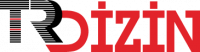
Detecting Pulp Stones with Automatic Deep Learning in Bitewing Radiographs: A Pilot Study of Artiőcial Intelligence
Yıl: 2023 Cilt: 50 Sayı: 1 Sayfa Aralığı: 12 - 16 Metin Dili: İngilizce DOI: 10.52037/eads.2023.0004 İndeks Tarihi: 31-05-2023
Detecting Pulp Stones with Automatic Deep Learning in Bitewing Radiographs: A Pilot Study of Artiőcial Intelligence
Öz: Purpose: This study aims to examine the diagnostic performance of detecting pulp stones with a deep learning model on bite-wing radiographs. Materials and Methods: 2203 radiographs were scanned retrospectively. 1745 pulp stones were marked on 1269 bite-wing radiographs with the CranioCatch labeling program (CranioCatch, Eskişehir, Turkey) in patients over 16 years old after the consensus of two experts of Maxillofacial Radiologists. This dataset was divided into 3 groups as training (n = 1017 (1396 labels), validation (n = 126 (174 labels)), and test (n = 126) (175 labels) sets, respectively. The conődence score of all tags was 84.04%; the trust of presence tags score of 85.82% and the conődence score of no labels were found to be 82.25%. The deep learning model was developed using Mask R-CNN architecture. A confusion matrix was used to evaluate the success of the model. Results: The results of precision, sensitivity, and F1 obtained using the Mask R-CNN architecture in the test dataset were found to be 0.9115, 0,8879, and 0.8995, respectively. Conclusions: Deep learning algorithms can detect pulp stones. With this, clinicians can use software systems based on artiőcial intelligence as a diagnostic support system. Mask R-CNN architecture can be used for pulp stone detection with approximately 90% sensitivity. The larger data sets increase the accuracy of deep learning systems. More studies are needed to increase the success rates of deep learning models.
Anahtar Kelime: Belge Türü: Makale Makale Türü: Araştırma Makalesi Erişim Türü: Erişime Açık
- 1. Mete U. Diş Pulpasında Meydana Gelen Kalsiőkasyonlar. J Istanb Univ Fac Dent. 2013;13(2):167ś188. doi:https://dergipark.org.tr/tr/pub/jiufd/issue/8944/111477.
- 2. Langeland K, Rodrigues H, Dowden W. Periodontal disease, bacteria, and pulpal histopathology. Oral Surg Oral Med Oral Pathol. 1974;37(2):257ś270. doi:10.1016/0030-4220(74)90421- 6.
- 3. Goga R, Chandler NP, Oginni AO. Pulp stones: a review. Int Endod J. 2008;41(6):457ś468. doi:10.1111/j.1365- 2591.2008.01374.x.
- 4. Hill TJ. Pathology of the dental pulp. J Am Dent Assoc. 1934;21(5):820ś844.
- 5. Sayegh F, Reed A. Calciőcation in the dental pulp. Oral Surg Oral Med Oral Pathol. 1968;25(6):873ś882. doi:10.1016/0030- 4220(68)90165-5.
- 6. Willman W. Numerical incidence of calciőcation in human pulps. J Dent Res. 1932;14:660. doi:10.11471/shikahozon.50.752.
- 7. James V. Early pulpal calciőcations of permanent teeth of young individuals. J Dent Res. 1958;37:973.
- 8. James VE, Schour I, Spence JM. Biology of the pulp and its defense. J Am Dent Assoc. 1959;59(5):903ś911. doi:10.14219/jada.archive.1959.0247.
- 9. Sundell JR, Stanley HR, White CL. The relationship of coronal pulp stone formation to experimental operative procedures. Oral Surg Oral Med Oral Pathol. 1968;25(4):579ś589. doi:10.1016/0030-4220(68)90303-4.
- 10. Tamse A, Kaffe I, Littner M, Shani R. Statistical evaluation of radiologic survey of pulp stones. J Endod. 1982;8(10):455ś458. doi:10.1016/S0099-2399(82)80150-7.
- 11. Deva V, Mogoantă L, Manolea H, Pancă OA, Vătu M, Vătăman M. Radiological and microscopic aspects of the denticles. Rom J Morphol Embryol. 2006;47(3):263ś268.
- 12. Moss-Salentijn L, Klyvert MH. Epithelially induced denticles in the pulps of recently erupted, noncarious human premolars. J Endod. 1983;9(12):554ś560. doi:10.1016/S0099- 2399(83)80060-0.
- 13. Willman W. Calciőcations in the pulp. Bur. 1934;34:73ś76.
- 14. Nayak M, Kumar J, Prasad LK. A radiographic correlation between systemic disorders and pulp stones. Indian J Dent Res. 2010;21(3):369. doi:10.4103/0970-9290.70806.
- 15. Moss-Salentijn L, Hendricks-Klyvert M. Calciőed structures in human dental pulps. J Endod. 1988;14(4):184ś189. doi:10.1016/S0099-2399(88)80262-0.
- 16. Hung K, Montalvao C, Tanaka R, Kawai T, Bornstein MM. The use and performance of artiőcial intelligence applications in dental and maxillofacial radiology: A systematic review. Dentomaxillofac Radiol. 2020;49(1):20190107. doi:10.1259/dmfr.20190107.
- 17. Pauwels R. A brief introduction to concepts and applications of artiőcial intelligence in dental imaging. Oral Radiol. 2021;37(1):153ś160. doi:10.1007/s11282-020-00468-5.
- 18. Kaur P, Singh G, Kaur P. A review of denoising medical images using machine learning approaches. Curr Med Imaging. 2018;14(5):675ś685. doi:10.2174/1573405613666170428154156.
- 19. Jia Y, Shelhamer E, Donahue J, Karayev S, Long J, Girshick R, et al. Caffe: Convolutional architecture for fast feature embedding. In: Proceedings of the 22nd ACM international conference on Multimedia; 2014. p. 675ś678. doi:10.1145/2647868.2654889.
- 20. Krizhevsky A, Sutskever I, Hinton GE. Imagenet classiőcation with deep convolutional neural networks. Commun ACM. 2017;60(6):84ś90. doi:10.1145/3065386.
- 21. Yang Z, Nevatia R. A multi-scale cascade fully convolutional network face detector. In: 2016 23rd International Conference on Pattern Recognition (ICPR). IEEE; 2016. p. 633ś638. doi:10.1109/ICPR.2016.7899705.
- 22. Girshick R, Donahue J, Darrell T, Malik J. Rich feature hierarchies for accurate object detection and semantic segmentation. In: Proceedings of the IEEE conference on computer vision and pattern recognition; 2015. p. 580ś587. doi:10.1109/CVPR.2014.81.
- 23. He K, Zhang X, Ren S, Sun J. Spatial pyramid pooling in deep convolutional networks for visual recognition. ECCV. 2015;37(9):1904ś1916. doi:10.1109/TPAMI.2015.2389824.
- 24. Girshick R. Fast r-cnn. In: Proceedings of the IEEE international conference on computer vision; 2015. p. 1440ś1448.
- 25. Ren S, He K, Girshick R, Sun J. Faster r-cnn: Towards real-time object detection with region proposal networks. Advances in neural information processing systems. 2015;28.
- 26. Dai J, Li Y, He K, Sun J. R-fcn: Object detection via regionbased fully convolutional networks. Adv Neural Inf Process Syst. 2016;29. doi:10.48550/arXiv.1605.06409.
- 27. Lin TY, DollAr P, Girshick R, He K, Hariharan B, Belongie S. Focal loss for dense object detection. In: Proceedings of the IEEE conference on computer vision and pattern recognition;2011. p. 2980ś2988.
- 28. He K, Gkioxari G, DollAr P, Girshick R. Mask r-cnn. In: Proceedings of the IEEE international conference on computer vision; 2017. p. 2961ś2969. doi:10.1109/ICCV.2017.322.
- 29. Santra A, Christy CJ. Genetic algorithm and confusion matrix for document clustering. Int J Comput Sci Issues. 2012;9(1):322ś 328.
- 30. Turkal M, Tan E, Uzgur R, Hamidi M, Colak H, Uzgur Z. Incidence and distribution of pulp stones found in radiographic dental examination of adult Turkish dental patients. Ann Med Health Sci Res. 2013;3(4):572ś576. doi:10.4103/2141- 9248.122115.
- 31. Tassoker M, Magat G, Sener S. A comparative study of conebeam computed tomography and digital panoramic radiography for detecting pulp stones. Imaging Sci Dent. 2018;48(3):201. doi:10.5624/isd.2018.48.3.201.
- 32. Hsieh CY, Wu YC, Su CC, Chung MP, Huang RY, Ting PY, et al. The prevalence and distribution of radiopaque, calciőed pulp stones: A cone-beam computed tomography study in a northern Taiwanese population. J Dent Sci. 2018;13(2):138ś144. doi:10.1016/j.jds.2017.06.005.
- 33. Orhan K, Bayrakdar I, Ezhov M, Kravtsov A, Özyürek T. Evaluation of artiőcial intelligence for detecting periapical pathosis on cone-beam computed tomography scans. Int Endod J. 2020;53(5):680ś689. doi:10.1111/iej.13265.
- 34. El-Damanhoury HM, Fakhruddin KS, Awad MA. Effectiveness of teaching International Caries Detection and Assessment System II and its e-learning program to freshman dental students on occlusal caries detection. Eur J Dent. 2014;8(04):493ś497. doi:10.4103/1305-7456.143631.
- 35. Minaee S, Boykov YY, Porikli F, Plaza AJ, Kehtarnavaz N, Terzopoulos D. ECCV. IEEE Transactions on Pattern Analysis and Machine Intelligence. 2021:3523ś3542. doi:10.1109/TPAMI.2021.3059968.
- 36. Bayraktar Y, Ayan E. Diagnosis of interproximal caries lesions with deep convolutional neural network in digital bitewing radiographs. Clin Oral Investig. 2022;26(1):623ś632. doi:10.1007/s00784-021-04040-1.
- 37. Rashid U, Javid A, Khan AR, Liu L, Ahmed A, Khalid O, et al. A hybrid mask RCNN-based tool to localize dental cavities from real-time mixed photographic images. PeerJ Comput Sci. 2022;8:e888. doi:10.7717/peerj-cs.888.
- 38. Kumar A, Bhadauria HS, Singh A. Descriptive analysis of dental X-ray images using various practical methods: a review. PeerJ Comput Sci. 2021;7:e620. doi:10.7717/peerj-cs.620.
- 39. Koutsouri GD, Berdouses E, Tripoliti EE, Oulis C, Fotiadis DI. Detection of occlusal caries based on digital image processing. In: 13th IEEE International Conference on BioInformatics and BioEngineering. IEEE; 2013. p. 1ś4. doi:10.1109/BIBE.2013.6701708.
- 40. Vashishth A, Kaushal B, Srivastava A. Caries detection technique for radiographic and intra oral camera images. Int J Soft Comput Eng. 2014;4(2):2231ś2307.
- 41. Moutselos K, Berdouses E, Oulis C, Maglogiannis I. Recognizing occlusal caries in dental intraoral images using deep learning. In: 2019 41st Annual International Conference of the IEEE Engineering in Medicine and Biology Society (EMBC). IEEE; 2019. p. 1617ś1620. doi:10.1109/EMBC.2019.8856553.
- 42. Silva G, Oliveira L, Pithon M. Automatic segmenting teeth in X-ray images: Trends, a novel data set, benchmarking and future perspectives. Expert Syst Appl. 2018;107:15ś31. doi:10.1016/j.eswa.2018.04.001.
- 43. Selmi A, Syed L, Abdulkareem B. Pulp Stone Detection Using Deep Learning Techniques. In: EAI International Conference on IoT Technologies for HealthCare. Springer; 2021. p. 113ś124. doi:10.1007/978-3-030-99197-5_10.
- 44. Koçoğlu F, Ozcan T. Dengeli-dengesiz veri seti dagiliminin asiri ogrenme makinesi yonetimi performansina etkisi; 2018. .
APA | Altındağ A, Uzun S, BAYRAKDAR I, Çelik Ö (2023). Detecting Pulp Stones with Automatic Deep Learning in Bitewing Radiographs: A Pilot Study of Artiőcial Intelligence. , 12 - 16. 10.52037/eads.2023.0004 |
Chicago | Altındağ Ali,Uzun Sultan,BAYRAKDAR Ibrahim Sevki,Çelik Özer Detecting Pulp Stones with Automatic Deep Learning in Bitewing Radiographs: A Pilot Study of Artiőcial Intelligence. (2023): 12 - 16. 10.52037/eads.2023.0004 |
MLA | Altındağ Ali,Uzun Sultan,BAYRAKDAR Ibrahim Sevki,Çelik Özer Detecting Pulp Stones with Automatic Deep Learning in Bitewing Radiographs: A Pilot Study of Artiőcial Intelligence. , 2023, ss.12 - 16. 10.52037/eads.2023.0004 |
AMA | Altındağ A,Uzun S,BAYRAKDAR I,Çelik Ö Detecting Pulp Stones with Automatic Deep Learning in Bitewing Radiographs: A Pilot Study of Artiőcial Intelligence. . 2023; 12 - 16. 10.52037/eads.2023.0004 |
Vancouver | Altındağ A,Uzun S,BAYRAKDAR I,Çelik Ö Detecting Pulp Stones with Automatic Deep Learning in Bitewing Radiographs: A Pilot Study of Artiőcial Intelligence. . 2023; 12 - 16. 10.52037/eads.2023.0004 |
IEEE | Altındağ A,Uzun S,BAYRAKDAR I,Çelik Ö "Detecting Pulp Stones with Automatic Deep Learning in Bitewing Radiographs: A Pilot Study of Artiőcial Intelligence." , ss.12 - 16, 2023. 10.52037/eads.2023.0004 |
ISNAD | Altındağ, Ali vd. "Detecting Pulp Stones with Automatic Deep Learning in Bitewing Radiographs: A Pilot Study of Artiőcial Intelligence". (2023), 12-16. https://doi.org/10.52037/eads.2023.0004 |
APA | Altındağ A, Uzun S, BAYRAKDAR I, Çelik Ö (2023). Detecting Pulp Stones with Automatic Deep Learning in Bitewing Radiographs: A Pilot Study of Artiőcial Intelligence. European annals of dental sciences (Online), 50(1), 12 - 16. 10.52037/eads.2023.0004 |
Chicago | Altındağ Ali,Uzun Sultan,BAYRAKDAR Ibrahim Sevki,Çelik Özer Detecting Pulp Stones with Automatic Deep Learning in Bitewing Radiographs: A Pilot Study of Artiőcial Intelligence. European annals of dental sciences (Online) 50, no.1 (2023): 12 - 16. 10.52037/eads.2023.0004 |
MLA | Altındağ Ali,Uzun Sultan,BAYRAKDAR Ibrahim Sevki,Çelik Özer Detecting Pulp Stones with Automatic Deep Learning in Bitewing Radiographs: A Pilot Study of Artiőcial Intelligence. European annals of dental sciences (Online), vol.50, no.1, 2023, ss.12 - 16. 10.52037/eads.2023.0004 |
AMA | Altındağ A,Uzun S,BAYRAKDAR I,Çelik Ö Detecting Pulp Stones with Automatic Deep Learning in Bitewing Radiographs: A Pilot Study of Artiőcial Intelligence. European annals of dental sciences (Online). 2023; 50(1): 12 - 16. 10.52037/eads.2023.0004 |
Vancouver | Altındağ A,Uzun S,BAYRAKDAR I,Çelik Ö Detecting Pulp Stones with Automatic Deep Learning in Bitewing Radiographs: A Pilot Study of Artiőcial Intelligence. European annals of dental sciences (Online). 2023; 50(1): 12 - 16. 10.52037/eads.2023.0004 |
IEEE | Altındağ A,Uzun S,BAYRAKDAR I,Çelik Ö "Detecting Pulp Stones with Automatic Deep Learning in Bitewing Radiographs: A Pilot Study of Artiőcial Intelligence." European annals of dental sciences (Online), 50, ss.12 - 16, 2023. 10.52037/eads.2023.0004 |
ISNAD | Altındağ, Ali vd. "Detecting Pulp Stones with Automatic Deep Learning in Bitewing Radiographs: A Pilot Study of Artiőcial Intelligence". European annals of dental sciences (Online) 50/1 (2023), 12-16. https://doi.org/10.52037/eads.2023.0004 |