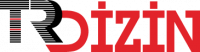
Obez ve Sağlıklı Çocuklarda Koroid Kalınlığının Klinik İlişkisi: Makine Öğrenimi Çalışması
Yıl: 2023 Cilt: 53 Sayı: 3 Sayfa Aralığı: 161 - 168 Metin Dili: Türkçe DOI: 10.4274/tjo.galenos.2022.36724 İndeks Tarihi: 05-07-2023
Obez ve Sağlıklı Çocuklarda Koroid Kalınlığının Klinik İlişkisi: Makine Öğrenimi Çalışması
Öz: Amaç: “Random forest” (RF), “support vector machine” (SVM) ve “multilayer perceptrons” (MLP) algoritmalarının performansını karşılaştırarak maküler koroid kalınlığı (MKT) ve peripapiller koroid kalınlığının (PPKT) obez ve sağlıklı çocukların sınıflandırılması üzerindeki etkisini analiz etmek. Gereç ve Yöntem: Bu prospektif karşılaştırmalı çalışmada yaşları 6-15 arasında olan 59 obez çocuk ve 35 sağlıklı çocuk optik koherens tomografi kullanılarak incelendi. MKT ve PPKT, fovea ve optik diskten 500 μm, 1.000 μm ve 1.500 μm mesafelerde ölçüldü. Çıkarılan tüm özelliklerin en belirgin olanlarını belirlemek için üç farklı özellik seçim algoritması kullanıldı. Çıkarılan özelliklerin sınıflandırma etkinliği, RF, SVM ve MLP algoritmaları kullanılarak analiz edildi ve bunların obezleri sağlıklı çocuklardan ayırt etmedeki etkinlikleri gösterildi. Ölçümlerin kesinliği ve güvenilirliği kappa analizi kullanılarak değerlendirildi. Bulgular: Korelasyon özellik seçimi algoritması, farklı özellik seçim yöntemleri arasında en başarılı sınıflandırma sonuçlarını üretmiştir. Obez ve sağlıklı grupları birbirinden ayırmada en belirgin özellikler PPKT temporal 500 μm, PPKT temporal 1.500 μm, PPKT nazal 1.500 μm, PPKT inferior 1.500 μm ve subfoveal MKT idi. RF, SVM ve MLP algoritmaları için sınıflandırma oranları sırasıyla %98,6, %96,8 ve %89 idi. Sonuç: Obezite, özellikle subfoveal bölgede ve optik disk başından 1.500 μm uzaklıktaki dış yarım dairede olmak üzere çocukların koroidal kalınlıkları üzerinde etkilidir. Hem RF hem de SVM algoritmaları, obez ve sağlıklı çocukları sınıflandırmada etkili ve doğru yöntemlerdir.
Anahtar Kelime: Clinical Relevance of Choroidal Thickness in Obese and Healthy Children: A Machine Learning Study
Öz: Objectives: To analyze the effect of macular choroidal thickness (MCT) and peripapillary choroidal thickness (PPCT) on the classification of obese and healthy children by comparing the performance of the random forest (RF), support vector machine (SVM), and multilayer perceptrons (MLP) algorithms. Materials and Methods: Fifty-nine obese children and 35 healthy children aged 6 to 15 years were studied in this prospective comparative study using optical coherence tomography. MCT and PPCT were measured at distances of 500 μm, 1,000 μm, and 1,500 μm from the fovea and optic disc. Three different feature selection algorithms were used to determine the most prominent features of all extracted features. The classification efficiency of the extracted features was analyzed using the RF, SVM, and MLP algorithms, demonstrating their efficacy for distinguishing obese from healthy children. The precision and reliability of measurements were assessed using kappa analysis. Results: The correlation feature selection algorithm produced the most successful classification results among the different feature selection methods. The most prominent features for distinguishing the obese and healthy groups from each other were PPCT temporal 500 μm, PPCT temporal 1,500 μm, PPCT nasal 1,500 μm, PPCT inferior 1,500 μm, and subfoveal MCT. The classification rates for the RF, SVM, and MLP algorithms were 98.6%, 96.8%, and 89%, respectively. Conclusion: Obesity has an effect on the choroidal thicknesses of children, particularly in the subfoveal region and the outer semi-circle at 1,500 μm from the optic disc head. Both the RF and SVM algorithms are effective and accurate at classifying obese and healthy children.
Anahtar Kelime: Belge Türü: Makale Makale Türü: Araştırma Makalesi Erişim Türü: Erişime Açık
- 1. Kosti RI, Panagiotakos DB. The epidemic of obesity in children and adolescents in the world. Cent Eur J Public Health. 2006;14:151-159.
- 2. Lobstein T, Baur L, Uauy R; IASO International Obesity TaskForce. Obesity in children and young people: a crisis in public health. Obes Rev. 2004;5(Suppl 1):4-104.
- 3. Mei Z, Grummer-Strawn LM. Standard deviation of anthropometric Z-scores as a data quality assessment tool using the 2006 WHO growth standards: a cross country analysis. Bull World Health Organ. 2007;85:441-448.
- 4. Cole TJ, Faith MS, Pietrobelli A, Heo M. What is the best measure of adiposity change in growing children: BMI, BMI %, BMI z-score or BMI centile? Eur J of Clin Nutr. 2005;59:419-425.
- 5. Age-Related Eye Disease Study Research Group. Risk factors associated with age-related nuclear and cortical cataract : a case-control study in the Age-Related Eye Disease Study, AREDS Report No. 5. Ophthalmology. 2001;108:1400-1408.
- 6. Abramson N, Abramson S. Hypercoagulability: clinical assessment and treatment. Sout Med J. 2001;94:1013-1020.
- 7. Karti O, Nalbantoglu O, Abali S, Tunc S, Ozkan B. The assessment of peripapillary retinal nerve fiber layer and macular ganglion cell layer changes in obese children: a cross-sectional study using optical coherence tomography. Int Ophthalmol. 2017;37:1031-1038.
- 8. Aiello LP, Avery RL, Arrigg PG, Keyt BA, Jampel HD, Shah ST, Pasquale LR, Thieme H, Iwamoto MA, Park JE, et al. Vascular endothelial growth factor in ocular fluid of patients with diabetic retinopathy and other retinal disorders. N Engl J Med. 1994;331:1480-1487.
- 9. Alam AA, Mitwalli AH, Al-Wakeel JS, Chaudhary AR, Zebaid MA. Plasma fibrinogen and its correlates in adult Saudi population. Saudi Med J. 2004;25:1593-1602.
- 10. Baran RT, Baran SO, Toraman NF, Filiz S, Demirbilek H. Evaluation of intraocular pressure and retinal nerve fiber layer, retinal ganglion cell, central macular thickness, and choroidal thickness using optical coherence tomography in obese children and healthy controls. Niger J Clin Pract. 2019;22:539-545.
- 11. Bulus AD, Can ME, Baytaroglu A, Can GD, Cakmak HB, Andiran N. Choroidal Thickness in Childhood Obesity. Ophthalmic Surg Lasers Imaging Retina. 2017;48:10-17.
- 12. Topcu-Yilmaz P, Akyurek N, Erdogan E. The effect of obesity and insulin resistance on macular choroidal thickness in a pediatric population as assessed by enhanced depth imaging optical coherence tomography. J Pediatr Endocrinol Metab. 2018;31:855-860.
- 13. Hansen M, Dubayah R, Defries R. Classification trees: an alternative to traditional land cover classifiers. Int J Remote Sens. 1996;17:1075-1081.
- 14. Huang C, Davis LS, Townshend JRG. An assessment of support vector machines for land cover classification. Int J Remote Sens. 2002;23:725-749.
- 15. Foody GM. Sample size determination for image classification accuracy assessment and comparison. Int J Remote Sens. 2009;30:5273-5291.
- 16. Friedl MA, Brodley CE, Strahler AH. Maximizing land cover classification accuracies produced by decision trees at continental to global scale. IEEE Trans Geosci Remote Sens.1999;37:969-977.
- 17. Svetnik V, Liaw A, Tong C, Culberson JC, Sheridan RP, Feuston BP. Random forest: a classification and regression tool for compound classification and QSAR modeling. J Chem Inf Comput Sci. 2003;43:1947-1958.
- 18. Jayadeva, Khemchandani R, Chandra S. Twin Support Vector Machines for pattern classification. IEEE Trans Pattern Anal Mach Intell. 2007;29:905- 910.
- 19. Liu M, Wang M, Wang J, Li D. Comparison of random forest, support vector machine and back propagation neural network for electronic tongue data classification: Application to the recognition of orange beverage and Chinese vinegar. Sens Actuators B Chem. 2013;177:970-980.
- 20. Dong Y, Zhang Y, Yue J, Hu Z. Comparison of random forest, random ferns and support vector machine for eye state classification. Multimed Tools Appl. 2016;75:11763-11783.
- 21. Agarwal S, Kumar M, Jangir SK, Sharma C. Computer-Aided Cataract Detection Using MLP and SVM. In Artificial Intelligence and Global Society. 2021:103-114.
- 22. Improta G, Ricciardi C, Cesarelli G, D’Addio G, Bifulco P, Cesarelli M. Machine learning models for the prediction of acuity and variability of eye-positioning using features extracted from oculography. Health and Technology. 2020;10:961-968.
- 23. Avilés-Rodríguez GJ, Nieto-Hipólito JI, Cosío-León MLÁ, Romo-Cárdenas GS, Sánchez-López JD, Radilla-Chávez P, Vázquez-Briseño M. Topological Data Analysis for Eye Fundus Image Quality Assessment. Diagnostics (Basel). 2021;11:1322.
- 24. da Cruz LB, Souza JC, de Sousa JA, Santos AM, de Paiva AC, de Almeida JDS, Silva AC, Junior GB, Gattass M. Interferometer eye image classification for dry eye categorization using phylogenetic diversity indexes for texture analysis. Comput Methods Programs Biomed. 2020;188:105269.
- 25. Lurbe E, Agabiti-Rosei E, Cruickshank JK, Dominiczak A, Erdine S, Hirth A, Invitti C, Litwin M, Mancia G, Pall D, Rascher W, Redon J, Schaefer F, Seeman T, Sinha M, Stabouli S, Webb NJ, Wühl E, Zanchetti A. 2016 European Society of Hypertension guidelines for the management of high blood pressure in children and adolescents. J Hypertens. 2016;34:1887-1920.
- 26. Ho J, Branchini L, Regatieri C, Krishnan C, Fujimoto JG, Duker JS. Analysis of normal peripapillary choroidal thickness via spectral domain optical coherence tomography. Ophthalmology. 2011;118:2001-2007.
- 27. Landis JR, Koch GG. The measurement of observer agreement for categorical data. Biometrics. 1977;33:159-174.
- 28. Read SA, Alonso-Caneiro D, Vincent SJ, Collins MJ. Peripapillary choroidal thickness in childhood. Exp Eye Res. 2015;135:164-173.
- 29. Ozcimen M, Sakarya Y, Kurtipek E, Bekci TT, Goktas S, Sakarya R, Yener HI, Demir LS, Erdogan E, Ivacik IS, Alpfidan I, Bukus A. Peripapillary choroidal thickness in patients with chronic obstructive pulmonary disease. Cutan Ocul Toxicol. 2016;35:26-30.
- 30. Komma S, Chhablani J, Ali MH, Garudadri CS, Senthil S. Comparison of peripapillary and subfoveal choroidal thickness in normal versus primary open- angle glaucoma (POAG) subjects using spectral domain optical coherence tomography (SD-OCT) and swept source optical coherence tomography (SS-OCT). BMJ Open Ophthalmol. 2019;4:e000258.
- 31. Ruiz Hidalgo I, Rozema JJ, Saad A, Gatinel D, Rodriguez P, Zakaria N, Koppen C. Validation of an Objective Keratoconus Detection System Implemented in a Scheimpflug Tomographer and Comparison With Other Methods. Cornea. 2017;36:689-695.
- 32. An G, Omodaka K, Tsuda S, Shiga Y, Takada N, Kikawa T, Nakazawa T, Yokota H, Akiba M. Comparison of Machine-Learning Classification Models for Glaucoma Management. J Healthc Eng. 2018;2018:6874765.
- 33. Cartes C, López D, Salinas D, Segovia C, Ahumada C, Pérez N, Valenzuela F, Lanza N, López Solís RO, Perez VL, Zegers P, Fuentes A, Alarcón C, Traipe L. Dry eye is matched by increased intrasubject variability in tear osmolarity as confirmed by machine learning approach. Arch Soc Esp Oftalmol (Engl Ed). 2019;94:337-342.
- 34. Tan Z, Scheetz J, He M. Artificial Intelligence in Ophthalmology: Accuracy, Challenges, and Clinical Application. Asia Pac J Ophthalmol (Phila). 2019;8:197-199.
APA | Bulut E, KOPRUBASI S, dayi o, Bulut H (2023). Obez ve Sağlıklı Çocuklarda Koroid Kalınlığının Klinik İlişkisi: Makine Öğrenimi Çalışması. , 161 - 168. 10.4274/tjo.galenos.2022.36724 |
Chicago | Bulut Erkan,KOPRUBASI SUMEYRA,dayi ozlem,Bulut Hatice Obez ve Sağlıklı Çocuklarda Koroid Kalınlığının Klinik İlişkisi: Makine Öğrenimi Çalışması. (2023): 161 - 168. 10.4274/tjo.galenos.2022.36724 |
MLA | Bulut Erkan,KOPRUBASI SUMEYRA,dayi ozlem,Bulut Hatice Obez ve Sağlıklı Çocuklarda Koroid Kalınlığının Klinik İlişkisi: Makine Öğrenimi Çalışması. , 2023, ss.161 - 168. 10.4274/tjo.galenos.2022.36724 |
AMA | Bulut E,KOPRUBASI S,dayi o,Bulut H Obez ve Sağlıklı Çocuklarda Koroid Kalınlığının Klinik İlişkisi: Makine Öğrenimi Çalışması. . 2023; 161 - 168. 10.4274/tjo.galenos.2022.36724 |
Vancouver | Bulut E,KOPRUBASI S,dayi o,Bulut H Obez ve Sağlıklı Çocuklarda Koroid Kalınlığının Klinik İlişkisi: Makine Öğrenimi Çalışması. . 2023; 161 - 168. 10.4274/tjo.galenos.2022.36724 |
IEEE | Bulut E,KOPRUBASI S,dayi o,Bulut H "Obez ve Sağlıklı Çocuklarda Koroid Kalınlığının Klinik İlişkisi: Makine Öğrenimi Çalışması." , ss.161 - 168, 2023. 10.4274/tjo.galenos.2022.36724 |
ISNAD | Bulut, Erkan vd. "Obez ve Sağlıklı Çocuklarda Koroid Kalınlığının Klinik İlişkisi: Makine Öğrenimi Çalışması". (2023), 161-168. https://doi.org/10.4274/tjo.galenos.2022.36724 |
APA | Bulut E, KOPRUBASI S, dayi o, Bulut H (2023). Obez ve Sağlıklı Çocuklarda Koroid Kalınlığının Klinik İlişkisi: Makine Öğrenimi Çalışması. Türk Oftalmoloji Dergisi, 53(3), 161 - 168. 10.4274/tjo.galenos.2022.36724 |
Chicago | Bulut Erkan,KOPRUBASI SUMEYRA,dayi ozlem,Bulut Hatice Obez ve Sağlıklı Çocuklarda Koroid Kalınlığının Klinik İlişkisi: Makine Öğrenimi Çalışması. Türk Oftalmoloji Dergisi 53, no.3 (2023): 161 - 168. 10.4274/tjo.galenos.2022.36724 |
MLA | Bulut Erkan,KOPRUBASI SUMEYRA,dayi ozlem,Bulut Hatice Obez ve Sağlıklı Çocuklarda Koroid Kalınlığının Klinik İlişkisi: Makine Öğrenimi Çalışması. Türk Oftalmoloji Dergisi, vol.53, no.3, 2023, ss.161 - 168. 10.4274/tjo.galenos.2022.36724 |
AMA | Bulut E,KOPRUBASI S,dayi o,Bulut H Obez ve Sağlıklı Çocuklarda Koroid Kalınlığının Klinik İlişkisi: Makine Öğrenimi Çalışması. Türk Oftalmoloji Dergisi. 2023; 53(3): 161 - 168. 10.4274/tjo.galenos.2022.36724 |
Vancouver | Bulut E,KOPRUBASI S,dayi o,Bulut H Obez ve Sağlıklı Çocuklarda Koroid Kalınlığının Klinik İlişkisi: Makine Öğrenimi Çalışması. Türk Oftalmoloji Dergisi. 2023; 53(3): 161 - 168. 10.4274/tjo.galenos.2022.36724 |
IEEE | Bulut E,KOPRUBASI S,dayi o,Bulut H "Obez ve Sağlıklı Çocuklarda Koroid Kalınlığının Klinik İlişkisi: Makine Öğrenimi Çalışması." Türk Oftalmoloji Dergisi, 53, ss.161 - 168, 2023. 10.4274/tjo.galenos.2022.36724 |
ISNAD | Bulut, Erkan vd. "Obez ve Sağlıklı Çocuklarda Koroid Kalınlığının Klinik İlişkisi: Makine Öğrenimi Çalışması". Türk Oftalmoloji Dergisi 53/3 (2023), 161-168. https://doi.org/10.4274/tjo.galenos.2022.36724 |