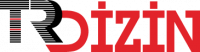
Algı Temelli Çizim İşleme
Proje Grubu: EEEAG Sayfa Sayısı: 75 Proje No: 120E489 Proje Bitiş Tarihi: 01.11.2023 Metin Dili: Türkçe DOI: 120E489 İndeks Tarihi: 04-04-2024
Algı Temelli Çizim İşleme
Öz: Çizimler insanların fikirlerini, tasarımlarını ve konseptlerini görsel olarak aktarmaları için hızlı ve etkili bir araçtır. Teknolojinin ilerlemesinin bir sonucu olarak dokunmatik ekranların kullanımı artmıştır ve çizimler hayatımızda her geçen gün daha etkin bir rol oynamaktadır. Bu nedenle çizimlerin tanınması ve analizi gerekli bir çalışma konusu haline gelmiştir. Günlük hayattaki çizimler az ayrıntı içeren düşük kaliteli bir yapıya sahiptir. Bu çizimler bulunduğu çevreye göre anlam kazanır. Fakat çizim alanındaki temel çalışmalar, çizim objelerinin tek başına tanınabilirliği üzerine kuruludur ve literatürdeki veri setleri de ya tekil çizim objeleri içermektedir ya da günlük hayatta oluşturduğumuz çizimlerin özelliklerini yeteri kadar taşımamaktadır. Projemizde, bu problemleri temel alan çözümler önerilmiştir. Projemizin ilk amacı çizimlerin çok-çağrışımlı özelliğini ön plana çıkaran bir yaklaşım sunmaktır. Bu amaçla toplanan SketchNet veri setimiz literatürdeki çizim veri setlerine gündelik hayattaki çizim davranışlarını daha iyi temsil eden bir veri setini kazandırmıştır. Böylelikle çizimlerin farklı şartlarda ne çağrıştırdığını da sorgulayan bir yaklaşım getirilmiştir. Projemizin ikinci amacı sahne içerisinde yer alan çizim nesnelerinin bir arada değerlendirilerek tanındığı bir yapı ortaya koymaktır. Bu amaç doğrultusunda, çizim bölütleme ve tanıma yapay zeka modelleri geliştirilmiştir. Hedefimiz, sahne nesne çizimlerinin tanınmasında harici yazılı ve görsel bilgi kaynaklarının da kullanılması ile daha iyi bir tanıma yapmaktır. Bu amaç doğrultusunda, üç aşamadan oluşan bir yapay zeka yapısı tasarlanmıştır. İlk aşamada, sahnede bulunan nesne çizimlerini bölütleme algoritması çalışmaktadır. Bu algoritmanın amacı, sahnedeki her bir nesnenin vuruşlarına göre ayırt edilmesidir. İkinci aşamada, projemizin bir ana çıktısı olarak toplanan SketchNet veri setinden yararlanılarak sahnedeki her bir nesne için anlam listeleri oluşturulmuştur. Üçüncü aşamada ise sahnedeki nesnelerin konum, boyut ve nesne özellikleri kullanılarak mekansal özelliklerin nesne tanımadaki katkısını öne çıkartmak hedeflenmiştir. Bu çalışma, sahne çizim tanıma alanında hem yazılı hem görsel bilgiyi işleyerek nesnelerin mekansal özelliklerinden yararlanan ilk çalışma olması açısından literatürde önem arz etmektedir.
Anahtar Kelime: Human Perception Based Sketch Processing
Öz: Sketching is a quick and effective tool for individuals to visually express their thoughts, designs, and concepts. With technological progress, the prevalence of touch screens has grown, elevating the significance of sketches in our daily lives. Consequently, the recognition and analysis of sketches have become a necessary subject of study. Daily-life sketches have a low-quality structure with few details. These sketches gain meaning depending on their environment. However, foundational research in sketch studies often focuses on the recognizable aspects of individual drawn objects. Moreover, the datasets in the literature either feature single sketch objects or lack the nuanced characteristics found in our daily-life sketches. In our project, we present solutions addressing these issues. The first aim of our project is to present an approach that highlights the multi-associative nature of drawings. Our SketchNet dataset collected for this purpose has provided a dataset that better represents drawing behaviors in daily life compared to the sketch datasets in the literature. Thus, an approach was introduced that questions what the sketches evoke in different conditions. The second aim of our project is to establish a framework for the joint evaluation and recognition of drawing objects within a scene. To achieve this, we have developed two artificial intelligence models for sketch scene segmentation and sketch recognition. Our goal is to make better recognition of scene sketches by using external written and visual information sources. For this purpose, we designed an artificial intelligence structure comprising three stages. In the initial stage, the segmentation model operates to differentiate each object within the scene at both stroke and instance levels. Subsequently, meaning lists are extracted for each object using the SketchNet dataset, a principal output of our project. Finally, in the third stage, our focus is to highlight the contribution of spatial features to object recognition by incorporating the location, size, and specific features of the objects within the scene. This study is important as it is the first study to benefit from the spatial features of sketch objects by processing both written and visual information in the field of scene sketch recognition.
Anahtar Kelime: Erişim Türü: Bibliyografik
- ● Bar, M. and Aminoff, E. (2003) Cortical analysis of visual context. Neuron 38, 347–358
- ● Barbot, B., Besançon, M., & I Lubart, T. (2011). Assessing creativity in the classroom. The open
- ● Barenholtz, E. (2014). Quantifying the role of context in visual object recognition. Visual Cognition, 22(1), 30-56.
- ● Biederman, I. (1981). On the semantics of a glance at a scene. Perceptual organization, 213, 253.
- ● Blei, D. M., Ng, A. Y., & Jordan, M. I. (2003). Latent dirichlet allocation. Journal of machine Learning research, 3(Jan), 993-1022.
- ● Brooks, J. L. (2012). Counterbalancing for serial order carryover effects in experimental condition orders. Psychological methods, 17(4), 600.
- ● Bulling, A., & Roggen, D. (2011, September). Recognition of visual memory recall processes using eye movement analysis. In Proceedings of the 13th international conference on Ubiquitous computing (pp. 455-464).
- ● Chowdhury, P. N., Sain, A., Bhunia, A. K., Xiang, T., Gryaditskaya, Y., & Song, Y. Z. (2022, October). Fs-coco: Towards understanding of freehand sketches of common objects in context. In European Conference on Computer Vision (pp. 253-270). Cham: Springer Nature Switzerland.
- ● Eckert, C., & Stacey, M. (2000). Sources of inspiration: a language of design. Design studies, 21(5), 523-538.
- ● Eitz, M., Hays, J., & Alexa, M. (2012). How do humans sketch objects?. ACM Transactions on graphics (TOG), 31(4), 1-10.
- ● Finke, R. A., & Slayton, K. (1988). Explorations of creative visual synthesis in mental imagery. Memory & cognition, 16(3), 252-257.
- ● Fonteyn, M. E., Kuipers, B., & Grobe, S. J. (1993). A description of think aloud method and protocol analysis. Qualitative Health Research, 3(4), 430-441.
- ● Gao, C., Liu, Q., Xu, Q., Wang, L., Liu, J., & Zou, C. (2020). Sketchycoco: Image generation from freehand scene sketches. In Proceedings of the IEEE/CVF conference on computer vision and pattern recognition (pp. 5174-5183).
- ● Ge, C., Sun, H., Song, Y. Z., Ma, Z., & Liao, J. (2022). Exploring local detail perception for scene sketch semantic segmentation. IEEE Transactions on Image Processing, 31, 1447-1461.
- ● Ge, Z., Liu, S., Wang, F., Li, Z., & Sun, J. (2021). Yolox: Exceeding yolo series in 2021. arXiv preprint arXiv:2107.08430.
- ● Gero, J. S. (1990). Design prototypes: a knowledge representation schema for design. AI magazine, 11(4), 26-26.
- ● Goodfellow, I., Pouget-Abadie, J., Mirza, M., Xu, B., Warde-Farley, D., Ozair, S., Courville, A., Bengio, Y. 2014. “Generative adversarial nets”, Advances in neural information processing systems, 2672-80.
- ● Greenberg, S. (2001). Context as a dynamic construct. Human–Computer Interaction, 16(2-4), 257-268.
- ● Griffiths, T., Jordan, M., Tenenbaum, J., & Blei, D. (2003). Hierarchical topic models and the nested Chinese restaurant process. Advances in neural information processing systems, 16.
- ● Ha, D., & Eck, D. (2017). A neural representation of sketch drawings. arXiv preprint arXiv:1704.03477.
- ● Huang, Z., Fu, H., & Lau, R. W. (2014). Data-driven segmentation and labeling of freehand sketches. ACM Transactions on Graphics (TOG), 33(6), 1-10.
- ● Jongejan, J., Rowley, H., Kawashima, T., Kim, J., & Fox-Gieg, N. (2017). The quick, draw! dataset. 2017-5-15)[2021-03-02]. https://github. com/googlecreativelab/quickdraw-dataset.
- ● Karimi, P., Rezwana, J., Siddiqui, S., Maher, M. L., & Dehbozorgi, N. (2020, March). Creative sketching partner: an analysis of human-AI co-creativity. In Proceedings of the 25th International Conference on Intelligent User Interfaces (pp. 221-230).
- ● Lin, T. Y., Maire, M., Belongie, S., Hays, J., Perona, P., Ramanan, D., ... & Zitnick, C. L. (2014, September). Microsoft coco: Common objects in context. In European conference on computer vision (pp. 740-755). Springer, Cham.
- ● MacQueen, J. (1967, June). Some methods for classification and analysis of multivariate observations. In Proceedings of the fifth Berkeley symposium on mathematical statistics and probability (Vol. 1, No. 14, pp. 281-297).
- ● Malmberg, K. J., Criss, A. H., Gangwani, T. H., & Shiffrin, R. M. (2012). Overcoming the negative consequences of interference from recognition memory testing. Psychological Science, 23(2), 115-119.
- ● Mikolov, T., Chen, K., Corrado, G., & Dean, J. (2013). Efficient estimation of word representations in vector space. arXiv preprint arXiv:1301.3781.
- ● Murnane, K., & Phelps, M. P. (1994). When does a different environmental context make a difference in recognition? A global activation model. Memory & Cognition, 22(5), 584-590.
- ● Radford, A., Metz, L., Chintala, S. 2015. “Unsupervised representation learning with deep convolutional generative adversarial networks”, arXiv, 1511.06434.
- ● Reimers, N., & Gurevych, I. (2019). Sentence-bert: Sentence embeddings using siamese bert-networks. arXiv preprint arXiv:1908.10084.
- ● Ribeiro, L. S. F., Bui, T., Collomosse, J., & Ponti, M. (2020). Sketchformer: Transformer-based representation for sketched structure. In Proceedings of the IEEE/CVF conference on computer vision and pattern recognition (pp. 14153-14162).
- ● Robin, J., & Olsen, R. K. (2019). Scenes facilitate associative memory and integration. Learning & Memory, 26(7), 252-261
- ● Rodrigues, S., Figueiras, A., & Alexandre, I. (2019, July). Once Upon a Time in a Land Far Away: Guidelines for Spatio-Temporal Narrative Visualization. In 2019 23rd International Conference Information Visualisation (IV) (pp. 44-49). IEEE.
- ● Saggar, M., Quintin, E. M., Kienitz, E., Bott, N. T., Sun, Z., Hong, W. C., ... & Reiss, A. L. (2015).
- ● Sangkloy, P., Burnell, N., Ham, C., & Hays, J. (2016). The sketchy database: learning to retrieve badly drawn bunnies. ACM Transactions on Graphics (TOG), 35(4), 1-12.
- ● Smith, S. M., Glenberg, A., & Bjork, R. A. (1978). Environmental context and human memory. Memory & Cognition, 6(4), 342-353.
- ● Stiny, G. 2006. Shape: talking about seeing and doing. Cambridge, MA.: The MIT Press.
- ● Stones, C., & Cassidy, T. (2010). Seeing and Discovering: How do Student Designers Reinterpret Sketches and Digital Marks During Graphic Design Ideation? Design Studies, 31(5), 439-460.
- ● Sun, Z., Wang, C., Zhang, L., & Zhang, L. (2012). Free hand-drawn sketch segmentation. In Computer Vision–ECCV 2012: 12th European Conference on Computer Vision, Florence, Italy, October 7-13, 2012, Proceedings, Part I 12 (pp. 626-639). Springer Berlin Heidelberg.
- ● Tırkaz, C., Yanıkoğlu B., Sezgin T.M. 2012. “Sketched symbol recognition with auto-completion”, Pattern Recognition, 45(11), 3926-37.
- ● Topal, B. B., Yuret, D., & Sezgin, T. M. (2023, August). Domain-adaptive self-supervised face & body detection in drawings. In Proceedings of the Thirty-Second International Joint Conference on Artificial Intelligence (pp. 1432-1439).
- ● Tversky, B. (2009). Spatial cognition: Embodied and situated.
- ● Tversky, B., & Hemenway, K. (1983). Categories of environmental scenes. Cognitive psychology, 15(1), 121-149.
- ● Wu, X., Qi, Y., Liu, J., & Yang, J. (2018, September). Sketchsegnet: A rnn model for labeling sketch strokes. In 2018 IEEE 28th International Workshop on Machine Learning for Signal Processing (MLSP) (pp. 1-6). IEEE.
- ● Yan, X., Yang, J., Sohn, K., Lee, H. 2016. “Attribute2image: Conditional image generation from visual attributes”, European Conference on Computer Vision, 776-91.
- ● Yu, Q., Yang, Y., Song, Y. Z., Xiang, T., & Hospedales, T. (2015). Sketch-a-net that beats humans. arXiv preprint arXiv:1501.07873.
- ● Zhang, Z., Deng, X., Li, J., Lai, Y., Ma, C., Liu, Y., & Wang, H. (2023). Stroke-based semantic segmentation for scene-level free-hand sketches. The Visual Computer, 39(12), 6309-6321.
- ● Zhao, T., Yang, J., Zhang, H., & Siu, K. W. M. (2021). Creative idea generation method based on deep learning technology. International Journal of Technology and Design Education, 31(2), 421-440.
- ● Zheng, Y., Yao, H., & Sun, X. (2020). Deep semantic parsing of freehand sketches with homogeneous transformation, soft-weighted loss, and staged learning. IEEE Transactions on Multimedia, 23, 3590-3602.
- ● Zou, C., Yu, Q., Du, R., Mo, H., Song, Y. Z., Xiang, T., ... & Zhang, H. (2018). Sketchyscene: Richly-annotated scene sketches. In Proceedings of the european conference on computer vision (ECCV) (pp. 421-436).
- ● Xu, P., Joshi, C. K., & Bresson, X. (2021). Multigraph transformer for free-hand sketch recognition. IEEE Transactions on Neural Networks and Learning Systems, 33(10), 5150-5161.
APA | SEZGİN T, Urgen B, ÇELİK B, DEDE E, KÜTÜK A (2023). Algı Temelli Çizim İşleme. , 0 - 75. 120E489 |
Chicago | SEZGİN Tevfik Metin,Urgen Burcu A.,ÇELİK BİRKAN,DEDE Ezgi,KÜTÜK ALEYNA Algı Temelli Çizim İşleme. (2023): 0 - 75. 120E489 |
MLA | SEZGİN Tevfik Metin,Urgen Burcu A.,ÇELİK BİRKAN,DEDE Ezgi,KÜTÜK ALEYNA Algı Temelli Çizim İşleme. , 2023, ss.0 - 75. 120E489 |
AMA | SEZGİN T,Urgen B,ÇELİK B,DEDE E,KÜTÜK A Algı Temelli Çizim İşleme. . 2023; 0 - 75. 120E489 |
Vancouver | SEZGİN T,Urgen B,ÇELİK B,DEDE E,KÜTÜK A Algı Temelli Çizim İşleme. . 2023; 0 - 75. 120E489 |
IEEE | SEZGİN T,Urgen B,ÇELİK B,DEDE E,KÜTÜK A "Algı Temelli Çizim İşleme." , ss.0 - 75, 2023. 120E489 |
ISNAD | SEZGİN, Tevfik Metin vd. "Algı Temelli Çizim İşleme". (2023), 0-75. https://doi.org/120E489 |
APA | SEZGİN T, Urgen B, ÇELİK B, DEDE E, KÜTÜK A (2023). Algı Temelli Çizim İşleme. , 0 - 75. 120E489 |
Chicago | SEZGİN Tevfik Metin,Urgen Burcu A.,ÇELİK BİRKAN,DEDE Ezgi,KÜTÜK ALEYNA Algı Temelli Çizim İşleme. (2023): 0 - 75. 120E489 |
MLA | SEZGİN Tevfik Metin,Urgen Burcu A.,ÇELİK BİRKAN,DEDE Ezgi,KÜTÜK ALEYNA Algı Temelli Çizim İşleme. , 2023, ss.0 - 75. 120E489 |
AMA | SEZGİN T,Urgen B,ÇELİK B,DEDE E,KÜTÜK A Algı Temelli Çizim İşleme. . 2023; 0 - 75. 120E489 |
Vancouver | SEZGİN T,Urgen B,ÇELİK B,DEDE E,KÜTÜK A Algı Temelli Çizim İşleme. . 2023; 0 - 75. 120E489 |
IEEE | SEZGİN T,Urgen B,ÇELİK B,DEDE E,KÜTÜK A "Algı Temelli Çizim İşleme." , ss.0 - 75, 2023. 120E489 |
ISNAD | SEZGİN, Tevfik Metin vd. "Algı Temelli Çizim İşleme". (2023), 0-75. https://doi.org/120E489 |