prodromidou A, Andreakos P, Kazakos C, Vlachos DE, Perrea D, Pergialiotis V. The diagnostic efficacy of platelet-to-lymphocyte ratio and neutrophil-to-lymphocyte ratio in ovarian cancer. Inflammation Research. 2017;66:467-75.
- 31. Asher V, Lee J, Bali A. Preoperative serum albumin is an independent prognostic predictor of survival in ovarian cancer. Medical oncology. 2012;29:2005-9.
- 32. Parker D, Bradley C, Bogle S, et al. Serum albumin and CA125 are powerful predictors of survival in epithelial ovarian cancer. BJOG: An International Journal of Obstetrics & Gynaecology. 1994;101:888-93.
- 33. Williams KA, Labidi-Galy SI, Terry KL, et al. Prognostic significance and predictors of the neutrophil-to-lymphocyte ratio in ovarian cancer. Gynecologic oncology. 2014;132:542-50.
- 34. Chen G, Zhu L, Yang Y, et al. Prognostic role of neutrophil to lymphocyte ratio in ovarian cancer: a meta-analysis. Technology in cancer research & treatment. 2018;17:1533033818791500.
APA | Ozhan O, KÜÇÜKAKÇALI Z, BALIKCI CICEK I (2023). Machine learning-based ovarian cancer prediction with XGboost and stochastic gradient boosting models. , 231 - 237. 10.5455/medscience.2022.09.207 |
Chicago | Ozhan Onural,KÜÇÜKAKÇALI ZEYNEP,BALIKCI CICEK IPEK Machine learning-based ovarian cancer prediction with XGboost and stochastic gradient boosting models. (2023): 231 - 237. 10.5455/medscience.2022.09.207 |
MLA | Ozhan Onural,KÜÇÜKAKÇALI ZEYNEP,BALIKCI CICEK IPEK Machine learning-based ovarian cancer prediction with XGboost and stochastic gradient boosting models. , 2023, ss.231 - 237. 10.5455/medscience.2022.09.207 |
AMA | Ozhan O,KÜÇÜKAKÇALI Z,BALIKCI CICEK I Machine learning-based ovarian cancer prediction with XGboost and stochastic gradient boosting models. . 2023; 231 - 237. 10.5455/medscience.2022.09.207 |
Vancouver | Ozhan O,KÜÇÜKAKÇALI Z,BALIKCI CICEK I Machine learning-based ovarian cancer prediction with XGboost and stochastic gradient boosting models. . 2023; 231 - 237. 10.5455/medscience.2022.09.207 |
IEEE | Ozhan O,KÜÇÜKAKÇALI Z,BALIKCI CICEK I "Machine learning-based ovarian cancer prediction with XGboost and stochastic gradient boosting models." , ss.231 - 237, 2023. 10.5455/medscience.2022.09.207 |
ISNAD | Ozhan, Onural vd. "Machine learning-based ovarian cancer prediction with XGboost and stochastic gradient boosting models". (2023), 231-237. https://doi.org/10.5455/medscience.2022.09.207 |
APA | Ozhan O, KÜÇÜKAKÇALI Z, BALIKCI CICEK I (2023). Machine learning-based ovarian cancer prediction with XGboost and stochastic gradient boosting models. Medicine Science, 12(1), 231 - 237. 10.5455/medscience.2022.09.207 |
Chicago | Ozhan Onural,KÜÇÜKAKÇALI ZEYNEP,BALIKCI CICEK IPEK Machine learning-based ovarian cancer prediction with XGboost and stochastic gradient boosting models. Medicine Science 12, no.1 (2023): 231 - 237. 10.5455/medscience.2022.09.207 |
MLA | Ozhan Onural,KÜÇÜKAKÇALI ZEYNEP,BALIKCI CICEK IPEK Machine learning-based ovarian cancer prediction with XGboost and stochastic gradient boosting models. Medicine Science, vol.12, no.1, 2023, ss.231 - 237. 10.5455/medscience.2022.09.207 |
AMA | Ozhan O,KÜÇÜKAKÇALI Z,BALIKCI CICEK I Machine learning-based ovarian cancer prediction with XGboost and stochastic gradient boosting models. Medicine Science. 2023; 12(1): 231 - 237. 10.5455/medscience.2022.09.207 |
Vancouver | Ozhan O,KÜÇÜKAKÇALI Z,BALIKCI CICEK I Machine learning-based ovarian cancer prediction with XGboost and stochastic gradient boosting models. Medicine Science. 2023; 12(1): 231 - 237. 10.5455/medscience.2022.09.207 |
IEEE | Ozhan O,KÜÇÜKAKÇALI Z,BALIKCI CICEK I "Machine learning-based ovarian cancer prediction with XGboost and stochastic gradient boosting models." Medicine Science, 12, ss.231 - 237, 2023. 10.5455/medscience.2022.09.207 |
ISNAD | Ozhan, Onural vd. "Machine learning-based ovarian cancer prediction with XGboost and stochastic gradient boosting models". Medicine Science 12/1 (2023), 231-237. https://doi.org/10.5455/medscience.2022.09.207 |