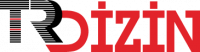
Tek-Hücre Seviyeli Proteomik Veri Kümelerinin Yatay Bütünleştirilmesi
Proje Grubu: EEEAG Sayfa Sayısı: 62 Proje No: 122E587 Proje Bitiş Tarihi: 15.12.2023 Metin Dili: Türkçe DOI: 122E587 İndeks Tarihi: 16-04-2024
Tek-Hücre Seviyeli Proteomik Veri Kümelerinin Yatay Bütünleştirilmesi
Öz: Kütle spektrometresi ve antikor tabanlı dizileme teknolojilerinin tek-hücre düzeyinde kullanılması, tek-hücre proteomik veri kümelerinin artmasını sağlamıştır. Bu veri kümelerini bütünleştirmek, dizilenen moleküllerin azlığı nedeniyle sıklıkla ortaya çıkan toplu etkiyi ortadan kaldırmak için çok önemlidir. Yüksek boyutlu tek-hücre transkriptomik veri kümelerini yatay bütünleştirmeye yönelik geliştirilen yöntemler tek-hücre proteomik veri kümelerine de uygulanabilmesine karşın, düşük boyutlu proteomik veri kümeleri için özel olarak tasarlanmış bir yaklaşım bütünleştirme işlemini geliştirebilmektedir. Bu projede, antikor tabanlı tek-hücre proteomik veri kümelerinin yatay bütünleştirilmesi amacıyla SCPRO-HI algoritması geliştirilmiştir. Bu yöntem farklı veri kümelerindeki benzer hücreleri eşleştirirken hücre kümeleri için ayırt edici proteinlerin benzerliğine dayalı hiyerarşik bir hücre eşleştirme tekniği önermektedir. Özgün bir varyasyonel otomatik kodlayıcı model kullanılarak protein bollukları daha düşük boyutlu yeni bir uzaya taşımadan üzerlerindeki toplu etkilerden arındırılmaktadır. SCPRO-HI algoritmasının performansı, sentetik ve gerçek dünya tek-hücre proteomik veri kümeleri kullanılarak test edilmiştir. Elde edilen bulgulara göre, geliştirilen algoritmanın yüksek performanslı rakip yöntemlerden daha iyi başarım göstermiştir. Deneysel sonuçlar geliştirilen yöntemin rakiplerinden %75 daha yüksek siluet skoru elde ederken önemli proteinlerin mevcudiyetini %13 daha iyi koruduğunu göstermektedir. Ayrıca, algoritma transkriptomik veri kümelerini ve kütle spektrometresi tabanlı proteomik veri kümelerini bütünleştirmede rekabetçi performans göstererek yüksek boyutlu veri kümelerini bütünleştirmede de kullanılabileceğini göstermiştir.
Anahtar Kelime: Horizontal Integration of Single-cell Proteomic Datasets
Öz: The use of mass spectrometry and antibody-based sequencing technologies at the single-cell level has led to an increase in single-cell proteomic datasets. Integrating these datasets is crucial to eliminate the batch effect that often arises due to their limited sequencing molecules. Although methods for horizontally integrating high-dimensional single-cell transcriptomic datasets can also be applied to single-cell proteomic datasets, a specialized approach explicitly tailored for low-dimensional proteomic datasets may enhance the integration process. In this project, the SCPRO-HI algorithm was developed for horizontal integration of antibody-based single-cell proteomics datasets. This method proposes a hierarchical cell matching technique based on the similarity of distinctive proteins for cell clusters while matching similar cells in different datasets. Using a unique variational autoencoder model, protein abundances are cleaned from batch effects without mapping them to a new lower-dimensional space. The performance of the SCPRO-HI algorithm is tested using synthetic and real-world single-cell proteomics datasets. According to the results, the proposed algorithm outperforms the high-performance competing methods. Experimental results show that the proposed method achieves 75% higher silhouette scores than its competitors while maintaining the presence of important proteins 13% better. Furthermore, the algorithm showed competitive performance in integrating transcriptomics datasets and mass spectrometry-based proteomics datasets, demonstrating that it can also be used to integrate high-dimensional datasets.
Anahtar Kelime: Erişim Türü: Erişime Açık
- Angelidis, I., Simon, L. M., Fernandez, I. E., Strunz, M., Mayr, C. H., Greiffo, F. R., ... Schiller, H. B. 2019. "An atlas of the aging lung mapped by single cell transcriptomics and deep tissue proteomics". Nature Communications, 10(1), 963.
- Argelaguet, R., Cuomo, A. S. E., Stegle, O., Marioni, J. C. 2021. "Computational principles and challenges in single-cell data integration". Nature Biotechnology, 39(10), 1202-1215.
- Bennett, H. M., Stephenson, W., Rose, C. M., Darmanis, S. 2023. "Single-cell proteomics enabled by next-generation sequencing or mass spectrometry". Nature Methods, 20(3), 363-374.
- Bjornson-Hooper, Z. B., Fragiadakis, G. K., Spitzer, M. H., Chen, H., Madhireddy, D., Hu, K., ... Nolan, G. P. 2022. "A Comprehensive Atlas of Immunological Differences Between Humans, Mice, and Non-Human Primates". Frontiers in Immunology, 13, 867015.
- Ester, M., Kriegel, H.-P., Sander, J., Xu, X. 1996. "A density-based algorithm for discovering clusters in large spatial databases with noise". İçinde Proceedings of the Second International Conference on Knowledge Discovery and Data Mining (ss. 226-231). Portland, Oregon: AAAI Press.
- Forcato, M., Romano, O., Bicciato, S. 2021. "Computational methods for the integrative analysis of single-cell data". Briefings in Bioinformatics, 22(3), bbaa042.
- Grønbech, C. H., Vording, M. F., Timshel, P. N., Sønderby, C. K., Pers, T. H., Winther, O. 2020. "scVAE: variational auto-encoders for single-cell gene expression data". Bioinformatics, 36(16), 4415-4422.
- Haghverdi, L., Lun, A. T. L., Morgan, M. D., Marioni, J. C. 2018. "Batch effects in single-cell RNA-sequencing data are corrected by matching mutual nearest neighbors". Nature Biotechnology, 36(5), 421-427.
- Hao, Y., Hao, S., Andersen-Nissen, E., Mauck, W. M., Zheng, S., Butler, A., ... Satija, R. 2021. "Integrated analysis of multimodal single-cell data". Cell, 184(13), 3573-3587.e29.
- Hicks, S. C., Townes, F. W., Teng, M., Irizarry, R. A. 2018. "Missing data and technical variability in single-cell RNA-sequencing experiments". Biostatistics, 19(4), 562-578.
- Hie, B., Bryson, B., Berger, B. 2019. "Efficient integration of heterogeneous single- cell transcriptomes using Scanorama". Nature Biotechnology, 37(6), 685- 691.
- Jackson, C. A., Vogel, C. 2022. "New horizons in the stormy sea of multimodal single-cell data integration". Molecular Cell, 82(2), 248-259.
- Johansen, N., Quon, G. 2019. "scAlign: a tool for alignment, integration, and rare cell identification from scRNA-seq data". Genome Biology, 20(1), 166.
- Kingma, D. P., Welling, M. 2022, Aralık 10. "Auto-Encoding Variational Bayes". arXiv.
- Korsunsky, I., Millard, N., Fan, J., Slowikowski, K., Zhang, F., Wei, K., ... Raychaudhuri, S. 2019. "Fast, sensitive and accurate integration of single- cell data with Harmony". Nature Methods, 16(12), 1289-1296.
- Kullback, S., Leibler, R. A. 1951. "On Information and Sufficiency". The Annals of Mathematical Statistics, 22(1), 79-86.
- Leduc, A., Huffman, R. G., Cantlon, J., Khan, S., Slavov, N. 2022. "Exploring functional protein covariation across single cells using nPOP". Genome Biology, 23(1), 1-31.
- Leek, J. T. 2014. "svaseq: removing batch effects and other unwanted noise from sequencing data". Nucleic Acids Research, 42(21), e161.
- Lin, Y., Ghazanfar, S., Wang, K. Y. X., Gagnon-Bartsch, J. A., Lo, K. K., Su, X., ... Yang, J. Y. H. 2019. "scMerge leverages factor analysis, stable expression, and pseudoreplication to merge multiple single-cell RNA-seq datasets". Proceedings of the National Academy of Sciences, 116(20), 9775-9784.
- Longo, S. K., Guo, M. G., Ji, A. L., Khavari, P. A. 2021. "Integrating single-cell and spatial transcriptomics to elucidate intercellular tissue dynamics". Nature Reviews Genetics, 22(10), 627-644.
- Lopez, R., Regier, J., Cole, M. B., Jordan, M. I., Yosef, N. 2018. "Deep generative modeling for single-cell transcriptomics". Nature Methods, 15(12), 1053- 1058.
- Lotfollahi, M., Litinetskaya, A., Theis, F. J. 2022, Mart 17. "Multigrate: single-cell multi-omic data integration". bioRxiv.
- Lotfollahi, M., Naghipourfar, M., Theis, F. J., Wolf, F. A. 2020. "Conditional out-of- distribution generation for unpaired data using transfer VAE". Bioinformatics, 36(Supplement_2), i610-i617.
- Luecken, M. D., Büttner, M., Chaichoompu, K., Danese, A., Interlandi, M., Mueller, M. F., ... Theis, F. J. 2022. "Benchmarking atlas-level data integration in single-cell genomics". Nature Methods, 19(1), 41-50.
- McInnes, L., Healy, J., Melville, J. 2020, Eylül 17. "UMAP: Uniform Manifold Approximation and Projection for Dimension Reduction". arXiv.
- Polański, K., Young, M. D., Miao, Z., Meyer, K. B., Teichmann, S. A., Park, J.-E. 2020. "BBKNN: fast batch alignment of single cell transcriptomes". Bioinformatics, 36(3), 964-965.
- Rahil, Z., Leylek, R., Schürch, C. M., Chen, H., Bjornson-Hooper, Z., Christensen, S. R., ... McIlwain, D. R. 2020. "Landscape of coordinated immune responses to H1N1 challenge in humans". The Journal of Clinical Investigation, 130(11), 5800-5816.
- Rahmah, N., Sitanggang, I. S. 2016. "Determination of Optimal Epsilon (Eps) Value on DBSCAN Algorithm to Clustering Data on Peatland Hotspots in Sumatra". IOP Conference Series: Earth and Environmental Science, 31(1), 012012.
- Saelens, W., Cannoodt, R., Todorov, H., Saeys, Y. 2019. "A comparison of single- cell trajectory inference methods". Nature Biotechnology, 37(5), 547-554.
- Stuart, T., Butler, A., Hoffman, P., Hafemeister, C., Papalexi, E., Mauck, W. M., ... Satija, R. 2019. "Comprehensive Integration of Single-Cell Data". Cell, 177(7), 1888-1902.e21.
- Stuart, T., Satija, R. 2019. "Integrative single-cell analysis". Nature Reviews Genetics, 20(5), 257-272.
- Xu, C., Lopez, R., Mehlman, E., Regier, J., Jordan, M. I., Yosef, N. 2021. "Probabilistic harmonization and annotation of single-cell transcriptomics data with deep generative models". Molecular Systems Biology, 17(1), e9620.
- Yan, X., Zheng, R., Wu, F., Li, M. 2023. "CLAIRE: contrastive learning-based batch correction framework for better balance between batch mixing and preservation of cellular heterogeneity". Bioinformatics, 39(3), btad099.
- Yang, Y., Li, G., Qian, H., Wilhelmsen, K. C., Shen, Y., Li, Y. 2021. "SMNN: batch effect correction for single-cell RNA-seq data via supervised mutual nearest neighbor detection". Briefings in Bioinformatics, 22(3), bbaa097.
- Zhang, F., Wu, Y., Tian, W. 2019. "A novel approach to remove the batch effect of single-cell data". Cell Discovery, 5(1), 1-4.
- Zhu, B., Chen, S., Bai, Y., Chen, H., Liao, G., Mukherjee, N., ... Jiang, S. 2023. "Robust single-cell matching and multimodal analysis using shared and distinct features". Nature Methods, 1-12
APA | KOCA M, Sevilgen F, YANAR F, GÜLOCAK S, YAKAR F (2023). Tek-Hücre Seviyeli Proteomik Veri Kümelerinin Yatay Bütünleştirilmesi. , 0 - 62. 122E587 |
Chicago | KOCA MEHMET BURAK,Sevilgen Fatih Erdoğan,YANAR FATİH,GÜLOCAK SEDA NUR,YAKAR FATİH SELİM Tek-Hücre Seviyeli Proteomik Veri Kümelerinin Yatay Bütünleştirilmesi. (2023): 0 - 62. 122E587 |
MLA | KOCA MEHMET BURAK,Sevilgen Fatih Erdoğan,YANAR FATİH,GÜLOCAK SEDA NUR,YAKAR FATİH SELİM Tek-Hücre Seviyeli Proteomik Veri Kümelerinin Yatay Bütünleştirilmesi. , 2023, ss.0 - 62. 122E587 |
AMA | KOCA M,Sevilgen F,YANAR F,GÜLOCAK S,YAKAR F Tek-Hücre Seviyeli Proteomik Veri Kümelerinin Yatay Bütünleştirilmesi. . 2023; 0 - 62. 122E587 |
Vancouver | KOCA M,Sevilgen F,YANAR F,GÜLOCAK S,YAKAR F Tek-Hücre Seviyeli Proteomik Veri Kümelerinin Yatay Bütünleştirilmesi. . 2023; 0 - 62. 122E587 |
IEEE | KOCA M,Sevilgen F,YANAR F,GÜLOCAK S,YAKAR F "Tek-Hücre Seviyeli Proteomik Veri Kümelerinin Yatay Bütünleştirilmesi." , ss.0 - 62, 2023. 122E587 |
ISNAD | KOCA, MEHMET BURAK vd. "Tek-Hücre Seviyeli Proteomik Veri Kümelerinin Yatay Bütünleştirilmesi". (2023), 0-62. https://doi.org/122E587 |
APA | KOCA M, Sevilgen F, YANAR F, GÜLOCAK S, YAKAR F (2023). Tek-Hücre Seviyeli Proteomik Veri Kümelerinin Yatay Bütünleştirilmesi. , 0 - 62. 122E587 |
Chicago | KOCA MEHMET BURAK,Sevilgen Fatih Erdoğan,YANAR FATİH,GÜLOCAK SEDA NUR,YAKAR FATİH SELİM Tek-Hücre Seviyeli Proteomik Veri Kümelerinin Yatay Bütünleştirilmesi. (2023): 0 - 62. 122E587 |
MLA | KOCA MEHMET BURAK,Sevilgen Fatih Erdoğan,YANAR FATİH,GÜLOCAK SEDA NUR,YAKAR FATİH SELİM Tek-Hücre Seviyeli Proteomik Veri Kümelerinin Yatay Bütünleştirilmesi. , 2023, ss.0 - 62. 122E587 |
AMA | KOCA M,Sevilgen F,YANAR F,GÜLOCAK S,YAKAR F Tek-Hücre Seviyeli Proteomik Veri Kümelerinin Yatay Bütünleştirilmesi. . 2023; 0 - 62. 122E587 |
Vancouver | KOCA M,Sevilgen F,YANAR F,GÜLOCAK S,YAKAR F Tek-Hücre Seviyeli Proteomik Veri Kümelerinin Yatay Bütünleştirilmesi. . 2023; 0 - 62. 122E587 |
IEEE | KOCA M,Sevilgen F,YANAR F,GÜLOCAK S,YAKAR F "Tek-Hücre Seviyeli Proteomik Veri Kümelerinin Yatay Bütünleştirilmesi." , ss.0 - 62, 2023. 122E587 |
ISNAD | KOCA, MEHMET BURAK vd. "Tek-Hücre Seviyeli Proteomik Veri Kümelerinin Yatay Bütünleştirilmesi". (2023), 0-62. https://doi.org/122E587 |