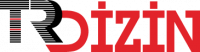
The diagnosis of femoroacetabular impingement can be made on pelvis radiographs using deep learning methods
Yıl: 2023 Cilt: 34 Sayı: 2 Sayfa Aralığı: 298 - 304 Metin Dili: İngilizce DOI: 10.52312/jdrs.2023.996 İndeks Tarihi: 11-07-2023
The diagnosis of femoroacetabular impingement can be made on pelvis radiographs using deep learning methods
Öz: Objectives: The aim of this study was to evaluate diagnostic ability of deep learning models, particularly convolutional neural network models used for image classification, for femoroacetabular impingement (FAI) using hip radiographs. Materials and methods: Between January 2010 and December 2020, pelvic radiographs of a total of 516 patients (270 males, 246 females; mean age: 39.1±3.8 years; range, 20 to 78 years) with hip pain were retrospectively analyzed. Based on inclusion and exclusion criteria, a total of 888 hip radiographs (308 diagnosed with FAI and 508 considered normal) were evaluated using deep learning methods. Pre-trained VGG-16, ResNet-101, MobileNetV2, and Inceptionv3 models were used for transfer learning. Results: As assessed by performance measures such as accuracy, sensitivity, specificity, precision, F-1 score, and area under the curve (AUC), the VGG-16 model outperformed other pre-trained networks in diagnosing FAI. With the pre-trained VGG-16 model, the results showed 86.6% accuracy, 82.5% sensitivity, 89.6% specificity, 85.5% precision, 83.9% F1 score, and 0.92 AUC. Conclusion: In patients with suspected FAI, pelvic radiography is the first imaging method to be applied, and deep learning methods can help in the diagnosis of this syndrome.
Anahtar Kelime: Belge Türü: Makale Makale Türü: Araştırma Makalesi Erişim Türü: Erişime Açık
- 1. Ganz R, Parvizi J, Beck M, Leunig M, Nötzli H, Siebenrock KA. Femoroacetabular impingement: A cause for osteoarthritis of the hip. Clin Orthop Relat Res 2003;(417):112-20. doi: 10.1097/01.blo.0000096804.78689.c2.
- 2. Dooley PJ. Femoroacetabular impingement syndrome: Nonarthritic hip pain in young adults. Can Fam Physician 2008;54:42-7.
- 3. Macfarlane RJ, Haddad FS. The diagnosis and management of femoro-acetabular impingement. Ann R Coll Surg Engl 2010;92:363-7. doi: 10.1308/003588410X12699663903791.
- 4. Jesse MK, Petersen B, Strickland C, Mei-Dan O. Normal anatomy and imaging of the hip: Emphasis on impingement assessment. Semin Musculoskelet Radiol 2013;17:229-47. doi: 10.1055/s-0033-1348090.
- 5. Kim HE, Cosa-Linan A, Santhanam N, Jannesari M, Maros ME, Ganslandt T. Transfer learning for medical image classification: A literature review. BMC Med Imaging 2022;22:69. doi: 10.1186/s12880-022-00793-7.
- 6. Anwar SM, Majid M, Qayyum A, Awais M, Alnowami M, Khan MK. Medical image analysis using convolutional neural networks: A review. J Med Syst 2018;42:226. doi: 10.1007/s10916-018-1088-1.
- 7. Maraş Y, Tokdemir G, Üreten K, Atalar E, Duran S, Maraş H. Diagnosis of osteoarthritic changes, loss of cervical lordosis, and disc space narrowing on cervical radiographs with deep learning methods. Jt Dis Relat Surg 2022;33:93- 101. doi: 10.52312/jdrs.2022.445.
- 8. Wang H, Pujos-Guillot E, Comte B, de Miranda JL, Spiwok V, Chorbev I, et al. Deep learning in systems medicine. Brief Bioinform 2021;22:1543-59. doi: 10.1093/bib/bbaa237.
- 9. Singh S, Maxwell J, Baker JA, Nicholas JL, Lo JY. Computer- aided classification of breast masses: performance and interobserver variability of expert radiologists versus residents. Radiology 2011;258:73-80. doi: 10.1148/ radiol.10081308.
- 10. Litjens G, Kooi T, Bejnordi BE, Setio AAA, Ciompi F, Ghafoorian M, et al. A survey on deep learning in medical image analysis. Med Image Anal 2017;42:60-88. doi: 10.1016/j. media.2017.07.005.
- 11. Olsen TG, Jackson BH, Feeser TA, Kent MN, Moad JC, Krishnamurthy S, et al. Diagnostic performance of deep learning algorithms applied to three common diagnoses in dermatopathology. J Pathol Inform 2018;9:32. doi: 10.4103/ jpi.jpi_31_18.
- 12. Choi RY, Coyner AS, Kalpathy-Cramer J, Chiang MF, Campbell JP. Introduction to machine learning, neural networks, and deep learning. Transl Vis Sci Technol 2020;9:14. doi: 10.1167/tvst.9.2.14.
- 13. Bizopoulos P, Koutsouris D. Deep learning in cardiology. IEEE Rev Biomed Eng 2019;12:168-93. doi: 10.1109/ RBME.2018.2885714.
- 14. Atalar H, Üreten K, Tokdemir G, Tolunay T, Çiçeklidağ M, Atik OŞ. The Diagnosis of developmental dysplasia of the hip from hip ultrasonography images with deep learning methods. J Pediatr Orthop 2023;43:132-7. doi: 10.1097/BPO.0000000000002294.
- 15. Atik OŞ. Artificial intelligence, machine learning, and deep learning in orthopedic surgery. Jt Dis Relat Surg 2022;33:484-5. doi: 10.52312/jdrs.2022.57906.
- 16. Üreten K, Maraş Y, Duran S, Gök K. Deep learning methods in the diagnosis of sacroiliitis from plain pelvic radiographs. Mod Rheumatol 2023;33:202-6. doi: 10.1093/mr/roab124.
- 17. Zou L, Yu S, Meng T, Zhang Z, Liang X, Xie Y. A Technical review of convolutional neural network-based mammographic breast cancer diagnosis. Comput Math Methods Med 2019;2019:6509357. doi: 10.1155/2019/6509357.
- 18. Tulbure AA, Tulbure AA, Dulf EH. A review on modern defect detection models using DCNNs - deep convolutional neural networks. J Adv Res 2021;35:33-48. doi: 10.1016/j. jare.2021.03.015.
- 19. Kellgren JH, Lawrence JS. Radiological assessment of osteo- arthrosis. Ann Rheum Dis 1957;16:494-502. doi: 10.1136/ ard.16.4.494.
- 20. Rothe R, Guillaumin M, Gool LV. Non-maximum suppression for object detection by passing messages between windows. In: ?????, editors. Asian conference on computer vision. Cham: Springer; 2014. p. 290-306.
- 21. Nie Y, Sommella P, O’Nils M, Liguori C, Lundgren J. Automatic detection of melanoma with yolo deep convolutional neural networks. In: E-Health and Bioengineering Conference EHB 2019. September 30, 2019 Iasi, Romania: 2019. p. 1-4.
- 22. Yamashita R, Nishio M, Do RKG, Togashi K. Convolutional neural networks: An overview and application in radiology. Insights Imaging 2018;9:611-29. doi: 10.1007/s13244-018-0639- 9.
- 23. Li J, Li S, Li X, Miao S, Dong C, Gao C, et al. Primary bone tumor detection and classification in full-field bone radiographs via YOLO deep learning model. Eur Radiol 2022. doi: 10.1007/s00330-022-09289-y.
- 24. Üreten K, Arslan T, Gültekin KE, Demir AND, Özer HF, Bilgili Y. Detection of hip osteoarthritis by using plain pelvic radiographs with deep learning methods. Skeletal Radiol 2020;49:1369-74. doi: 10.1007/s00256-020-03433-9.
- 25. Xue Y, Zhang R, Deng Y, Chen K, Jiang T. A preliminary examination of the diagnostic value of deep learning in hip osteoarthritis. PLoS One 2017;12:e0178992. doi: 10.1371/ journal.pone.0178992.
- 26. von Schacky CE, Sohn JH, Liu F, Ozhinsky E, Jungmann PM, Nardo L, et al. Development and validation of a multitask deep learning model for severity grading of hip osteoarthritis features on radiographs. radiology. 2020 Apr;295(1):136-45. doi: 10.1148/radiol.2020190925.
- 27. Jang SJ, Kunze KN, Vigdorchik JM, Jerabek SA, Mayman DJ, Sculco PK. John Charnley Award: Deep learning prediction of hip joint center on standard pelvis radiographs. J Arthroplasty 2022;37:S400-S407.e1. doi: 10.1016/j. arth.2022.03.033.
- 28. Peters CL, Erickson JA. Treatment of femoro-acetabular impingement with surgical dislocation and débridement in young adults. J Bone Joint Surg Am 2006;88:1735-41. doi: 10.2106/JBJS.E.00514.
- 29. Menge TJ, Briggs KK, Rahl MD, Philippon MJ. Hip arthroscopy for femoroacetabular impingement in adolescents: 10-year patient-reported outcomes. Am J Sports Med 2021;49:76-81. doi: 10.1177/0363546520973977.
APA | atalar e, Üreten K, Kanatlı U, Çiçeklidağ M, kaya İ, vural a, MARAŞ Y (2023). The diagnosis of femoroacetabular impingement can be made on pelvis radiographs using deep learning methods. , 298 - 304. 10.52312/jdrs.2023.996 |
Chicago | atalar ebru,Üreten Kemal,Kanatlı Ulunay,Çiçeklidağ Murat,kaya İbrahim,vural abdurrahman,MARAŞ YÜKSEL The diagnosis of femoroacetabular impingement can be made on pelvis radiographs using deep learning methods. (2023): 298 - 304. 10.52312/jdrs.2023.996 |
MLA | atalar ebru,Üreten Kemal,Kanatlı Ulunay,Çiçeklidağ Murat,kaya İbrahim,vural abdurrahman,MARAŞ YÜKSEL The diagnosis of femoroacetabular impingement can be made on pelvis radiographs using deep learning methods. , 2023, ss.298 - 304. 10.52312/jdrs.2023.996 |
AMA | atalar e,Üreten K,Kanatlı U,Çiçeklidağ M,kaya İ,vural a,MARAŞ Y The diagnosis of femoroacetabular impingement can be made on pelvis radiographs using deep learning methods. . 2023; 298 - 304. 10.52312/jdrs.2023.996 |
Vancouver | atalar e,Üreten K,Kanatlı U,Çiçeklidağ M,kaya İ,vural a,MARAŞ Y The diagnosis of femoroacetabular impingement can be made on pelvis radiographs using deep learning methods. . 2023; 298 - 304. 10.52312/jdrs.2023.996 |
IEEE | atalar e,Üreten K,Kanatlı U,Çiçeklidağ M,kaya İ,vural a,MARAŞ Y "The diagnosis of femoroacetabular impingement can be made on pelvis radiographs using deep learning methods." , ss.298 - 304, 2023. 10.52312/jdrs.2023.996 |
ISNAD | atalar, ebru vd. "The diagnosis of femoroacetabular impingement can be made on pelvis radiographs using deep learning methods". (2023), 298-304. https://doi.org/10.52312/jdrs.2023.996 |
APA | atalar e, Üreten K, Kanatlı U, Çiçeklidağ M, kaya İ, vural a, MARAŞ Y (2023). The diagnosis of femoroacetabular impingement can be made on pelvis radiographs using deep learning methods. Joint diseases and related surgery, 34(2), 298 - 304. 10.52312/jdrs.2023.996 |
Chicago | atalar ebru,Üreten Kemal,Kanatlı Ulunay,Çiçeklidağ Murat,kaya İbrahim,vural abdurrahman,MARAŞ YÜKSEL The diagnosis of femoroacetabular impingement can be made on pelvis radiographs using deep learning methods. Joint diseases and related surgery 34, no.2 (2023): 298 - 304. 10.52312/jdrs.2023.996 |
MLA | atalar ebru,Üreten Kemal,Kanatlı Ulunay,Çiçeklidağ Murat,kaya İbrahim,vural abdurrahman,MARAŞ YÜKSEL The diagnosis of femoroacetabular impingement can be made on pelvis radiographs using deep learning methods. Joint diseases and related surgery, vol.34, no.2, 2023, ss.298 - 304. 10.52312/jdrs.2023.996 |
AMA | atalar e,Üreten K,Kanatlı U,Çiçeklidağ M,kaya İ,vural a,MARAŞ Y The diagnosis of femoroacetabular impingement can be made on pelvis radiographs using deep learning methods. Joint diseases and related surgery. 2023; 34(2): 298 - 304. 10.52312/jdrs.2023.996 |
Vancouver | atalar e,Üreten K,Kanatlı U,Çiçeklidağ M,kaya İ,vural a,MARAŞ Y The diagnosis of femoroacetabular impingement can be made on pelvis radiographs using deep learning methods. Joint diseases and related surgery. 2023; 34(2): 298 - 304. 10.52312/jdrs.2023.996 |
IEEE | atalar e,Üreten K,Kanatlı U,Çiçeklidağ M,kaya İ,vural a,MARAŞ Y "The diagnosis of femoroacetabular impingement can be made on pelvis radiographs using deep learning methods." Joint diseases and related surgery, 34, ss.298 - 304, 2023. 10.52312/jdrs.2023.996 |
ISNAD | atalar, ebru vd. "The diagnosis of femoroacetabular impingement can be made on pelvis radiographs using deep learning methods". Joint diseases and related surgery 34/2 (2023), 298-304. https://doi.org/10.52312/jdrs.2023.996 |