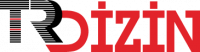
Differentially private online Bayesian estimation with adaptive truncation
Yıl: 2024 Cilt: 32 Sayı: 1 Sayfa Aralığı: 34 - 50 Metin Dili: İngilizce DOI: 10.55730/1300-0632.4054 İndeks Tarihi: 14-03-2024
Differentially private online Bayesian estimation with adaptive truncation
Öz: In this paper, a novel online and adaptive truncation method is proposed for differentially private Bayesian online estimation of a static parameter regarding a population. A local differential privacy setting is assumed where sensitive information from individuals is collected on an individual level and sequentially. The inferential aim is to estimate, on the fly, a static parameter regarding the population to which those individuals belong. We propose sequential Monte Carlo to perform online Bayesian estimation. When individuals provide sensitive information in response to a query, it is necessary to corrupt it with privacy-preserving noise to ensure the privacy of those individuals. The amount of corruption is proportional to the sensitivity of the query, which is determined usually by the range of the queried information. The proposed truncation technique adapts to the previously collected data to adjust the query range for the next individual. The idea is that, based on previous data, one can carefully arrange the interval into which the next individual’s information is to be truncated before being distorted with privacy-preserving noise. In this way, predictive queries are designed with small sensitivity, hence small privacy-preserving noise, enabling more accurate estimation while maintaining the same level of privacy. To decide on the location and the width of the interval, an exploration-exploitation approach is employed, a la Thompson sampling, with an objective function based on Fisher information. The merits of the methodology are shown with numerical examples.
Anahtar Kelime: Belge Türü: Makale Makale Türü: Araştırma Makalesi Erişim Türü: Erişime Açık
- [1] Dwork C. Differential privacy. In: Bugliesi M, Preneel B, Sassone V, Wegener I, editors, Automata, Languages and Programming; Berlin, Heidelberg. Springer Berlin Heidelberg; 2006. pp. 1-12.
- [2] Dwork C, Roth A. The algorithmic foundations of differential privacy. Theoretical Computer Science 2013; 9 (3- 4):211-407.
- [3] Kasiviswanathan SP, Lee HK, Nissim K, Raskhodnikova S, Smith A. What can we learn privately? SIAM Journal on Computing 2011; 40 (3):793-826
- [4] Chopin N. A sequential particle filter method for static models. Biometrika 2002; 89 (3):539-551
- [5] Barnes LP, Chen WN, Özgür A. Fisher information under local differential privacy. IEEE Journal on Selected Areas in Information Theory 2020; 1 (3):645-659.
- [6] Alparslan B, Yıldırım S. Statistic selection and MCMC for differentially private Bayesian estimation. Statistics and Computing 2022; 32 (5):66.
- [7] Russo DJ, Roy BV, Kazerouni A, Osband I, Wen Z. A tutorial on Thompson sampling. Foundations and Trends in Machine Learning 2018; 11 (1):1-96.
- [8] Dwork C. Differential privacy: A survey of results. In: Agrawal M, Du D, Duan Z, Li A, editors, Theory and Applications of Models of Computation; Berlin, Heidelberg. Springer Berlin Heidelberg; 2008. pp. 1-19.
- [9] Dong J, Roth A, Su WJ. Gaussian differential privacy. Journal of the Royal Statistical Society: Series B (Statistical Methodology) 2022; 84 (1):3-37.
- [10] Bun M, Steinke T. Concentrated differential privacy: Simplifications, extensions, and lower bounds. In: Proceedings, Part I, of the 14th International Conference on Theory of Cryptography-Volume 9985; New York, NY, USA. Springer- Verlag New York, Inc.; 2016: 635-658.
- [11] Wang YX, Fienberg S, Smola A. Privacy for free: Posterior sampling and stochastic gradient Monte Carlo. In: Blei D, Bach F, editors, Proceedings of the 32nd International Conference on Machine Learning (ICML-15). JMLR Workshop and Conference Proceedings; 2015:2493-2502.
- [12] Li B, Chen C, Liu H, Carin L. On connecting stochastic gradient MCMC and differential privacy. In: Chaudhuri K, Sugiyama M, editors, Proceedings of the Twenty-Second International Conference on Artificial Intelligence and Statistics; volume 89 of Proceedings of Machine Learning Research. PMLR; 2019: 557-566.
- [13] Heikkilä M, Jälkö J, Dikmen O, Honkela A. Differentially private Markov chain Monte Carlo. In: Wallach H, Larochelle H, Beygelzimer A, d'Alché-Buc F, Fox E, Garnett R, editors, Advances in Neural Information Processing Systems; volume 32. Curran Associates, Inc.; 2019.
- [14] Yıldırım S, Ermiş B. Exact MCMC with differentially private moves. Statistics and Computing 2019; 29 (5):947-963.
- [15] Räisä O, Koskela A, Honkela A. Differentially Private Hamiltonian Monte Carlo. In: NeurIPS 2021 Workshop Privacy in Machine Learning; 2021.
- [16] Foulds J, Geumlek J, Welling M, Chaudhuri K. On the theory and practice of privacy-preserving Bayesian data analysis. In: Proceedings of the Thirty-Second Conference on Uncertainty in Artificial Intelligence; UAI’16; Arlington, Virginia, USA. AUAI Press; 2016:192-201.
- [17] Williams O, Mcsherry F. Probabilistic inference and differential privacy. In: Lafferty J, Williams C, Shawe-Taylor J, Zemel R, Culotta A, editors, Advances in Neural Information Processing Systems; volume 23. Curran Associates, Inc.; 2010.
- [18] Karwa V, Slavković AB, Krivitsky P. Differentially private exponential random graphs. In: Domingo-Ferrer J, editor, Privacy in Statistical Databases; Cham. Springer International Publishing; 2014. pp. 143-155.
- [19] Bernstein G, Sheldon DR. Differentially private Bayesian inference for exponential families. In: Bengio S, Wallach H, Larochelle H, Grauman K, Cesa-Bianchi N, Garnett R, editors, Advances in Neural Information Processing Systems; volume 31. Curran Associates, Inc.; 2018.
- [20] Park M, Vinaroz M, Jitkrittum W. ABCDP: Approximate Bayesian computation with differential privacy. Entropy 2021; 23 (8).
- [21] Gong R. Exact inference with approximate computation for differentially private data via perturbations. Journal of Privacy and Confidentiality 2022; 12 (2).
- [22] Dwork C, Naor M, Pitassi T, Rothblum GN. Differential privacy under continual observation. In: Proceedings of the Forty-Second ACM Symposium on Theory of Computing; STOC ’10; New York, NY, USA. Association for Computing Machinery; 2010: 715-724.
- [23] Chan T, Shi E, Song D. Private and continual release of statistics. ACM Transactions on Information and System Security 2011; 14 (3).
- [24] Cao Y, Yoshikawa M, Xiao Y, Xiong L. Quantifying differential privacy under temporal correlations. In: 2017 IEEE 33rd International Conference on Data Engineering (ICDE); 2017. pp. 821-832.
- [25] Wang T, Blocki J, Li N, Jha S. Locally differentially private protocols for frequency estimation. In: Proceedings of the 26th USENIX Conference on Security Symposium; SEC’17; USA. USENIX Association; 2017: 729-745.
- [26] Steinberger L. Efficiency in local differential privacy. arXiv preprint arXiv:2301.10600 2023.
- [27] Lopuhaä-Zwakenberg M, Boris S, Li N. Fisher information as a utility metric for frequency estimation under local differential privacy. In: Proceedings of the 21st Workshop on Privacy in the Electronic Society; WPES’22; New York, NY, USA. Association for Computing Machinery; 2022: 41-53.
- [28] Gilks WR, Berzuini C. Following a moving target-Monte Carlo inference for dynamic Bayesian models. Journal of the Royal Statistical Society: Series B (Statistical Methodology) 2001; 63 (1):127-146.
- [29] Andrieu C, Yıldırım S, Doucet A, Chopin N. Metropolis-Hastings with averaged acceptance ratios. arXiv:2101.01253 2020.
- [30] Jones MC, Noufaily A. Log-location-scale-log-concave distributions for survival and reliability analysis. Electronic Journal of Statistics 2015; 9 (2):2732-2750.
- [31] Geweke J. Bayesian inference in econometric models using Monte Carlo integration. Econometrica 1989; 57 (6):1317- 1339.
APA | Yildirim S (2024). Differentially private online Bayesian estimation with adaptive truncation. , 34 - 50. 10.55730/1300-0632.4054 |
Chicago | Yildirim Sinan Differentially private online Bayesian estimation with adaptive truncation. (2024): 34 - 50. 10.55730/1300-0632.4054 |
MLA | Yildirim Sinan Differentially private online Bayesian estimation with adaptive truncation. , 2024, ss.34 - 50. 10.55730/1300-0632.4054 |
AMA | Yildirim S Differentially private online Bayesian estimation with adaptive truncation. . 2024; 34 - 50. 10.55730/1300-0632.4054 |
Vancouver | Yildirim S Differentially private online Bayesian estimation with adaptive truncation. . 2024; 34 - 50. 10.55730/1300-0632.4054 |
IEEE | Yildirim S "Differentially private online Bayesian estimation with adaptive truncation." , ss.34 - 50, 2024. 10.55730/1300-0632.4054 |
ISNAD | Yildirim, Sinan. "Differentially private online Bayesian estimation with adaptive truncation". (2024), 34-50. https://doi.org/10.55730/1300-0632.4054 |
APA | Yildirim S (2024). Differentially private online Bayesian estimation with adaptive truncation. Turkish Journal of Electrical Engineering and Computer Sciences, 32(1), 34 - 50. 10.55730/1300-0632.4054 |
Chicago | Yildirim Sinan Differentially private online Bayesian estimation with adaptive truncation. Turkish Journal of Electrical Engineering and Computer Sciences 32, no.1 (2024): 34 - 50. 10.55730/1300-0632.4054 |
MLA | Yildirim Sinan Differentially private online Bayesian estimation with adaptive truncation. Turkish Journal of Electrical Engineering and Computer Sciences, vol.32, no.1, 2024, ss.34 - 50. 10.55730/1300-0632.4054 |
AMA | Yildirim S Differentially private online Bayesian estimation with adaptive truncation. Turkish Journal of Electrical Engineering and Computer Sciences. 2024; 32(1): 34 - 50. 10.55730/1300-0632.4054 |
Vancouver | Yildirim S Differentially private online Bayesian estimation with adaptive truncation. Turkish Journal of Electrical Engineering and Computer Sciences. 2024; 32(1): 34 - 50. 10.55730/1300-0632.4054 |
IEEE | Yildirim S "Differentially private online Bayesian estimation with adaptive truncation." Turkish Journal of Electrical Engineering and Computer Sciences, 32, ss.34 - 50, 2024. 10.55730/1300-0632.4054 |
ISNAD | Yildirim, Sinan. "Differentially private online Bayesian estimation with adaptive truncation". Turkish Journal of Electrical Engineering and Computer Sciences 32/1 (2024), 34-50. https://doi.org/10.55730/1300-0632.4054 |