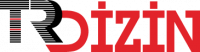
Heterojen Müşteri Segmentleri ve Talep Belirsizliği Varsayımlarıyla Ağ Tasarımı Problemi İçin Bir Model Önerisi
Yıl: 2023 Cilt: 58 Sayı: 3 Sayfa Aralığı: 1945 - 1971 Metin Dili: Türkçe DOI: 10.15659/3.sektor-sosyal-ekonomi.23.08.2152 İndeks Tarihi: 26-09-2023
Heterojen Müşteri Segmentleri ve Talep Belirsizliği Varsayımlarıyla Ağ Tasarımı Problemi İçin Bir Model Önerisi
Öz: Bu çalışmada, talep belirsizliği varsayımı altında aralarında yatay iş birliği bulunan üst düzey müşteriler, orta düzey müşteriler ve temel düzey müşteriler şeklinde heterojen müşteri segmentlerine sahip, dağıtım kanalı seçimi, dağıtım planlaması, envanter, aktarma ve atama kararlarını içeren ağ tasarımı problemine yönelik bir doğrusal tam sayılı programlama modeli geliştirilmiştir. Geliştirilen matematiksel modelin sunulmasının ardından, ele alınan ağ tasarımı problemi genel amaçlı bir çözücü kullanılarak bir örnek olay ve bu örnek olaydan türetilen farklı senaryolar için çözülmüştür. Ardından, örnek olay çözümü ve senaryo çözümlerine yönelik nümerik analizler yapılmış, modelin işlevselliği gösterilmiş ve belirlenen temel performans kriterleri üzerinden değerlendirmeler yapılmıştır. Nümerik analizler sonucunda, benzer karar süreçlerini içeren durumlarla karşılaşan karar vericiler için müşteri segmentasyonu ve yatay iş birliğinin önemi ortaya koyulmuştur. Üst düzey müşteriler arasında ve üst düzey müşterilerden orta düzey müşterilere ürün aktarımı seçeneğinin bulunmamasının toplam maliyeti yükselttiği görülmüştür. Bu sebeple, tedarik zinciri elemanları arasında kurulan yatay iş birliklerinin toplam ağ maliyetinde iyileşme sağladığı söylenebilmektedir.
Anahtar Kelime: A Model Proposal For The Network Design Problem With Heterogeneous Customer Segments And Demand Uncertainty Assumptions
Öz: This study addresses a network design problem with horizontal collaboration between heterogeneous customer segments consisting of high-tier customers, mid-tier customers, and basic customers under the assumption of demand uncertainty. A linear integer programming model is developed for the problem. The model involves distribution channel selection, distribution planning, inventory, transshipment and assignment decisions. After the presentation of the developed mathematical model, the network design problem is solved using a general purpose solver for a case study and different scenarios derived from this case study. Then, numerical analyses were performed for the case study and scenario solutions, the functionality of the model was demonstrated and evaluations were made based on the key performance criteria. As a result of the numerical analysis, some crucial outputs have been revealed for decision makers confronted with similar decision processes. It has been observed that the absence of the option to transshipment products among top customers and from high tier customers to mid tier customers increases the total cost. As a result, horizontal cooperation built across supply chain actors can be said to improve total network cost.
Anahtar Kelime: Belge Türü: Makale Makale Türü: Araştırma Makalesi Erişim Türü: Erişime Açık
- Adams, F. G., Richey Jr, R. G., Autry, C. W., Morgan, T. R., & Gabler, C. B. (2014). Supply chain collaboration, integration, and relational technology: How complex operant resources increase performance outcomes. Journal of Business Logistics, 35(4), 299-317.
- Aghalari, A., Nur, F., & Marufuzzaman, M. (2020). A Bender’s based nested decomposition algorithm to solve a stochastic inland waterway port management problem considering perishable product. International Journal of Production Economics, 229, 107863.
- Aghdaie, M. H., Tafreshi, P. F., & Behzadian, M. (2014). Customer-oriented benefit segmentation: an integrated approach. International Journal of Business Innovation and Research, 8(2), 168-189.
- Alamdari, N. E., & Savard, G. (2021). Deep reinforcement learning in seat inventory control problem: an action generation approach. Journal of Revenue and Pricing Management, 1-14.
- Alavidoost, M. H., Tarimoradi, M., & Zarandi, M. F. (2018). Bi-objective mixed-integer nonlinear programming for multi-commodity tri-echelon supply chain networks. Journal of intelligent manufacturing, 29, 809-826.
- Altıparmak, F., Gen, M., Lin, L., & Karaoglan, I. (2009). A steady-state genetic algorithm for multi-product supply chain network design. Computers & industrial engineering, 56(2), 521-537.
- Basso, F., D'Amours, S., Rönnqvist, M., & Weintraub, A. (2019). A survey on obstacles and difficulties of practical implementation of horizontal collaboration in logistics. International Transactions in Operational Research, 26(3), 775-793.
- Battarra, M., Balcik, B., & Xu, H. (2018). Disaster preparedness using risk-assessment methods from earthquake engineering. European journal of operational research, 269(2), 423-435.
- Bayram, V., Gzara, F., & Elhedhli, S. (2019). Joint capacity, inventory, and demand allocation decisions in manufacturing systems. IISE Transactions, 51(3), 248-265.
- Björnfot, A., & Torjussen, L. (2012). Extent and effect of horizontal supply chain collaboration among construction SME. Journal of Engineering, Project, and Production Management, 2(1), 47-55.
- Chen, Z & Rossi, R 2017, 'Single item stochastic lot sizing problem considering capital flow and business overdraft', Paper presented at the 8th International Workshop on Lot Sizing, Glasgow, United Kingdom, 23/08/17 - 25/08/17 pp. 69-73.Chopra, S., & Meindl, P. (2013). Supply Chain Management: Strategy, planning, and Operation (5th ed.). Pearson Education. Cordeau, J. F., Pasin, F., & Solomon, M. M. (2006). An integrated model for logistics network design. Annals of operations research, 144(1), 59-82.
- Dai, Y., Chao, X., Fang, S. C., & Nuttle, H. L. (2005). Game theoretic analysis of a distribution system with customer market search. Annals of Operations Research, 135(1), 223-228.
- Dong, J. X., & Song, D. P. (2009). Container fleet sizing and empty repositioning in liner shipping systems. Transportation Research Part E: Logistics and Transportation Review, 45(6), 860-877.
- Ehsani, B., Karimi, H., Bakhshi, A., Aghsami, A., & Rabbani, M. (2023). Designing humanitarian logistics network for managing epidemic outbreaks in disasters using Internet-of-Things. A case study: An earthquake in Salas-e-Babajani city. Computers & industrial engineering, 175, 108821.
- Esfandiari, N., & Seifbarghy, M. (2013). Modeling a stochastic multi-objective supplier quota allocation problem with price-dependent ordering. Applied Mathematical Modelling, 37(8), 5790-5800.
- Fakhrzad, M. B., & Goodarzian, F. (2019). A fuzzy multi-objective programming approach to develop a green closed-loop supply chain network design problem under uncertainty: modifications of imperialist competitive algorithm. RAIRO-Operations Research, 53(3), 963-990.
- Gebennini, E., Gamberini, R., & Manzini, R. (2009). An integrated production–distribution model for the dynamic location and allocation problem with safety stock optimization. International Journal of Production Economics, 122(1), 286-304.
- Ghezavati, V. R., Jabal-Ameli, M. S., & Makui, A. (2009). A new heuristic method for distribution networks considering service level constraint and coverage radius. Expert Systems with Applications, 36(3), 5620-5629.
- Gou, Q., Zhang, J., Liang, L., Huang, Z., & Ashley, A. (2014). Horizontal cooperative programmes and cooperative advertising. International Journal of Production Research, 52(3), 691-712.
- Guo, P., Liu, F., & Wang, Y. (2020). Pre positioning and Deployment of Reserved Inventories in a Supply Network: Structural Properties. Production and Operations Management, 29(4), 893-906.
- Habibi, M. K., Allaoui, H., & Goncalves, G. (2018). Collaborative hub location problem under cost uncertainty. Computers & Industrial Engineering, 124, 393-410.
- Han, C., & Damrongwongsiri, M. (2005). Stochastic modeling of a two echelon multiple sourcing supply chain system with genetic algorithm. Journal of Manufacturing Technology Management, 16(1), 87-108. Han, G., Dong, M., & Shao, X. (2011). A stochastic dynamic programming approach-based yield management with substitution and uncertainty in semiconductor manufacturing. Computers & Mathematics with Applications, 61(4), 1241-1253.
- Han, G., Dong, M., & Shao, X. (2012). Yield management with downward substitution and uncertainty demand in semiconductor manufacturing. International Journal of Production Research, 50(3), 743-756.
- Hemmati, M., & Pasandideh, S. H. R. (2021). A bi-objective supplier location, supplier selection and order allocation problem with green constraints: scenario-based approach. Journal of Ambient Intelligence and Humanized Computing, 12, 8205-8228.
- Hwang, H., Jung, T., & Suh, E. (2004). An LTV model and customer segmentation based on customer value: a case study on the wireless telecommunication industry. Expert systems with applications, 26(2), 181-188.
- Jackson, P. L., Muckstadt, J. A., & Li, Y. (2019). Multiperiod stock allocation via robust optimization. Management Science, 65(2), 794-818.
- Juhari, T., & Juarna, A. (2022). Implementation RFM Analysis Model For Customer Segmentation Using The K-Means Algorithm Case Study XYZ Online Bookstore. Explore, 12(1), 107-118.
- Kim, J. S., Jeon, E., Noh, J., & Park, J. H. (2019). A model and an algorithm for a large-scale sustainable supplier selection and order allocation problem. Mathematics, 6(12), 325.
- Klosterhalfen, S. T., Kallrath, J., & Fischer, G. (2014). Rail car fleet design: Optimization of structure and size. International Journal of Production Economics, 157, 112-119.
- Li, X. (2013). An integrated modeling framework for design of logistics networks with expedited shipment services. Transportation Research Part E: Logistics and Transportation Review, 56, 46-63.
- Lin, Y. S., & Wang, K. J. (2018). A two-stage stochastic optimization model for warehouse configuration and inventory policy of deteriorating items. Computers & Industrial Engineering, 120, 83-93.
- Lv, Y., Wang, G., & Tang, L. (2014). Scenario-based modeling approach and scatter search algorithm for the stochastic slab allocation problem in steel industry. ISIJ international, 54(6), 1324-1333.
- Maji, A., Bhunia, A. K., & Mondal, S. K. (2022). A production-reliability-inventory model for a series-parallel system with mixed strategy considering shortage, warranty period, credit period in crisp and stochastic sense. OPSEARCH, 59(3), 862-907.
- Manupati, V. K., Schoenherr, T., Wagner, S. M., Soni, B., Panigrahi, S., & Ramkumar, M. (2021). Convalescent plasma bank facility location-allocation problem for COVID-19. Transportation Research Part E: Logistics and Transportation Review, 156, 102517.
- McDonald, M. (2012). Market segmentation: How to do it and how to profit from it. John Wiley & Sons.
- Memari, A., Ahmad, R., Rahim, A. R. A., & Hassan, A. (2018). Optimizing a Just-In-Time logistics network problem under fuzzy supply and demand: two parameter-tuned metaheuristics algorithms. Neural Computing and Applications, 30, 3221-3233.
- Mousavi, S. M., Pardalos, P. M., Niaki, S. T. A., Fügenschuh, A., & Fathi, M. (2019). Solving a continuous periodic review inventory-location allocation problem in vendor-buyer supply chain under uncertainty. Computers & Industrial Engineering, 128, 541-552.
- Natarajan, K. V., & Swaminathan, J. M. (2017). Multi treatment inventory allocation in humanitarian health settings under funding constraints. Production and Operations Management, 26(6), 1015-1034.
- Paksoy, T., Pehlivan, N., & Ozceylan, E. (2010). Application of Fuzzy mathematical programming approach to the aggregate production/distribution planning in a supply chain network problem. Scientific research and Essays, 5(22), 3384-3397.
- Papadopoulos, H. T., & Vidalis, M. I. (2001). Minimizing WIP inventory in reliable production lines. International Journal of Production Economics, 70(2), 185-197.
- Pasandideh, S. H. R., Niaki, S. T. A., & Asadi, K. (2015). Bi-objective optimization of a multi-product multi-period three-echelon supply chain problem under uncertain environments: NSGA-II and NRGA. Information Sciences, 292, 57-74.
- Patne, K., Shukla, N., Kiridena, S., & Tiwari, M. K. (2018). Solving closed-loop supply chain problems using game theoretic particle swarm optimisation. International Journal of Production Research, 56(17), 5836-5853.
- Ramezani, M., Kimiagari, A. M., & Karimi, B. (2015). Interrelating physical and financial flows in a bi-objective closed-loop supply chain network problem with uncertainty. Scientia Iranica E, 22(3), 1278-1293.
- Rezaei, A., Shahedi, T., Aghsami, A., Jolai, F., & Feili, H. (2021). Optimizing a bi-objective location-allocation-inventory problem in a dual-channel supply chain network with stochastic demands. RAIRO-Operations Research, 55(5), 3245-3279.
- Rushton, A., Croucher, P., & Baker, P. (2022). The handbook of logistics and distribution management: Understanding the supply chain. Kogan Page Publishers.
- Schulz, S. F., & Blecken, A. (2010). Horizontal cooperation in disaster relief logistics: benefits and impediments. International Journal of Physical Distribution & Logistics Management, 40(8/9), 636-656.
- Simatupang, T. M., & Sridharan, R. (2002). The collaborative supply chain. The international journal of logistics management, 13(1), 15-30.
- Soysal, M., Bloemhof-Ruwaard, J. M., & Van Der Vorst, J. G. (2014). Modelling food logistics networks with emission considerations: The case of an international beef supply chain. International Journal of Production Economics, 152, 57-70.
- Soysal, M., Bloemhof-Ruwaard, J. M., Haijema, R., & Van Der Vorst, J. G. (2015). Modeling an inventory routing problem for perishable products with environmental considerations and demand uncertainty. International Journal of Production Economics, 164, 118-133.
- Soysal, M., Bloemhof-Ruwaard, J. M., Haijema, R., & van der Vorst, J. G. (2018). Modeling a green inventory routing problem for perishable products with horizontal collaboration. Computers & Operations Research, 89, 168-182.
- Soysal, M., Çimen, M., Belbağ, S., & Toğrul, E. (2019). A review on sustainable inventory routing. Computers & Industrial Engineering, 132, 395-411.
- Soysal, M., Belbağ, S., & Erişkan, S. (2022). Horizontal Collaboration among SMEs through a Supply and Distribution Cooperative. Open Transportation Journal, 16.
- Soysal, M., Koç, Ç., Çimen, M., & İbiş, M. (2023). Managing returnable transport items in a vendor managed inventory system. Socio-Economic Planning Sciences, 86, 101504.
- Swaminathan, J. M., & Srinivasan, R. (1999). Managing individual customer service constraints under stochastic demand. Operations research letters, 24(3), 115-125.
- Syarif, A., Yun, Y., & Gen, M. (2002). Study on multi-stage logistic chain network: a spanning tree-based genetic algorithm approach. Computers & Industrial Engineering, 43(1-2), 299-314.
- Şentürk, H., & Selçuk, A. L. P. (2023). Perakende Sektöründe RFM Analizi ile Müşteri Segmentasyonu. İstanbul Ticaret Üniversitesi Girişimcilik Dergisi, 7(13), 1-10.
- Taghavi, S. M., Ghezavati, V., Bidhandi, H. M., & Al-e-Hashem, S. M. J. M. (2022). Green-Resilient Supplier Selection and Order Allocation Under Disruption by Utilizing Conditional Value at Risk: Mixed Response Strategies. Process Integration and Optimization for Sustainability, 1-22.
- Tanonkou, G. A., Benyoucef, L., & Xie, X. (2008). A scenario analysis of a location problem with uncertain demand. International Journal of Computer Applications in Technology, 32(4), 290-297.
- Tikani, H., Honarvar, M., & Mehrjerdi, Y. Z. (2018). Developing an integrated hub location and revenue management model considering multi-classes of customers in the airline industry. Computational and Applied Mathematics, 37, 3334-3364.
- Topaloglu, H., & Kunnumkal, S. (2006). Approximate dynamic programming methods for an inventory allocation problem under uncertainty. Naval Research Logistics (NRL), 53(8), 822-841.
- Wu, Y., Wang, J., & Li, C. (2019). Decisions of supply chain considering chain-to-chain competition and service negative spillover effect. Sustainability, 11(6), 1612.
- Zheng, M., Du, N., Zhao, H., Huang, E., & Wu, K. (2021). A study on the optimal inventory allocation for clinical trial supply chains. Applied Mathematical Modelling, 98, 161-184.
- Zhou, Y., Zhao, L., Zhao, X., & Jiang, J. (2011). A supplier selection and order allocation problem with stochastic demands. International Journal of Systems Science, 42(8), 1323-1338.
APA | Kazanç H, Yavrucu E, SOYSAL M (2023). Heterojen Müşteri Segmentleri ve Talep Belirsizliği Varsayımlarıyla Ağ Tasarımı Problemi İçin Bir Model Önerisi. , 1945 - 1971. 10.15659/3.sektor-sosyal-ekonomi.23.08.2152 |
Chicago | Kazanç Hande Cansın,Yavrucu Erencan,SOYSAL MEHMET Heterojen Müşteri Segmentleri ve Talep Belirsizliği Varsayımlarıyla Ağ Tasarımı Problemi İçin Bir Model Önerisi. (2023): 1945 - 1971. 10.15659/3.sektor-sosyal-ekonomi.23.08.2152 |
MLA | Kazanç Hande Cansın,Yavrucu Erencan,SOYSAL MEHMET Heterojen Müşteri Segmentleri ve Talep Belirsizliği Varsayımlarıyla Ağ Tasarımı Problemi İçin Bir Model Önerisi. , 2023, ss.1945 - 1971. 10.15659/3.sektor-sosyal-ekonomi.23.08.2152 |
AMA | Kazanç H,Yavrucu E,SOYSAL M Heterojen Müşteri Segmentleri ve Talep Belirsizliği Varsayımlarıyla Ağ Tasarımı Problemi İçin Bir Model Önerisi. . 2023; 1945 - 1971. 10.15659/3.sektor-sosyal-ekonomi.23.08.2152 |
Vancouver | Kazanç H,Yavrucu E,SOYSAL M Heterojen Müşteri Segmentleri ve Talep Belirsizliği Varsayımlarıyla Ağ Tasarımı Problemi İçin Bir Model Önerisi. . 2023; 1945 - 1971. 10.15659/3.sektor-sosyal-ekonomi.23.08.2152 |
IEEE | Kazanç H,Yavrucu E,SOYSAL M "Heterojen Müşteri Segmentleri ve Talep Belirsizliği Varsayımlarıyla Ağ Tasarımı Problemi İçin Bir Model Önerisi." , ss.1945 - 1971, 2023. 10.15659/3.sektor-sosyal-ekonomi.23.08.2152 |
ISNAD | Kazanç, Hande Cansın vd. "Heterojen Müşteri Segmentleri ve Talep Belirsizliği Varsayımlarıyla Ağ Tasarımı Problemi İçin Bir Model Önerisi". (2023), 1945-1971. https://doi.org/10.15659/3.sektor-sosyal-ekonomi.23.08.2152 |
APA | Kazanç H, Yavrucu E, SOYSAL M (2023). Heterojen Müşteri Segmentleri ve Talep Belirsizliği Varsayımlarıyla Ağ Tasarımı Problemi İçin Bir Model Önerisi. Üçüncü Sektör Sosyal Ekonomi, 58(3), 1945 - 1971. 10.15659/3.sektor-sosyal-ekonomi.23.08.2152 |
Chicago | Kazanç Hande Cansın,Yavrucu Erencan,SOYSAL MEHMET Heterojen Müşteri Segmentleri ve Talep Belirsizliği Varsayımlarıyla Ağ Tasarımı Problemi İçin Bir Model Önerisi. Üçüncü Sektör Sosyal Ekonomi 58, no.3 (2023): 1945 - 1971. 10.15659/3.sektor-sosyal-ekonomi.23.08.2152 |
MLA | Kazanç Hande Cansın,Yavrucu Erencan,SOYSAL MEHMET Heterojen Müşteri Segmentleri ve Talep Belirsizliği Varsayımlarıyla Ağ Tasarımı Problemi İçin Bir Model Önerisi. Üçüncü Sektör Sosyal Ekonomi, vol.58, no.3, 2023, ss.1945 - 1971. 10.15659/3.sektor-sosyal-ekonomi.23.08.2152 |
AMA | Kazanç H,Yavrucu E,SOYSAL M Heterojen Müşteri Segmentleri ve Talep Belirsizliği Varsayımlarıyla Ağ Tasarımı Problemi İçin Bir Model Önerisi. Üçüncü Sektör Sosyal Ekonomi. 2023; 58(3): 1945 - 1971. 10.15659/3.sektor-sosyal-ekonomi.23.08.2152 |
Vancouver | Kazanç H,Yavrucu E,SOYSAL M Heterojen Müşteri Segmentleri ve Talep Belirsizliği Varsayımlarıyla Ağ Tasarımı Problemi İçin Bir Model Önerisi. Üçüncü Sektör Sosyal Ekonomi. 2023; 58(3): 1945 - 1971. 10.15659/3.sektor-sosyal-ekonomi.23.08.2152 |
IEEE | Kazanç H,Yavrucu E,SOYSAL M "Heterojen Müşteri Segmentleri ve Talep Belirsizliği Varsayımlarıyla Ağ Tasarımı Problemi İçin Bir Model Önerisi." Üçüncü Sektör Sosyal Ekonomi, 58, ss.1945 - 1971, 2023. 10.15659/3.sektor-sosyal-ekonomi.23.08.2152 |
ISNAD | Kazanç, Hande Cansın vd. "Heterojen Müşteri Segmentleri ve Talep Belirsizliği Varsayımlarıyla Ağ Tasarımı Problemi İçin Bir Model Önerisi". Üçüncü Sektör Sosyal Ekonomi 58/3 (2023), 1945-1971. https://doi.org/10.15659/3.sektor-sosyal-ekonomi.23.08.2152 |