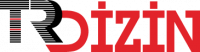
An alternative word embedding approach for knowledge representation in online consumers’ reviews
Yıl: 2023 Cilt: 29 Sayı: 3 Sayfa Aralığı: 220 - 229 Metin Dili: İngilizce DOI: 10.5505/pajes.2022.10369 İndeks Tarihi: 23-07-2023
An alternative word embedding approach for knowledge representation in online consumers’ reviews
Öz: Purchasing decisions in e-commerce shopping websites are highly influenced by online reviews. Although online reviews contain fine- grained consumers’ opinions that reflect their preferences towards products; an important challenge, is that the number of online reviews can be very huge for fast and effective analysis. Hence, discovering the thematic structure of documents plays an important role in analyzing online reviews. The proposed system in this paper aims to discover the main consumer interests in online reviews on Turkish e-commerce websites. For this aim, a novel hybrid method combining Latent Dirichlet Allocation (LDA) and word2vec is proposed. Finally, we compare the performance of our work with those of several state-of-the- art baselines on 7 datasets collected from well-known Turkish e- commerce websites. The experimental results show how our proposed approach was able to provide significantly improved performance over baselines. Besides, our method enables us to discover very specific topics complying with consumer interests.
Anahtar Kelime: Çevrimiçi kullanıcı yorumlarının bilgi temsili için alternatif bir kelime gömme yaklaşımı
Öz: E-ticaret alışveriş sitelerinde satın alma kararları, çevrimiçi yorumlardan oldukça etkilenir. Çevrimiçi yorumlar, ürünlere yönelik tercihleri yansıtan ayrıntılı tüketici görüşleri içerse de; önemli bir zorluk, çevrimiçi yorumların miktarının hızlı ve etkili bir analiz için çok büyük olabileceğidir. Bu nedenle, belgelerin tematik yapısını keşfetmek, çevrimiçi yorumları analiz etmede önemli bir rol oynar. Bu çalışmada önerilen sistem, Türk e-ticaret web sitelerindeki çevrimiçi yorumlardaki tüketicilerin ana ilgi alanlarını keşfetmeyi amaçlamaktadır. Bu amaçla, Gizli Dirichlet Ayırımı (GDA) ve word2vec'i birleştiren yeni bir hibrit yöntem önerilmiştir. Son olarak, çalışmamızın performansını, güncel yöntemlerin performansıyla tanınmış Türk e-ticaret sitelerinden toplanan 7 veri kümesi üzerinden karşılaştırdık. Deneysel sonuçlar, önerilen yaklaşımımızın güncel yöntemlere göre önemli ölçüde gelişmiş performans sağlayabildiğini göstermektedir. Ayrıca yöntemimiz, tüketici ilgi alanlarına uygun çok özel konuları keşfetmeyi sağlar.
Anahtar Kelime: Belge Türü: Makale Makale Türü: Araştırma Makalesi Erişim Türü: Erişime Açık
- [1] Zhu G, Wu Z, Wang Y, Cao S, Cao J. “Online purchase decisions for tourism e-commerce”. Electronic Commerce Research and Applications, 38, 1-13, 2019.
- [2] Huang Z, Benyoucef M. “The effects of social commerce esign on consumer purchase decision-making: An empirical study”. Electronic Commerce Research and Applications, 25, 40-58, 2017.
- [3] Griffiths TL, Steyvers M. Prediction and semantic association. Editors: Thrun S, Saul LK, Schölkopf B. Advances in neural information processing systems 16, Proceedings of the Seventeenth Annual Conference on Neural Information Processing Systems, 11-18, Vancouver, Canada, 2003.
- [4] Chang J, Gerrish S, Wang C, Boyd-Graber JL, Blei DM. Reading Tea Leaves: How Humans İnterpret Topic Models. Editors: Bengio Y, Schuurmans D, Lafferty J, Williams C, Culotta A. Advances in Neural İnformation Processing Systems 22, Proceedings of the Twenty-Third Annual Conference on Neural Information Processing Systems, 288-296, Vancouver, Canada, 2009.
- [5] Mikolov T, Chen K, Corrado G, Dean J. “Efficient estimation of word representations in vector space”. arXiv, 2022. https://arxiv.org/abs/1301.3781.
- [6] Yang Y, Chen C, Bao FS. “Aspect-based helpfulness prediction for online product reviews”. 2016 IEEE 28th International Conference on Tools with Artificial Intelligence, San Jose, CA, USA, 6-8 November 2016.
- [7] Guo Y, Barnes SJ, Jia Q. “Mining meaning from online ratings and reviews: Tourist satisfaction analysis using latent dirichlet allocation An empirical study”. Tourism Management, 59, 467-483, 2017.
- [8] Heng Y, Gao Z, Jiang Y, Chen X. “Exploring hidden factors behind online food shopping from Amazon reviews: A topic mining approach”. Journal of Retailing and Consumer Services, 42, 161-168, 2018.
- [9] Li X, Wu C, Mai F. “The effect of online reviews on product sales: A joint sentiment-topic analysis.”. Information & Management, 56, 172-184, 2019.
- [10] Mou J, Ren G, Qin C, Kurcz K. “An exploration of cross- border e-commerce consumer feedbacks: An LDA approach”. The Seventeenth Wuhan International Conference On E-Business, Wuhan, P.R. China, 25-27 May 2018.
- [11] Mou J, Ren G, Qin C, Kurcz K. “Understanding the topics of export cross-border e-commerce consumers feedback: an LDA approach”. Electronic Commerce Research, 19, 749-777, 2019.
- [12] Situmeang F, de Boer N, Zhang A. “Looking beyond the stars: A description of text mining technique to extract latent dimensions from online product reviews.”. International Journal of Market Research, 62, 195-215, 2020.
- [13] Xu X. “How do consumers in the sharing economy value sharing? Evidence from online reviews”. Decision Support Systems, 128, 1-13, 2020.
- [14] Lang C, Li M, Zhao L. “Understanding consumers’ online fashion renting experiences: A text-mining approach”. Sustainable Production and Consumption, 21, 132-144, 2020.
- [15] Das R, Zaheer M, Dyer C. Gaussian lda for topic models with Word embedding. Editors: Zong C, Strube M. the 53rd Annual Meeting of the Association for Computational Linguistics and the 7th International Joint Conference on Natural Language Processing, 795-804, Beijing, China, 2015.
- [16] Nguyen DQ, Billingsley R, Du L, Johnson M. “Improving topic models with latent feature word representations”. Transactions of the Association for Computational Linguistics, 3, 299-313, 2015.
- [17] Liu Y, Liu Z, Chua TS, Sun M. “Topical word embeddings”. Twenty-Ninth AAAI Conference on Artificial Intelligence, Austin, Texas, USA, 25-30 January 2015.
- [18] Niu L, Dai X, Zhang J, Chen J. “Topic2Vec: learning distributed representations of topics”. 2015 International Conference on Asian Language Processing, Suzhou, China, 24-25 October 2015.
- [19] Sridhar VKR. “Unsupervised topic modeling for short texts using distributed representations of words”. 1st Workshop on Vector Space Modeling for Natural Language Processing, Denver, Colorado, USA, 5 June 2015.
- [20] Wang Z, Ma L, Zhang Y. “A hybrid document feature extraction method using latent Dirichlet allocation and word2vec”. 2016 IEEE First International Conference on Data Science in Cyberspace, Changsha, China, June 2016.
- [21] Hu W, Tsujii J. A Latent Concept Topic Model for Robust Topic Inference Using Word Embeddings. Editors: Erk K, Smith NA. 54th Annual Meeting of the Association for Computational Linguistics, 380-386 Berlin, Germany, 2016.
- [22] Li S, Chua TS, Zhu J, Miao C. Generative Topic Embedding: A Continuous Representation of Documents. Editors: Erk K, Smith NA. 54th Annual Meeting of the Association for Computational Linguistics, 666-675, Berlin, Germany, 2016.
- [23] Moody CE. “Mixing dirichlet topic models and word embeddings to make lda2vec”. arXiv, 2022. https://arxiv.org/abs/1605.02019
- [24] Batmanghelich K, Saeedi A, Narasimhan K, Gershman S. Nonparametric Spherical Topic Modeling With Word Embeddings. Editors: Erk K, Smith NA. 54th Annual Meeting of the Association for Computational Linguistics, 537-542, Berlin, Germany, 2016.
- [25] Li C, Wang H, Zhang Z, Sun A, Ma Z. “Topic modeling for short texts with auxiliary word embeddings”. 39th International ACM SIGIR conference on Research and Development in Information Retrieval, Pisa, Italy, 17-21 July 2016.
- [26] Xun G, Li Y, Zhao WX, Gao J, Zhang A. “A correlated topic model using word embeddings”. Twenty-Sixth International Joint Conference on Artificial Intelligence, Melbourne, Canada, 19-25 August 2017.
- [27] Bicalho P, Pita M, Pedrosa G, Lacerda A, Pappa GL. “A general framework to expand short text for topic modeling”. Information Sciences, 393, 66-81, 2017.
- [28] Law J, Zhuo HH, He J, Rong E. “Ltsg: Latent topical skipgram for mutually learning topic model and vector representations”. arXiv, 2022. https://arxiv.org/abs/1702.07117
- [29] Garc ́ıa-Pablos A, Cuadros M, Rigau G. “W2VLDA: almost unsupervised system for aspect based sentiment analysis”. Expert Systems with Applications, 91, 127-137, 2018.
- [30] Zhao H, Du L, Buntine W, Zhou M. Inter and Intra Topic Structure Learning with Word Embeddings. Editors: Dy j, Krause A. 35th International Conference on Machine Learning, 5892-5901, Stockholm, Sweden, July 2018.
- [31] Shi T, Kang K, Choo J, Reddy CK. “Short-text topic modeling via non-negative matrix factorization enriched with local word-context correlations”. 2018 World Wide Web Conference, Lyon, France, 23-27 April 2018.
- [32] Viegas F, Canuto S, Gomes C, Luiz W, Rosa T, Ribas S, Rocha L, Gonçalves MA. “CluWords: Exploiting Semantic Word Clustering Representation for Enhanced Topic Modeling”. Twelfth ACM International Conference on Web Search and Data Mining, Melbourne, Canada, 11-15 February 2019.
- [33] Li D, Zhang J, Li P. TMSA: A Mutual Learning Model for Topic Discovery and Word Embedding. Editors: Berger -Wolf T, Chawla N. 2019 SIAM International Conference on Data Mining, 684-692, Calgary, Alberta, Canada, 2019.
- [34] Al-Janabi OM, Malim NHAH, Cheah YN. Aspect Categorization Using Domain-Trained Word Embedding and Topic Modelling. Editors: Zakaria Z, Ahmad R. Advances in Electronics Engineering, 191-198, Kuala Lumpur, Malaysia, December 2019.
- [35] Çoban Ö, Tümüklü Özyer G. “The impact of term weighting method on Twitter sentiment analysis”. Pamukkale University Journal of Engineering Sciences, 24(2), 283-291, 2018.
- [36] Akın MD, Akın AA. “Türk dilleri için açık kaynaklı doğal dil işleme kütüphanesi: ZEMBEREK”. Elektrik Mühendisliği, 431, 38-44, 2007.
- [37] Mykowiecka A, Marciniak M, Rychlik P. “Testing word embeddings for Polish”. Cognitive Studies, 17, 1-19, 2017.
- [38] Ekinci E. “Classification of Imbalanced Offensive Dataset - Sentence Generation for Minority Class with LSTM”. Sakarya University Journal of Computer and Information Sciences, 5(1), 121-133, 2022.
- [39] Guthrie D, Allison B, Liu W, Guthrie L, Wilks Y. “A closer look at skip-gram modelling”. Fifth International Conference on Language Resources and Evaluation, Genoa, Italy, 24-26 May 2006.
- [40] Ekinci E, Ilhan Omurca S. “Concept-LDA: Incorporating Babelfy into LDA for aspect extraction”. Journal of Information Science, 46, 406-418, 2020.
- [41] Wallach HM, Mimno DM, McCallum A. Rethinking LDA: Why Priors Matter. Editors: Bengio Y, Schuurmans D, Lafferty J, Williams C, Culotta A. Twenty-Third Annual Conference on Neural Information Processing Systems, 1973-1981, Vancouver, Canada, 2009.
- [42] Atıcı B, Ilhan Omurca S, Ekinci E. “Product aspect detection in customer complaints by using latent dirichlet allocation”. 2017 International Conference on Computer Science and Engineering, Antalya, Türkiye, 5-8 October 2017.
- [43] Ekinci E, Ilhan Omurca S. “NET-LDA: a novel topic modeling method based on semantic document similarity”. Turkish Journal of Electrical Engineering & Computer Sciences, 28, 2244-2260, 2020.
- [44] Blei DM, Ng AY, Jordan MI. “Latent dirichlet allocation”. Journal of Machine Learning Research, 3, 993-1022, 2003.
- [45] Salur MU, Aydın İ, Jamous M. “An ensemble approach for aspect term extraction in Turkish texts”. Pamukkale University Journal of Engineering Sciences, 28(5), 769-776 2022.
- [46] Nguyen DQ. “jLDADMM: A Java package for the LDA and DMM topic models”. arXiv, 2022. https://arxiv.org/abs/1808.03835
- [47] Yan X, Guo J, Lan Y, Cheng X. “A biterm topic model for short texts”. 22nd International Conference on World Wide Web, Rio de Janeiro, Brasil, 13-17May 2013.
- [48] Chen Z, Liu B. “Topic modeling using topics from many domains, lifelong learning and big data”. 31st International Conference on Machine Learning, Beijing, China, 21-26 June 2014.
- [49] Chen Z, Liu B. “Mining topics in documents: standing on the shoulders of big data”. 20th ACM SIGKDD International Conference on Knowledge Discovery and Data Mining, New York, USA, 24-27 August 2014.
- [50] Nikolenko SI, Koltcov S, Koltsova O. “Topic modelling for qualitative studies”. Journal of Information Science, 43, 88-102, 2017.
APA | Ekinci E, ilhan omurca s (2023). An alternative word embedding approach for knowledge representation in online consumers’ reviews. , 220 - 229. 10.5505/pajes.2022.10369 |
Chicago | Ekinci Ekin,ilhan omurca sevinç An alternative word embedding approach for knowledge representation in online consumers’ reviews. (2023): 220 - 229. 10.5505/pajes.2022.10369 |
MLA | Ekinci Ekin,ilhan omurca sevinç An alternative word embedding approach for knowledge representation in online consumers’ reviews. , 2023, ss.220 - 229. 10.5505/pajes.2022.10369 |
AMA | Ekinci E,ilhan omurca s An alternative word embedding approach for knowledge representation in online consumers’ reviews. . 2023; 220 - 229. 10.5505/pajes.2022.10369 |
Vancouver | Ekinci E,ilhan omurca s An alternative word embedding approach for knowledge representation in online consumers’ reviews. . 2023; 220 - 229. 10.5505/pajes.2022.10369 |
IEEE | Ekinci E,ilhan omurca s "An alternative word embedding approach for knowledge representation in online consumers’ reviews." , ss.220 - 229, 2023. 10.5505/pajes.2022.10369 |
ISNAD | Ekinci, Ekin - ilhan omurca, sevinç. "An alternative word embedding approach for knowledge representation in online consumers’ reviews". (2023), 220-229. https://doi.org/10.5505/pajes.2022.10369 |
APA | Ekinci E, ilhan omurca s (2023). An alternative word embedding approach for knowledge representation in online consumers’ reviews. Pamukkale Üniversitesi Mühendislik Bilimleri Dergisi, 29(3), 220 - 229. 10.5505/pajes.2022.10369 |
Chicago | Ekinci Ekin,ilhan omurca sevinç An alternative word embedding approach for knowledge representation in online consumers’ reviews. Pamukkale Üniversitesi Mühendislik Bilimleri Dergisi 29, no.3 (2023): 220 - 229. 10.5505/pajes.2022.10369 |
MLA | Ekinci Ekin,ilhan omurca sevinç An alternative word embedding approach for knowledge representation in online consumers’ reviews. Pamukkale Üniversitesi Mühendislik Bilimleri Dergisi, vol.29, no.3, 2023, ss.220 - 229. 10.5505/pajes.2022.10369 |
AMA | Ekinci E,ilhan omurca s An alternative word embedding approach for knowledge representation in online consumers’ reviews. Pamukkale Üniversitesi Mühendislik Bilimleri Dergisi. 2023; 29(3): 220 - 229. 10.5505/pajes.2022.10369 |
Vancouver | Ekinci E,ilhan omurca s An alternative word embedding approach for knowledge representation in online consumers’ reviews. Pamukkale Üniversitesi Mühendislik Bilimleri Dergisi. 2023; 29(3): 220 - 229. 10.5505/pajes.2022.10369 |
IEEE | Ekinci E,ilhan omurca s "An alternative word embedding approach for knowledge representation in online consumers’ reviews." Pamukkale Üniversitesi Mühendislik Bilimleri Dergisi, 29, ss.220 - 229, 2023. 10.5505/pajes.2022.10369 |
ISNAD | Ekinci, Ekin - ilhan omurca, sevinç. "An alternative word embedding approach for knowledge representation in online consumers’ reviews". Pamukkale Üniversitesi Mühendislik Bilimleri Dergisi 29/3 (2023), 220-229. https://doi.org/10.5505/pajes.2022.10369 |