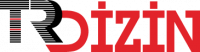
Investigation of Variables Affecting PISA Reading Comprehension Achievement Levels of Countries with Different Levels of Achievement with CRT and RF Methods
Yıl: 2023 Cilt: 20 Sayı: 2 Sayfa Aralığı: 459 - 483 Metin Dili: İngilizce DOI: 10.33711/yyuefd.1221541 İndeks Tarihi: 27-09-2023
Investigation of Variables Affecting PISA Reading Comprehension Achievement Levels of Countries with Different Levels of Achievement with CRT and RF Methods
Öz: The aim of this research is to determine the important variables that predict the PISA 2018 reading comprehension achievement score of countries with different achievement levels, using 34 independent variables obtained from the student questionnaire given to the students who participated in PISA in 2018. For this purpose, 79 countries that participated PISA were ranked according to their success percentages then, these countries were sorted into lower, middle and upper group countries. A sample of lower, middle and upper group countries was formed then, three countries were selected from each of the lower group, middle group and upper group countries and a sample of lower, middle and upper group countries was formed. Data mining analyzes were carried out on the samples obtained by using the Classification and Regression Tree and Random Forest methods. It has been observed that the number of important variables that predict reading comprehension success can be reduced from 34 to three to eight. Like this; Data mining classification prediction models, which can predict the success level of PISA, were obtained by using a small number of variables. It has been determined that the models obtained have an acceptable level of predictive performance in predicting success in three categories (low, medium-high). The most important predictor variables obtained from the models are information and communication technologies resources, perception of reading difficulty, professional status expected from the student, perception of difficulty in the PISA test, reading pleasure, weekly test language learning time, disciplinary climate, socio-economic status index.
Anahtar Kelime: CRT ve RF Yöntemleri ile Farklı Başarı Düzeyine Sahip Ülkelerin PISA Okuduğunu Anlama Başarı Düzeylerini Etkileyen Değişkenlerin İncelenmesi
Öz: Bu araştırmanın amacı, 2018 yılında PISA’ya katılan öğrencilere sınavla birlikte verilen öğrenci anketinden elde edilen 34 bağımsız değişkeni kullanarak, farklı başarı düzeyine sahip ülkelerin PISA 2018 okuduğunu anlama başarı puanını yordayan önemli değişkenleri belirlemektir. Bu amaç için PISA’ya giren 79 ülke başarı yüzdeliklerine göre sıralanmış ve bu sıralamaya göre bu ülkeler alt, orta ve üst grup ülkeler olarak ayrılmıştır. Daha sonra alt grup, orta grup ve üst grup ülkelerin her birinden üçer ülke seçilerek alt, orta ve üst grup ülkeler örneklemi oluşturulmuştur. Elde edilen örneklemler üzerinde Sınıflama ve Regresyon Ağacı ve Rastgele Orman yöntemleri kullanılarak veri madenciliği analizleri gerçekleştirilmiştir. Yapılan uygulamalarda okuduğunu anlama başarısını yordayan önemli değişkenlerin sayısının 34’ten üç ile sekiz arasında bir sayıya indirgenebildiği görülmüştür. Böylece; az sayıda değişken kullanılarak PISA başarı düzeyini yordayabilen veri madenciliği sınıflama tahmin modelleri elde edilmiştir. Elde edilen modellerin başarıyı üç kategorili (düşük, orta yüksek) yordama da kabul edilebilir düzeyde tahmin performansına sahip oldukları saptanmıştır. Modellerden elde edilen tahmin edici değişkenlerden en önemli olanları bilgi iletişim teknolojileri kaynakları, okuma zorluk algısı, öğrenciden beklenen mesleki statü, PISA testinin zorluk algısı, okuma keyfi, haftalık test dili öğrenme süresi, disiplin iklimi, sosyo-ekonomik durum indeksi biçimindedir.
Anahtar Kelime: Belge Türü: Makale Makale Türü: Araştırma Makalesi Erişim Türü: Erişime Açık
- Abad, F. M., & Lopez, A. C. (2016). Data-mining techniques in detecting factors linked to academic achievement. An International Journal of Research, Policy and Practice, 28 (1), 39-55. https://doi.org /10.1080/09243453.2016.1235591
- Abdous, M., He, W., & Yen, C. J. (2012). Using data mining for predicting relationships between online question theme and final grade. Journal of Educational Technology ve Society, 15(3), 77-88.
- Akman, M. (2010). Veri madenciliğine genel bakış ve random forests yönteminin incelenmesi: Sağlık alanında bir uygulama [Yayınlanmamış yüksek lisans tezi]. Ankara Üniversitesi Sağlık Bilimleri Enstitüsü, Ankara.
- Aksu, G., & Güzeller, C. O. (2016). Classification of PISA 2012 mathematical literacy scores using decision-tree method. Journal of Education and Science, 41(185), 101-122. https://doi.org 10.15390/EB.2016.4766
- Altunkaynak, B. (2019). Veri madenciliği yöntemleri ve r uygulamaları. (2.Baskı). Seçkin yayıncılık.
- Arıcı, Ö., & Altıntaş, Ö. (2014). An investigation of the PISA 2009 reading literacy in terms of socio-economical backgrounds and receiving pre-school education. Journal of Faculty of Educational Sciences, 47(1), 423-448.
- Arıkan, S., Özer, F., Şeker, V., & Ertaş, G. (2020). Geniş ölçekli testlerde örneklem ağırlıklarının ve olası değerlerin önemi. Eğitimde ve Psikolojide Ölçme ve Değerlendirme Dergisi, 11(1), 43-60. https://doi.org 10.21031/epod.602765
- Atasever, Ü. H. (2011). Uydu görüntülerinin sınıflandırılmasında hızlandırma (boosting), destek vektör makineleri, rastgele orman (random forest) ve regresyon ağaçları yöntemlerinin kullanılması [Yayınlanmamış yüksek lisans tezi]. Erciyes Üniversitesi Fen Bilimleri Enstitüsü, Kayseri.
- Bezek Güre, Ö., Kayri, M., & Erdoğan, F. (2020). Analysis of factors effecting PISA 2015 mathematics literacy via educational data mining. Journal of Education and Science 45(202), 393-415. https://doi.org 10.15390/EB.2020.8477
- Bhalla, D. (2014). Random forest in R: Step by Step Tutorial. http//www.listendata.com/2014/11/random-forest-with-r.html sayfasından erişilmiştir.
- Breiman, L. (2001). Random forests. Machine Learning, 45(1), 5-32.
- Breiman, L. (2004). Consistency for a simple model of random forests. Technical Report 670, Statistics Department, University Of California At Berkeley.
- Büyüköztürk, Ş., Kılıç-Çakmak, E., Akgün, Ö.E., Karadeniz, Ş. ve Demirel, F. (2018). Bilimsel Araştırma Yöntemleri. (25.Baskı). Pegem Yayıncılık.
- Coulombe S., Tremblay, J. F., & Marchand, S. (2004). Literacy scores, human capital and growth across fourteen OECD countries, Statistics Canada, Ottawa.
- Field, A. (2009). Discovering Statistics using SPSS. London: Sage.
- Gamazo, A., & Abad, F. M. (2020). An exploration of factors linked to academic performance in PISA 2018 through data mining techniques. Journal Frontiers in Psycholog, 11, 575167. https://doi.org doi: 10.3389/fpsyg.2020.575167
- Güner, Z. B. (2014). Veri madenciliğinde cart ve lojistik regresyon analizinin yeri: ilaç provizyon sistemi verileri üzerinde örnek bir uygulama. Sosyal Güvence Dergisi, 6, 53-99.
- Güzle Kayır, Ç. (2012). PISA 2009 Türkiye verilerine dayanarak okuma becerileri alanında başarılı okullar ile başarısız okulları ayırt eden okul içi etmenler ve sosyo-ekonomik faktörler [Yayınlanmamış yüksek lisans tezi]. Akdeniz Üniversitesi Sosyal Bilimler Enstitüsü, Antalya.
- He, Y. (2006). Missing data imputation for tree-based models. [Yayınlanmamış Doktora Tezi]. California Üniversity.
- Kayri, M. (2008). Elektronik portfolyo değerlendirmeleri için veri madenciliği yaklaşımı. Yüzüncü Yıl Üniversitesi Eğitim Fakültesi Dergisi, 5(1), 98-110.
- Kiray, S.A., Gok, B., & Bozkir, A.S. (2015). Identifying the factors affecting science and mathematics achievement using data mining methods. Journal of Education in Science, 1(1), 28-48. https://doi.org/10.21891/jeseh.41216
- Köse, İ. (2018). Veri madenciliği teori uygulama ve felsefesi, (1.Baskı), İstanbul: Papatya yayıncılık, 48-50.
- Larose, D. T. (2005). Discovering knowledge in data: An introduction to data mining. John Wiley ve Sons, New Jersey.
- Liao, Z., Ju, Y., & Zou, Q. (2016). Prediction of G Protein-Coupled Receptors with SVM-Prot Features and Random Forest. Journal of Scientifica, 8309253. https://doi.org/ 10.1155/2016/8309253.
- Liaw, A., & Wiener, M. (2002). Classification and regression by random forest. R news, 2(3), 18-22.
- Loh, W.Y. (2011). Classification and regression trees. Wires Data Mining and Knowledge Discovery, 1, 14-23. https://doi.org/10.1002/widm.8
- MEB (2010). PISA 2009 ulusal ön raporu. Ankara: http://pisa.meb.gov.tr/wp-content/ uploads/2013/07/PISA-2009-Ulusal-On-Rapor.pdf.
- MEB (2019). PISA 2018 Ulusal Ön Raporu. Ankara: T.C. Milli Eğitim Bakanlığı
- OECD (2019). PISA 2018 Technical Report, OECD Publishing, Paris.
- Özarslan, S. (2014). Öğrenci performansının veri madenciliği yöntemleri ile belirlenmesi [Yüksek Lisans Tezi]. Kırıkkale Üniversitesi Fen Bilimleri Enstitüsü, Kırıkkale.
- Özkan, Y. (2008). Veri madenciliği yöntemleri. İstanbul: Papatya yayıncılık.
- Polikar, R. (2006). Ensemble based systems in decision making. IEEE Circuits and Systems Magazine, 6(3),21–44.
- Savaş, S., Topaloğlu, N., & Yılmaz, M. (2012). Veri madenciliği ve Türkiye’deki uygulama örnekleri. İstanbul Ticaret Üniversitesi Fen Bilimleri Dergisi, 11(21), 1-23.
- Sieber, J. E. (2008). Knowledge discovery for human research ethics. J Empir Res Hum Res Ethics, 3 (3), 1-2. https://doi.org/10.1525/jer.2008.3.1.1 Silahtaroğlu, G. (2016). Veri madenciliği kavram ve algoritmaları. (3.Baskı). İstanbul: Papatya yayıncılık. 12.
- Tabachnick, B. G., & Fidell, L. S. (2007). Using multivariate statistics. Boston: Pearson/Allyn and Bacon.
- Tavşancıl, E., Yıldırım, Ö., & Bilican Demir, S. (2019). Direct and indirect effects of learning strategies and reading enjoyment on PISA 2009 reading performance. Eurasian Journal of Educational Research, 82, 169-190.
- Urfalı Dadandı, P., Dadandı, İ., & Koca, F. (2018). PISA 2015 türkiye sonuçlarına göre sosyoekonomik faktörler ile okuma becerileri arasındaki ilişkiler. Uluslararası Türkçe Edebiyat Kültür Eğitim Dergisi, 7(2), 1239-1252.
- Wu, M. L., & Adams, R. J., (2002). Plausible Values: Why they are important. Paper presented at the International Objective Measurement workshop, New Orleans, LA.
- Yu, C. H., Kaprolet C., Pennel, A. J., & Digangi, S. (2012). A data mining approach to comparing american and canadian grade 10 students’ pisa science test performance. Journal of Data Science 10, 441-464. https://doi.org/10.6339/JDS.201207_10(3).0006
- Yue, Y. (2021). The prediction model of college english performance based on data mining under the concept of obe. Engineering Intelligent Systems, 2, 89–96.
- Yung, J. L., Hsu, Y. C., & Rice, K. (2012). Integrating data mining in program evaluation of k-12 online education. Journal of Educational Technology ve Society, 15(3), 27-41.
APA | Kasap Y, DOĞAN N, Kocak C (2023). Investigation of Variables Affecting PISA Reading Comprehension Achievement Levels of Countries with Different Levels of Achievement with CRT and RF Methods. , 459 - 483. 10.33711/yyuefd.1221541 |
Chicago | Kasap Yusuf,DOĞAN NURİ,Kocak Cem Investigation of Variables Affecting PISA Reading Comprehension Achievement Levels of Countries with Different Levels of Achievement with CRT and RF Methods. (2023): 459 - 483. 10.33711/yyuefd.1221541 |
MLA | Kasap Yusuf,DOĞAN NURİ,Kocak Cem Investigation of Variables Affecting PISA Reading Comprehension Achievement Levels of Countries with Different Levels of Achievement with CRT and RF Methods. , 2023, ss.459 - 483. 10.33711/yyuefd.1221541 |
AMA | Kasap Y,DOĞAN N,Kocak C Investigation of Variables Affecting PISA Reading Comprehension Achievement Levels of Countries with Different Levels of Achievement with CRT and RF Methods. . 2023; 459 - 483. 10.33711/yyuefd.1221541 |
Vancouver | Kasap Y,DOĞAN N,Kocak C Investigation of Variables Affecting PISA Reading Comprehension Achievement Levels of Countries with Different Levels of Achievement with CRT and RF Methods. . 2023; 459 - 483. 10.33711/yyuefd.1221541 |
IEEE | Kasap Y,DOĞAN N,Kocak C "Investigation of Variables Affecting PISA Reading Comprehension Achievement Levels of Countries with Different Levels of Achievement with CRT and RF Methods." , ss.459 - 483, 2023. 10.33711/yyuefd.1221541 |
ISNAD | Kasap, Yusuf vd. "Investigation of Variables Affecting PISA Reading Comprehension Achievement Levels of Countries with Different Levels of Achievement with CRT and RF Methods". (2023), 459-483. https://doi.org/10.33711/yyuefd.1221541 |
APA | Kasap Y, DOĞAN N, Kocak C (2023). Investigation of Variables Affecting PISA Reading Comprehension Achievement Levels of Countries with Different Levels of Achievement with CRT and RF Methods. Van Yüzüncü Yıl Üniversitesi Eğitim Fakültesi Dergisi, 20(2), 459 - 483. 10.33711/yyuefd.1221541 |
Chicago | Kasap Yusuf,DOĞAN NURİ,Kocak Cem Investigation of Variables Affecting PISA Reading Comprehension Achievement Levels of Countries with Different Levels of Achievement with CRT and RF Methods. Van Yüzüncü Yıl Üniversitesi Eğitim Fakültesi Dergisi 20, no.2 (2023): 459 - 483. 10.33711/yyuefd.1221541 |
MLA | Kasap Yusuf,DOĞAN NURİ,Kocak Cem Investigation of Variables Affecting PISA Reading Comprehension Achievement Levels of Countries with Different Levels of Achievement with CRT and RF Methods. Van Yüzüncü Yıl Üniversitesi Eğitim Fakültesi Dergisi, vol.20, no.2, 2023, ss.459 - 483. 10.33711/yyuefd.1221541 |
AMA | Kasap Y,DOĞAN N,Kocak C Investigation of Variables Affecting PISA Reading Comprehension Achievement Levels of Countries with Different Levels of Achievement with CRT and RF Methods. Van Yüzüncü Yıl Üniversitesi Eğitim Fakültesi Dergisi. 2023; 20(2): 459 - 483. 10.33711/yyuefd.1221541 |
Vancouver | Kasap Y,DOĞAN N,Kocak C Investigation of Variables Affecting PISA Reading Comprehension Achievement Levels of Countries with Different Levels of Achievement with CRT and RF Methods. Van Yüzüncü Yıl Üniversitesi Eğitim Fakültesi Dergisi. 2023; 20(2): 459 - 483. 10.33711/yyuefd.1221541 |
IEEE | Kasap Y,DOĞAN N,Kocak C "Investigation of Variables Affecting PISA Reading Comprehension Achievement Levels of Countries with Different Levels of Achievement with CRT and RF Methods." Van Yüzüncü Yıl Üniversitesi Eğitim Fakültesi Dergisi, 20, ss.459 - 483, 2023. 10.33711/yyuefd.1221541 |
ISNAD | Kasap, Yusuf vd. "Investigation of Variables Affecting PISA Reading Comprehension Achievement Levels of Countries with Different Levels of Achievement with CRT and RF Methods". Van Yüzüncü Yıl Üniversitesi Eğitim Fakültesi Dergisi 20/2 (2023), 459-483. https://doi.org/10.33711/yyuefd.1221541 |