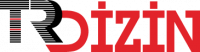
A new bearing fault diagnosis approach based on common spatial pattern features
Yıl: 2023 Cilt: 12 Sayı: 4 Sayfa Aralığı: 1545 - 1557 Metin Dili: İngilizce DOI: 10.28948/ngumuh.1330864 İndeks Tarihi: 23-10-2023
A new bearing fault diagnosis approach based on common spatial pattern features
Öz: Condition monitoring in machines holds significant importance for early fault detection, optimizing maintenance processes, and ensuring operational continuity. In this study, a novel intelligent detection approach for rolling bearings is introduced, utilizing the Common Spatial Pattern (CSP) method to extract distinctive features related to bearing faults. By maximizing the variance ratio of signal matrices from distinct sources, CSP sets itself apart from conventional frequency-based features. This technique captures characteristic vibration patterns unique to each measurement, enabling differentiation between faulty and healthy bearings. The effectiveness of the proposed method was assessed using Artificial Neural Network (ANN), Support Vector Machine (SVM), and K-Nearest Neighbour (k-NN) algorithms across two diverse datasets. The results indicated an 88.5% accuracy in two-class fault detection and 93.5% in fault classification when employing ANN. Comparison with traditional time domain feature sets highlighted the superior performance of CSP features, exhibiting elevated accuracy rates in both two-class and multiclass scenarios. Thus, CSP features emerge as a promising avenue for effectively monitoring bearing conditions through vibration data.
Anahtar Kelime: Ortak uzamsal örüntü özniteliklerine dayalı yeni bir rulman arızası teşhisi yaklaşımı
Öz: Makinelerde durum izleme, erken arıza tespiti, bakım süreçlerinin optimize edilmesi ve iş sürekliliğinin sağlanması açısından büyük öneme sahiptir. Bu çalışmada, rulmanlar için yeni bir akıllı tespit yaklaşımı sunulmuş, rulman arızalarıyla ilgili ayırt edici öznitelikleri çıkarmak için Ortak Uzamsal Örüntü (OUÖ) yöntemi kullanılmıştır. Farklı kaynaklardan gelen sinyal matrislerinin varyans oranını maksimize eden OUÖ, geleneksel frekans temelli özniteliklerden ayrılır. Bu teknik, her ölçümde benzersiz titreşim desenlerini yakalayarak arızalı ve sağlam rulmanlar arasındaki farkı belirlemeyi sağlar. Önerilen yöntemin etkinliği Yapay Sinir Ağı (YSA), Destek Vektör Makinesi (DVM) ve K-En Yakın Komşu (k-YK) algoritmaları kullanılarak iki farklı veri kümesinde değerlendirildi. Sonuçlar, YSA kullanıldığında iki sınıflı arıza tespitinde %88.5 doğruluk ve arıza sınıflandırmasında %93.5 doğruluk elde edilebileceğini gösterdi. Geleneksel zaman alanı öznitelikleri ile yapılan karşılaştırma, OUÖ özniteliklerinin üstün performansını ortaya koydu. OUÖ iki sınıflı ve çoklu sınıflı senaryolarda yüksek doğruluk oranları sergiledi. Böylece, OUÖ öznitelikleri titreşim verileri aracılığıyla rulman arızalarının etkili bir şekilde tespit edilmesi için umut verici bir yol olarak ortaya çıkmaktadır.
Anahtar Kelime: Belge Türü: Makale Makale Türü: Araştırma Makalesi Erişim Türü: Erişime Açık
- J. Chen, C. Lin, D. Peng and H. Ge, Fault diagnosis of rotating machinery: A review and bibliometric analysis. Ieee Access, 8, 224985-225003, 2020. https://doi.org/10.1109/ACCESS.2020.3043743
- O. AlShorman, M. Irfan, N. Saad, D. Zhen, N. Haider, A. Glowacz and A. AlShorman, A review of artificial intelligence methods for condition monitoring and fault diagnosis of rolling element bearings for induction motor. Shock and vibration, ID 8843759, 2020. https://doi.org/10.1155/2020/8843759
- P. Bangalore and L.B. Tjernberg, An artificial neural network approach for early fault detection of gearbox bearings. IEEE Transactions on Smart Grid, 6(2), 980-987, 2015. https://doi.org/10.1109/TSG.2014.2386305
- P. Gupta and M.K. Pradhan, Fault detection analysis in rolling element bearing: A review. Materials Today: Proceedings, 4(2), 2085-2094, 2017. https://doi.org/10.1016/j.matpr.2017.02.054
- R. Yao, H. Jiang, Z. Wu and K. Wang, Periodicity-enhanced sparse representation for rolling bearing incipient fault detection. ISA transactions, 118, 219-237, 2021. https://doi.org/10.1016/j.isatra.2021.02.023
- S. Lu, P. Zhou, X. Wang, Y. Liu, F. Liu and J. Zhao, Condition monitoring and fault diagnosis of motor bearings using undersampled vibration signals from a wireless sensor network. Journal of Sound and Vibration, 414, 81-96, 2018. https://doi.org/10.1016/j.jsv.2017.11.007
- J. Wang, M. Xu, C. Zhang, B. Huang and F. Gu, Online bearing clearance monitoring based on an accurate vibration analysis. Energies, 13(2), 389, 2020. https://doi.org/10.3390/en13020389
- H.O. Omoregbee and P.S. Heyns, Fault classification of low-speed bearings based on support vector machine for regression and genetic algorithms using acoustic emission. Journal of Vibration Engineering & Technologies, 7, 455-464, 2019. https://doi.org/10.1007/s42417-019-00143-y
- B.R.F. Rende, A.A. Cavalini Jr and I.F. Santos, Fault detection using vibration data-driven models—a simple and well-controlled experimental example. Journal of the Brazilian Society of Mechanical Sciences and Engineering, 44(6), 229, 2022. https://doi.org/10.1007/s40430-022-03462-6
- H. Wang and P. Chen, A feature extraction method based on information theory for fault diagnosis of reciprocating machinery. Sensors, 9(4), 2415-2436, 2009. https://doi.org/10.3390/s90402415
- C. Malla and I. Panigrahi, Review of condition monitoring of rolling element bearing using vibration analysis and other techniques. Journal of Vibration Engineering & Technologies, 7, 407-414, 2019. https://doi.org/10.1007/s42417-019-00119-y
- S.K. Gundewar and P.V. Kane, Condition monitoring and fault diagnosis of induction motor. Journal of Vibration Engineering & Technologies, 9, 643-674, 2021. https://doi.org/10.1007/s42417-020-00253-y
- Q. He, Y. Liu, Q. Long and J. Wang, Time-frequency manifold as a signature for machine health diagnosis. IEEE Transactions on Instrumentation and Measurement, 61(5), 1218-1230, 2012. https://doi.org/10.1109/TIM.2012.2183402
- H. Cao, F. Fan, K. Zhou and Z. He, Wheel-bearing fault diagnosis of trains using empirical wavelet transform. Measurement, 82, 439-449, 2016. https://doi.org/10.1016/j.measurement.2016.01.023
- Y. Gao, D. Yu and H. Wang, Fault diagnosis of rolling bearings using weighted horizontal visibility graph and graph Fourier transform. Measurement, 149, 107036, 2020. https://doi.org/10.1016/j.measurement.2019.107036
- D. Zhao, W. Cheng, R.X. Gao, R. Yan and P. Wang, Generalized Vold–Kalman filtering for nonstationary compound faults feature extraction of bearing and gear. IEEE Transactions on Instrumentation and Measurement, 69(2), 401-410, 2019. https://doi.org/10.1109/TIM.2019.2903700
- B. Muruganatham, M.A. Sanjith, B. Krishnakumar and S.S. Murty, Roller element bearing fault diagnosis using singular spectrum analysis. Mechanical systems and signal processing, 35(1-2), 150-166, 2013. https://doi.org/10.1016/j.ymssp.2012.08.019
- J.B. Ali, N. Fnaiech, L. Saidi, B. Chebel-Morello and F. Fnaiech, Application of empirical mode decomposition and artificial neural network for automatic bearing fault diagnosis based on vibration signals. Applied Acoustics, 89, 16-27, 2015. https://doi.org/10.1016/j.apacoust.2014.08.016
- Y. Li, Q. Gao, B. Miao, W. Zhang, J. Liu and Y. Zhu, Application of the refined multiscale permutation entropy method to fault detection of rolling bearing. Journal of the Brazilian Society of Mechanical Sciences and Engineering, 43(5), 280, 2021. https://doi.org/10.1007/s40430-021-02986-7
- Y. Cheng, S. Wang, B. Chen, G. Mei, W. Zhang, H. Peng and G. Tian, An improved envelope spectrum via candidate fault frequency optimization-gram for bearing fault diagnosis. Journal of Sound and Vibration, 523, 116746, 2022. https://doi.org/10.1016/j.jsv.2022.116746
- Y. Wang and M. Liang, Identification of multiple transient faults based on the adaptive spectral kurtosis method. Journal of Sound and Vibration, 331(2), 470-486, 2012. https://doi.org/10.1016/j.jsv.2011.08.029
- J.K. Alsalaet, Fast averaged cyclic periodogram method to compute spectral correlation and coherence. ISA transactions, 129, 609-630, 2022. https://doi.org/10.1016/j.isatra.2022.01.029
- Z. Feng, H. Ma and M.J. Zuo, Spectral negentropy based sidebands and demodulation analysis for planet bearing fault diagnosis. Journal of Sound and Vibration, 410, 124-150, 2017. https://doi.org/10.1016/j.jsv.2017.08.024
- D. Wang, X. Zhao, L.L. Kou, Y. Qin, Y. Zhao and K.L. Tsui, A simple and fast guideline for generating enhanced/squared envelope spectra from spectral coherence for bearing fault diagnosis. Mechanical Systems and Signal Processing, 122, 754-768, 2019. https://doi.org/10.1016/j.ymssp.2018.12.055
- B. Tang, W. Liu and T. Song, Wind turbine fault diagnosis based on Morlet wavelet transformation and Wigner-Ville distribution. Renewable Energy, 35(12), 2862-2866, 2010. https://doi.org/10.1016/j.renene.2010.05.012
- W. Sun, G. An Yang, Q. Chen, A. Palazoglu and K. Feng, Fault diagnosis of rolling bearing based on wavelet transform and envelope spectrum correlation. Journal of Vibration and Control, 19(6), 924-941, 2013. https://doi.org/10.1177/1077546311435348
- F. Zou, H. Zhang, S. Sang, X. Li, W. He and X. Liu, Bearing fault diagnosis based on combined multi-scale weighted entropy morphological filtering and bi-LSTM. Applied Intelligence, 1-18, 2021. https://doi.org/10.1007/s10489-021-02229-1
- Z. Feng, D. Zhang and M. J. Zuo, Adaptive mode decomposition methods and their applications in signal analysis for machinery fault diagnosis: a review with examples. IEEE access, 5, 24301-24331, 2017. https://doi.org/10.1109/ACCESS.2017.2766232
- W. Ying, J. Tong, Z. Dong, H. Pan, Q. Liu and J. Zheng, Composite multivariate multi-Scale permutation entropy and laplacian score based fault diagnosis of rolling bearing. Entropy, 24(2), 160, 2022. https://doi.org/10.3390/e24020160
- J. Altmann and J. Mathew, Multiple band-pass autoregressive demodulation for rolling-element bearing fault diagnosis. Mechanical systems and signal processing, 15(5), 963-977, 2001. https://doi.org/10.1006/mssp.2001.1410
- C. Xu, C. Wang and Liu, Nonstationary vibration signal analysis using Wavelet-based time–frequency filter and wigner–ville distribution. Journal of Vibration and Acoustics, 138(5), 051009, 2016. https://doi.org/10.1115/1.4033641
- H. Ramoser, J. Muller-Gerking, and G. Pfurtscheller, Optimal spatial filtering of single trial EEG during imagined hand movement. IEEE transactions on rehabilitation engineering, 8(4), 441-446, 2000. https://doi.org/%2010.1109/86.895946
- I. Xygonakis, A. Athanasiou, N. Pandria, D. Kugiumtzis and P.D. Bamidis, Decoding motor imagery through common spatial pattern filters at the EEG source space. Computational intelligence and neuroscience, ID 7957408, 2018. https://doi.org/10.1155/2018/7957408
- Y.E. Karabacak and N.G. Özmen, Common spatial pattern-based feature extraction and worm gear fault detection through vibration and acoustic measurements. Measurement, 187, 110366, 2022. https://doi.org/10.1016/j.measurement.2021.110366
- Z. Li, Y. Lv, R. Yuan and Q. Zhang, An intelligent fault diagnosis method of rolling bearings via variational mode decomposition and common spatial pattern-based feature extraction. IEEE Sensors Journal, 22(15), 15169-15177, 2022. https://ieeexplore.ieee.org/document/9807645
- Case Western Reserve University Bearing Data Center Website. http://csegroups.case.edu/bearingdatacenter, Accessed 14.7.2023
- R.B. Randall, Vibration-based condition monitoring: industrial, automotive and aerospace applications. John Wiley & Sons, NJ, 2021.
- A. Gabrielli, M. Battarra, E. Mucchi and G. Dalpiaz, Acceleration signals of rolling element bearings with artificial defects. Mendeley Data, 2022. https://doi.org/10.17632/8wdzm5gwng.1
- P. Kumar and A.S. Hati, Review on machine learning algorithm based fault detection in induction motors. Archives of Computational Methods in Engineering, 28, 1929-1940, 2021. https://doi.org/10.1007/s11831-020-09446-w
- T. Han, D. Jiang, Q. Zhao, L. Wang and K. Yin, Comparison of random forest, artificial neural networks and support vector machine for intelligent diagnosis of rotating machinery. Transactions of the Institute of Measurement and Control, 40(8), 2681-2693, 2018. https://doi.org/10.1177/0142331217708242
- L.B. Jack and A.K. Nandi, Fault detection using support vector machines and artificial neural networks, augmented by genetic algorithms. Mechanical systems and signal processing, 16(2-3), 373-390, 2002. https://doi.org/10.1006/mssp.2001.1454
- S. Liu, J. Xie, C. Shen, X. Shang, D. Wang and Zhu, Z, Bearing fault diagnosis based on improved convolutional deep belief network. Applied Sciences, 10(18), 6359, 2020. https://doi.org/10.3390/app10186359
- R. Liu, B. Yang, E. Zio and X. Chen, Artificial intelligence for fault diagnosis of rotating machinery: A review. Mechanical Systems and Signal Processing, 108, 33-47, 2018. https://doi.org/10.1016/j.ymssp.2018.02.016
- H. Zhao, X. Yang, B. Chen, H. Chen and W. Deng, Bearing fault diagnosis using transfer learning and optimized deep belief network. Measurement Science and Technology, 33(6), 065009, 2022. https://doi.org/10.1088/1361-6501/ac543a
- Y. Kaya, M. Kuncan, K. Kaplan, M.R. Minaz and H.M. Ertunç, A new feature extraction approach based on one dimensional gray level co-occurrence matrices for bearing fault classification. Journal of Experimental & Theoretical Artificial Intelligence, 33(1), 161-178, 2021. https://doi.org/10.1080/ 0952813X.2020.1735530
- S. Bayram, K. Kaplan, M. Kuncan and H. M. Ertunç, The effect of bearings faults to coefficients obtaned by using wavelet transform. 22nd Signal Processing and Communications Applications Conference (SIU), pp. 991-994, Trabzon, Turkey, 23-25 April 2014.
- Y. Kaya, F. Kuncan and H.M. Ertunç, A new automatic bearing fault size diagnosis using time-frequency images of CWT and deep transfer learning methods. Turkish Journal of Electrical Engineering and Computer Sciences, 30(5), 1851-1867, (2022). https://doi.org/10.55730/1300-0632.3909
- M. Kuncan, An Intelligent Approach for Bearing Fault Diagnosis: Combination of 1D-LBP and GRA. IEEE Access, 8, 137517-137529, 2020. https://doi.org/ 10.1109/ ACCESS.2020.3011980.
- H. Yang, X. Li and W. Zhang, Interpretability of deep convolutional neural networks on rolling bearing fault diagnosis. Measurement Science and Technology, 33(5), 055005, 2022. https://doi.org/10.1088/1361-6501/ac41a5
- N.M. Thoppil, V. Vasu and C.S.P. Rao, Deep learning algorithms for machinery health prognostics using time-series data: A review. Journal of Vibration Engineering & Technologies, 1-23, 2021. https://doi.org/10.1007/s42417-021-00286-x
- W.A. Smith and R.B. Randall, Rolling element bearing diagnostics using the Case Western Reserve University data: A benchmark study. Mechanical systems and signal processing, 64, 100-131, 2015. https://doi.org/10.1016/j.ymssp.2015.04.021
- H. Nguyen-Schäfer, Computational design of rolling bearings. Springer International Publishing, Cham, 2016.
- T. Nguyen, I. Hettiarachchi, A. Khatami, L. Gordon-Brown, C.P. Lim and S. Nahavandi, Classification of multi-class BCI data by common spatial pattern and fuzzy system. IEEE access, 6, 27873-27884, 2018. https://doi.org/10.1109/ACCESS.2018.2841051
- V. Martínez-Cagigal, Common Spatial Patterns (CSP), MATLAB Central File Exchange. https://www.mathworks.com/matlabcentral/fileexchange/72204-common-spatial-patterns-csp, Accessed 14.07.2023.
- Y.E. Karabacak, N.G. Özmen and L. Gümüşel, Intelligent worm gearbox fault diagnosis under various working conditions using vibration, sound and thermal features. Applied Acoustics, 186, 108463, 2022. https://doi.org/10.1016/j.apacoust.2021.108463
- D.J. Bordoloi and R. Tiwari, Optimum multi-fault classification of gears with integration of evolutionary and SVM algorithms. Mechanism and Machine Theory, 73, 49-60, 2014. https://doi.org/10.1016/j.mechmachtheory.2013.10.006
- Q. Wang, S. Wang, B. Wei, W. Chen and Y. Zhang, Weighted K-NN classification method of bearings fault diagnosis with multi-dimensional sensitive features. IEEE Access, 9, 45428-45440, 2021. https://doi.org/10.1109/ACCESS.2021.3066489
- A.A.A. Mohd Amiruddin, H. Zabiri, S.A.A. Taqvi and L.D. Tufa, Neural network applications in fault diagnosis and detection: an overview of implementations in engineering-related systems. Neural Computing and Applications, 32, 447-472, 2020. https://doi.org/10.1007/s00521-018-3911-5
- Y. Bengio and Y. LeCun, Scaling Learning Algorithms towards AI. in: L. Bottou, O. Chapelle, D. DeCoste, J. Weston (Eds.), Large-Scale Kernel Machines, MIT Press, NY, 2007.
- Z. Xia, S. Xia, L. Wan and S. Cai, Spectral regression based fault feature extraction for bearing accelerometer sensor signals. Sensors, 12(10), 13694-13719, 2012. https://doi.org/10.3390/s121013694
- J. Sun, C. Yan and J. Wen, Intelligent bearing fault diagnosis method combining compressed data acquisition and deep learning. IEEE Transactions on Instrumentation and Measurement, 67(1), 185-195 2017. https://doi.org/10.1109/TIM.2017.2759418
- M. Sun, Z. Song, X. Jiang, J. Pan and Y. Pang, Learning pooling for convolutional neural network. Neurocomputing, 224, 96-104, 2017. https://doi.org/10.1016/j.neucom.2016.10.049
- Y.E. Karabacak and N. Gürsel Özmen, Application of Deep Learning Method for Condition Monitoring and Fault Diagnosis from Vibration Data in Bearings. Konya Journal of Engineering Sciences, 10(2), 346-365, 2022. https://doi.org/10.36306/konjes.1049489
APA | Gursel Ozmen N, karabacak y (2023). A new bearing fault diagnosis approach based on common spatial pattern features. , 1545 - 1557. 10.28948/ngumuh.1330864 |
Chicago | Gursel Ozmen Nurhan,karabacak yunus emre A new bearing fault diagnosis approach based on common spatial pattern features. (2023): 1545 - 1557. 10.28948/ngumuh.1330864 |
MLA | Gursel Ozmen Nurhan,karabacak yunus emre A new bearing fault diagnosis approach based on common spatial pattern features. , 2023, ss.1545 - 1557. 10.28948/ngumuh.1330864 |
AMA | Gursel Ozmen N,karabacak y A new bearing fault diagnosis approach based on common spatial pattern features. . 2023; 1545 - 1557. 10.28948/ngumuh.1330864 |
Vancouver | Gursel Ozmen N,karabacak y A new bearing fault diagnosis approach based on common spatial pattern features. . 2023; 1545 - 1557. 10.28948/ngumuh.1330864 |
IEEE | Gursel Ozmen N,karabacak y "A new bearing fault diagnosis approach based on common spatial pattern features." , ss.1545 - 1557, 2023. 10.28948/ngumuh.1330864 |
ISNAD | Gursel Ozmen, Nurhan - karabacak, yunus emre. "A new bearing fault diagnosis approach based on common spatial pattern features". (2023), 1545-1557. https://doi.org/10.28948/ngumuh.1330864 |
APA | Gursel Ozmen N, karabacak y (2023). A new bearing fault diagnosis approach based on common spatial pattern features. Niğde Ömer Halisdemir Üniversitesi Mühendislik Bilimleri Dergisi, 12(4), 1545 - 1557. 10.28948/ngumuh.1330864 |
Chicago | Gursel Ozmen Nurhan,karabacak yunus emre A new bearing fault diagnosis approach based on common spatial pattern features. Niğde Ömer Halisdemir Üniversitesi Mühendislik Bilimleri Dergisi 12, no.4 (2023): 1545 - 1557. 10.28948/ngumuh.1330864 |
MLA | Gursel Ozmen Nurhan,karabacak yunus emre A new bearing fault diagnosis approach based on common spatial pattern features. Niğde Ömer Halisdemir Üniversitesi Mühendislik Bilimleri Dergisi, vol.12, no.4, 2023, ss.1545 - 1557. 10.28948/ngumuh.1330864 |
AMA | Gursel Ozmen N,karabacak y A new bearing fault diagnosis approach based on common spatial pattern features. Niğde Ömer Halisdemir Üniversitesi Mühendislik Bilimleri Dergisi. 2023; 12(4): 1545 - 1557. 10.28948/ngumuh.1330864 |
Vancouver | Gursel Ozmen N,karabacak y A new bearing fault diagnosis approach based on common spatial pattern features. Niğde Ömer Halisdemir Üniversitesi Mühendislik Bilimleri Dergisi. 2023; 12(4): 1545 - 1557. 10.28948/ngumuh.1330864 |
IEEE | Gursel Ozmen N,karabacak y "A new bearing fault diagnosis approach based on common spatial pattern features." Niğde Ömer Halisdemir Üniversitesi Mühendislik Bilimleri Dergisi, 12, ss.1545 - 1557, 2023. 10.28948/ngumuh.1330864 |
ISNAD | Gursel Ozmen, Nurhan - karabacak, yunus emre. "A new bearing fault diagnosis approach based on common spatial pattern features". Niğde Ömer Halisdemir Üniversitesi Mühendislik Bilimleri Dergisi 12/4 (2023), 1545-1557. https://doi.org/10.28948/ngumuh.1330864 |