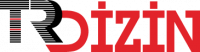
Value of fecal calprotectin in prediction of acute appendicitis based on a proposed model of machine learning
Yıl: 2023 Cilt: 29 Sayı: 6 Sayfa Aralığı: 655 - 662 Metin Dili: İngilizce DOI: 10.14744/tjtes.2023.10001 İndeks Tarihi: 31-07-2023
Value of fecal calprotectin in prediction of acute appendicitis based on a proposed model of machine learning
Öz: BACKGROUND: The aim of this study is to apply random forest (RF), one of the machine learning (ML) algorithms, to a dataset consisting of patients with a presumed diagnosis of acute appendicitis (AAp) and to reveal the most important factors associated with the diagnosis of AAp based on the variable importance. METHODS: An open-access dataset comparing two patient groups with (n=40) and without (n=44) AAp to predict biomarkers for AAp was used for this case−control study. RF was used for modeling the data set. The data were divided into two training and test dataset (80:20). Accuracy, balanced accuracy (BC), sensitivity, specificity, positive predictive value (PPV), and negative predictive value (NPV) performance metrics were appraised for model performance. RESULTS: Accuracy, BC, sensitivity, specificity, PPV, NPV, and F1 scores pertaining to the RF model were 93.8%, 93.8%, 87.5%, 100%, 100%, 88.9%, and 93.3%, respectively. Following the variable importance values regarding the model, the variables most associated with the diagnosis and prediction of AAp were fecal calprotectin (100 %), radiological imaging (89.9%), white blood test (51.8%), C-reactive protein (47.1%), from symptoms onset to the hospital visit (19.3%), patients age (18.4%), alanine aminotransferase levels >40 (<1%), fever (<1%), and nausea/vomiting (<1%), respectively. CONCLUSION: A prediction model was developed for AAp with the ML method in this study. Thanks to this model, biomarkers that predict AAp with high accuracy were determined. Thus, the decision-making process of clinicians for diagnosing AAp will be facilitated, and the risks of perforation and unnecessary operations will be minimized thanks to the timely diagnosis with high accuracy.
Anahtar Kelime: Önerilen bir makine öğrenimi modeline dayalı akut apandisit öngörüsünde fekal kalprotektinin değeri
Öz: AMAÇ: Bu çalışmanın amacı, makine öğrenmesi (ML) algoritmalarından biri olan Random Forest’i (RF) akut apandisit (AAp) ön tanısı olan hastalardan oluşan bir veri setine uygulamak ve AAp tanısı ile ilişkili en önemli faktörleri değişken önemliliğine göre ortaya koymaktır. GEREÇ VE YÖNTEM: Bu vaka-kontrol çalışmasında AAp için biyobelirteçleri tahmin etmek üzere AAp’si olan (n=40) ve olmayan (n=44) iki hasta grubunu karşılaştıran açık erişimli bir veri seti kullanıldı. Veri setinin modellenmesinde RF kullanıldı. Veriler eğitim ve test veri seti olmak üzere ikiye ayrıldı (80: 20). Model performansı için doğruluk, dengeli doğruluk, duyarlılık, özgüllük, pozitif tahmin değeri (PPV) ve negatif tahmin değeri (NPV) performans metrikleri değerlendirildi. BULGULAR: RF modeline ait doğruluk, dengeli doğruluk, duyarlılık, özgüllük, PPV, NPV ve F1 skorları sırasıyla %93.8, %93.8, %87.5, %100, %100, %88.9 ve %93.3 olarak hesaplandı. Modele ilişkin değişken önem değerlerinin ardından, AAp tanısı ve öngürüsü ile en çok ilişkili olan değişkenler sırasıyla fekal kalprotektin (%100), radyolojik görüntüleme (%89,9), beyaz kan testi (%51,8), C-reaktif protein (%47,1), semptomların hastane ziyaretinde başlaması (%19.3), hasta yaşı (%18.4), ALT düzeyleri >40 (<%1), ateş (< %1) ve mide bulantısı/kusma (<%1) olarak belirlendi. TARTIŞMA: Bu çalışmada ML yöntemi ile AAp için bir tahmin modeli geliştirildi. Bu model sayesinde AAp’i yüksek doğruluk ile öngören biyobelirteçler belirlendi. Böylece klinisyenlerin AAp tanısına karar verme süreci kolaylaşacak, yüksek doğruluk ile zamanında tanı konulabilmesi sayesinde perforasyon ve gereksiz ameliyat riskleri en aza indirilecektir.
Anahtar Kelime: Belge Türü: Makale Makale Türü: Araştırma Makalesi Erişim Türü: Erişime Açık
- 1. Akbulut S, Bahce ZS, Oztas T, Gumus S, Sogutcu N, Sakarya H, et al. Assessment of demographic, clinical and histopathological features of patients who underwent appendectomy due to a presumed diagnosis of acute appendicitis. Ulus Travma Acil Cerrahi Derg 2021;27:315–24.
- 2. Sarici KB, Akbulut S, Koc C, Tuncer A, Yılmaz S. Liver transplant versus non-liver transplant patients underwent appendectomy with presumed diagnosis of acute appendicitis: Case-control study. Ulus Travma Acil Cerrahi Derg 2020;26:705–12.
- 3. Wu J, Jiang H, Li S, Wu X, Wang P, Sawyer R, et al. Optimising the treatment for uncomplicated acute appendicitis (OPTIMA trial): A protocol for a multicentre, randomised, double-blinded placebo-controlled study. BMJ Open 2022;12:e057793.
- 4. Wickramasinghe DP, Xavier C, Samarasekera DN. The worldwide epidemiology of acute appendicitis: An analysis of the global health data exchange dataset. World J Surg 2021;45:1999–2008.
- 5. Ferris M, Quan S, Kaplan BS, Molodecky N, Ball CG, Chernoff GW, et al. The global incidence of appendicitis: A systematic review of population- based studies. Ann Surg 2017;266:237–41.
- 6. Ahmed HO, Muhedin R, Boujan A, Aziz AH, Hardi RA, Abdulla AA, et al. A five-year longitudinal observational study in morbidity and mortality of negative appendectomy in Sulaimani teaching Hospital/Kurdistan Region/Iraq. Sci Rep 2020;10:2028.
- 7. Su D, Li Q, Zhang T, Veliz P, Chen Y, He K, et al. Prediction of acute appendicitis among patients with undifferentiated abdominal pain at emergency department. BMC Med Res Methodol 2022;22:18.
- 8. Akbulut S, Koc C, Sahin TT, Sahin E, Tuncer A, Demyati K, et al. An investigation into the factors predicting acute appendicitis and perforated appendicitis. Ulus Travma Acil Cerrahi Derg 2021;27:434–42.
- 9. Jeon BG, Kim HJ, Heo SC. CT scan findings can predict the safety of delayed appendectomy for acute appendicitis. J Gastrointest Surg 2019;23:1856–66.
- 10. Yang Z, Sun F, Ai S, Wang J, Guan W, Liu S. Meta-analysis of studies comparing conservative treatment with antibiotics and appendectomy for acute appendicitis in the adult. BMC Surg 2019;19:110.
- 11. Akbulut S, Caliskan A, Ekin A, Yagmur Y. Left-sided acute appendicitis with situs inversus totalis: Review of 63 published cases and report of two cases. J Gastrointest Surg 2010;14:1422–8.
- 12. Kim J, Kim K, Kim J, Yoo J, Jeong W, Cho S, et al. The learning curve in diagnosing acute appendicitis with emergency sonography among novice emergency medicine residents. J Clin Ultrasound 2018;46:305–10.
- 13. Galai T, Beloosesky OZ, Scolnik D, Rimon A, Glatstein M. Misdiagnosis of acute appendicitis in children attending the emergency department: The experience of a large, tertiary care pediatric hospital. Eur J Pediatr Surg 2017;27:138–41.
- 14. Chang YJ, Chao HC, Kong MS, Hsia SH, Yan DC. Misdiagnosed acute appendicitis in children in the emergency department. Chang Gung Med J 2010;33:551–7.
- 15. Graff L, Russell J, Seashore J, Tate J, Elwell A, Prete M, et al. False negative and false positive errors in abdominal pain evaluation: Failure to diagnose acute appendicitis and unnecessary surgery. Acad Emerg Med 2000;7:1244–55.
- 16. Ahuja AS. The impact of artificial intelligence in medicine on the future role of the physician. PeerJ 2019;7:e7702.
- 17. Davenport T, Kalakota R. The potential for artificial intelligence in healthcare. Future Healthc J 2019;6:94–8.
- 18. Mijwil MM, Abttan RA. Artificial intelligence: A survey on evolution and future trends. Asian J Appl Sci 2021;9:87–92.
- 19. Polikar R. Ensemble Learning. In: Cha Z, Yunqian M, editors. Ensemble Machine Learning. 1st ed. New York: Springer; 2012. p. 1–34.
- 20. Shin B, Chokshi FH, Lee T, Choi JD. Classification of Radiology Reports using Neural Attention Models. In: Proceedings of the 2017 International Joint Conference on Neural Networks (IJCNN). United States: IEEE; 2017. p. 4363–70.
- 21. Luz CF, Vollmer M, Decruyenaere J, Nijsten MW, Glasner C, Sinha B. Machine learning in infection management using routine electronic health records: Tools, techniques, and reporting of future technologies. Clin Microbiol Infect 2020;26:1291–9.
- 22. Ekelik H, Altas D. Classification of potential residential buyers by using random forest method taking advantage of digital advertising data. J Res Econ 2019;3:28–45.
- 23. Bestil Hİ, Guvensan MA. A novel multi-tier filtering architecture and smart SMS box for classification of Turkish short messages. Int J Adv Eng Pure Sci 2019;31:17–28.
- 24. Korkmaz D, Çelik HE, Kapar M. Botnet detection by using classification and regression trees with random forest algorithms: Example of Van Yüzüncü Yil University. Yuzuncu Yil Univ J Inst Nat Appl Sci 2018;23:297–307.
- 25. Erdem F, Derinpinar MA, Nasirzadehdizaji R, Selen O, Seker DZ, Bayram B. Coastline extraction by using random forest method; a case study of Istanbul. J Geomatics 2018;3:100–7.
- 26. Zhou W, Qiao H, Yuan W, Yao Z, Liu K, Wang J, et al. Diagnostic utility of fecal calprotectin in patients presenting to the emergency department with suspected acute appendicitis. Am J Emerg Med 2020;38:2303–7.
- 27. Breiman L. Random forests. Machine Learning 2001;45:5–32.
- 28. Cutler A, Cutler DR, Stevens JR. Random forests. In: Cha Z, Yunqian M, editors. Ensemble Machine Learning. 1st edition. New York: Springer; 2012. p. 157–75.
- 29. Mather PM, Koch M. Computer Processing of Remotely-sensed Images: An Introduction. 4th edition. United States: John Wiley and Sons; 2011. 30. Pal M. Random forest classifier for remote sensing classification. Int J Remote Sens 2005;26:217–22.
- 31. Arslan AK, Yasar S, Colak C, Yologlu S. WSSPAS: An interactive web application for sample size and power analysis with R using shiny. Turk Klin J Biostat 2018;10:224–46.
- 32. Rosen MP, Ding A, Blake MA, Baker ME, Cash BD, Fidler JL, et al. ACR Appropriateness Criteria® right lower quadrant pain--suspected appendicitis. J Am Coll Radiol 2011;8:749–55.
- 33. Akbar F, Yousuf M, Morgan RJ, Maw A. Changing management of suspected appendicitis in the laparoscopic era. Ann R Coll Surg Engl 2010;92:65–8.
- 34. Lim J, Pang Q, Alexander R. One year negative appendicectomy rates at a district general hospital: A retrospective cohort study. Int J Surg 2016;31:1–4.
- 35. Noureldin K, Hatim Ali AA, Issa M, Shah H, Ayantunde B, Ayantunde A. Negative appendicectomy rate: Incidence and predictors. Cureus 2022;14:e21489.
- 36. Yu KH, Beam AL, Kohane IS. Artificial intelligence in healthcare. Nat Biomed Eng 2018;2:719–31.
- 37. Ricciuto A, Griffiths AM. Clinical value of fecal calprotectin. Crit Rev Clin Lab Sci 2019;56:307–20.
- 38. Ambe PC, Godde D, Bonicke L, Papadakis M, Storkel S, Zirngibl H. Calprotectin could be a potential biomarker for acute appendicitis. J Transl Med 2016;14:107.
- 39. Cikot M, Peker KD, Bozkurt MA, Kocatas A, Kones O, Binboga S, et al. Plasma calprotectin level: Usage in distinction of uncomplicated from complicated acute appendicitis. World J Emerg Surg 2016;11:7.
- 40. Akmese OF, Dogan G, Kor H, Erbay H, Demir E. The use of machine learning approaches for the diagnosis of acute appendicitis. Emerg Med Int 2020;2020:7306435.
- 41. Aydin E, Turkmen İU, Namli G, Ozturk C, Esen AB, Eray YN, et al. A novel and simple machine learning algorithm for preoperative diagnosis of acute appendicitis in children. Pediatr Surg Int 2020;36:735–42.
- 42. Mijwil MM, Aggarwal K. A diagnostic testing for people with appendicitis using machine learning techniques. Multimed Tools Appl 2022;81:7011–23.
- 43. Gunasingha RM, Grey SF, Munoz B, Schobel S, Lee J, Erwin C, et al. To scan or not to scan: Development of a clinical decision support tool to determine if imaging would aid in the diagnosis of appendicitis. World J Surg 2021;45:3056–64.
APA | KÜÇÜKAKÇALI Z, Akbulut S, ÇOLAK C (2023). Value of fecal calprotectin in prediction of acute appendicitis based on a proposed model of machine learning. , 655 - 662. 10.14744/tjtes.2023.10001 |
Chicago | KÜÇÜKAKÇALI ZEYNEP,Akbulut Sami,ÇOLAK Cemil Value of fecal calprotectin in prediction of acute appendicitis based on a proposed model of machine learning. (2023): 655 - 662. 10.14744/tjtes.2023.10001 |
MLA | KÜÇÜKAKÇALI ZEYNEP,Akbulut Sami,ÇOLAK Cemil Value of fecal calprotectin in prediction of acute appendicitis based on a proposed model of machine learning. , 2023, ss.655 - 662. 10.14744/tjtes.2023.10001 |
AMA | KÜÇÜKAKÇALI Z,Akbulut S,ÇOLAK C Value of fecal calprotectin in prediction of acute appendicitis based on a proposed model of machine learning. . 2023; 655 - 662. 10.14744/tjtes.2023.10001 |
Vancouver | KÜÇÜKAKÇALI Z,Akbulut S,ÇOLAK C Value of fecal calprotectin in prediction of acute appendicitis based on a proposed model of machine learning. . 2023; 655 - 662. 10.14744/tjtes.2023.10001 |
IEEE | KÜÇÜKAKÇALI Z,Akbulut S,ÇOLAK C "Value of fecal calprotectin in prediction of acute appendicitis based on a proposed model of machine learning." , ss.655 - 662, 2023. 10.14744/tjtes.2023.10001 |
ISNAD | KÜÇÜKAKÇALI, ZEYNEP vd. "Value of fecal calprotectin in prediction of acute appendicitis based on a proposed model of machine learning". (2023), 655-662. https://doi.org/10.14744/tjtes.2023.10001 |
APA | KÜÇÜKAKÇALI Z, Akbulut S, ÇOLAK C (2023). Value of fecal calprotectin in prediction of acute appendicitis based on a proposed model of machine learning. Ulusal Travma ve Acil Cerrahi Dergisi, 29(6), 655 - 662. 10.14744/tjtes.2023.10001 |
Chicago | KÜÇÜKAKÇALI ZEYNEP,Akbulut Sami,ÇOLAK Cemil Value of fecal calprotectin in prediction of acute appendicitis based on a proposed model of machine learning. Ulusal Travma ve Acil Cerrahi Dergisi 29, no.6 (2023): 655 - 662. 10.14744/tjtes.2023.10001 |
MLA | KÜÇÜKAKÇALI ZEYNEP,Akbulut Sami,ÇOLAK Cemil Value of fecal calprotectin in prediction of acute appendicitis based on a proposed model of machine learning. Ulusal Travma ve Acil Cerrahi Dergisi, vol.29, no.6, 2023, ss.655 - 662. 10.14744/tjtes.2023.10001 |
AMA | KÜÇÜKAKÇALI Z,Akbulut S,ÇOLAK C Value of fecal calprotectin in prediction of acute appendicitis based on a proposed model of machine learning. Ulusal Travma ve Acil Cerrahi Dergisi. 2023; 29(6): 655 - 662. 10.14744/tjtes.2023.10001 |
Vancouver | KÜÇÜKAKÇALI Z,Akbulut S,ÇOLAK C Value of fecal calprotectin in prediction of acute appendicitis based on a proposed model of machine learning. Ulusal Travma ve Acil Cerrahi Dergisi. 2023; 29(6): 655 - 662. 10.14744/tjtes.2023.10001 |
IEEE | KÜÇÜKAKÇALI Z,Akbulut S,ÇOLAK C "Value of fecal calprotectin in prediction of acute appendicitis based on a proposed model of machine learning." Ulusal Travma ve Acil Cerrahi Dergisi, 29, ss.655 - 662, 2023. 10.14744/tjtes.2023.10001 |
ISNAD | KÜÇÜKAKÇALI, ZEYNEP vd. "Value of fecal calprotectin in prediction of acute appendicitis based on a proposed model of machine learning". Ulusal Travma ve Acil Cerrahi Dergisi 29/6 (2023), 655-662. https://doi.org/10.14744/tjtes.2023.10001 |