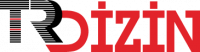
Segmentation of acute pulmonary embolism in computed tomography pulmonary angiography using the deep learning method
Yıl: 2023 Cilt: 71 Sayı: 2 Sayfa Aralığı: 131 - 137 Metin Dili: İngilizce DOI: 10.5578/tt.20239916 İndeks Tarihi: 07-07-2023
Segmentation of acute pulmonary embolism in computed tomography pulmonary angiography using the deep learning method
Öz: Introduction: Pulmonary embolism is a type of thromboembolism seen in the main pulmonary artery and its branches. This study aimed to diagnose acute pulmonary embolism using the deep learning method in computed tomograp- hic pulmonary angiography (CTPA) and perform the segmentation of pulmo- nary embolism data. Materials and Methods: The CTPA images of patients diagnosed with pulmo- nary embolism who underwent scheduled imaging were retrospectively eva- luated. After data collection, the areas that were diagnosed as embolisms in the axial section images were segmented. The dataset was divided into three parts: training, validation, and testing. The results were calculated by selecting 50% as the cut-off value for the intersection over the union. Results: Images were obtained from 1.550 patients. The mean age of the pati- ents was 64.23 ± 15.45 years. A total of 2.339 axial computed tomography images obtained from the 1.550 patients were used. The PyTorch U-Net was used to train 400 epochs, and the best model, epoch 178, was recorded. In the testing group, the number of true positives was determined as 471, the number of false positives as 35, and 27 cases were not detected. The sensitivity of CTPA segmentation was 0.95, the precision value was 0.93, and the F1 score value was 0.94. The area under the curve value obtained in the receiver operating characteristic analysis was calculated as 0.88. Conclusion: In this study, the deep learning method was successfully emplo- yed for the segmentation of acute pulmonary embolism in CTPA, yielding positive outcomes.
Anahtar Kelime: Derin öğrenme yöntemiyle akut pulmoner embolinin bilgisayarlı tomografik pulmoner anjiografide segmentasyonu
Öz: Giriş: Pulmoner emboli, ana pulmoner arterde ve dallarında izlenen tromboemboli çeşididir. Çalışmamızda akut pulmoner emboli tanısını bilgisayarlı tomografik pulmoner anjiyografide (BTPA) derin öğrenme metoduyla koyabilmek, pulmoner embolinin segmen- tasyonunu yapmak amaçlandı. Materyal ve Metod: Randevulu çekim yapılan pulmoner emboli tanısı almış hastaların BTPA görüntüleri retrospektif olarak değerlen- dirildi. Mediasten penceresinde aksiyel kesit görüntüler alındı. Veri koleksiyonu yapıldıktan sonra aksiyel kesit görüntülerde emboli tanısı konulan alanlar segmente edildi. Veri seti eğitim-doğrulama-test olacak şekilde üç parçaya bölündü. Birleşim Üzerinde Kesişim istatistiğinin eşik değeri olarak %50 seçilerek sonuçlar hesaplandı. Bulgular: Çalışmamıza toplamda 1550 hastadan elde edilen görüntüler dahil edildi. Hastaların yaş ortalamaları 64,23 ± 15,45 yıl idi. Çalışmaya dahil edilen 1550 hastadan elde edilen toplam 2339 adet aksiyel bilgisayarlı tomografi görüntüsü kullanıldı. Pytorch Unet ile 400 epoch eğitildi, en iyi model olan 178 epoch modeli kaydedildi. Test grubunda doğru bulunan, 471; yanlış bulunan, 35; bulu- namayan, 27 olarak saptandı. Çalışmamızın sensitivitesi 0,95; precision değeri 0,93; F1 skor değeri 0,94 olarak bulundu. Çalışmaya ait receiver operating characteristics (ROC) analizinde elde edilen AUC değeri 0,88 olarak hesaplandı. Sonuç: Sonuç olarak çalışmamızda derin öğrenme yöntemi kullanarak akut pulmoner embolinin bilgisayarlı tomografik pulmoner anjiyografide segmentasyonu yapılmış olup başarılı sonuçlar elde edilmiştir.
Anahtar Kelime: Belge Türü: Makale Makale Türü: Araştırma Makalesi Erişim Türü: Erişime Açık
- 1. Agnelli G, Becattini C. Acute pulmonary embolism. N Engl J Med 2010; 363(3): 266-74. https://doi.org/10.1056/ NEJMra0907731
- 2. Brown MD, Rowe BH, Reeves MJ, Bermingham JM, Goldhaber SZ. The accuracy of the enzyme-linked immu- nosorbent assay D-dimer test in the diagnosis of pulmonary embolism: A meta-analysis. Ann Emerg Med 2002; 40(2): 133-44. https://doi.org/10.1067/mem.2002.124755
- 3. PIOPED Investigators. Value of the ventilation/perfusion scan in acute pulmonary embolism. Results of the pro- spective investigation of pulmonary embolism diagnosis (PIOPED). JAMA 1990; 263(20): 2753-9. https://doi. org/10.1001/jama.263.20.2753
- 4. Borris LC, Christiansen HM, Lassen MR, Olsen AD, Schøtt P. Comparison of real-time B-mode ultrasonography and bilat- eral ascending phlebography for detection of postoperative deep vein thrombosis following elective hip surgery. The Venous Thrombosis Group. Thromb Haemost 1989; 61(3): 363-5. https://doi.org/10.1055/s-0038-1646596
- 5. Rathbun SW, Raskob GE, Whitsett TL. Sensitivity and spec- ificity of helical computed tomography in the diagnosis of pulmonary embolism: A systematic review. Ann Intern Med 2000; 132(3): 227-32. https://doi.org/10.7326/0003- 4819-132-3-200002010-00009
- 6. Stein PD, Fowler SE, Goodman LR, Gottschalk A, Hales CA, Hull RD, et al. Multidetector computed tomography for acute pulmonary embolism. N Engl J Med 2006; 354(22): 2317-27. https://doi.org/10.1056/NEJMoa052367
- 7. Cozzi D, Moroni C, Cavigli E, Bindi A, Caviglioli C, Nazerian P, et al. Prognostic value of CT pulmonary angi- ography parameters in acute pulmonary embolism. Radiol Med 2021; 126(8): 1030-6. https://doi.org/10.1007/ s11547-021-01364-6
- 8. Gottschalk A, Stein PD, Goodman LR, Sostman HD. Overview of Prospective Investigation of Pulmonary Embolism Diagnosis II. Semin Nucl Med 2002; 32(3): 173-82. https://doi.org/10.1053/snuc.2002.124177
- 9. Estrada-Y-Martin RM, Oldham SA. CTPA as the gold stan- dard for the diagnosis of pulmonary embolism. Int J Comput Assist Radiol Surg 2011; 6(4): 557-63. https:// doi.org/10.1007/s11548-010-0526-4
- 10. Ghaye B, Remy J, Remy-Jardin M. Non-traumatic thoracic emergencies: CT diagnosis of acute pulmonary embolism: The first 10 years. Eur Radiol 2002; 12(8): 1886-905. https://doi.org/10.1007/s00330-002-1506-z
- 11. Washington L, Goodman LR, Gonyo MB. CT for thrombo- embolic disease. Radiol Clin North Am 2002; 40(4): 751- 71. https://doi.org/10.1016/S0033-8389(02)00018-0
- 12. Wittram C, Maher MM, Yoo AJ, Kalra MK, Shepard JA, McLoud TC. CT angiography of pulmonary embolism: Diagnostic criteria and causes of misdiagnosis. Radiographics 2004; 24(5): 1219-38. https://doi. org/10.1148/rg.245045008
- 13. Akkus Z, Galimzianova A, Hoogi A, Rubin DL, Erickson BJ. Deep Learning for Brain MRI Segmentation: State of the Art and Future Directions. J Digit Imaging 2017; 30(4): 449-59. https://doi.org/10.1007/s10278-017-9983-4
- 14. Gaonkar B, Hovda D, Martin N, Macyszyn L. Deep learn- ing in the small sample size setting: cascaded feed forward neural networks for medical image segmentation. In Medical Imaging 2016: Computer-Aided Diagnosis 2016: 97852I. https://doi.org/10.1117/12.2216555
- 15. Liu W, Liu M, Guo X, Zhang P, Zhang L, Zhang R, et al. Evaluation of acute pulmonary embolism and clot burden on CTPA with deep learning. Eur Radiol 2020; 30(6): 3567-75. https://doi.org/10.1007/s00330-020-06699-8
- 16. Ronneberger O, Fischer P, Brox T. U-Net: Convolutional Networks for Biomedical Image Segmentation. In: Navab N, Hornegger J, Wells W, Frangi A eds. Medical Image Computing and Computer-Assisted Intervention - MICCAI 2015. Birkhäuser Verlag AG: Springer, Cham, 2015: 234- 41. https://doi.org/10.1007/978-3-319-24574-4_28
- 17. Tan PN, Steinbach M, Kumar V. Introduction to Data Mining. Boston: Pearson Addison Wesley, 2005.
- 18. Weikert T, Winkel DJ, Bremerich J, Stieltjes B, Parmar V, Sauter AW, et al. Automated detection of pulmonary embolism in CT pulmonary angiograms using an AI-powered algorithm. Eur Radiol 2020; 30: 6545-53. https://doi.org/10.1007/s00330-020-06998-0
- 19. Wittenberg R, Peters JF, Sonnemans JJ, Prokop M, Schaefer- Prokop CM. Computer-assisted detection of pulmonary embolism: Evaluation of pulmonary CT angiograms per- formed in an on-call setting. Eur Radiol 2010; 20(4): 801- 6. https://doi.org/10.1007/s00330-009-1628-7
- 20. Rucco M, Sousa-Rodrigues D, Merelli E, Johnson JH, Falsetti L, Nitti C, et al. Neural hypernetwork approach for pulmonary embolism diagnosis. BMC Res Notes 2015; 8: 617. https://doi.org/10.1186/s13104-015-1554-5
- 21. Huang SC, Pareek A, Zamanian R, Banerjee I, Lungren MP. Multimodal fusion with deep neural networks for leverag- ing CT imaging and electronic health record: A case-study in pulmonary embolism detection. Sci Rep 2020; 10(1): 22147. https://doi.org/10.1038/s41598-020-78888-w
- 22. Huang SC, Kothari T, Banerjee I, Chute C, Ball RL, Borus N, et al. PENet-a scalable deep-learning model for automated diagnosis of pulmonary embolism using volumetric CT imaging. NPJ Digit Med 2020; 3: 61. https://doi. org/10.1038/s41746-020-0266-y
- 23. Liu W, Liu M, Guo X, Zhang P, Zhang L, Zhang R, et al. Evaluation of acute pulmonary embolism and clot burden on CTPA with deep learning. Eur Radiol 2020; 30(6): 3567-75. https://doi.org/10.1007/s00330-020-06699-8
- 24. Ma X, Ferguson EC, Jiang X, Savitz SI, Shams S. A multitask deep learning approach for pulmonary embolism detec- tion and identification. Sci Rep 2022; 12(1): 13087. https://doi.org/10.1038/s41598-022-16976-9
- 25. Islam NU, Gehlot S, Zhou Z, Gotway MB, Liang J. Seeking an Optimal Approach for Computer-Aided Pulmonary Embolism Detection. Mach Learn Med Imaging 2021; 12966: 692-702. https://doi.org/10.1007/978-3-030- 87589-3_71
APA | Aydın N, CIHAN C, Çelik Ö, ASLAN A, Odabas A, ALATAS F, Yıldırım H (2023). Segmentation of acute pulmonary embolism in computed tomography pulmonary angiography using the deep learning method. , 131 - 137. 10.5578/tt.20239916 |
Chicago | Aydın Nevin,CIHAN CAGATAY,Çelik Özer,ASLAN Ahmet Faruk,Odabas Alper,ALATAS FÜSUN,Yıldırım Huseyin Segmentation of acute pulmonary embolism in computed tomography pulmonary angiography using the deep learning method. (2023): 131 - 137. 10.5578/tt.20239916 |
MLA | Aydın Nevin,CIHAN CAGATAY,Çelik Özer,ASLAN Ahmet Faruk,Odabas Alper,ALATAS FÜSUN,Yıldırım Huseyin Segmentation of acute pulmonary embolism in computed tomography pulmonary angiography using the deep learning method. , 2023, ss.131 - 137. 10.5578/tt.20239916 |
AMA | Aydın N,CIHAN C,Çelik Ö,ASLAN A,Odabas A,ALATAS F,Yıldırım H Segmentation of acute pulmonary embolism in computed tomography pulmonary angiography using the deep learning method. . 2023; 131 - 137. 10.5578/tt.20239916 |
Vancouver | Aydın N,CIHAN C,Çelik Ö,ASLAN A,Odabas A,ALATAS F,Yıldırım H Segmentation of acute pulmonary embolism in computed tomography pulmonary angiography using the deep learning method. . 2023; 131 - 137. 10.5578/tt.20239916 |
IEEE | Aydın N,CIHAN C,Çelik Ö,ASLAN A,Odabas A,ALATAS F,Yıldırım H "Segmentation of acute pulmonary embolism in computed tomography pulmonary angiography using the deep learning method." , ss.131 - 137, 2023. 10.5578/tt.20239916 |
ISNAD | Aydın, Nevin vd. "Segmentation of acute pulmonary embolism in computed tomography pulmonary angiography using the deep learning method". (2023), 131-137. https://doi.org/10.5578/tt.20239916 |
APA | Aydın N, CIHAN C, Çelik Ö, ASLAN A, Odabas A, ALATAS F, Yıldırım H (2023). Segmentation of acute pulmonary embolism in computed tomography pulmonary angiography using the deep learning method. Tüberküloz ve Toraks, 71(2), 131 - 137. 10.5578/tt.20239916 |
Chicago | Aydın Nevin,CIHAN CAGATAY,Çelik Özer,ASLAN Ahmet Faruk,Odabas Alper,ALATAS FÜSUN,Yıldırım Huseyin Segmentation of acute pulmonary embolism in computed tomography pulmonary angiography using the deep learning method. Tüberküloz ve Toraks 71, no.2 (2023): 131 - 137. 10.5578/tt.20239916 |
MLA | Aydın Nevin,CIHAN CAGATAY,Çelik Özer,ASLAN Ahmet Faruk,Odabas Alper,ALATAS FÜSUN,Yıldırım Huseyin Segmentation of acute pulmonary embolism in computed tomography pulmonary angiography using the deep learning method. Tüberküloz ve Toraks, vol.71, no.2, 2023, ss.131 - 137. 10.5578/tt.20239916 |
AMA | Aydın N,CIHAN C,Çelik Ö,ASLAN A,Odabas A,ALATAS F,Yıldırım H Segmentation of acute pulmonary embolism in computed tomography pulmonary angiography using the deep learning method. Tüberküloz ve Toraks. 2023; 71(2): 131 - 137. 10.5578/tt.20239916 |
Vancouver | Aydın N,CIHAN C,Çelik Ö,ASLAN A,Odabas A,ALATAS F,Yıldırım H Segmentation of acute pulmonary embolism in computed tomography pulmonary angiography using the deep learning method. Tüberküloz ve Toraks. 2023; 71(2): 131 - 137. 10.5578/tt.20239916 |
IEEE | Aydın N,CIHAN C,Çelik Ö,ASLAN A,Odabas A,ALATAS F,Yıldırım H "Segmentation of acute pulmonary embolism in computed tomography pulmonary angiography using the deep learning method." Tüberküloz ve Toraks, 71, ss.131 - 137, 2023. 10.5578/tt.20239916 |
ISNAD | Aydın, Nevin vd. "Segmentation of acute pulmonary embolism in computed tomography pulmonary angiography using the deep learning method". Tüberküloz ve Toraks 71/2 (2023), 131-137. https://doi.org/10.5578/tt.20239916 |