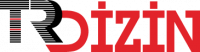
Unbiased federated learning in energy harvesting error-prone channels
Yıl: 2023 Cilt: 31 Sayı: 3 Sayfa Aralığı: 612 - 625 Metin Dili: İngilizce DOI: 10.55730/1300-0632.4005 İndeks Tarihi: 12-06-2023
Unbiased federated learning in energy harvesting error-prone channels
Öz: Federated learning (FL) is a communication-efficient and privacy-preserving learning technique for collabo- rative training of machine learning models on vast amounts of data produced and stored locally on the distributed users. This paper investigates unbiased FL methods that achieve a similar convergence as state-of-the-art methods in scenarios with various constraints like an error-prone channel or intermittent energy availability. For this purpose, we propose FL algorithms that jointly design unbiased user scheduling and gradient weighting according to each user’s distinct energy and channel profile. In addition, we exploit a prevalent metric called the age of information (AoI), which quantifies the staleness of the gradient updates at the parameter server and adaptive momentum attenuation to increase the accuracy and accelerate the convergence for nonhomogeneous data distribution of participant users. The effect of AoI and mo- mentum on fair FL with heterogeneous users on various datasets is studied, and the performance is demonstrated by experiments in several settings.
Anahtar Kelime: Belge Türü: Makale Makale Türü: Araştırma Makalesi Erişim Türü: Erişime Açık
- [1] Konečnỳ J, McMahan HB, Yu FX, Richtárik P, Suresh AT et al. Federated learning: Strategies for improving communication efficiency. arXiv preprint arXiv:1610.05492, 2016.
- [2] Kaul S, Gruteser M, Rai V, Kenney J. Minimizing age of information in vehicular networks. In: Sensor, Mesh and Ad-Hoc Communications and Networks (SECON), 8th Annual IEEE Communications Society Conference 2011; 350-358.
- [3] Kaul S, Yates RD, Gruteser M. Real-time status: How often should one update?. In: IEEE Conference on Computer Communications (INFOCOM) 2012; 2731–2735.
- [4] Konečnỳ J, McMahan B, Ramage D. Federated optimization: Distributed optimization beyond the datacenter. arXiv preprint arXiv:1511.03575; 2015.
- [5] McMahan B, Moore E, Ramage D, Hampson S, Arcas BA. Communication-efficient learning of deep networks from decentralized data. PMLR Artificial intelligence and statistics 2017; 1273-1282.
- [6] Li X, Huang K, Yang W, Wang S, Zhang Z. On the convergence of fedavg on non-iid data. arXiv preprint arXiv:1907.02189, 2019.
- [7] Xu J, Wang S, Wang L, Yao AC. Fedcm: Federated learning with client-level momentum. arXiv preprint arXiv:2106.10874, 2021.
- [8] Kim G, Kim J, Han B. Communication-Efficient Federated Learning with Acceleration of Global Momentum. arXiv preprint arXiv:2201.03172, 2022.
- [9] Liu W, Chen L, Chen Y, Zhang W. Accelerating federated learning via momentum gradient descent. IEEE Trans- actions on Parallel and Distributed Systems 2011; 31 (8): 1754-1766.
- [10] Shinohara N. Development of Rectenna with Wireless Communication System. In: Proceedings of the 5th European Conference on Antennas and Propagation (EUCAP) 2011; 3970-3973.
- [11] Popovic Z. Cut the cord: Low-power far-field wireless powering. IEEE Microwave Magazine 2013; 55-62.
- [12] Vyas RJ, Cook BB, Kawahara Y, Tentzeris MM. E-WEHP: A batteryless embedded sensor platform wirelessly powered from ambient digital-tv signal. IEEE Transactions on Microwave Theory and Techniques 2013; 61 (6): 2491-2505.
- [13] Güler B, Yener A. Energy-Harvesting Distributed Machine Learning. In: IEEE Int. Symp. on Information Theory (ISIT) 2021; 320-325.
- [14] Güler B, Yener A. Sustainable federated learning. arXiv preprint arXiv:2102.11274; 2021.
- [15] Gündüz D, Kurka DB, Jankowski M, Amiri MM, Ozfatura E et al. Communicate to learn at the edge. IEEE Communications Magazine 2020; 58 (12): 14-19.
- [16] Ozfatura E, Gunduz D, Poor HV. Collaborative learning over wireless networks: An introductory overview. arXiv preprint arXiv:2112.05559 2021.
- [17] Çakir Z, Ceran Arslan ET. Federated Learning with Channel and Energy Aware Scheduling. In: IEEE 30th Signal Processing and Communications Applications Conference; Karabük, Turkey 2022; 1-4. doi: 10.1109/SIU55565.2022.9864979
- [18] Aygün O, Kazemi M, Gündüz D, Duman TM. Over-the-Air Federated Learning with Energy Harvesting Devices. arXiv preprint arXiv:2205.12869, 2022.
- [19] Yang HH, Arafa A, Quek TQS, Poor HV. Age-based scheduling policy for federated learning in mobile edge networks. In: IEEE International Conference on Acoustics, Speech and Signal Processing (ICASSP) 2020; 8743-8747.
- [20] Buyukates B, Ulukus S. Timely communication in federated learning. In: IEEE Conference on Computer Commu- nications Workshops (INFOCOM WKSHPS) 2021; 1-6.
- [21] Liu X, Qin X, Chen H, Liu Y, Liu B et al. Age-aware Communication Strategy in Federated Learning with Energy Harvesting Devices. In: IEEE/CIC International Conference on Communications in China (ICCC) 2021; 358-363.
- [22] Krizhevsky A, Hinton G et al. Learning multiple layers of features from tiny images. Toronto, ON, Canada; 2009.
- [23] LeCun Y, Bottou L, Bengio Y, Haffner P. Gradient-based learning applied to document recognition. Proceedings of the IEEE 1998; 86 (11): 2278-2324.
- [24] Zhao Y, Li M, Lai L, Suda N, Civin D et al. Federated learning with non-iid data. arXiv preprint arXiv:1806.00582, 2018.
- [25] Wang H, Kaplan Z, Niu D, Li B. Optimizing federated learning on non-iid data with reinforcement learning. In: IEEE Conference on Computer Communications (INFOCOM) 2020; 1698-1707.
APA | ÇAKIR Z, CERAN E (2023). Unbiased federated learning in energy harvesting error-prone channels. , 612 - 625. 10.55730/1300-0632.4005 |
Chicago | ÇAKIR Zeynep,CERAN Elif Tuğçe Unbiased federated learning in energy harvesting error-prone channels. (2023): 612 - 625. 10.55730/1300-0632.4005 |
MLA | ÇAKIR Zeynep,CERAN Elif Tuğçe Unbiased federated learning in energy harvesting error-prone channels. , 2023, ss.612 - 625. 10.55730/1300-0632.4005 |
AMA | ÇAKIR Z,CERAN E Unbiased federated learning in energy harvesting error-prone channels. . 2023; 612 - 625. 10.55730/1300-0632.4005 |
Vancouver | ÇAKIR Z,CERAN E Unbiased federated learning in energy harvesting error-prone channels. . 2023; 612 - 625. 10.55730/1300-0632.4005 |
IEEE | ÇAKIR Z,CERAN E "Unbiased federated learning in energy harvesting error-prone channels." , ss.612 - 625, 2023. 10.55730/1300-0632.4005 |
ISNAD | ÇAKIR, Zeynep - CERAN, Elif Tuğçe. "Unbiased federated learning in energy harvesting error-prone channels". (2023), 612-625. https://doi.org/10.55730/1300-0632.4005 |
APA | ÇAKIR Z, CERAN E (2023). Unbiased federated learning in energy harvesting error-prone channels. Turkish Journal of Electrical Engineering and Computer Sciences, 31(3), 612 - 625. 10.55730/1300-0632.4005 |
Chicago | ÇAKIR Zeynep,CERAN Elif Tuğçe Unbiased federated learning in energy harvesting error-prone channels. Turkish Journal of Electrical Engineering and Computer Sciences 31, no.3 (2023): 612 - 625. 10.55730/1300-0632.4005 |
MLA | ÇAKIR Zeynep,CERAN Elif Tuğçe Unbiased federated learning in energy harvesting error-prone channels. Turkish Journal of Electrical Engineering and Computer Sciences, vol.31, no.3, 2023, ss.612 - 625. 10.55730/1300-0632.4005 |
AMA | ÇAKIR Z,CERAN E Unbiased federated learning in energy harvesting error-prone channels. Turkish Journal of Electrical Engineering and Computer Sciences. 2023; 31(3): 612 - 625. 10.55730/1300-0632.4005 |
Vancouver | ÇAKIR Z,CERAN E Unbiased federated learning in energy harvesting error-prone channels. Turkish Journal of Electrical Engineering and Computer Sciences. 2023; 31(3): 612 - 625. 10.55730/1300-0632.4005 |
IEEE | ÇAKIR Z,CERAN E "Unbiased federated learning in energy harvesting error-prone channels." Turkish Journal of Electrical Engineering and Computer Sciences, 31, ss.612 - 625, 2023. 10.55730/1300-0632.4005 |
ISNAD | ÇAKIR, Zeynep - CERAN, Elif Tuğçe. "Unbiased federated learning in energy harvesting error-prone channels". Turkish Journal of Electrical Engineering and Computer Sciences 31/3 (2023), 612-625. https://doi.org/10.55730/1300-0632.4005 |