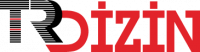
İnsansız Hava Aracı (İHA) ve Uzdenetim İle Elektromanyetik Radyasyon Ölçümü
Proje Grubu: EEEAG Sayfa Sayısı: 59 Proje No: 121E587 Proje Bitiş Tarihi: 15.10.2023 Metin Dili: Türkçe DOI: 121E587 İndeks Tarihi: 30-01-2024
İnsansız Hava Aracı (İHA) ve Uzdenetim İle Elektromanyetik Radyasyon Ölçümü
Öz: Bu projede insansız hava araçlarına (İHA) entegre edilmiş geniş bant ölçüm cihazları ile elektromanyetik haberleşme cihazlarına (alıcı/verici istasyonları) ait elektromanyetik alan şiddeti ölçümü (EMR) yaparak, elektromanyetik alan etkisinin limit değerlere uygunluğunun kontrolünü sağlayacak bir sistemin geliştirilmiştir. Bu bağlamda insansız hava aracına entegre edilen yeni nesil görüntüleme cihazları (kamera ve 3D lidar) ile hücresel elektromanyetik haberleşme cihazları kullanılarak baz istasyonlarındaki antenler tespit edilmiş ve belli lokasyonlarda EMR ölçümü yapılmıştır. Bu sebeple proje nesne konum bulma (object detection/localization), lidar (Laser Imaging Detection and Ranging) teknolojisi ve nokta bulutlarından nesne segmentasyonu, sensör füzyonu (sensor fusion), EMR ölçümü, telemetri sistemleri konularını içermektedir. Elektromanyetik haberleşme cihazlarının insan ve çevre sağlığına etkileri konusunda Dünya Sağlık Örgütü'nün (WHO) çalışmaları başta olmak üzere Uluslararası İyonlaştırmayan Radyasyondan Koruma Komisyonu'nun (ICNIRP) çalışmaları dikkate alınarak uyulması gereken limit değerler Bilgi Teknolojileri ve İletişim Kurumu tarafından belirlenmektedir. Yönetmelik kapsamında ölçümler, elektronik haberleşme cihazının anten yayın paterni dikkate alınarak antenin baktığı yönde, güvenlik mesafesinin dışında erişilebilen/ulaşılabilen en yakın yerde üç noktadan yapılmaktadır. Bu noktalar uzak alan olması nedeniyle ölçümler elektrik alan probu kullanılarak gerçekleştirilmektedir. Bu doğrultuda özel mülk ziyareti gereklilikleri, çatı gibi iş güvenliği açısından riskli yerlere çıkışlar, halk tepkisi nedeniyle ölçümler için uygun şartların sağlanamaması durumları oluşmaktadır. Projedeki amacımız yukarıda bahsedilen ölçüm kabiliyetlerini insansız hava araçlarına (İHA) kazandırmayı amaçlamıştır. Bu bağlamda İHA üzerine konumlandırılan görsel kamera ve 3B lidar kullanılarak GSM baz istasyonlarında bulunan antenlere ait elektromanyetik alan şiddeti ve yayın yapılan frekansın aralığını ölçen bir sistem geliştirilmiştir. Antenin konumunun bulunması için anten görüntülerini içeren bir veri tabanı oluşturulmuş ve You Only Look Once (YOLO) algoritmaları eğitilerek sahada baz istasyonlarındaki antenlerin gerçek zamanlı tespiti yapılmıştır. Sonrasında, kamera ve lidar kullanılarak İHA ve anten arası mesafe hesaplanmış ve antenden belli bir mesafede ölçümler yapılmıştır. Bu mesafenin doğru bir şekilde hesaplanabilmesi için kamera ve 3B lidarın kalibrasyonu için çalışmalar yürütülmüştür.
Anahtar Kelime: Electromagnetic Radiation Measurement with Unmanned Aerial Vehicle (UAV) and Autonomic
Öz: In this project, a system has been developed to measure the electromagnetic field strength (EFS) of integrated broadband measurement devices and electromagnetic communication devices (receiver/transmitter stations) on unmanned aerial vehicles (UAVs), ensuring the compliance of the electromagnetic field effect with limit values. In this context, next-generation imaging devices (camera and 3D lidar) and cellular electromagnetic communication devices integrated into unmanned aerial vehicles were used to detect antennas in base stations and perform EMR measurements at specific locations. Therefore, the project encompasses object detection/localization, lidar (Laser Imaging Detection and Ranging) technology, object segmentation from point clouds, sensor fusion, EFS measurement, and telemetry systems. The limit values that must be adhered to regarding the effects of electromagnetic communication devices on human health and the environment are determined by the Information Technologies and Communication Authority, taking into account the studies of the World Health Organization (WHO) and the International Commission on Non-Ionizing Radiation Protection (ICNIRP). Within the regulations, measurements are taken from three points at the nearest location accessible/outside the safety distance, considering the antenna radiation pattern of the electronic communication device and the direction in which the antenna is facing. Due to the remote nature of these points, measurements are carried out using an electric field probe. In this context, there are requirements for special property visits, access to risk-prone areas such as roofs for occupational safety reasons, and situations where suitable conditions for measurements cannot be provided due to public reactions. The aim of the project was to equip unmanned aerial vehicles (UAVs) with the measurement capabilities mentioned above. In this context, a system was developed using visual cameras and 3D lidars positioned on the UAV to measure the electromagnetic field strength and the frequency range of antennas in GSM base stations. A database containing antenna images was created for antenna location determination, and You Only Look Once (YOLO) algorithms were trained to achieve real-time detection of antennas in base stations in the field. Subsequently, using the camera and lidar, the distance between the UAV and the antenna was calculated, and measurements were taken at a certain distance from the antenna. Calibration studies were conducted for the camera and 3D lidar to accurately calculate this distance.
Anahtar Kelime: Erişim Türü: Erişime Açık
0
0
0
- [1] Aramco Services Company, Saudi Arabian Oil Company (2020) “Drone-based electromagnetics for early detection of shallow drilling hazards”, US Patent US10845498B2.
- [2] Bradski, G. and Kaehler, A. (2008). Learning OpenCV: Computer Vision with the OpenCV Library. O'Reilly Media, Inc.
- [3] Dellenbach, P., Deschaud, J.-E., Jacquet, B. , Goulette, F. (2021) “CT-ICP: Real-time Elastic LiDAR Odometry with Loop Closure,” arXiv:2109.12979v2.
- [4] Elaksher, A. F., Bhandari, S., Carreon-Limones, C., Lauf, R. (2017) “Potential of UAV lidar systems for geospatial mapping”, Proc. SPIE 10406, Lidar Remote Sensing for Environmental Monitoring.
- [5] Felzenszwalb,P., Mcallester, D., Ramanan, D. (2008). “A discriminatively trained, multiscale, deformable part model”, IEEE Conference on Computer Vision and Pattern Recognition.
- [6] Hartley, R. and Zisserman, A. (2004). Multiple View Geometry in Computer Vision. Cambridge University Press.
- [7] Hu, T., Sun, X., Su, Y., Guan, H., Sun, Q., Kelly, M., Guo, Q. (2021) “Development and performance evaluation of a very low-cost UAV-lidar system for forestry applications”, Remote Sensing, 13, 1-21.
- [8] Hu, Q., Yang, B., Xie, L., Rosa, S., Guo, Y., Wang, Z., Trigoni, N., Markham, A. (2020) “Randla-net: Efficient semantic segmentation of large-scale point clouds”, Proceedings of the IEEE Conference on Computer Vision and Pattern Recognition.
- [9] Kölle, M., Laupheimer, D., Schmohl, S., Haala, N., Rottensteiner, F., Wegner, J. D., Ledoux, H. (2021) “The Hessigheim 3D (H3D) Benchmark on Semantic Segmentation of High- Resolution 3D Point Clouds and Textured Meshes from UAV LiDAR and Multi-View-Stereo”, arXiv, 2021, eprint={2102.05346}
- [10] Lang, Alex H., Vora, S., Caesar, H., Zhou, L., Yang, J., Beijbom, O. (2019) "PointPillars: Fast Encoders for Object Detection From Point Clouds." In 2019 IEEE/CVF Conference on Computer Vision and Pattern Recognition (CVPR), 12689-12697. Long Beach, CA, USA.
- [11] Lee,G.-M., Lee, J.-H., Park, S.-Y. (2017). “Calibration of VLP-16 Lidar and multi-view cameras using a ball for 360 degree 3D color map acquisition", 2017 IEEE International Conference on Multisensor Fusion and Integration for Intelligent Systems (MFI).
- [12] Lin, T.-Y., Goyal, P., Girshick, R., He, K., Dollar, P. (2017) “Focal loss for dense object detection”, IEEE International Conference on Computer Vision (ICCV).
- [13] Lin, Y.-C., Cheng, Y.-T., Zhou, T., Ravi, R., Hasheminasab, Flatt, J. E., Troy, C., Habib, A. (2019) “Evaluation of UAV lidar for mapping coastal environments”, Remote Sensing, 11, 1-32.
- [14] Liu, W., Anguelov, D., Erhan, D., Szegedy, C., Reed, S., Fu, C.-Y., Berg, A. C. (2016) “SSD: Single shot MultiBox detector”, European Conference Computer Vision (ECCV). 49
- [15] Milioto, A., Vizzo, I., Behley, J., Stachniss, C. (2019) "RangeNet ++: Fast and Accurate LiDAR Semantic Segmentation," 2019 IEEE/RSJ International Conference on Intelligent Robots and Systems (IROS).
- [16] Morsdorf, F., Eck, C., Zgraggen, C., Imbach, B., Schneider, F. D., Kükenbrink, D. (2017) “UAV-based lidar acquisition for the derivation of high-resolution forest and ground information”, The Leading Edge, 36, 538-620.
- [17] Paigwar, A., Erkent, O., Wolf, C., Laugier, C. (2019) “Attentional PointNet for 3D-Object Detection in Point Clouds”, Proceedings of the IEEE/CVF Conference on Computer Vision and Pattern Recognition (CVPR) Workshops.
- [18] Pang, C., Tan, Y., Li,S., Li,Y., Ji, B.,Song, R. (2019). “Low-cost and high-accuracy LiDAR SLAM for large outdoor scenarios", 2019 IEEE International Conference on Real-time Computing and Robotics (RCAR).
- [19] Qi, C. R., Su, H., Mo, K., Guibas, L. J. (2017a) “PointNet: Deep learning on point sets for 3D classification and segmentation”, IEEE Society Conference on Computer Vision and Pattern Recognition (CVPR).
- [20] Qi, C. R., Su, H., Mo, K., Guibas, L. J. (2017b) “PointNet++: Deep hierarchical feature learning on point sets in a metric space,” Advances in Neural Information Processing Systems.
- [21] Quigley, M., Conley, K., Gerkey, B., Faust, J., Foote, T., Leibs, J., ... & Wheeler, R. (2009). ROS: an open-source Robot Operating System. In ICRA workshop on open source software (Vol. 3, No. 3.2).
- [22] Redmon, J., Divvala, S., Girshick, R., Farhadi, A. (2016). “You only look once: Unified, real-time object detection”, IEEE Conference on Computer Vision and Pattern Recognition (CVPR).
- [23] Redmon, J. (2016). “Darknet: Open source neural networks in C”, Website http://pjreddie.com/darknet.
- [24] Redmon, J., Farhadi, A. (2017). “YOLO9000: Better, faster, stronger”, IEEE Conference on Computer Vision and Pattern Recognition (CVPR).
- [25] Redmon, J., Farhadi, A. (2018). “Yolov3: An incremental improvement”, Report, RAND Corporation.
- [26] Ren, S., He, K., Girshick, R., Sun, J. (2017) “Faster r-CNN: Towards real-time object detection with region proposal networks”, IEEE Transactions on Pattern Analysis and Machine Intelligence, 39, 11-37-1149.
- [27] Rusu, R. B., Blodow, N., & Beetz, M. (2009). Fast point feature histograms (FPFH) for 3D registration. In Proceedings of the IEEE International Conference on Robotics and Automation (ICRA), Kobe, Japan, pp. 3212-3217.
- [28] Shaw, L., Helmholz, P., Belton, D., Addy, N. (2019) “Comparison of UAV lidar and imagery for beach monitoring”, The International Archives of the Photogrammetry, Remote Sensing and Spatial Information Sciences.
- [29] Sobczak, L., Filus, K., Domanski, A., Domanska, J. (2021) “LiDAR point cloud generation for SLAM algorithm evaluation", Sensors, 21,10.
- [30] Teng, G. E., Zhou, M., Li, C. R., Wu, H. H. , Li, W., Meng, F. R., Zhou, C. C., Ma, L. (2017) “Mini-UAV lidar for power line inspection”, The International Archives of the Photogrammetry, Remote Sensing and Spatial Information Science.
- [31] Tsai, R. Y. (1987). A versatile camera calibration technique for high-accuracy 3D machine vision metrology using off-the-shelf TV cameras and lenses. IEEE Journal on Robotics and Automation, 3(4), 323-344.
- [32] Univ. Michigan Regents (2020) “Rapid Characterization of the Sources of Electromagnetic Signals and Environmental Substances”, US Patent US2019057927W.
- [33] Wallace, L., Lucieer, A., Watson, C., Turner, D. (2012) “Development of a UAV-Lidar system with application to forest inventory”, Remote Sensing, 4, 1519-1543.
- [34] Wallace, L., Lucieer, A., Watson, C. S. (2014) “Evaluating tree detection and segmentation routines on very high resolution UAV lidar data”, IEEE Transactions on Geoscience and Remote Sensing, 52, 7619-7628.
- [35] Wang, C.-Y., Bochkovskiy, A., Liao, H.-y. M. (2023) “YOLOv7: Trainable Bag-of-Freebies Sets New State-of-the-Art for Real-Time Object Detectors,” IEEE/CVF Conference on Computer Vision and Pattern Recognition (CVPR).
- [36] Xie, Q., Lai, Y.-K., Wu, J., Wang, Z., Zhang, Y., Xu, K., Wang, J. (2020) "MLCVNet: Multi- Level Context VoteNet for 3D Object Detection," in 2020 IEEE/CVF Conference on Computer Vision and Pattern Recognition (CVPR), Seattle, WA, USA, pp. 10444-10453.
- [37] Yan, X., Zheng, C., Li, Z., Wang, S., Cui, S. (2020) "PointASNL: Robust point clouds processing using nonlocal neural networks with adaptive sampling", IEEE/CVF Conference on Computer Vision and Pattern Recognition (CVPR).
- [38] Yang, Z., Sun, L., Liu, J., & Wang, Z. (2021). Deep multi-modal fusion for object detection in LiDAR-camera systems. Remote Sensing, 13(14), 2773.
- [39] Ye, Y., Chen, H., Zhang, C., Hao, X., Zhang, Z. (2020) “SARPNET: Shape attention regional proposal network for liDAR-based 3D object detection”, Neurocomputing, Volume 379, Pages 53-63, ISSN 0925-2312,
- [40] Zhang, Z., Li, Y., & Tan, T. (2015). Self-calibration of LiDAR and camera system based on 3D object reconstruction. Journal of Sensors, 2015.
- [41] Zhang, J., Lin, H., Hu, Y., Wang, H., & Yang, H. (2021). A LiDAR intrinsic calibration method using planar targets. Sensors, 21(13), 4423.
- [42] Zhang, Z. (2000). A flexible new technique for camera calibration. IEEE Transactions on Pattern Analysis and Machine Intelligence, 22(11), 1330-1334.
- [43] Zhou, L., Gu, X., Cheng, S., Yang, G., Shu, M., Sun, Q. (2020) “Analysis of plant height changes of lodged maize using UAV-Lidar data”, Agriculture, 10, 1-14.
APA | GİRGİN T, ÇEVİKALP H, Saribaş H, DUTAĞACI H, YİĞİT K, DENİZ M, ISILAK C, BAYAR A (2023). İnsansız Hava Aracı (İHA) ve Uzdenetim İle Elektromanyetik Radyasyon Ölçümü. , 0 - 59. 121E587 |
Chicago | GİRGİN TUFAN,ÇEVİKALP Hakan,Saribaş Hasan,DUTAĞACI Helin,YİĞİT KÜBRA,DENİZ Meryem,ISILAK CEMAL,BAYAR ALPEREN ENES İnsansız Hava Aracı (İHA) ve Uzdenetim İle Elektromanyetik Radyasyon Ölçümü. (2023): 0 - 59. 121E587 |
MLA | GİRGİN TUFAN,ÇEVİKALP Hakan,Saribaş Hasan,DUTAĞACI Helin,YİĞİT KÜBRA,DENİZ Meryem,ISILAK CEMAL,BAYAR ALPEREN ENES İnsansız Hava Aracı (İHA) ve Uzdenetim İle Elektromanyetik Radyasyon Ölçümü. , 2023, ss.0 - 59. 121E587 |
AMA | GİRGİN T,ÇEVİKALP H,Saribaş H,DUTAĞACI H,YİĞİT K,DENİZ M,ISILAK C,BAYAR A İnsansız Hava Aracı (İHA) ve Uzdenetim İle Elektromanyetik Radyasyon Ölçümü. . 2023; 0 - 59. 121E587 |
Vancouver | GİRGİN T,ÇEVİKALP H,Saribaş H,DUTAĞACI H,YİĞİT K,DENİZ M,ISILAK C,BAYAR A İnsansız Hava Aracı (İHA) ve Uzdenetim İle Elektromanyetik Radyasyon Ölçümü. . 2023; 0 - 59. 121E587 |
IEEE | GİRGİN T,ÇEVİKALP H,Saribaş H,DUTAĞACI H,YİĞİT K,DENİZ M,ISILAK C,BAYAR A "İnsansız Hava Aracı (İHA) ve Uzdenetim İle Elektromanyetik Radyasyon Ölçümü." , ss.0 - 59, 2023. 121E587 |
ISNAD | GİRGİN, TUFAN vd. "İnsansız Hava Aracı (İHA) ve Uzdenetim İle Elektromanyetik Radyasyon Ölçümü". (2023), 0-59. https://doi.org/121E587 |
APA | GİRGİN T, ÇEVİKALP H, Saribaş H, DUTAĞACI H, YİĞİT K, DENİZ M, ISILAK C, BAYAR A (2023). İnsansız Hava Aracı (İHA) ve Uzdenetim İle Elektromanyetik Radyasyon Ölçümü. , 0 - 59. 121E587 |
Chicago | GİRGİN TUFAN,ÇEVİKALP Hakan,Saribaş Hasan,DUTAĞACI Helin,YİĞİT KÜBRA,DENİZ Meryem,ISILAK CEMAL,BAYAR ALPEREN ENES İnsansız Hava Aracı (İHA) ve Uzdenetim İle Elektromanyetik Radyasyon Ölçümü. (2023): 0 - 59. 121E587 |
MLA | GİRGİN TUFAN,ÇEVİKALP Hakan,Saribaş Hasan,DUTAĞACI Helin,YİĞİT KÜBRA,DENİZ Meryem,ISILAK CEMAL,BAYAR ALPEREN ENES İnsansız Hava Aracı (İHA) ve Uzdenetim İle Elektromanyetik Radyasyon Ölçümü. , 2023, ss.0 - 59. 121E587 |
AMA | GİRGİN T,ÇEVİKALP H,Saribaş H,DUTAĞACI H,YİĞİT K,DENİZ M,ISILAK C,BAYAR A İnsansız Hava Aracı (İHA) ve Uzdenetim İle Elektromanyetik Radyasyon Ölçümü. . 2023; 0 - 59. 121E587 |
Vancouver | GİRGİN T,ÇEVİKALP H,Saribaş H,DUTAĞACI H,YİĞİT K,DENİZ M,ISILAK C,BAYAR A İnsansız Hava Aracı (İHA) ve Uzdenetim İle Elektromanyetik Radyasyon Ölçümü. . 2023; 0 - 59. 121E587 |
IEEE | GİRGİN T,ÇEVİKALP H,Saribaş H,DUTAĞACI H,YİĞİT K,DENİZ M,ISILAK C,BAYAR A "İnsansız Hava Aracı (İHA) ve Uzdenetim İle Elektromanyetik Radyasyon Ölçümü." , ss.0 - 59, 2023. 121E587 |
ISNAD | GİRGİN, TUFAN vd. "İnsansız Hava Aracı (İHA) ve Uzdenetim İle Elektromanyetik Radyasyon Ölçümü". (2023), 0-59. https://doi.org/121E587 |