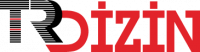
Topluluk Ve Derin Öğrenme Teknikleri Ile X-Ray Görüntülerinden Patlayıcı Devre Tespiti
Proje Grubu: EEEAG Sayfa Sayısı: 98 Proje No: 122E024 Proje Bitiş Tarihi: 15.10.2023 Metin Dili: Türkçe DOI: 122E024 İndeks Tarihi: 25-01-2024
Topluluk Ve Derin Öğrenme Teknikleri Ile X-Ray Görüntülerinden Patlayıcı Devre Tespiti
Öz: Patlayıcı madde tespiti özellikle havaalanı vb. yerlerde terör saldırılarının önlenerek güvenliğin sağlanması hususunda hayati öneme sahiptir. Halen bu yerlerde yolcuların ve bagajlarının taranarak X-ray vb. görüntüler üzerinden kontrol edilmesi güvenlik uzmanları tarafından manuel gerçekleştirilmektedir. Bu konu ve bu sürecin otomatikleştirilmesi ile alakalı çok sayıda araştırma, makale ve yöntem geliştirilmiştir. Nesne tespiti maksadıyla X-ray, gamma ışını (ray), elektromanyetik alan ve milimetrik dalgalar gibi birçok teknik ve teknolojiler kullanılmaktadır. Özellikle X-ray görüntüleme maddelerin tabi tutulduğu x-ışınının emilimine göre elde edilen görüntüdeki koyuluk veya parlaklıkları ile farklı maddelerin yansımalarının belirlenmesi olarak adlandırılabilir. Elde edilen bu görüntüler nesne belirleme, hastalık belirleme vb. çok farklı alanlarda ve amaçlarda kullanılmaktadır. Türkiye?de ve dünyada son yıllarda terör saldırılarının artışı ile birlikte havaalanı, alışveriş merkezleri, bakanlıklar gibi özel ve devlet kurumlarının güvenlik ihtiyacı vazgeçilmez hale gelmiştir. Bu kuruluşlarda güvenlik X-ray cihazları vasıtasıyla çanta ve bagaj içerisindeki eşyaların iki boyutlu veya üç boyutlu görüntüleri elde edilerek bir ekrana yansıtılması ve bu ekrana bakan bir insan uzman tarafından tehlikeli maddelerin tespit edilmesi şeklinde sağlanmaktadır. Ancak yapılan bu işlem zaman darlığı, iş yoğunluğu, uzman personel eksikliği gibi nedenlerden dolayı verimli ve/veya yeteri kadar güvenli olamamaktadır. Kontrolleri gerçekleştiren görevlilerin dikkatlerini sürekli bu ekranda tutmaları ve dikkatlerinin dağılmaması gerekmektedir. Ayrıca patlayıcı maddeler ve ilgili devre elemanlarının bu görüntü üzerinden tespiti oldukça zordur. Özellikle çanta ve bagajlarda eşyalar üst üste ve yan yana gelerek görüntüde tespit zorluğuna sebep olmaktadırlar. X-ray cihazlarından elektronik cihazlar (özellikle dizüstü bilgisayarlar) ayrı kutularda geçirilmesine rağmen görüntüyü takip eden uzman personelin elektronik cihazın içerisinde gizlenmiş bir bomba düzeneğini varsa belirlemesi beklenmektedir. Bu ise elektronik mühendisi veya bilgisayar mühendisi gibi dizüstü bilgisayarın içerisinde olması muhtemel kartları bilen bir uzman tarafından dahi zorlukla gerçekleştirilebilecek bir işlemdir. Bu proje çalışmasında ilgili sürecin görüntü işleme ve derin öğrenme vasıtasıyla otomatikleştirilip hata oranın en aza indirilmesi hedeflenmektedir. Proje kapsamında dizüstü bilgisayarın X-ray görüntüsü üzerinden gizlenen patlayıcı devre tespiti amaçlanmıştır. Bu amacı yerine getirebilmek maksadıyla belirli sayıda dizüstü bilgisayarın içine farklı devreler yerleştirilmiş ve farklı açılarda ve yerleşimlerde kutularla birlikte X-Ray cihazından görüntüler elde edilmiştir. Elde edilen bu görüntülerden oluşan veri seti, derin öğrenme yöntemlerinin eğitimi için kullanılmış ve görüntüler bu eğitilmiş ağlar ile sınıflandırılmıştır. Performansı artırmak amacıyla eğitilmiş ağlar topluluk stratejisi ile birleştirilmiş aynı veri seti üzerinde tekrar test edilmiştir. Ayrıca eğitilmiş ağlardan özellikler çıkarılmış, seçilmiş ve tekrar birleştirilmiştir. Bu oluşturulan birleştirilmiş veri seti tekrar eğitim ve sınıflandırma amacıyla kullanılmıştır. Son deneyde oluşturulan veri seti üzerinde 96,56% eğitim başarısı ve 96,46% test başarısı elde edilmiştir. Son olarak görüntülerde devrelerin konumlarını tespit etmek amacıyla derin öğrenme tabanlı bir uygulama geliştirilmiştir.
Anahtar Kelime: Explosive Circuit Detection from X-Ray Images using Ensemble and Deep Learning Techniques
Öz: Detecting explosive materials is of vital importance in places like airports to prevent terrorist attacks and ensure security. Currently, the security experts manually scan passengers and their luggage through X-ray and other imaging techniques at these locations. Some research, articles, and methods have been developed to automate this process. Various techniques and technologies, such as X-ray, gamma rays, electromagnetic fields, and millimeter waves are used for object detection from this type of images. In particular, X-ray imaging can be described as determining the reflections of different materials based on the absorption of X-rays that substances are subjected to, resulting in the darkness or brightness in the obtained image. These images are used for object identification, disease diagnosis, and various other purposes in different fields. In recent years, with the increase in terrorist attacks both in Turkey and worldwide, the need for security in places such as airports, shopping centers, and government institutions like ministries has become indispensable. In these organizations, security is achieved by obtaining two- or three-dimensional images of items inside bags and luggage using X-ray devices, displaying them on a screen, and having a human expert examine the screen to detect dangerous materials. However, this process is not efficient and/or secure enough due to factors such as time constraints, workload, and a shortage of expert personnel. Those responsible for the inspections must keep their attention on the screen continuously and not be distracted. Additionally, the detection of explosive materials and related circuit components from these images is quite challenging. Especially when items in bags and luggage are stacked on top of each other or placed side by side, it makes detection in the image difficult. Although electronic devices (especially laptops) are passed through X-ray machines in separate containers, the expert personnel following the image are expected to identify a hidden explosive circuit inside the electronic device, even if there is one. This is a task that can be challenging even for an expert who knows the possible circuits that might be inside a laptop, such as an electronic engineer or computer engineer. The aim of this project is to automate the relevant process using image processing and deep learning to minimize the error rate. In the project, the detection of explosive circuits hidden within laptop X-ray images is aimed for. To achieve this goal, different circuits were placed inside a certain number of laptops, and images were obtained from the X-ray device with different angles and arrangements, along with boxes. The dataset consisting of these images was used to train deep learning methods, and the images were classified using these trained networks. To improve classification performance, trained networks were combined using an ensemble strategy and tested again on the same data set. Additionally, features were extracted from the trained networks, selected, and recombined. This combined data set was used for retraining and classification purposes. In the final experiment, a training success rate of 96.56% and a test success rate of 96.46% were achieved on the created data set. Finally, a deep learning-based application was developed to detect the locations of circuits in the images.
Anahtar Kelime: Erişim Türü: Bibliyografik
- Akay, B. ,Karaboga, D. 2012. "A modified artificial bee colony algorithm for real-parameter optimization", Information sciences, 192, 120-142.
- Akcay, S., Atapour-Abarghouei, A. ,Breckon, T. P. 2018a. "Ganomaly: Semi-supervised anomaly detection via adversarial training", Asian conference on computer vision, Springer.
- Akcay, S. ,Breckon, T. P. 2017. "An evaluation of region based object detection strategies within x-ray baggage security imagery", 2017 IEEE International Conference on Image Processing (ICIP), IEEE.
- Akcay, S., Kundegorski, M. E., Willcocks, C. G. ,Breckon, T. P. 2018b. "Using deep convolutional neural network architectures for object classification and detection within x-ray baggage security imagery", IEEE transactions on information forensics and security, 13 (9), 2203-2215.
- Akçay, S., Kundegorski, M. E., Devereux, M. ,Breckon, T. P. 2016. "Transfer learning using convolutional neural networks for object classification within x-ray baggage security imagery", 2016 IEEE International Conference on Image Processing (ICIP), IEEE.
- ALTUN, S. ,TALU, M. F. 2021. "Derin sinir ağları için hiperparametre metodlarının ve kitlerinin incelenmesi", Dicle Üniversitesi Mühendislik Fakültesi Mühendislik Dergisi, 12 (2), 187-199.
- Andrews, J. T., Jaccard, N., Rogers, T. W. ,Griffin, L. D. 2017. "Representation-learning for anomaly detection in complex x-ray cargo imagery", Anomaly Detection and Imaging with X-Rays (ADIX) II, International Society for Optics and Photonics.
- AYDIN, I., KARAKOSE, M. ,Erhan, A. 2018. "A new approach for baggage inspection by using deep convolutional neural networks", 2018 International Conference on Artificial Intelligence and Data Processing (IDAP), IEEE.
- Benedykciuk, E., Denkowski, M. ,Dmitruk, K. 2021. "Material classification in X-ray images based on multi-scale CNN", Signal, Image and Video Processing, 1-9.
- Bergstra, J., Bardenet, R., Bengio, Y. ,Kégl, B. 2011. "Algorithms for hyper-parameter optimization", Advances in neural information processing systems, 24.
- Bhowmik, N., Gaus, Y. F. A., Akçay, S., Barker, J. W. ,Breckon, T. P. 2019a. "On the impact of object and sub-component level segmentation strategies for supervised anomaly detection within x-ray security imagery", 2019 18th IEEE International Conference On Machine Learning And Applications (ICMLA), IEEE.
- Bhowmik, N., Gaus, Y. F. A. ,Breckon, T. P. 2019b. "Using deep neural networks to address the evolving challenges of concealed threat detection within complex electronic items", 2019 IEEE International Symposium on Technologies for Homeland Security (HST), IEEE.
- Bhowmik, N., Wang, Q., Gaus, Y. F. A., Szarek, M. ,Breckon, T. P. 2019c. "The good, the bad and the ugly: Evaluating convolutional neural networks for prohibited item detection using real and synthetically composited X-ray imagery", arXiv preprint arXiv:1909.11508.
- Brochu, E., Cora, V. M. ,De Freitas, N. 2010. "A tutorial on Bayesian optimization of expensive cost functions, with application to active user modeling and hierarchical reinforcement learning", arXiv preprint arXiv:1012.2599.
- Caldwell, M. ,Griffin, L. D. 2019. "Limits on transfer learning from photographic image data to X-ray threat detection", Journal of X-ray science and technology, 27 (6), 1007-1020.
- Caldwell, M., Ransley, M., Rogers, T. W. ,Griffin, L. D. 2017. "Transferring x-ray based automated threat detection between scanners with different energies and resolution", Counterterrorism, Crime Fighting, Forensics, and Surveillance Technologies, International Society for Optics and Photonics.
- Cao, C., Huang, Y., Yang, Y., Wang, L., Wang, Z. ,Tan, T. 2018. "Feedback convolutional neural network for visual localization and segmentation", IEEE transactions on pattern analysis and machine intelligence, 41 (7), 1627-1640.
- Chang, A., Zhang, Y., Zhang, S., Zhong, L. ,Zhang, L. 2022. "Detecting prohibited objects with physical size constraint from cluttered X-ray baggage images", Knowledge-Based Systems, 237, 107916.
- Chollet, F. 2017. "Xception: Deep learning with depthwise separable convolutions", Proceedings of the IEEE conference on computer vision and pattern recognition.
- Chouai, M., Merah, M. ,Mimi, M. 2020. "CH-Net: Deep adversarial autoencoders for semantic segmentation in X-ray images of cabin baggage screening at airports", Journal of Transportation Security, 13 (1), 71-89.
- Dumagpi, J. K. ,Jeong, Y.-J. 2021a. "Evaluating GAN-Based Image Augmentation for Threat Detection in Large-Scale Xray Security Images", Applied Sciences, 11 (1), 36.
- Dumagpi, J. K. ,Jeong, Y.-J. 2021b. "Pixel-Level Analysis for Enhancing Threat Detection in Large-Scale X-ray Security Images", Applied Sciences, 11 (21), 10261.
- Dumagpi, J. K., Jung, W.-Y. ,Jeong, Y.-J. 2020. "A new GAN-based anomaly detection (GBAD) approach for multi-threat object classification on large-scale x-ray security images", IEICE TRANSACTIONS on Information and Systems, 103 (2), 454-458.
- Gaus, Y. F. A., Bhowmik, N., Akcay, S. ,Breckon, T. 2019a. "Evaluating the transferability and adversarial discrimination of convolutional neural networks for threat object detection and classification within x-ray security imagery", 2019 18th IEEE International Conference On Machine Learning And Applications (ICMLA), IEEE.
- Gaus, Y. F. A., Bhowmik, N., Akçay, S., Guillén-Garcia, P. M., Barker, J. W. ,Breckon, T. P. 2019b. "Evaluation of a dual convolutional neural network architecture for object-wise anomaly detection in cluttered X-ray security imagery", 2019 International Joint Conference on Neural Networks (IJCNN), IEEE.
- Gaus, Y. F. A., Bhowmik, N. ,Breckon, T. P. 2019c. "On the use of deep learning for the detection of firearms in x-ray baggage security imagery", 2019 IEEE International Symposium on Technologies for Homeland Security (HST), IEEE.
- Girshick, R., Donahue, J., Darrell, T. ,Malik, J. 2014. "Rich feature hierarchies for accurate object detection and semantic segmentation", Proceedings of the IEEE conference on computer vision and pattern recognition.
- Griffin, L. D., Caldwell, M., Andrews, J. T. ,Bohler, H. 2018. "“unexpected item in the bagging area”: Anomaly detection in x-ray security images", IEEE transactions on information forensics and security, 14 (6), 1539-1553.
- Guo, Y., Liu, Y., Oerlemans, A., Lao, S., Wu, S. ,Lew, M. S. 2016. "Deep learning for visual understanding: A review", Neurocomputing, 187, 27-48.
- He, K., Zhang, X., Ren, S. ,Sun, J. 2016. "Deep residual learning for image recognition", Proceedings of the IEEE conference on computer vision and pattern recognition.
- Howard, A. G., Zhu, M., Chen, B., Kalenichenko, D., Wang, W., Weyand, T., Andreetto, M. ,Adam, H. 2017. "Mobilenets: Efficient convolutional neural networks for mobile vision applications", arXiv preprint arXiv:1704.04861.
- Huang, G.-B., Zhu, Q.-Y. ,Siew, C.-K. 2004. "Extreme learning machine: a new learning scheme of feedforward neural networks", 2004 IEEE international joint conference on neural networks (IEEE Cat. No. 04CH37541), Ieee.
- Huang, G.-B., Zhu, Q.-Y. ,Siew, C.-K. 2006. "Extreme learning machine: theory and applications", Neurocomputing, 70 (1-3), 489-501.
- Huang, G., Liu, Z., Van Der Maaten, L. ,Weinberger, K. Q. 2017. "Densely connected convolutional networks", Proceedings of the IEEE conference on computer vision and pattern recognition.
- Hutter, F., Kotthoff, L. ,Vanschoren, J. 2019. "Automated machine learning: methods, systems, challenges", Springer Nature.
- Jaccard, N., Rogers, T. W., Morton, E. J. ,Griffin, L. D. 2016. "Tackling the X-ray cargo inspection challenge using machine learning", Anomaly Detection and Imaging with X-Rays (ADIX), International Society for Optics and Photonics.
- Jaccard, N., Rogers, T. W., Morton, E. J. ,Griffin, L. D. 2017. "Detection of concealed cars in complex cargo X-ray imagery using deep learning", Journal of X-ray science and technology, 25 (3), 323-339.
- Jain, D. K. 2019. "An evaluation of deep learning based object detection strategies for threat object detection in baggage security imagery", pattern recognition letters, 120, 112-119.
- Janiesch, C., Zschech, P. ,Heinrich, K. 2021. "Machine learning and deep learning", Electronic Markets, 31 (3), 685-695.
- Jia, D., Duan, X. ,Khan, M. K. 2014. "Binary artificial bee colony optimization using bitwise operation", Computers & Industrial Engineering, 76, 360-365.
- Karaboga, D. 2005. "An idea based on honey bee swarm for numerical optimization", Technical report- tr06, Erciyes university, engineering faculty, computer ....
- Karaboga, D., Akay, B. ,Ozturk, C. 2007. "Artificial bee colony (ABC) optimization algorithm for training feed-forward neural networks", Modeling Decisions for Artificial Intelligence: 4th International Conference, MDAI 2007, Kitakyushu, Japan, August 16-18, 2007. Proceedings 4, Springer.
- Kashan, M. H., Nahavandi, N. ,Kashan, A. H. 2012. "DisABC: a new artificial bee colony algorithm for binary optimization", Applied Soft Computing, 12 (1), 342-352.
- Keihani, A., Sajadi, S. S., Hasani, M. ,Ferrarelli, F. 2022. "Bayesian optimization of machine learning classification of resting-state EEG microstates in schizophrenia: a proof-of-concept preliminary study based on secondary analysis", Brain Sciences, 12 (11), 1497.
- Kiran, M. S. 2015. "The continuous artificial bee colony algorithm for binary optimization", Applied Soft Computing, 33, 15-23.
- Kiran, M. S. 2021. "A binary artificial bee colony algorithm and its performance assessment", Expert Systems with Applications, 175, 114817.
- Kiran, M. S. ,Gündüz, M. 2013. "XOR-based artificial bee colony algorithm for binary optimization", Turkish Journal of Electrical Engineering and Computer Sciences, 21 (8), 2307-2328.
- Krizhevsky, A., Sutskever, I. ,Hinton, G. E. 2012. "Imagenet classification with deep convolutional neural networks", Advances in neural information processing systems, 25.
- LeCun, Y., Bottou, L., Bengio, Y. ,Haffner, P. 1998. "Gradient-based learning applied to document recognition", Proceedings of the IEEE, 86 (11), 2278-2324.
- LeCun, Y., Jackel, L., Bottou, L., Brunot, A., Cortes, C., Denker, J., Drucker, H., Guyon, I., Muller, U. ,Sackinger, E. 1995. "Comparison of learning algorithms for handwritten digit recognition", International conference on artificial neural networks, Perth, Australia.
- Liang, K. J., Sigman, J. B., Spell, G. P., Strellis, D., Chang, W., Liu, F., Mehta, T. ,Carin, L. 2019. "Toward automatic threat recognition for airport X-ray baggage screening with deep convolutional object detection", arXiv preprint arXiv:1912.06329.
- Liu, D., Liu, J., Yuan, P. ,Yu, F. 2022a. "A data augmentation method for prohibited item x-ray pseudocolor images in x-ray security inspection based on Wasserstein generative adversarial network and spatial-and-channel attention block", Computational intelligence and neuroscience, 2022.
- Liu, D., Liu, J., Yuan, P. ,Yu, F. 2022b. "A Lightweight Dangerous Liquid Detection Method Based on Depthwise Separable Convolution for X-Ray Security Inspection", Computational intelligence and neuroscience, 2022.
- Liu, J., Leng, X. ,Liu, Y. 2019. "Deep convolutional neural network based object detector for X-Ray baggage security imagery", 2019 IEEE 31st International Conference on Tools with Artificial Intelligence (ICTAI), IEEE.
- Liu, Z., Li, J., Shu, Y. ,Zhang, D. 2018. "Detection and recognition of security detection object based on YOLO9000", 2018 5th International Conference on Systems and Informatics (ICSAI), IEEE.
- Ma, B., Jia, T., Su, M., Jia, X., Chen, D. ,Zhang, Y. 2022a. "Automated Segmentation of Prohibited Items in X-ray Baggage Images Using Dense De-overlap Attention Snake", IEEE Transactions on Multimedia.
- Ma, C., Zhuo, L., Li, J., Zhang, Y. ,Zhang, J. 2022b. "EAOD Net: Effective anomaly object detection networks for X ray images", IET Image Processing.
- MathWorks. 2023. "fitcauto." 2023, from https://www.mathworks.com/help/stats/fitcauto.html. Mery, D., Kaminetzky, A., Golborne, L., Figueroa, S. ,Saavedra, D. 2022. "Target Detection by Target Simulation in X-ray Testing", Journal of Nondestructive Evaluation, 41 (1), 1-12.
- Mery, D., Riffo, V., Zuccar, I. ,Pieringer, C. 2013. "Automated X-ray object recognition using an efficient search algorithm in multiple views", Proceedings of the IEEE conference on computer vision and pattern recognition workshops.
- Mery, D., Svec, E., Arias, M., Riffo, V., Saavedra, J. M. ,Banerjee, S. 2016. "Modern computer vision techniques for x-ray testing in baggage inspection", IEEE Transactions on Systems, Man, and Cybernetics: Systems, 47 (4), 682-692.
- Miao, C., Xie, L., Wan, F., Su, C., Liu, H., Jiao, J. ,Ye, Q. 2019. "Sixray: A large-scale security inspection x-ray benchmark for prohibited item discovery in overlapping images", Proceedings of the IEEE/CVF Conference on Computer Vision and Pattern Recognition.
- Močkus, J. 1975. "On Bayesian methods for seeking the extremum", Optimization Techniques IFIP Technical Conference: Novosibirsk, July 1–7, 1974, Springer.
- Morris, T., Chien, T. ,Goodman, E. 2018. "Convolutional neural networks for automatic threat detection in security X-Ray images", 2018 17th IEEE International Conference on Machine Learning and Applications (ICMLA), IEEE.
- Nguyen, H. D., Cai, R., Zhao, H., Kot, A. C. ,Wen, B. 2022. "Towards More Efficient Security Inspection via Deep Learning: A Task-Driven X-ray Image Cropping Scheme", Micromachines, 13 (4), 565.
- Pampará, G. ,Engelbrecht, A. P. 2011. "Binary artificial bee colony optimization", 2011 IEEE Symposium on Swarm Intelligence, IEEE.
- Petrozziello, A. ,Jordanov, I. 2019. "Automated deep learning for threat detection in luggage from X-ray images", International Symposium on Experimental Algorithms, Springer.
- Rawat, W. ,Wang, Z. 2017. "Deep convolutional neural networks for image classification: A comprehensive review", Neural computation, 29 (9), 2352-2449.
- Redmon, J., Divvala, S., Girshick, R. ,Farhadi, A. 2016. "You only look once: Unified, real-time object detection", Proceedings of the IEEE conference on computer vision and pattern recognition.
- Redmon, J. ,Farhadi, A. 2017. "YOLO9000: better, faster, stronger. In proceedings of the IEEE conference on computer vision and pattern recognition (pp. 7263-7271)".
- Redmon, J. ,Farhadi, A. 2018. "Yolov3: An incremental improvement", arXiv preprint arXiv:1804.02767.
- Rogers, T. W., Jaccard, N. ,Griffin, L. D. 2017. "A deep learning framework for the automated inspection of complex dual-energy x-ray cargo imagery", Anomaly Detection and Imaging with X-Rays (ADIX) II, International Society for Optics and Photonics.
- Roy, A. G., Navab, N. ,Wachinger, C. 2018. "Concurrent spatial and channel ‘squeeze & excitation’in fully convolutional networks", International conference on medical image computing and computer-assisted intervention, Springer.
- Saavedra, D., Banerjee, S. ,Mery, D. 2020. "Detection of threat objects in baggage inspection with X- ray images using deep learning", Neural Computing and Applications, 1-17.
- Santana Jr, C. J., Macedo, M., Siqueira, H., Gokhale, A. ,Bastos-Filho, C. J. 2019. "A novel binary artificial bee colony algorithm", Future Generation Computer Systems, 98, 180-196.
- Shao, F., Liu, J., Wu, P., Yang, Z. ,Wu, Z. 2022. "Exploiting foreground and background separation for prohibited item detection in overlapping X-Ray images", Pattern Recognition, 122, 108261.
- Sigman, J. B., Spell, G. P., Liang, K. J. ,Carin, L. 2020. "Background adaptive faster R-CNN for semi- supervised convolutional object detection of threats in x-ray images", Anomaly Detection and Imaging with X-Rays (ADIX) V, International Society for Optics and Photonics.
- Singh, S. ,Singh, M. 2003. "Explosives detection systems (EDS) for aviation security", Signal processing, 83 (1), 31-55.
- Snoek, J., Larochelle, H. ,Adams, R. P. 2012. "Practical bayesian optimization of machine learning algorithms", Advances in neural information processing systems, 25.
- Steitz, J.-M. O., Saeedan, F. ,Roth, S. 2018. "Multi-view x-ray R-CNN", German Conference on Pattern Recognition, Springer.
- Subramani, M., Rajaduari, K., Choudhury, S. D., Topkar, A. ,Ponnusamy, V. 2020. "Evaluating One Stage Detector Architecture of Convolutional Neural Network for Threat Object Detection Using X- Ray Baggage Security Imaging", Revue d'Intelligence Artificielle, 34 (4), 495-500.
- Szegedy, C., Liu, W., Jia, Y., Sermanet, P., Reed, S., Anguelov, D., Erhan, D., Vanhoucke, V. ,Rabinovich, A. 2015. "Going deeper with convolutions", Proceedings of the IEEE conference on computer vision and pattern recognition.
- Szegedy, C., Vanhoucke, V., Ioffe, S., Shlens, J. ,Wojna, Z. 2016. "Rethinking the inception architecture for computer vision", Proceedings of the IEEE conference on computer vision and pattern recognition.
- Tan, M. ,Le, Q. 2019. "Efficientnet: Rethinking model scaling for convolutional neural networks", International conference on machine learning, PMLR.
- Wang, Q., Bhowmik, N. ,Breckon, T. P. 2020. "Multi-Class 3D Object Detection Within Volumetric 3D Computed Tomography Baggage Security Screening Imagery", arXiv preprint arXiv:2008.01218.
- Wei, Y. ,Liu, X. 2020. "Dangerous goods detection based on transfer learning in X-ray images", Neural Computing and Applications, 32 (12), 8711-8724.
- Wikipedia. 26.08.2022. "X-ray." Retrieved 26.08.2022, from https://en.wikipedia.org/wiki/X-ray. Wu, J. ,Liao, S. 2022. "Intelligent Detection of Dangerous Goods in Security Inspection Based on Cascade Cross Stage YOLOv3 Model", Tehnički vjesnik, 29 (3), 888-895.
- Wu, Z., Shen, C. ,Van Den Hengel, A. 2019. "Wider or deeper: Revisiting the resnet model for visual recognition", Pattern Recognition, 90, 119-133.
- Xu, M., Zhang, H. ,Yang, J. 2018. "Prohibited item detection in airport X-ray security images via attention mechanism based CNN", Chinese Conference on Pattern Recognition and Computer Vision (PRCV), Springer.
- Yang, J., Zhao, Z., Zhang, H. ,Shi, Y. 2019. "Data augmentation for X-ray prohibited item images using generative adversarial networks", IEEE Access, 7, 28894-28902.
- Yao, S.-q., Su, Z.-g., Yang, J.-f. ,Zhang, H.-g. 2021. "A prohibited items identification approach based on semantic segmentation", Optoelectronics Letters, 17 (4), 247-251.
- Zhang, D., Guan, X., Tang, Y. ,Tang, Y. 2011. "Modified artificial bee colony algorithms for numerical optimization", 2011 3rd International Workshop on Intelligent Systems and Applications, IEEE.
- Zhang, X., Zhou, X., Lin, M. ,Sun, J. 2018. "Shufflenet: An extremely efficient convolutional neural network for mobile devices", Proceedings of the IEEE conference on computer vision and pattern recognition.
- Zhao, C., Zhu, L., Dou, S., Deng, W. ,Wang, L. 2022. "Detecting Overlapped Objects in X-Ray Security Imagery by a Label-Aware Mechanism", IEEE transactions on information forensics and security, 17, 998-1009.
- Zhou, Z.-H. 2021. "Machine learning", Springer Nature.
- Zou, L., Yusuke, T. ,Hitoshi, I. 2018. "Dangerous objects detection of X-ray images using convolution neural network", International Conference on Security with Intelligent Computing and Big-data Services, Springer
APA | Kıran M, Eşme E (2023). Topluluk Ve Derin Öğrenme Teknikleri Ile X-Ray Görüntülerinden Patlayıcı Devre Tespiti. , 0 - 98. 122E024 |
Chicago | Kıran Mustafa Servet,Eşme Engin Topluluk Ve Derin Öğrenme Teknikleri Ile X-Ray Görüntülerinden Patlayıcı Devre Tespiti. (2023): 0 - 98. 122E024 |
MLA | Kıran Mustafa Servet,Eşme Engin Topluluk Ve Derin Öğrenme Teknikleri Ile X-Ray Görüntülerinden Patlayıcı Devre Tespiti. , 2023, ss.0 - 98. 122E024 |
AMA | Kıran M,Eşme E Topluluk Ve Derin Öğrenme Teknikleri Ile X-Ray Görüntülerinden Patlayıcı Devre Tespiti. . 2023; 0 - 98. 122E024 |
Vancouver | Kıran M,Eşme E Topluluk Ve Derin Öğrenme Teknikleri Ile X-Ray Görüntülerinden Patlayıcı Devre Tespiti. . 2023; 0 - 98. 122E024 |
IEEE | Kıran M,Eşme E "Topluluk Ve Derin Öğrenme Teknikleri Ile X-Ray Görüntülerinden Patlayıcı Devre Tespiti." , ss.0 - 98, 2023. 122E024 |
ISNAD | Kıran, Mustafa Servet - Eşme, Engin. "Topluluk Ve Derin Öğrenme Teknikleri Ile X-Ray Görüntülerinden Patlayıcı Devre Tespiti". (2023), 0-98. https://doi.org/122E024 |
APA | Kıran M, Eşme E (2023). Topluluk Ve Derin Öğrenme Teknikleri Ile X-Ray Görüntülerinden Patlayıcı Devre Tespiti. , 0 - 98. 122E024 |
Chicago | Kıran Mustafa Servet,Eşme Engin Topluluk Ve Derin Öğrenme Teknikleri Ile X-Ray Görüntülerinden Patlayıcı Devre Tespiti. (2023): 0 - 98. 122E024 |
MLA | Kıran Mustafa Servet,Eşme Engin Topluluk Ve Derin Öğrenme Teknikleri Ile X-Ray Görüntülerinden Patlayıcı Devre Tespiti. , 2023, ss.0 - 98. 122E024 |
AMA | Kıran M,Eşme E Topluluk Ve Derin Öğrenme Teknikleri Ile X-Ray Görüntülerinden Patlayıcı Devre Tespiti. . 2023; 0 - 98. 122E024 |
Vancouver | Kıran M,Eşme E Topluluk Ve Derin Öğrenme Teknikleri Ile X-Ray Görüntülerinden Patlayıcı Devre Tespiti. . 2023; 0 - 98. 122E024 |
IEEE | Kıran M,Eşme E "Topluluk Ve Derin Öğrenme Teknikleri Ile X-Ray Görüntülerinden Patlayıcı Devre Tespiti." , ss.0 - 98, 2023. 122E024 |
ISNAD | Kıran, Mustafa Servet - Eşme, Engin. "Topluluk Ve Derin Öğrenme Teknikleri Ile X-Ray Görüntülerinden Patlayıcı Devre Tespiti". (2023), 0-98. https://doi.org/122E024 |