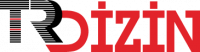
Artificial intelligence-based evaluation of the factors affecting the sales of an iron and steel company
Yıl: 2024 Cilt: 32 Sayı: 1 Sayfa Aralığı: 51 - 67 Metin Dili: İngilizce DOI: 10.55730/1300-0632.4055 İndeks Tarihi: 14-03-2024
Artificial intelligence-based evaluation of the factors affecting the sales of an iron and steel company
Öz: It is important to predict the sales of an iron and steel company and to identify the variables that influence these sales for future planning. The aim in this study was to identify and model the key factors that influence the sales volume of an iron and steel company using artificial neural networks (ANNs). We attempted to obtain an integrated result from the performance/sales levels of 5 models, to use the ANN approach with hybrid algorithms, and also to present an exemplary application in the base metals industry, where there is a limited number of studies. This study contributes to the literature as the first application of artificial intelligence methods in the iron and steel industry. The ANN models incorporated 6 macroeconomic variables and price-to-sales data and their results were evaluated. An ordinary least squares regression model was also used to facilitate the comparison of results, while gray relational analysis (GRA) was used to draw a comprehensive conclusion based on the ANN results. The results showed that the variables USD/TL exchange rate, product prices, and interest rates, in descending order, had the highest degree of influence in determining the sales of the iron and steel company. Furthermore, these variables are crucial for forecasting future sales and strategic planning. The study showed that the ANN outperformed classical regression models in terms of prediction accuracy. In the model applications conducted for 5 different product groups, it was observed that 3 models (models 2, 3, and 4), including model 4, which sold a higher volume of products than the total of the other products, had an overall performance above 80%. In addition, GRA was found to be a valuable tool for synthesizing insights from different ANN models based on their respective performance levels.
Anahtar Kelime: Belge Türü: Makale Makale Türü: Araştırma Makalesi Erişim Türü: Erişime Açık
- [1] Pekkaya M, Uysal Z. Pandemi döneminde demir çelik işletmelerinde satış değerlendirmesi ve satış modellemesi. Uluslararası Yönetim İktisat ve İşletme Dergisi 2021; 17: 69-83 (in Turkish).
- [2] Altan A, Karasu S, Bekiros S. Digital currency forecasting with chaotic meta-heuristic bio-inspired signal processing techniques. Chaos, Solitons & Fractals 2019; 126: 325-336.
- [3] Chang V. Towards data analysis for weather cloud computing. Knowledge-Based Systems 2017; 127: 29-45.
- [4] Chen A, Blue J. Performance analysis of demand planning approaches for aggregating, forecasting and disaggre- gating interrelated demands. International Journal of Production Economics 2010; 128 (2): 586-602.
- [5] Jeon S, Hong B, Chang V. Pattern graph tracking-based stock price prediction using big data. Future Generation Computer Systems 2018; 80: 171-187.
- [6] Karasu S, Altan A, Bekiros S, Ahmad W. A new forecasting model with wrapper-based feature selection approach using multi-objective optimization technique for chaotic crude oil time series. Energy 2020; 212: 118750.
- [7] Gashler MS, Ashmore SC. Modeling time series data with deep Fourier neural networks. Neurocomputing 2016; 188: 3-11.
- [8] Huh KS. Steel consumption and economic growth in Korea: long-term and short-term evidence. Resources Policy 2011; 36 (2): 107-113.
- [9] Evans M. Modelling steel demand in the UK. Ironmaking & Steelmaking 1996; 23 (1): 17-24.
- [10] Abbott AJ, Lawler KA, Armistead C. The UK demand for steel. Applied Economics 1999; 31 (11): 1299-1302.
- [11] Crompton P. Future trends in Japanese steel consumption. Resources Policy 2000; 26 (2): 103-114.
- [12] Barska M. Demand forecast with business climate index for a steel and iron industry representative. Metody Ilościowe w Badaniach Ekonomicznych 2014; 15 (2): 27-36.
- [13] Crompton P, Wu Y. Bayesian vector autoregression forecasts of Chinese steel consumption. Journal of Chinese Economic and Business Studies 2003; 1 (2): 205-219.
- [14] Evans M. An alternative approach to estimating the parameters of a generalised Grey Verhulst model: an application to steel intensity of use in the UK. Expert Systems with Applications 2014; 41 (4): 1236-1244.
- [15] Chang PC, Wang YW, Tsai CY. Evolving neural network for printed circuit board sales forecasting. Expert Systems with Applications 2005; 29 (1): 83-92.
- [16] Psiloglou BE, Giannakopoulos C, Majithia S, Petrakis M. Factors affecting electricity demand in Athens, Greece and London, UK: a comparative assessment. Energy 2009; 34 (11): 1855-1863.
- [17] Efendigil T, Önüt S, Kahraman C. A decision support system for demand forecasting with artificial neural networks and neuro-fuzzy models: a comparative analysis. Expert Systems with Applications 2009; 36 (3): 6697-6707.
- [18] Hicham A, Mohammed B, Anas S. Hybrid intelligent system for sale forecasting using Delphi and adaptive fuzzy back-propagation neural networks. Editorial Preface 2012.
- [19] Oğcu G, Demirel OF, Zaim S. Forecasting electricity consumption with neural networks and support vector regression. Procedia - Social and Behavioral Sciences 2012; 58: 1576-1585.
- [20] Azadeh A, Neshat N, Mardan E, Saberi M. Optimization of steel demand forecasting with complex and uncertain economic inputs by an integrated neural network–fuzzy mathematical programming approach. The International Journal of Advanced Manufacturing Technology 2013; 65: 833-841.
- [21] Aziz AA, Mustapha NHN, Ismail R. Factors affecting energy demand in developing countries: a dynamic panel analysis. International Journal of Energy Economics and Policy 2013; 3 (4): 1-6.
- [22] Akyurt İZ. Talep tahmininin yapay sinir ağlarıyla modellenmesi: yerli otomobil örneği. Ekonometri ve İstatistik Dergisi 2015; 23: 147-157 (in Turkish).
- [23] Koochakpour K, Tarokh MJ. Sales budget forecasting and revision by adaptive network fuzzy base inference system and optimization methods. Journal of Computer & Robotics 2016; 9 (1): 25-38.
- [24] Ecemiş O. Model ağaç yöntemiyle satış tahmini: paslanmaz çelik sektöründe bir uygulama. The Journal of Academic Social Science 2019; 84 (84): 336-350 (in Turkish).
- [25] Jain AK, Mao J, Mohiuddin KM. Artificial neural networks: a tutorial. Computer 1996; 29 (3): 31-44.
- [26] Agatonovic-Kustrin S, Beresford R. Basic concepts of artificial neural network (ANN) modeling and its application in pharmaceutical research. Journal of Pharmaceutical and Biomedical Analysis 2000; 22 (5): 717-727.
- [27] Pekkaya M, Pulat Ö, Koca H. Evaluation of healthcare service quality via Servqual scale: an application on a hospital. International Journal of Healthcare Management 2019; 12 (4): 340-347.
- [28] Pekkaya M, Pulat Ö, Zeydan İ. Service quality determiners in higher education: the student’s perspective. Inter- national Journal of Services, Economics and Management 2023; 14 (3): 270-300.
- [29] Dökmen G, Pekkaya M, Saymaz N. Sigara bağımlılığı ve devletin sigara tüketimi ile mücadele yöntemleri arasındaki ilişki. Maliye Dergisi 2019; 176: 599-623 (in Turkish).
- [30] Madhukumar M, Sebastian A, Liang X, Jamil M, Shabbir MNSK. Regression model-based short-term load fore- casting for university campus load. IEEE Access 2022; 10: 8891-8905.
- [31] Singh U, Rizwan M, Alaraj M, Alsaidan I. A machine learning-based gradient boosting regression approach for wind power production forecasting: a step towards smart grid environments. Energies 2021; 14 (16): 5196.
- [32] Pekkaya M. ARFIMA ve FIGARCH yöntemlerinin Markowitz ortalama varyans portföy optimizasyonunda kullanıl- ması: İMKB-30 endeks hisseleri üzerine bir uygulama. İstanbul Üniversitesi İşletme Fakültesi Dergisi 2013; 42 (1): 93-112 (in Turkish).
- [33] Hayes AF, Cai L. Using heteroskedasticity-consistent standard error estimators in OLS regression: an introduction and software implementation. Behavior Research Methods 2007; 39: 709-722.
- [34] Hamzaçebi C, Pekkaya M. Determining of stock investments with grey relational analysis. Expert Systems with Applications 2011; 38 (8): 9186-9195.
APA | Pekkaya M, UYSAL Z, ALTAN A, Karasu S (2024). Artificial intelligence-based evaluation of the factors affecting the sales of an iron and steel company. , 51 - 67. 10.55730/1300-0632.4055 |
Chicago | Pekkaya Mehmet,UYSAL ZAFER,ALTAN AYTAÇ,Karasu Seçkin Artificial intelligence-based evaluation of the factors affecting the sales of an iron and steel company. (2024): 51 - 67. 10.55730/1300-0632.4055 |
MLA | Pekkaya Mehmet,UYSAL ZAFER,ALTAN AYTAÇ,Karasu Seçkin Artificial intelligence-based evaluation of the factors affecting the sales of an iron and steel company. , 2024, ss.51 - 67. 10.55730/1300-0632.4055 |
AMA | Pekkaya M,UYSAL Z,ALTAN A,Karasu S Artificial intelligence-based evaluation of the factors affecting the sales of an iron and steel company. . 2024; 51 - 67. 10.55730/1300-0632.4055 |
Vancouver | Pekkaya M,UYSAL Z,ALTAN A,Karasu S Artificial intelligence-based evaluation of the factors affecting the sales of an iron and steel company. . 2024; 51 - 67. 10.55730/1300-0632.4055 |
IEEE | Pekkaya M,UYSAL Z,ALTAN A,Karasu S "Artificial intelligence-based evaluation of the factors affecting the sales of an iron and steel company." , ss.51 - 67, 2024. 10.55730/1300-0632.4055 |
ISNAD | Pekkaya, Mehmet vd. "Artificial intelligence-based evaluation of the factors affecting the sales of an iron and steel company". (2024), 51-67. https://doi.org/10.55730/1300-0632.4055 |
APA | Pekkaya M, UYSAL Z, ALTAN A, Karasu S (2024). Artificial intelligence-based evaluation of the factors affecting the sales of an iron and steel company. Turkish Journal of Electrical Engineering and Computer Sciences, 32(1), 51 - 67. 10.55730/1300-0632.4055 |
Chicago | Pekkaya Mehmet,UYSAL ZAFER,ALTAN AYTAÇ,Karasu Seçkin Artificial intelligence-based evaluation of the factors affecting the sales of an iron and steel company. Turkish Journal of Electrical Engineering and Computer Sciences 32, no.1 (2024): 51 - 67. 10.55730/1300-0632.4055 |
MLA | Pekkaya Mehmet,UYSAL ZAFER,ALTAN AYTAÇ,Karasu Seçkin Artificial intelligence-based evaluation of the factors affecting the sales of an iron and steel company. Turkish Journal of Electrical Engineering and Computer Sciences, vol.32, no.1, 2024, ss.51 - 67. 10.55730/1300-0632.4055 |
AMA | Pekkaya M,UYSAL Z,ALTAN A,Karasu S Artificial intelligence-based evaluation of the factors affecting the sales of an iron and steel company. Turkish Journal of Electrical Engineering and Computer Sciences. 2024; 32(1): 51 - 67. 10.55730/1300-0632.4055 |
Vancouver | Pekkaya M,UYSAL Z,ALTAN A,Karasu S Artificial intelligence-based evaluation of the factors affecting the sales of an iron and steel company. Turkish Journal of Electrical Engineering and Computer Sciences. 2024; 32(1): 51 - 67. 10.55730/1300-0632.4055 |
IEEE | Pekkaya M,UYSAL Z,ALTAN A,Karasu S "Artificial intelligence-based evaluation of the factors affecting the sales of an iron and steel company." Turkish Journal of Electrical Engineering and Computer Sciences, 32, ss.51 - 67, 2024. 10.55730/1300-0632.4055 |
ISNAD | Pekkaya, Mehmet vd. "Artificial intelligence-based evaluation of the factors affecting the sales of an iron and steel company". Turkish Journal of Electrical Engineering and Computer Sciences 32/1 (2024), 51-67. https://doi.org/10.55730/1300-0632.4055 |