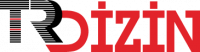
Deep Learning Based Classification for Hoverflies (Diptera: Syrphidae)
Yıl: 2023 Cilt: 25 Sayı: 3 Sayfa Aralığı: 529 - 544 Metin Dili: İngilizce DOI: 10.51963/jers.v25i3.2445 İndeks Tarihi: 24-11-2023
Deep Learning Based Classification for Hoverflies (Diptera: Syrphidae)
Öz: Syrphidae is essential in pollinating many flowering plants and cereals and is a family with high species diversity in the order Diptera. These family species are also used in biodiversity and conservation studies. This study proposes an image-based CNN model for easy, fast, and accurate identification of Syrphidae species. Seven hundred twenty-seven hoverfly images were used to train and test the developed deep-learning model. Four hundred seventy-nine of these images were allocated to the training set and two hundred forty-eight to the test dataset. There are a total of 15 species in the dataset. With the CNN-based deep learning model developed in this study, accuracy 0.96, precision 0.97, recall 0.96, and f-measure 0.96 values were obtained for the dataset. The experimental results showed that the proposed CNN-based deep learning model had a high success rate in distinguishing the Syrphidae species.
Anahtar Kelime: Belge Türü: Makale Makale Türü: Araştırma Makalesi Erişim Türü: Erişime Açık
- Al-Saqer, S. M., & Hassan G. M. (2011). Artificial neural networks based red palm weevil (Rynchophorus ferrugineous Olivier) recognition system. American Journal of Agricultural and Biological Sciences, 6, 356-364. doi: https://doi.org/10.3844/ajabssp.2011.356.364.
- Arbuckle, T., Schröder, S., Steinhage, V., & Wittmann, D. (2001). Biodiversity informatics in action: identication and monitoring of bee species using ABIS. 15th International Conference on Environmental Informatics, Zurich, October 10-12.
- Beccaloni, G. W., & Gaston, K. J. (1995). Predicting the species richness of Neotropical forest butterflies: Ithominae (Lepidoptera: Nymphalidae) as indicators. Biological Conservation, 71, 77–86. doi: https://doi.org/10.1016/0006-3207(94)00023-J.
- Dennis, R. L. H., Dapporto, L., Shreeve, T. G., John, E., Coutsis, J. G., Kudrna, O., Saarinen, K., & Ryrholm, N. (2008). Butterflies of European islands: The implications of the geography and ecology of rarity and endemicity for conservation. Journal of Insect Conservation, 12, 205–236. doi: https://doi.org/10.1007/s10841-008-9148-3.
- Dietrich, C. H., Emigh, T. H., & Deitz, L. L. (1991). Morphometric discrimination among females of sibling species of Aconophorini (Homoptera: Membracidae). Systematic Entomology, 16(3), 311-318. doi: https://doi.org/ 10.1111/J.1365-3113.1991.Tb00691.X.
- Dietrich, C. H. & Pooley, C. D. (1994). Automated identification of leafhoppers (Homoptera: Cicadellidae: Draeculacephala Ball). Annals of the Entomological Society of America, 87(4), 412-423. doi: https://doi.org/10.1093/Aesa/87.4.412.
- Do, M. J., Harp, K., & Norris, A. (1999). Test of a pattern recognition system for identification of spiders. Bulletin of Entomological Research, 89(3), 217-224. doi: https://doi.org/10.1017/S0007485399000334.
- Dover, J., Sparks, T., Clarke, S., Gobbett, K., & Glossop, S. (2000). Linear features and butterflies: the importance of green lanes. Agriculture, Ecosystems & Environment, 80, 227–242. doi: https://doi.org/10.1016/S0167-8809(00)00149-3.
- Faria, F. A., Perreb, P., Zucchic, R. A., Jorged, L. R., Lewinsohnd, T. M., Rochaa, A., & Torresa, R. da S. (2014). Automatic identification of fruit flies (Diptera: Tephritidae). Journal of Visual Communication and Image Representation, 25(7), 1516-1527. doi: https://doi.org/10.1016/j.jvcir.2014.06.014.
- Fedor, P., Vaňhara, J., Havel, J., Malenovský, I., & Spellerberg, I. (2009). Artificial intelligence in pest insect monitoring. Systematic Entomology, 34, 398–400. doi: https://doi.org/10.1111/j.1365-3113.2008.00461.x.
- Francoy, T. M., Wittmann, D., Drauschke, M., Müller, S., Steinhage, V., Bezerra-Laure, M. A. F., Jong, D., & Gonçalves, L. S. (2008).Identifiation of Africanized honey bees through wing morphometrics: two fast and efficient procedures. Apidologie, 39(5), 488-494. doi: https://doi.org/10.1051/apido:2008028.
- Gaston, K. J. & May, R. M. (1992). Taxonomy of taxonomists. Nature, 356, 281–281. doi: https://doi.org/10.1038/356281a0.
- Gaston, K. J. & Mound, L. A. (1993). Taxonomy, hypothesis testing and the biodiversity crisis. Proceedings of the Royal Society of London Series B, 251, 139–142.
- Gaston, K. J. & O’Neill, M. A. (2004). Automated species identication: why not?. Philosophical Transactions of the Royal Society B: Biological Sciences, 359(1444), 655-667. doi: https://doi.org/10.1098/rstb.2003.1442.
- Hassan, S. N. A., Rahman, N.N.S.A., Htike, Z. Z., & Win, S. I. (2014). Vision Based Entomology: A Survey. International Journal of Computer Science & Engineering Survey, 5(1), 19-31. doi: https://doi.org/10.5121/ijcses.2014.5103.
- Karar, M.E., Alsunaydi, F., Albusaymi, S., & Alotaibi, S. (2021). A new mobile application of agricultural pests recognition using deep learning in cloud computing. Alexandria Engineering Journal, 60, 4423–4432. doi: https://doi.org/10.1016/j.aej.2021.03.009
- Kasinathan, T., Singaraju, D., & Uyyala, S. R. (2020). Insect classification and detection in field crops using modern machine learning techniques. Information Processing in Agriculture, 8(3), 446-457. doi: https://doi.org/10.1016/j.inpa.2020.09.006.
- Kaya, Y. & Kaycı, L. (2014). Application of artificial neural network for automatic detection of butterfly species using colour and texture features. Vis Comput, 30, 71–79. doi: https://doi.org/10.1007/s00371-013-0782-8.
- Klecka, J., Hadrava, J., Biella, P., & Akter, A. (2018). Flower visitation by hoverflies (Diptera: Syrphidae) in a temperate plant-pollinator network. PeerJ, 6, e6025. doi: https://doi.org/10.7287/peerj.preprints.26516v2.
- Kočiš Tubić, N., Ståhls G., Ačanski J., Đan M., Vidaković D. O., Hayat R., Khaghaninia S., Vujić, A., & Radenković S. (2018). An integrative approach in the assessment of species delimitation and structure of the Merodon nanus species group (Diptera: Syrphidae). Organisms Diversity & Evolution, 18(4), 479-497.
- Larios, N., Deng, H., Zhang, W., Sarpola, M., Yuen, J., Paasch, R., Moldenke, A., Lytle, D. A., Correa, S. R., Mortensen, E. N., Shapiro, L. G., & Dietterich, T. G. (2008). Automated insect identification through concatenated histograms of local appearance features: feature vector generation and region detection for deformable objects. Machine Vision and Applications, 19(2), 105-123. doi: https://doi.org/ 10.1007/s00138-007-0086-y.
- Leow, L. K., Chew, L. L., Chong, V. C., & Dhillon, S. K. (2015). Automated identification of copepods using digital image processing and artificial neural network. BMC Bioinformatics, 16(18), 1-12. doi: https://doi.org/10.1186/1471-2105-16-S18-S4.
- Li, W., Zheng, T., Yang, Z., Li, M., Sun, C., & Yang, X. (2021). Classification and detection of insects from field images using deep learning for smart pest management: A systematic review. Ecological Informatics, 66, 101460.
- Likov, L., Vujić A., Kočiš Tubić N., Đan M., Veličković N., Rojo R., Pérez-Bañón C., Veselić S., Barkalov A., Hayat R., & Radenković S. (2020). Systematic position and composition of Merodon nigritarsis and M. avidus groups (Diptera, Syrphidae) with a description of four new hoverflies species. Contributions to Zoology, 89, 74-125.
- Martineau, M., Conte, D., Raveaux, R., Arnault, I., Munier, D., & Venturini, G. (2017). A survey on image-based insect classification. Pattern Recognition, 65, 273–284. doi: https://doi.org/10.1016/j.patcog.2016.12.020.
- Mayo, M. & Watson, A. T. (2007). Automatic species identification of live moths. Knowledge-Based Systems, 20(2), 195-202. doi: https://doi.org/10.1016/j.knosys.2006.11.012.
- O’Neill, M. A. (2008). DAISY: a practical tool for semi-automated species identication.” In Automated Taxon Identication in Systematics: Theory, Approaches and Applications. N. MacLeod (ed.), London, CRC Press.
- Peck, V. (1988). Family: Syrphidae. In: A. SOOS and L. Papp. (Eds), Catalogue of Palaearctic Diptera. Syrphidae-Conopidae, Budapest.
- Rotheray, G. E. & Gilbert, F. (2011). The natural history of hoverflies. Forrest Text.
- Qing, Y. A. O., Jun, L. V., Liu, Q.-J., Diao, G.-Q., Yang, B.-J., Chen, H.-M., & Tang, J. (2012). An insect imaging system to automate rice light-trap pest identification. Journal of Integrative Agriculture, 11(6), 978-985. doi: https://doi.org/10.1016/S2095-3119(12)60089-6.
- Silva, F. L. d., Grassi Sella, M. L., Francoy, T. M., & Costa, A. H. R. (2015). Evaluating classification and feature selection techniques for honeybee subspecies identification using wing images. Computers and Electronics in Agriculture, 114, 68-77. doi: https://doi.org/10.1016/j.compag.2015.03.012.
- Sommaggio, D. (1999). Syrphidae: can they be used as environmental bioindicators?. Agriculture, Ecosystems and Environment, 74, 343-356. doi: https://doi.org/10.1016/S0167-8809(99)00042-0.
- Sommaggio, D. & Burgio, G. (2014). The use of Syrphidae as functional bioindicator to compare vineyards with different managements. Bulletin of Insectology, 67(1), 147-156.
- Speight, M.C.D. (2018). StN key for the identification of the genera of European Syrphidae (Diptera) 2018. Syrph the Net, the database of European Syrphidae, 101, 1- 45.
- Speight, M.C.D. (2020). StN key for the identification of the genera of European Syrphidae (Diptera) 2020. Syrph the Net, 105, 1-46.
- Speight, M.C.D. & Sarthou, J. P. (2012). StN keys for the identification of adult European Syrphidae (Diptera) 2012. Syrph the Net, 70, 1-130.
- Thenmozhi, K. & Reddy, U. S. (2019). Crop pest classification based on deep convolutional neural network and transfer learning. Computers and Electronics in Agriculture, 164, 104906.
- Tiwari, V., Patel, H., Muttreja, R., Goyal, M., Ojha, M., Gupta, S., & Jain, S. (2021). Real-time soybean crop insect classification using customized deep learning models. Data Management, Analytics and Innovation: Proceedings of ICDMAI 2021, 1, 143-156, Springer Singapore.
- Tofiski, A. (2004). DrawWing, a program for numerical description of insect wings. Journal of Insect Science, 4(1). doi: https://doi.org/10.1093/jis/4.1.17.
- Van Driesche R., Hoddle M., & Enter T. (2008). Control of Pests and Weeds by Natural Enemies. An IntroDuction to Biological Control. Blackwell, Malden, MA & Oxford.
- Vujić, A., Likov L., Radenković S., Kočiš Tubić N., Đan M., Šebić A., Pérez-Bañón C., Barkalov A., Hayat R., Rojo S., Andrić A., & Ståhls G. (2020). Revision of Merodon serrulatus group (Diptera, Syrphidae). ZooKeys, 909, 79-158.
- Vujić, A., Radenković S., Ačanski J., Grković A., Taylor M., Şenol S. G. & Hayat R. (2015). Revision of the species of the Merodon nanus group (Diptera: Syrphidae) including three new species. Zootaxa, 4006(3), 439-462.
- Vujić, A., Nedeljković Z., Hayat R., Demirözer O., Mengual X., & Kazerani F. (2017). New data on the genus Chrysotoxum Meigen (Diptera: Syrphidae) from North-East Turkey, Armenia, Azerbaijan and Iran including descriptions of three new species. Zoology in the Middle East, 63(3), 250-268.
- Wang, J., Lin, C., Ji, L., & Liang, A. (2012). A new automatic identification system of insect images at the order level. Knowledge-Based Systems, 33, 102-110. doi: https://doi.org/10.1016/j.knosys.2012.03.014.
- Wang, J., Ji, L., Liang, A., & Decheng, Y. (2011). The identification of butterfly families using content-based image retrieval. Biosystems Engineering, 3, 24–32. doi: https://doi.org/10.1016/j.biosystemseng.2011.10.003.
- Wen, C., Guyer, D. E., & Li, W. (2009). Local feature-based identification and classification for orchard insects. Biosystems Engineering, 104(3), 299-307. doi: https://doi.org/10.1016/j.biosystemseng.2009.07.002.
- Wen, C., Wu, D., Hu, H., & Pan, W. (2015). Pose estimation-dependent identification method for field moth images using deep learning architecture. Biosystems Engineering, 136, 117-128. doi: https://doi.org/10.1016/j.biosystemseng.2015.06.002.
- Wen, C. & Zhu, Q. (2010). Dimension reduction analysis in image-based species classification. IEEE International Conference on Intelligent Computing and Intelligent Systems (ICIS), China, October 29-31.
- Xia, D., Chen, P., Wang, B., Zhang, J., & Xie, C. (2018). Insect Detection and Classification Based on an Improved Convolutional Neural Network. Sensors (Basel), 18(12), 4169. doi: https://doi.org/10.3390/s18124169.
- Yaakob, S. N. & Jain, L. (2012). An insect classification analysis based on shape features using quality threshold ARTMAP and moment invariant. Applied Intelligence, 37(1), 12-30. doi: https://doi.org/10.1007/s10489-011-0310-3.
- Yang, H.P., Ma, C.S., Wen, H., Zhan, Q. B., & Wang, X. L. (2015). A tool for developing an automatic insect identification system based on wing outlines. Scientific Reports, 5, 1-11. doi: https://doi.org/10.1038/srep12786.
- Zamora, J., Verdu, J.R., & Galante, E. (2007). Species richness in Mediterranean agroecosystems: Spatial and temporal analysis for biodiversity conservation. Biological Conservation, 134, 113–121. doi: https://doi.org/10.1016/j.biocon.2006.08.011.
- Zayas, I. Y. & Flinn, P. W. (1998). Detection of Insects in Bulk Wheat Samples with Machine Vision. Transactions of the ASAE 41(3), 883-888. doi: https://doi.org/10.13031/2013.17206.
- Zhu, L. Q., Ma, M. Y., Zhang, Z., Zhang, P. Y., Wu, W., Wang, D. D., & Wang, H. Y. (2017). Hybrid deep learning for automated lepidopteran insect image classification. Oriental Insects, 51(2), 79-91.
- Zupan, S., Bužan, E., Grubar, V. B., & Jugovic, J. 2020. Importance of traditional landscapes in Slovenia for conservation of endangered butterfly. Open Geosciences, 12, 610–625. doi: https://doi.org/10.1515/geo-2020-0179.
APA | UTKU A, AYAZ Z, Çiftçi D, Akcayol M (2023). Deep Learning Based Classification for Hoverflies (Diptera: Syrphidae). , 529 - 544. 10.51963/jers.v25i3.2445 |
Chicago | UTKU Anıl,AYAZ ZAFER,Çiftçi Derya,Akcayol M. Ali Deep Learning Based Classification for Hoverflies (Diptera: Syrphidae). (2023): 529 - 544. 10.51963/jers.v25i3.2445 |
MLA | UTKU Anıl,AYAZ ZAFER,Çiftçi Derya,Akcayol M. Ali Deep Learning Based Classification for Hoverflies (Diptera: Syrphidae). , 2023, ss.529 - 544. 10.51963/jers.v25i3.2445 |
AMA | UTKU A,AYAZ Z,Çiftçi D,Akcayol M Deep Learning Based Classification for Hoverflies (Diptera: Syrphidae). . 2023; 529 - 544. 10.51963/jers.v25i3.2445 |
Vancouver | UTKU A,AYAZ Z,Çiftçi D,Akcayol M Deep Learning Based Classification for Hoverflies (Diptera: Syrphidae). . 2023; 529 - 544. 10.51963/jers.v25i3.2445 |
IEEE | UTKU A,AYAZ Z,Çiftçi D,Akcayol M "Deep Learning Based Classification for Hoverflies (Diptera: Syrphidae)." , ss.529 - 544, 2023. 10.51963/jers.v25i3.2445 |
ISNAD | UTKU, Anıl vd. "Deep Learning Based Classification for Hoverflies (Diptera: Syrphidae)". (2023), 529-544. https://doi.org/10.51963/jers.v25i3.2445 |
APA | UTKU A, AYAZ Z, Çiftçi D, Akcayol M (2023). Deep Learning Based Classification for Hoverflies (Diptera: Syrphidae). Journal of the Entomological Research Society, 25(3), 529 - 544. 10.51963/jers.v25i3.2445 |
Chicago | UTKU Anıl,AYAZ ZAFER,Çiftçi Derya,Akcayol M. Ali Deep Learning Based Classification for Hoverflies (Diptera: Syrphidae). Journal of the Entomological Research Society 25, no.3 (2023): 529 - 544. 10.51963/jers.v25i3.2445 |
MLA | UTKU Anıl,AYAZ ZAFER,Çiftçi Derya,Akcayol M. Ali Deep Learning Based Classification for Hoverflies (Diptera: Syrphidae). Journal of the Entomological Research Society, vol.25, no.3, 2023, ss.529 - 544. 10.51963/jers.v25i3.2445 |
AMA | UTKU A,AYAZ Z,Çiftçi D,Akcayol M Deep Learning Based Classification for Hoverflies (Diptera: Syrphidae). Journal of the Entomological Research Society. 2023; 25(3): 529 - 544. 10.51963/jers.v25i3.2445 |
Vancouver | UTKU A,AYAZ Z,Çiftçi D,Akcayol M Deep Learning Based Classification for Hoverflies (Diptera: Syrphidae). Journal of the Entomological Research Society. 2023; 25(3): 529 - 544. 10.51963/jers.v25i3.2445 |
IEEE | UTKU A,AYAZ Z,Çiftçi D,Akcayol M "Deep Learning Based Classification for Hoverflies (Diptera: Syrphidae)." Journal of the Entomological Research Society, 25, ss.529 - 544, 2023. 10.51963/jers.v25i3.2445 |
ISNAD | UTKU, Anıl vd. "Deep Learning Based Classification for Hoverflies (Diptera: Syrphidae)". Journal of the Entomological Research Society 25/3 (2023), 529-544. https://doi.org/10.51963/jers.v25i3.2445 |