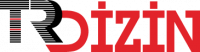
Histopatoloji Görüntülerinde Meme Kanseri Tespiti Için Derin Öğrenme Tabanlı Metodolojinin Geliştirilmesi
Proje Grubu: EEEAG Sayfa Sayısı: 101 Proje No: 121E379 Proje Bitiş Tarihi: 15.11.2023 Metin Dili: Türkçe DOI: 121E379 İndeks Tarihi: 15-03-2024
Histopatoloji Görüntülerinde Meme Kanseri Tespiti Için Derin Öğrenme Tabanlı Metodolojinin Geliştirilmesi
Öz: Teknolojinin ilerlemesiyle birlikte kanser teşhisindeki araştırmaların odak noktası, histopatoloji görüntülerinin dijital ortama aktarılmasıyla değişti. Özellikle meme kanseri teşhisi için kullanılan değerlendirme sürecinde; renk tutarsızlıkları, çekirdek tespiti, mitoz tespiti, tübüler oluşumların tespiti ve kanserli bölgelerin belirlenmesi gibi zorluklar ortaya çıktı. Proje kapsamında bu zorluklara çözüm olarak, otomatik meme kanseri teşhisi ve derecelendirmesi için yeni bir metodoloji önerildi. Önerilen metodoloji yedi aşamadan oluşmaktadır: 1) Renk Normalizasyonu; 2) Çekirdek Segmentasyonu; 3) Mitoz Sayımı ve Tespiti; 4) Nükleer Atipi Değerlendirme; 5) Kanserli Bölge Tespiti; 6) Tübüler Oluşumların Tespiti ve 7) Veri Setleri. Bu adımlar ile beraber patologların subjektif değerlendirmelerine alternatif olmak, tanılarını nicel değerler ile desteklemeleri sağlanacaktır. Bu çalışmanın amacı, otomatik veya yarı otomatik meme kanseri tespit ve derecelendirme sistemi geliştirilmesine yardımcı olabilecek özgün bir metodoloji geliştirmektir. Önerilen yedi aşamalı metodoloji için yapılan katkılar şöyle açıklanabilir: 1) Histopatoloji görüntülerinde boyama tutarsızlığını en aza indiren bir renk normalizasyonu yöntemi uygulandı. 2) Çekirdek segmentasyonu için özgün iki derin öğrenme mimarisi (Lightweight U-Net ve ComSegNet) önerildi. 3) Mitoz sayımı ve tespiti için derin öğrenme ve bulanık mantık yöntemlerini içeren özgün bir metodoloji geliştirildi. 4) Histopatolojik görüntülerde morfolojik olarak bulunan doku heterojenliğini özetleyen bir nükleer atipi değerlendirme yaklaşımı uygulandı. 5) Kanserli bölge tespiti için literatürdeki derin öğrenme yöntemleri uygulandı. 6) Tübüler oluşumların tespiti için özgün bir dikkat modelli U-Net modeli önerildi. 7) Çekirdek ve mitoz verileri içeren, özgün iki adet HE boyalı dijital histopatoloji veri seti oluşturuldu. Proje, disiplinler arası bir projedir. Projede, Ankara Üniversitesi Patoloji Anabilim Dalı ile ortak çalışarak, meme dokusu üzerine HE boyalı dijital histopatoloji veri seti oluşturulmuştur. Bu çalışma, meme kanseri teşhisinde daha doğru, objektif ve güvenilir bir yöntem sunarak klinik uygulamalara katkıda bulunmayı hedeflemektedir.
Anahtar Kelime: Title : Development of a Deep Learning Based Methodology for Breast Cancer Detection in Histopathology Images
Öz: With the advancement of technology, research focus in cancer diagnosis has shifted towards digitizing histopathology images. Particularly in the assessment process for breast cancer diagnosis, challenges such as color inconsistencies, nuclei segmentation, mitosis detection, identification of tubular formations, and delineation of cancerous regions have emerged. As a solution within this project, a new methodology has been proposed for automated breast cancer diagnosis and grading. The suggested methodology comprises seven stages: 1) Color Normalization; 2) Nuclei Segmentation; 3) Mitosis Enumeration and Detection; 4) Nuclei Atypia Evaluation; 5) Cancerous Region Detection; 6) Tubular Segmentation; and 7) Datasets. These steps aim to offer an alternative to pathologists' subjective assessments, encouraging their diagnoses to be supported by quantitative values. The objective of this study is to develop an original methodology that could assist in the creation of an automatic or semi-automatic breast cancer detection and grading system. Contributions made to the proposed seven-stage methodology are outlined as follows: 1) Application of a color normalization technique to minimize staining inconsistencies in histopathology images. 2) Proposal of two unique deep learning architectures (Lightweight U-Net and ComSegNet) for nuclei segmentation. 3) Development of an original methodology incorporating deep learning and fuzzy logic for mitosis enumeration and detection. 4) Implementation of a nuclear atypia evaluation approach summarizing morphological tissue heterogeneity in histopathological images. 5) Utilization of deep learning methods from the literature for cancerous region detection. 6) Proposal of a unique attention-based U-Net model for segmentation of tubular formations. 7) Creation of two original HE stained digital histopathology datasets containing nuclei and mitosis data. This interdisciplinary project, conducted in collaboration with Ankara University's Department of Pathology, aimed to generate HE stained digital histopathology datasets on breast tissue. Its goal is to contribute to clinical applications by presenting a more accurate, objective, and reliable method for breast cancer diagnosis.
Anahtar Kelime: Erişim Türü: Bibliyografik
- [1] J. Wardle, K. Robb, S. Vernon, J. Waller, “Screening for prevention and early diagnosis of cancer,” American psychologist, vol. 70, no. 2, p.119, 2015.
- [2] T. Mathew, R. K. Jyoti, and R. Jeny, “Computational methods for automated mitosis detection in histopathology images: A review,” Biocybernetics and Biomedical Engineering, vol. 41, no.1, pp. 64-82, 2021.
- [3] R. Ludovic, R. Daniel, L. Nicolas, K. Maria, I. Humayun, K. Jacques and G.Catherine, “Mitosis detection in breast cancer histological images An ICPR 2012 contest,” Journal of pathology informatics, vol. 4, no. 1, p. 8, 2013.
- [4] SE. Singletary, C. Allred, P. Ashley, LW. Bassett, D. Berry, K. Bland, “Revision of the American joint committee on cancer staging system for breast cancer,” J Clin Oncol, vol. 20, no. 17, pp. 3628–36, 2002.
- [5] N. Nemati, E. Hancer, R. Samet, Z. Yıldırım and M. Traore,”A Comparative Study Of Deep Semantic Segmentation Architectures For Mitosis Detection In Histopathology Images,” 9ROXPH, 2022, p. 363.
- [6] M. T. Mali, E. Hancer, R. Samet, Z. Yıldırım, and N Nemati, “Detection of Colorectal Cancer with Vision Transformers,” in 2022 of IEEE Innovations in Intelligent Systems and Applications Conference (ASYU), 2022, pp.1-6.
- [7] H. U. Khan, B.Raza, M. H. Shah, S. M. Usama, P. Tiwari and S. S. Band, “SMDetector: Small mitotic detector in histopathology images using faster R-CNN with dilated convolutions in backbone model,” Biomedical Signal Processing and Control, vol 81, p. 104414, 2023.
- [8] S. Razavi, F. D. Khameneh, H. Nouri, D. Androutsos, S. J. Done and A. Khademi, “MiNuGAN: Dual Segmentation of Mitoses and Nuclei Using Conditional GANs on Multi-center Breast H&E Images,” Journal of pathology informatics, vol. 13, p. 100002, 2022.
- [9] N. Banerjee, A. S. Sethi, M. Singh, G. S. Anagh, B. S. Upendra, A. Krohn-Grimberghe and R. Vyas, “Mitosis Detection Using Image Segmentation and Object Detection” in 2019 IEEE Conference on Information and Communication Technology, 2019, pp. 1-6.
- [10] R. E. Yancey, “Deep Feature Fusion for Mitosis Counting” in 2022 3 rd IEEE International Conference on Pattern Recognition and Machine Learning (PRML), 2022, pp. 151-158. MVML 111-7
- [11] S. Çayır, G. Solmaz, H. Kusetogullari, F. Tokat, E. Bozaba, S. Karakaya, O. Kılıç, “MITNET: a novel dataset and a two-stage deep learning approach for mitosis recognition in whole slide images of breast cancer tissue,” Neural Computing and Applications, pp. 1-15, 2022.
- [12] R. Nateghi, H. Danyali, and M. S. Helfroush, “A deep learning approach for mitosis detection: Application in tumor proliferation prediction from whole slide images,” Artificial intelligence in medicine, vol. 114. p. 102048, 2021.
- [13] M. U. Rehman, S. Akhtar, M. Zakwan and M. H. Mahmood, “Novel architecture with selected feature vector for effective classification of mitotic and non-mitotic cells in breast cancer histology images,” Biomedical Signal Processing and Control, vol. 71, p. 103212, 2022.
- [14] R. M. Thomas and J. Jisha. “Detection and segmentation of mitotic cell nuclei in breast histopathology images,” in:2017 International Conference on Networks & Advances in Computational Technologies (NetACT). IEEE, 2017. pp. 246-250.
- [15] X. Wang, J. Zhang, S. Yang, J. Xiang, F. Luo, M. Wang and X. Han, “A generalizable and robust deep learning algorithm for mitosis detection in multicenter breast histopathological images,” Medical Image Analysis, vol. 84, p. 102703, 2022.
- [16] M. Hwang, D. Wang, C. Wu, W.C. Jiang, X.X. Kong, K.S. Hwang, and K. Ding, “A fuzzy segmentation method to learn classification of mitosis,” International Journal of Fuzzy Systems, vol. 22, no. 5, pp. 1653-1664, 2020.
- [17] B. Lakshmanan, S. Priyadharsini and B. Selvakumar, “Computer-assisted mitotic figure detection in histopathology images based on DenseNetPCA framework”, Materials Today: Proceedings, 2022.
- [18] N. Maroof, A. Khan, S. A. Qureshi, A. ul Rehman, R, K. Khalil, and S. O. Shim, “Mitosis detection in breast cancer histopathology images using hybrid feature space,” Photodiagnosis and Photodynamic Therapy, vol. 31, p. 101885, 2020.
- [19] L. Roux, D. Racoceanu, F. Capron, J. Calvo, E. Attieh, G. Le Naour and A. Gloaguen, “MITOS & ATYPIA-Detection of mitosis and evaluation of nuclear atypia score in breast cancer histological images,” IPAL, Agency Sci, Technol Res Inst Infocom Res. Technol. Res. Inst. Infocom Res., Singapore, Tech. Rep.
- [20] M. Veta, Y. Heng, N. Stathonikos, B. E. Bejnordi, F. Beca, T. Wollmann and J.P. Pluim, “Predicting breast tumor proliferation from whole-slide images” the TUPAC16 challenge. Medical image analysis,vol. 54, pp. 111-121, 2019.
- [21] M. Aubreville, N. Stathonikos, C. A. Bertram, R. Klopleisch, N. ter Hoeve, F. Ciompi and K.Breininger, “Mitosis domain generalization in histopathology images,” The MIDOG challenge, arXiv preprint arXiv:2204.03742, 2022.
- [22] M. Veta, M. A. Viergever, J. P. Pluim, N. Stathonikos, and P. J. van Diest, “MICCAI Grand challenge: Assessment of mitosis detection algorithms,” AMIDA13.
- [23] Z. Yıldırım, E. Hançer, R. Samet, M. T. Mali and N. Nemati, “Effect of Color Normalization on Nuclei Segmentation Problem in H&E Stained Histopathology Images,” in 2022 30th IEEE Signal Processing and Communications Applications Conference (SIU), 2022, pp. 1-4.
- [24] M. Macenko, M. Niethammer, J. S. Marron, D. Borland, J. T. Woosley, X. Guan and N. E. Thomas, “A method for normalizing histology slides for quantitative analysis,” in 2009 IEEE international symposium on biomedical imaging: from nano to macro, 2009, pp. 1107-1110.
- [25] B. Lakshmanan, S. Anand and T. Jenitha, "Stain removal through color normalization of hematoxylin and eosin images: A review," Journal of Physics: Conference Series. Vol. 1362. No. 1. P. 012108, 2019.
- [26] G. Jocher, A. Stoken and J. Borovec, “NanoCode012, ChristopherSTAN, L,” Changyu, Laughing, tkianai, A. Hogan, lorenzomammana, yxNONG, AlexWang1900, L. Diaconu, Marc, wanghaoyang0106, ml5ah, Doug, F. Ingham, Frederik, Guilhen, Hatovix, J. Poznanski, J. Fang, L. Yu, changyu98, M. Wang, N. Gupta, O. Akhtar, PetrDvoracek, and P. Rai, ultralytics/yolov5: v3, vol. 1, 2020.
- [27] L. Zhu,X. Geng, Z. Li, and C. Liu, “Improving yolov5 with attention mechanism for detecting boulders from planetary images,” Remote Sensing, vol. 13, no. 18, p. 3776, 2021.
- [28] P. Bonissone, J. M. Cadenas, M. C. Garrido and R. A. Díaz-Valladares, “A fuzzy random forest”. International Journal of Approximate Reasoning, vol. 51, no. 7, pp. 729-747, 2010. MVML 111-8
- [29] J. M. Keller, M. R. Gray, and J. A. Givens, “A fuzzy k-nearest neighbor algorithm,” IEEE Trans. Syst., Man, Cybern., Vol. 15, pp. 580–5, Jul.–Aug. 1985.
- [30] P. K. Simpson, “Fuzzy min-max neural networks. I. Classification,” IEEE Trans. Neural Netw., vol. 3, no. 5, pp. 776– 786, Sep. 1992.
APA | SAMET R, YILDIRIM Z, Kirmizi A, Hancer E, SAK S, TOLAKAN N, TRAROE M, AKKAŞ E (2023). Histopatoloji Görüntülerinde Meme Kanseri Tespiti Için Derin Öğrenme Tabanlı Metodolojinin Geliştirilmesi. , 0 - 101. 121E379 |
Chicago | SAMET REFİK,YILDIRIM Zeynep,Kirmizi Ayca,Hancer Emrah,SAK SERPİL,TOLAKAN NOOSHIN NEMATI,TRAROE MOHAMED,AKKAŞ EYYÜB ENSAR Histopatoloji Görüntülerinde Meme Kanseri Tespiti Için Derin Öğrenme Tabanlı Metodolojinin Geliştirilmesi. (2023): 0 - 101. 121E379 |
MLA | SAMET REFİK,YILDIRIM Zeynep,Kirmizi Ayca,Hancer Emrah,SAK SERPİL,TOLAKAN NOOSHIN NEMATI,TRAROE MOHAMED,AKKAŞ EYYÜB ENSAR Histopatoloji Görüntülerinde Meme Kanseri Tespiti Için Derin Öğrenme Tabanlı Metodolojinin Geliştirilmesi. , 2023, ss.0 - 101. 121E379 |
AMA | SAMET R,YILDIRIM Z,Kirmizi A,Hancer E,SAK S,TOLAKAN N,TRAROE M,AKKAŞ E Histopatoloji Görüntülerinde Meme Kanseri Tespiti Için Derin Öğrenme Tabanlı Metodolojinin Geliştirilmesi. . 2023; 0 - 101. 121E379 |
Vancouver | SAMET R,YILDIRIM Z,Kirmizi A,Hancer E,SAK S,TOLAKAN N,TRAROE M,AKKAŞ E Histopatoloji Görüntülerinde Meme Kanseri Tespiti Için Derin Öğrenme Tabanlı Metodolojinin Geliştirilmesi. . 2023; 0 - 101. 121E379 |
IEEE | SAMET R,YILDIRIM Z,Kirmizi A,Hancer E,SAK S,TOLAKAN N,TRAROE M,AKKAŞ E "Histopatoloji Görüntülerinde Meme Kanseri Tespiti Için Derin Öğrenme Tabanlı Metodolojinin Geliştirilmesi." , ss.0 - 101, 2023. 121E379 |
ISNAD | SAMET, REFİK vd. "Histopatoloji Görüntülerinde Meme Kanseri Tespiti Için Derin Öğrenme Tabanlı Metodolojinin Geliştirilmesi". (2023), 0-101. https://doi.org/121E379 |
APA | SAMET R, YILDIRIM Z, Kirmizi A, Hancer E, SAK S, TOLAKAN N, TRAROE M, AKKAŞ E (2023). Histopatoloji Görüntülerinde Meme Kanseri Tespiti Için Derin Öğrenme Tabanlı Metodolojinin Geliştirilmesi. , 0 - 101. 121E379 |
Chicago | SAMET REFİK,YILDIRIM Zeynep,Kirmizi Ayca,Hancer Emrah,SAK SERPİL,TOLAKAN NOOSHIN NEMATI,TRAROE MOHAMED,AKKAŞ EYYÜB ENSAR Histopatoloji Görüntülerinde Meme Kanseri Tespiti Için Derin Öğrenme Tabanlı Metodolojinin Geliştirilmesi. (2023): 0 - 101. 121E379 |
MLA | SAMET REFİK,YILDIRIM Zeynep,Kirmizi Ayca,Hancer Emrah,SAK SERPİL,TOLAKAN NOOSHIN NEMATI,TRAROE MOHAMED,AKKAŞ EYYÜB ENSAR Histopatoloji Görüntülerinde Meme Kanseri Tespiti Için Derin Öğrenme Tabanlı Metodolojinin Geliştirilmesi. , 2023, ss.0 - 101. 121E379 |
AMA | SAMET R,YILDIRIM Z,Kirmizi A,Hancer E,SAK S,TOLAKAN N,TRAROE M,AKKAŞ E Histopatoloji Görüntülerinde Meme Kanseri Tespiti Için Derin Öğrenme Tabanlı Metodolojinin Geliştirilmesi. . 2023; 0 - 101. 121E379 |
Vancouver | SAMET R,YILDIRIM Z,Kirmizi A,Hancer E,SAK S,TOLAKAN N,TRAROE M,AKKAŞ E Histopatoloji Görüntülerinde Meme Kanseri Tespiti Için Derin Öğrenme Tabanlı Metodolojinin Geliştirilmesi. . 2023; 0 - 101. 121E379 |
IEEE | SAMET R,YILDIRIM Z,Kirmizi A,Hancer E,SAK S,TOLAKAN N,TRAROE M,AKKAŞ E "Histopatoloji Görüntülerinde Meme Kanseri Tespiti Için Derin Öğrenme Tabanlı Metodolojinin Geliştirilmesi." , ss.0 - 101, 2023. 121E379 |
ISNAD | SAMET, REFİK vd. "Histopatoloji Görüntülerinde Meme Kanseri Tespiti Için Derin Öğrenme Tabanlı Metodolojinin Geliştirilmesi". (2023), 0-101. https://doi.org/121E379 |