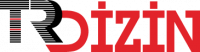
Longitudinal attacks against iterative data collection with local differential privacy
Yıl: 2024 Cilt: 32 Sayı: 1 Sayfa Aralığı: 198 - 218 Metin Dili: İngilizce İndeks Tarihi: 14-03-2024
Longitudinal attacks against iterative data collection with local differential privacy
Öz: Local differential privacy (LDP) has recently emerged as an accepted standard for privacy-preserving collection of users’ data from smartphones and IoT devices. In many practical scenarios, users’ data needs to be collected repeatedly across multiple iterations. In such cases, although each collection satisfies LDP individually by itself, a longitudinal collection of multiple responses from the same user degrades that user’s privacy. To demonstrate this claim, in this paper, we propose longitudinal attacks against iterative data collection with LDP. We formulate a general Bayesian adversary model, and then individually show the application of this adversary model on six popular LDP protocols: GRR, BLH, OLR, RAPPOR, OUE, and SS. We experimentally demonstrate the effectiveness of our attacks using two metrics, three datasets, and various privacy and domain parameters. The effectiveness of our attacks highlights the privacy risks associated with longitudinal data collection in a practical and quantifiable manner and motivates the need for appropriate countermeasures.
Anahtar Kelime: Belge Türü: Makale Makale Türü: Araştırma Makalesi Erişim Türü: Erişime Açık
- [1] Cormode G, Jha S, Kulkarni T, Li N, Srivastava D et al. Privacy at scale: local differential privacy in practice. In: Proceedings of the 2018 International Conference on Management of Data; Houston, TX, USA; 2018. pp. 1655-1658.
- [2] Wang T, Blocki J, Li N, Jha S. Locally differentially private protocols for frequency estimation. In: Proceedings of the 26th USENIX Security Symposium; Vancouver, BC, Canada; 2017. pp. 729-745.
- [3] Xiong X, Liu S, Li D, Cai Z, Niu X. A comprehensive survey on local differential privacy. Security and Communi- cation Networks 2020; 2020: 1-29. https://doi.org/10.1155/2020/8829523
- [4] Cunningham T, Cormode G, Ferhatosmanoglu H, Srivastava D. Real-world trajectory sharing with local differential privacy. Proceedings of the VLDB Endowment 2021; 14 (11): 2283-2295. https://doi.org/10.14778/3476249.3476280
- [5] Wang H, Hong H, Xiong L, Qin Z, Hong Y. L-srr: Local differential privacy for location-based services with staircase randomized response. In: Proceedings of the 2022 ACM SIGSAC Conference on Computer and Communications Security; New York, NY, USA; 2022. pp. 2809-2823.
- [6] Kim JW, Jang B. Workload-aware indoor positioning data collection via local differential privacy. IEEE Commu- nications Letters 2019; 23 (8): 1352-1356. https://doi.org/10.1109/LCOMM.2019.2922963
- [7] Navidan H, Moghtadaiee V, Nazaran N, Alishahi M. Hide me behind the noise: local differential privacy for indoor location privacy. In: 2022 IEEE European Symposium on Security and Privacy Workshops (EuroS&PW); Genoa, Italy; 2022. pp. 514-523.
- [8] Marchioro T, Kazlouski A, Markatos EP. Practical crowdsourcing of wearable IoT data with local differential privacy. In: Proceedings of the 8th ACM/IEEE Conference on Internet of Things Design and Implementation; San Antonio, TX, USA; 2023. pp. 275-287.
- [9] Wu X, Khosravi MR, Qi L, Ji G, Dou W et al. Locally private frequency estimation of physical symptoms for infectious disease analysis in internet of medical things. Computer Communications 2020; 162: 139-151. https://doi.org/10.1016/j.comcom.2020.08.015
- [10] Kim JW, Lim JH, Moon SM, Jang B. Collecting health lifelog data from smartwatch users in a privacy-preserving manner. IEEE Transactions on Consumer Electronics 2019; 65 (3): 369-378. https://doi.org/10.1109/TCE.2019.2924466
- [11] Zhao P, Zhang S, Wan S, Liu G, Umer T. A survey of local differential privacy for securing internet of vehicles. The Journal of Supercomputing 2020; 76 (11): 8391-8412. https://doi.org/10.1007/s11227-019-03104-0
- [12] Gursoy ME, Tamersoy A, Truex S, Wei W, Liu L. Secure and utility-aware data collection with condensed local differential privacy. IEEE Transactions on Dependable and Secure Computing 2021; 18 (5): 2365-2378. https://doi.org/10.1109/TDSC.2019.2949041
- [13] Ou L, Qin Z, Liao S, Li T, Zhang D. Singular spectrum analysis for local differential privacy of classifications in the smart grid. IEEE Internet of Things Journal 2020; 7 (6): 5246-5255. https://doi.org/10.1109/JIOT.2020.2977220
- [14] Gai N, Xue K, Zhu B, Yang J, Liu J et al. An efficient data aggregation scheme with local differential privacy in smart grid. Digital Communications and Networks 2022; 8 (3): 333-342. https://doi.org/10.1016/j.dcan.2022.01.004
- [15] Erlingsson U, Pihur V, Korolova A. Rappor: randomized aggregatable privacy-preserving ordinal response. In: Proceedings of the 2014 ACM SIGSAC Conference on Computer and Communications Security; Scottsdale, AZ, USA; 2014. pp. 1054-1067.
- [16] Differential Privacy Team, Apple. Learning with privacy at scale. 2020. https://docs-assets.developer.apple.com/ml- research/papers/learning-with-privacy-at-scale.pdf
- [17] Thakurta AG, Vyrros AH, Vaishampayan US, Kapoor G, Freudinger J et al. Emoji frequency detection and deep link frequency. 2017. US Patent App. 15/640,266.
- [18] Ding B, Kulkarni J, Yekhanin S. Collecting telemetry data privately. In: Advances in Neural Information Processing Systems; Long Beach, CA, USA; 2017. pp. 3571-3580.
- [19] Apple. Apple differential privacy technical overview. 2017. https://www.apple.com/privacy/docs/Differential- _Privacy_Overview.pdf.
- [20] Li T, Sahu AK, Talwalkar A, Smith V. Federated learning: challenges, methods, and future directions. IEEE Signal Processing Magazine 2020; 37 (3): 50-60. https://doi.org/10.1109/MSP.2020.2975749
- [21] Truex S, Liu L, Chow KH, Gursoy ME, Wei W. LDP-Fed: Federated learning with local differential privacy. In: Proceedings of the Third ACM International Workshop on Edge Systems, Analytics and Networking; Crete, Greece; 2020. pp. 61-66.
- [22] Arcolezi HH, Couchot JF, Al Bouna B, Xiao X. Improving the utility of locally differentially private protocols for longitudinal and multidimensional frequency estimates. Digital Communications and Networks 2022; in press. https://doi.org/10.1016/j.dcan.2022.07.003
- [23] Arcolezi HH, Pinzon C, Palamidessi C, Gambs S. Frequency estimation of evolving data under local differential privacy. In: 26th International Conference on Extending Database Technology; Ioannina, Greece; 2023. pp. 512-525.
- [24] Bassily R, Smith A. Local, private, efficient protocols for succinct histograms. In: Proceedings of the 47th Annual ACM Symposium on Theory of Computing; Portland, OR, USA; 2015. pp. 127-135.
- [25] Fanti G, Pihur V, Erlingsson U. Building a RAPPOR with the unknown: privacy-preserving learning of associations and data dictionaries. Proceedings on Privacy Enhancing Technologies 2016; 2016 (3): 41-61. https://doi.org/10.1515/popets-2016-0015
- [26] Gu X, Li M, Cheng Y, Xiong L, Cao Y. Pckv: locally differentially private correlated key-value data collection with optimized utility. In: Proceedings of the 29th USENIX Security Symposium; online; 2020. pp. 967–984.
- [27] Wang T, Li N, Jha S. Locally differentially private frequent itemset mining. In: IEEE Symposium on Security and Privacy (S&P); San Francisco, CA, USA; 2018. pp. 127-143.
- [28] Jia J, Gong NZ. Calibrate: frequency estimation and heavy hitter identification with local differential privacy via incorporating prior knowledge. In: IEEE International Conference on Computer Communications (INFOCOM); Paris, France; 2019. pp. 2008-2016.
- [29] Wang T, Li N, Jha S. Locally differentially private heavy hitter identification. IEEE Transactions on Dependable and Secure Computing 2019; 18 (2): 982-993. https://doi.org/10.1109/TDSC.2019.2927695
- [30] Wang T, Lopuhaa-Zwakenberg M, Li Z, Skoric B, Li N. Locally differentially private frequency estimation with consistency. In: Network and Distributed System Security Symposium (NDSS); San Diego, CA, USA; 2020.
- [31] Qin Z, Yang Y, Yu T, Khalil I, Xiao X et al. Heavy hitter estimation over set-valued data with local differential privacy. In: Proceedings of the 2016 ACM SIGSAC Conference on Computer and Communications Security; Vienna, Austria; 2016. pp. 192–203.
- [32] Qin Z, Yu T, Yang Y, Khalil I, Xiao X et al. Generating synthetic decentralized social graphs with local differential privacy. In: Proceedings of the 2017 ACM SIGSAC Conference on Computer and Communications Security; Dallas, TX, USA; 2017. pp. 425-438.
- [33] Wang S, Huang L, Wang P, Nie Y, Hu X et al. Mutual information optimally local private discrete distribution estimation. https://arxiv.org/pdf/1607.08025.pdf
- [34] Murakami T, Takahashi K. Toward evaluating re-identification risks in the local privacy model. Transactions on Data Privacy 2021; 14 (3): 79-116.
- [35] Gursoy ME, Liu L, Chow KH, Truex S, Wei W. An adversarial approach to protocol analysis and selection in local differential privacy. IEEE Transactions on Information Forensics and Security 2022; 17: 1785-1799. https://doi.org/10.1109/TIFS.2022.3170242
- [36] Gadotti A, Houssiau F, Annamalai MSMS, de Montjoye YA. Pool inference attacks on local differential privacy: quantifying the privacy guarantees of Apple’s count mean sketch in practice. In: Proceedings of the 31st USENIX Security Symposium; Boston, MA, USA; 2022. pp. 501-518.
- [37] Arcolezi HH, Gambs S, Couchot JF, Palamidessi C. On the risks of collecting multidimensional data under local differential privacy. Proceedings of the VLDB Endowment 2023; 16 (5): 1126-1139. https://doi.org/10.14778/3579075.3579086
- [38] Arcolezi HH, Couchot JF, Al Bouna B, Xiao X. Random sampling plus fake data: multidimensional frequency estimates with local differential privacy. In: Proceedings of the 30th ACM International Conference on Information and Knowledge Management (CIKM); Queensland, Australia; 2021. pp. 47-57.
- [39] Chatzikokolakis K, Cherubin G, Palamidessi C, Troncoso C. Bayes security: a not so average metric. In: IEEE 36th Computer Security Foundations Symposium (CSF); Dubrovnik, Croatia; 2023. pp. 388-406.
- [40] Kellaris G, Papadopoulos S, Xiao X, Papadias D. Differentially private event sequences over infinite streams. Proceedings of the VLDB Endowment 2014; 7 (12): 1155-1166. https://doi.org/10.14778/2732977.2732989
- [41] Schäler C, Hütter T, Schäler M. Benchmarking the utility of w-event differential privacy mechanisms – when baselines become mighty competitors. Proceedings of the VLDB Endowment 2023; 16 (8): 1830-1842. https://doi.org/10.14778/3594512.3594515
APA | Gursoy M (2024). Longitudinal attacks against iterative data collection with local differential privacy. , 198 - 218. |
Chicago | Gursoy M. Emre Longitudinal attacks against iterative data collection with local differential privacy. (2024): 198 - 218. |
MLA | Gursoy M. Emre Longitudinal attacks against iterative data collection with local differential privacy. , 2024, ss.198 - 218. |
AMA | Gursoy M Longitudinal attacks against iterative data collection with local differential privacy. . 2024; 198 - 218. |
Vancouver | Gursoy M Longitudinal attacks against iterative data collection with local differential privacy. . 2024; 198 - 218. |
IEEE | Gursoy M "Longitudinal attacks against iterative data collection with local differential privacy." , ss.198 - 218, 2024. |
ISNAD | Gursoy, M. Emre. "Longitudinal attacks against iterative data collection with local differential privacy". (2024), 198-218. |
APA | Gursoy M (2024). Longitudinal attacks against iterative data collection with local differential privacy. Turkish Journal of Electrical Engineering and Computer Sciences, 32(1), 198 - 218. |
Chicago | Gursoy M. Emre Longitudinal attacks against iterative data collection with local differential privacy. Turkish Journal of Electrical Engineering and Computer Sciences 32, no.1 (2024): 198 - 218. |
MLA | Gursoy M. Emre Longitudinal attacks against iterative data collection with local differential privacy. Turkish Journal of Electrical Engineering and Computer Sciences, vol.32, no.1, 2024, ss.198 - 218. |
AMA | Gursoy M Longitudinal attacks against iterative data collection with local differential privacy. Turkish Journal of Electrical Engineering and Computer Sciences. 2024; 32(1): 198 - 218. |
Vancouver | Gursoy M Longitudinal attacks against iterative data collection with local differential privacy. Turkish Journal of Electrical Engineering and Computer Sciences. 2024; 32(1): 198 - 218. |
IEEE | Gursoy M "Longitudinal attacks against iterative data collection with local differential privacy." Turkish Journal of Electrical Engineering and Computer Sciences, 32, ss.198 - 218, 2024. |
ISNAD | Gursoy, M. Emre. "Longitudinal attacks against iterative data collection with local differential privacy". Turkish Journal of Electrical Engineering and Computer Sciences 32/1 (2024), 198-218. |