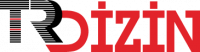
Prediction of brucellosis based on hematological biomarkers via ensemble classification methods
Yıl: 2023 Cilt: 30 Sayı: 12 Sayfa Aralığı: 1516 - 1522 Metin Dili: İngilizce DOI: 10.5455/annalsmedres.2023.10.273 İndeks Tarihi: 02-01-2024
Prediction of brucellosis based on hematological biomarkers via ensemble classification methods
Öz: Aim: Some hematological changes are frequently observed in the clinical course of brucellosis. This study aimed to predict the diagnosis of brucellosis based on some hematological biomarkers with the help of ensemble classification methods. Materials and Methods: A total of 23 ensemble classification methods, including 10 bagging, 9 boosting, and 4 stacking approaches were applied to the brucellosis data set. Each subject in the brucellosis data set contains 13 features, including age, gender, and 10 hematological variables. Results: This study included a total of 257 participants [173 (67.3%) brucellosis patients and 84 (32.7%) healthy controls]. The mean values of white blood cells (WBC), hemoglobin (HGB), neutrophil (NEUT), neutrophil/lymphocytes (NEUT/LYMP), and monocytes/lymphocytes (MO/LYMP) of brucellosis patients were found to be significantly lower than those of healthy controls. Random Forest with Gini criterion (RF2) was selected to be the best fit model with a mean accuracy of 0.728. HGB (mean score = 0.1814), age (0.1311), NEUT/LYMP (0.0938), WBC (0.0817) and mean platelet volume (MPV) (0.0815) were determined as most diagnostic parameters in brucellosis. Conclusion: The lower levels of HGB, WBC, and NEUT/LYMP and higher levels of age and MPV may be important indicators for the diagnosis of brucellosis.
Anahtar Kelime: Belge Türü: Makale Makale Türü: Araştırma Makalesi Erişim Türü: Erişime Açık
- 1. Bagheri Nejad R, Krecek RC, et al. Brucellosis in the Middle East: Current situation and a pathway forward. PLoS Negl Trop Dis. 2020;14(5):e0008071. doi:10.1371/journal.pntd.0008071.
- 2. Pappas G, Papadimitriou P, Akritidis N, et al. The new global map of human brucellosis. Lancet Infect Dis. 2006;6(2):91-9. doi:10.1016/s1473-3099(06)70382-6.
- 3. Golshani M, Buozari S. A review of Brucellosis in Iran: Epidemiology, Risk Factors, Diagnosis, Control, and Prevention. Iran Biomed J. 2017;21(6):349-59. doi:10.18869/acadpub.ibj.21.6.349.
- 4. Yagupsky P, Baron EJ. Laboratory exposures to brucellae and implications for bioterrorism. Emerg Infect Dis. 2005;11(8):1180- 5. doi:10.3201/eid1108.041197.
- 5. Buzgan T, Karahocagil MK, Irmak H, et al. Clinical manifestations and complications in 1028 cases of brucellosis: a retrospective evaluation and review of the literature. Int J Infect Dis. 2010;14(6):e469-78. doi:10.1016/j.ijid.2009.06.031.
- 6. Yagupsky P, Morata P, Colmenero JD. Laboratory Diagnosis of Human Brucellosis. Clin Microbiol Rev. 2019;33(1). doi:10.1128/cmr.00073-19.
- 7. Rawson TM, Hernandez B, Moore LSP, et al. Supervised machine learning for the prediction of infection on admission to hospital: a prospective observational cohort study. J Antimicrob Chemother. 2019;74(4):1108-15. doi:10.1093/jac/dky514.
- 8. Qiu J, Wu Q, Ding G, et al. A survey of machine learning for big data processing. EURASIP Journal on Advances in Signal Processing. 2016;2016(1):67. doi:10.1186/s13634-016-0355-x.
- 9. Hamida S, el Gannour O, Cherradi B, et al. Optimization of Machine Learning Algorithms Hyper-Parameters for Improving the Prediction of Patients Infected with COVID-19. 2020.
- 10. Esfandiari N, Babavalian MR, Moghadam A-ME, Tabar VK. Knowledge discovery in medicine: Current issue and future trend. Expert Systems with Applications. 2014;41(9):4434-63.
- 11. Hosni M, Abnane I, Idri A, et al. Reviewing ensemble classification methods in breast cancer. Comput Methods Programs Biomed. 2019;177:89-112. doi:10.1016/j.cmpb.2019.05.019.
- 12. Chandra A, Yao X. Ensemble Learning Using Multi-Objective Evolutionary Algorithms. Journal of Mathematical Modelling and Algorithms. 2006;5(4):417-45. doi:10.1007/s10852-005-9020- 3.
- 13. Troyanskaya O, Cantor M, Sherlock G, et al. Missing value estimation methods for DNA microarrays. Bioinformatics. 2001;17(6):520-5. doi:10.1093/bioinformatics/17.6.520.
- 14. Leys C, Ley C, Klein O, et al. Detecting outliers: Do not use standard deviation around the mean, use absolute deviation around the median. Journal of Experimental Social Psychology. 2013;49:764–6. doi:10.1016/j.jesp.2013.03.013.
- 15. Ren Y, Zhang L, Suganthan PN. Ensemble Classification and Regression-Recent Developments, Applications and Future Directions [Review Article]. IEEE Computational Intelligence Magazine. 2016;11:41-53.
- 16. Priasni T, Oswari T. Comparative study of standalone classifier and ensemble classifier. TELKOMNIKA (Telecommunication Computing Electronics and Control). 2021;19:1747. doi:10.12928/telkomnika.v19i5.19508.
- 17. Breiman L. Bagging predictors. Machine learning. 1996;24:123- 40.
- 18. Freund Y. Boosting a Weak Learning Algorithm by Majority. Information and Computation. 1995;121(2):256-85. doi:https://doi.org/10.1006/inco.1995.1136.
- 19. Wolpert D. Stacked Generalization. Neural Networks. 1992;5:241-59. doi:10.1016/S0893-6080(05)80023-1.
- 20. Shahhosseini M, Hu G, Pham H. Optimizing ensemble weights and hyperparameters of machine learning models for regression problems. Machine Learning with Applications. 2022;7:100251. doi:10.1016/j.mlwa.2022.100251.
- 21. Özkan Y, Demirarslan M, Suner A. Effect of data preprocessing on ensemble learning for classification in disease diagnosis. Communications in Statistics-Simulation and Computation. 2022:1- 21.
- 22. Breiman L. Random Forests. Machine Learning. 2001;45:5-32. doi:10.1023/A:1010950718922.
- 23. Akya A, Bozorgomid A, Ghadiri K, et al. Usefulness of Blood Parameters for Preliminary Diagnosis of Brucellosis. J Blood Med. 2020;11:107-13. doi:10.2147/jbm.S245513.
- 24. Çelik M, Ceylan M, Altındağ D, et al. Diagnostic significance of hematological parameters in brucellosis. Journal of Clinical Medicine of Kazakhstan. 2023;20:50-5. doi:10.23950/jcmk/12929.
- 25. El-Koumi MAM, Afify MM, Al-Zahrani SHM. A prospective study of brucellosis in children: relative frequency of pancytopenia. Iran J Pediatr. 2014;24(2):155-60.
- 26. Olt S, Ergenç H, Açıkgöz SB. Predictive contribution of neutrophil/lymphocyte ratio in diagnosis of brucellosis. Biomed Res Int. 2015;2015:210502. doi:10.1155/2015/210502.
- 27. Bozdemir Ş E, Altıntop YA, Uytun S, et al. Diagnostic role of mean platelet volume and neutrophil to lymphocyte ratio in childhood brucellosis. Korean J Intern Med. 2017;32(6):1075-81. doi:10.3904/kjim.2016.092.
- 28. Yan Y, Chen C, Liu Y, et al. Application of Machine Learning for the Prediction of Etiological Types of Classic Fever of Unknown Origin. Front Public Health. 2021;9:800549. doi:10.3389/fpubh.2021.800549.
- 29. Abbasi B, Goldenholz DM. Machine learning applications in epilepsy. Epilepsia. 2019;60(10):2037-47. doi:10.1111/epi.16333.
- 30. Allen A, Mataraso S, Siefkas A, et al. A Racially Unbiased, Machine Learning Approach to Prediction of Mortality: Algorithm Development Study. JMIR Public Health Surveill. 2020;6(4):e22400. doi:10.2196/22400.
- 31. Jo YT, Joo SW, Shon SH, et al. Diagnosing schizophrenia with network analysis and a machine learning method. Int J Methods Psychiatr Res. 2020;29(1):e1818. doi:10.1002/mpr.1818.
- 32. Brinati D, Campagner A, Ferrari D, et al. Detection of COVID-19 Infection from Routine Blood Exams with Machine Learning: A Feasibility Study. J Med Syst. 2020;44(8):135. doi:10.1007/s10916-020-01597-4.
- 33. Chicco D, Jurman G. An Ensemble Learning Approach for Enhanced Classification of Patients With Hepatitis and Cirrhosis. IEEE Access. 2021;PP:1-. doi:10.1109/ACCESS.2021.3057196.
- 34. Meng D, Xu J, Zhao J. Analysis and prediction of hand, foot and mouth disease incidence in China using Random Forest and XGBoost. PLoS One. 2021;16(12):e0261629. doi:10.1371/journal.pone.0261629.
- 35. Alim M, Ye GH, Guan P, et al. Comparison of ARIMA model and XGBoost model for prediction of human brucellosis in mainland China: a time-series study. BMJ Open. 2020;10(12):e039676. doi:10.1136/bmjopen-2020-039676.
APA | ŞAHİN A, ÇELİK M, REŞAT M, ALTINDAĞ D, Gürbüz E, güler n, ALKAN S (2023). Prediction of brucellosis based on hematological biomarkers via ensemble classification methods. , 1516 - 1522. 10.5455/annalsmedres.2023.10.273 |
Chicago | ŞAHİN AHMET,ÇELİK MEHMET,REŞAT MEHMET,ALTINDAĞ DENİZ,Gürbüz Esra,güler nevin,ALKAN Sevil Prediction of brucellosis based on hematological biomarkers via ensemble classification methods. (2023): 1516 - 1522. 10.5455/annalsmedres.2023.10.273 |
MLA | ŞAHİN AHMET,ÇELİK MEHMET,REŞAT MEHMET,ALTINDAĞ DENİZ,Gürbüz Esra,güler nevin,ALKAN Sevil Prediction of brucellosis based on hematological biomarkers via ensemble classification methods. , 2023, ss.1516 - 1522. 10.5455/annalsmedres.2023.10.273 |
AMA | ŞAHİN A,ÇELİK M,REŞAT M,ALTINDAĞ D,Gürbüz E,güler n,ALKAN S Prediction of brucellosis based on hematological biomarkers via ensemble classification methods. . 2023; 1516 - 1522. 10.5455/annalsmedres.2023.10.273 |
Vancouver | ŞAHİN A,ÇELİK M,REŞAT M,ALTINDAĞ D,Gürbüz E,güler n,ALKAN S Prediction of brucellosis based on hematological biomarkers via ensemble classification methods. . 2023; 1516 - 1522. 10.5455/annalsmedres.2023.10.273 |
IEEE | ŞAHİN A,ÇELİK M,REŞAT M,ALTINDAĞ D,Gürbüz E,güler n,ALKAN S "Prediction of brucellosis based on hematological biomarkers via ensemble classification methods." , ss.1516 - 1522, 2023. 10.5455/annalsmedres.2023.10.273 |
ISNAD | ŞAHİN, AHMET vd. "Prediction of brucellosis based on hematological biomarkers via ensemble classification methods". (2023), 1516-1522. https://doi.org/10.5455/annalsmedres.2023.10.273 |
APA | ŞAHİN A, ÇELİK M, REŞAT M, ALTINDAĞ D, Gürbüz E, güler n, ALKAN S (2023). Prediction of brucellosis based on hematological biomarkers via ensemble classification methods. Annals of Medical Research, 30(12), 1516 - 1522. 10.5455/annalsmedres.2023.10.273 |
Chicago | ŞAHİN AHMET,ÇELİK MEHMET,REŞAT MEHMET,ALTINDAĞ DENİZ,Gürbüz Esra,güler nevin,ALKAN Sevil Prediction of brucellosis based on hematological biomarkers via ensemble classification methods. Annals of Medical Research 30, no.12 (2023): 1516 - 1522. 10.5455/annalsmedres.2023.10.273 |
MLA | ŞAHİN AHMET,ÇELİK MEHMET,REŞAT MEHMET,ALTINDAĞ DENİZ,Gürbüz Esra,güler nevin,ALKAN Sevil Prediction of brucellosis based on hematological biomarkers via ensemble classification methods. Annals of Medical Research, vol.30, no.12, 2023, ss.1516 - 1522. 10.5455/annalsmedres.2023.10.273 |
AMA | ŞAHİN A,ÇELİK M,REŞAT M,ALTINDAĞ D,Gürbüz E,güler n,ALKAN S Prediction of brucellosis based on hematological biomarkers via ensemble classification methods. Annals of Medical Research. 2023; 30(12): 1516 - 1522. 10.5455/annalsmedres.2023.10.273 |
Vancouver | ŞAHİN A,ÇELİK M,REŞAT M,ALTINDAĞ D,Gürbüz E,güler n,ALKAN S Prediction of brucellosis based on hematological biomarkers via ensemble classification methods. Annals of Medical Research. 2023; 30(12): 1516 - 1522. 10.5455/annalsmedres.2023.10.273 |
IEEE | ŞAHİN A,ÇELİK M,REŞAT M,ALTINDAĞ D,Gürbüz E,güler n,ALKAN S "Prediction of brucellosis based on hematological biomarkers via ensemble classification methods." Annals of Medical Research, 30, ss.1516 - 1522, 2023. 10.5455/annalsmedres.2023.10.273 |
ISNAD | ŞAHİN, AHMET vd. "Prediction of brucellosis based on hematological biomarkers via ensemble classification methods". Annals of Medical Research 30/12 (2023), 1516-1522. https://doi.org/10.5455/annalsmedres.2023.10.273 |