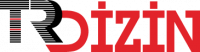
AŞIRI ÖĞRENME MAKİNELERİ İLE HİSSE SENEDİ FİYAT TAHMİNİ
Yıl: 2017 Cilt: 35 Sayı: 1 Sayfa Aralığı: 67 - 89 Metin Dili: Türkçe İndeks Tarihi: 29-07-2022
AŞIRI ÖĞRENME MAKİNELERİ İLE HİSSE SENEDİ FİYAT TAHMİNİ
Öz: Hisse senedi fiyatlarının doğru bir Yrd.Doç.Dr., Kilis7Aralık Universitesi, şekilde tahmin edilebilmesinin birçok İktisadi ve İdari Bilimler Fakültesi, faydalı tarafları mevcuttur. Yapay sinir Uluslararası Ticaret ve Lojistik Bölümü, ağları hisse senedi fiyat tahmininde mozcalici©kilis.edu.tr başarıyla kullanılan yöntemlerden biridir. Fakat öğrenme sürecinin yavaş olması, kullanıcının birçok parametreye karar vermek zorunda olması bu yöntemin dezavantajlarını oluşturmaktadır. Bu olumsuz durumları ortadan kaldırmak için Aşırı Öğrenme Makineleri (AÖM) adı verilen yöntem geliştirilmiştir. Literatürde henüz farklı çeşitlerde aktivasyon fonksiyonu içeren AÖM modellerinin hisse senedi tahmin performansı üzerindeki etkisi incelenmemiştir. Bu çalışmada on dört farklı aktivasyon fonksiyonunun kullanıldığı modeller oluşturulmuş ve modellerin performansları istatistik-- sel ve finansal açıdan değerlendirmeye tabi tutulmuştur. Çalışmada Goodyear, Amazon ve Wal-- Mart isimli şirketlere ve SP500 Endeksine ait geçmiş fiyat ve işlem hacmi bilgilerini kullanmak suretiyle 12 adet teknik gösterge hesaplanmış ve modellerin ğirdi değişkeni olarak kullanılmıştır. Çıktı değişkeni ise bir sonraki güne ait kapanış fiyatlarını içermektedir. Modellerin performansı geleneksel yapay sinir ağları modeli ile karşılaştırılmıştır. Sonuçta %59.32 "ye varan oranda fiyatların yönü doğru bir şekilde öngörülebilmiştir. Ayrıca kâğıt üzerinde, al ve tut stratejisinden daha yüksek oranda ğetiriler elde edilmiştir. Sonuçlar, AÖM modelinin hisse senedi fiyat tahmininde güçlü bir alternatif olduğunu göstermektedir.
Anahtar Kelime: Konular:
PREDICTING STOCK PRICES WITH EXTREME LEARNING MACHINES
Öz: There are various benefits of forecasting stock prices before they actually occur. Artificial Neural Network is one of the methods that is used for forecasting stock prices. However, the difficulties such as the low speed of training process and the complexity of determining the parameters make it difficult to implement. In order to eliminate these difficulties new method named Extreme Learning Machine (ELM) is developed. The performance of ELM with different activation functions have not examined in stock price forecasting problem. In this study, ELM models with fourteen different activation functions are designed and their performance are compared with statistical and financial measurements. 12 technical indicators are calculated using the historical price and volume information belong to Goodyear, Amazon and Wal—Mart as well as SPSOO Index. The output of the model is the closing price of the next day. The performances of the models are compared with regular artificial neural network models. The stock prices are correctly forecasted up to 59.32% hit rate. Moreover, higher paper—retums are obtained from passive buy&hold strategy. The results obtained in this study shows that ELM is powerful alternative for stock price forecasting.
Anahtar Kelime: Konular:
Belge Türü: Makale Makale Türü: Araştırma Makalesi Erişim Türü: Erişime Açık
- Adebiyi, A.A., A.O. Adewumi, C.K. Ayo (2014), “Comparison of ARIMA and Artificial Neural Network Models for Stock Price Forecasting”, Journal of Applied Mathematics, 7.
- Adhikari, R., R.K. Agrawal, L. Kant (2013), “PSO Based neural Networks vs Traditional Statistical Models for Seasonal Time Series Forecasting, IEEE International Advanced Computing Conference (IACC) 2013.
- Armano, G., M. Marchesi, A. Murru (2005), “A Hybridgenetic-Neural Architecture for Stock Indexes Forecasting”, Information Sciences, 170, 3-33.
- Asadi, S., E. Hadavandi, F. Mehmanpazir, M.M. Nakhostin (2012), “Hybridization of Evolutionary LevenbergMarquardt Neural Networks and data Pre-Processing for Stock Market Prediction”, Knowledge-Based Systems, 35, 245-258.
- Atsalakis, G.S., K.P. Valavanis (2009), “Forecasting Stock Market Short-Term Trends using a Neuro-Fuzzy Based Methdolody”, Expert Systems with Applications, 36, 10696-10707.
- Atsalakis, G., K.P. Valavanis (2010), “Surveying Stock Market Forecasting Techniques – Part – I: Conventional Methods”, in Zopounidis (ed.) Computation Optimization in Economics and Finance Research Compendium, Nova Science Publishers, 49-104.
- Atsalakis, G., E.E. Protopapadakis, K.P. Valavanis (2016), “Stock Trend Forecasting in Turbulent Market Periods using Neuro-Fuzzy Systems”, Operational Research, 16, 245-269.
- Chang, J.R., L.Y. Wei, C.H. Cheng (2011), “A hybrid ANFIS Model Based on AR and Volatility for TAIEX Forecasting”, Applied Soft Computing, 11, 1388-1395.
- Chen, Q., C. Li (2006), “Comparison of Forecasting Performance of AR, STAR and ANN Models on the Chinese Stock Market Index”, Advances in Neural Networks – ISNN 2006.
- Dash, R., P.K. Dash, R. Bisoi (2014), “A self Adaptive Differential Harmony Search Based Optimized Extreme Learning Machine for Financial time Series Prediction”, Swarm and Evolutionary Computation, 19, 25-42.
- Fausett, L. (1994), Fundamentals of Neural Networks: Architectures, Algorithms and Applications, Englewood Cliffs: Prentice-Hall.
- Haykin, S. (2005), Neural Networks – A Comprehensive Foundation, India: Pearson Eductation.
- Huang, G.B., Q.Y. Zhu, C.K. Sieq (2006), “Extreme Learning Machine: Theory and Applications”, Neurocomputing, 70, 489-501.
- Huang, C.J., D.X. Yang, Y.T. Chuang (2008), “Application of Wrapper Approach and Composite Classifier to the Stock Trend Prediction”, Expert Systems with Applications, 34, 2870- 2878.
- Huang, G.B., D.H. Wang, Y. Lan (2011), “Extreme Learning Machines: a Survey”, International Journal of Machine Learning and Cybernetics, 2, 107-122.
- Huang, G., G.B. Huang, S. Song, K. You (2015), “Trends in Extreme Learning Machines: A Review”, Neural Networks, 61, 32-48.
- Huang, W., Y. Nakamori, S.Y. Wang (2005), “Forecasting Stock Market Movement Dirction with Support Vector Machine”, Computers & Operations Research, 32, 2513-2522.
- Ince, H., T.B. Trafalis (2007), “Kernel Principal Component Analysis and Support Vector Machines for Stock Price Prediction”, IIE Transactions, 39, 629-637.
- Isenah, G.M., O.E. Olubusove (2014), “Forecasting Nigerian Stock Market Returns Using ARIMA and Artificial Neural Networks”, CBN Journal of Applied Statistics, 5(2), 25-48.
- Kanas, A., A. Yannopoulos (2001), “Comparing Linear and Nonlinear Forecasts for Stock Returns”, International Review of Economics and Finance, 10(4), 383-398.
- Kara, Y., M.A. Boyacıoğlu, Ö.K. Baykan (2011), “Predicting Direction of Stock Price Index Movement Using Artificial Neural Networks and Support Vector Machines: The Sample of the Istanbul Stock Exchange”, Expert Systems with Applications, 38, 5311- 5319.
- Li, X., H. Xie, R. Wang, Y. Cai, J. Cao, F. Wang, H. Min, X. Deng (2016), “Empirical Analysis: Stock Market Prediction via Extreme Learning Machine”, Neural Computing & Applications, 27, 67-78.
- Mahmud, M.S., P. Meesad (2015), “An Innovative Recurrent Error-Based Neuro-Fuzzy System with Momentum for Stock Price Prediction”, Soft Computing, 20(10), 4173-4191.
- MSCI (2016). https://www.msci.com/gics, E.T. :10.11.2016.
- Özçalıcı, M. (2016), “Hisse Senetlerinin Doğru Tahmin Oranları ile Kümelendirilmesi”, Marmara Üniversitesi İktisadi ve İdari Bilimler Dergisi, 38(1), 279-293.
- Palit, A.K., D. Popovic (2005), Computational Intelligence in Time Series Forecasting: Theory and Engineering Applications, London: Springer-Verlag.
- Smith, K.A., J.N.D. Gupta (2000), “Neural Networks in Business: Techniques and Applications for the Operations Researcher”, Computers & Operation Research, 27, 1023-1044.
- Sumathi, S., P. Surekha (2010), Computational Intelligence Paradigms: Theory and Applications using MATLAB. Taylor and Francis Group, LLC. [NN_02]
- Sun, Z.L., T.M. Choi, K.F. Au, Y. Yu (2008), “Sales Forecasting Using Extreme Learning Machnie with Applications in Fashion Retailing”, Decision Support Systems, 46(1), 411-419.
- Wang, J.Z., J.J. Wang, Z.G. Zhang, S.P. Guo (2011). “Forecasting Stock Indices with back Propagation Neural Network”, Expert Systems with Applications, 38, 14346-14355.
- Wong, W.K., Z.X. Guo (2010), “A Hybridintelligent Model for Medium-Term Sales Forecasting in Fashion Retail Supply Chains Using Extreme Learning Machine and Harmony Search Algorithm”, International Journal of Production Economics, 128, 614-624.
- Xia, M., Y. Zhang, L. Weng, X. Ye (2012), “Fashion Retailing Forecasting Based on Extreme Learning Machine with Adaptive Metrics of Inputs”, Knowledge-Based Systems, 36, 253-259.
- Xia, C., Z.Y. Dong, K. Meng, Y. Xu, K.P. Wong, H.W. Ngan (2012), “Electricity Price Forecasting with Extreme Learning Machine and Bootstrapping”, IEEE Transactions on Power Systems, 27(4), 1-8.
- Yan, Q., S. Wang, B. Li B. (2014), “Forecasting Uranium Resource Price Prediction by Extreme Learning Machine with Empirical Mode Decomposition and Phase Space Reconstruction”, Discrete Dynamics in Nature and Society, 2014, 1-10.
APA | ÖZÇALICI M (2017). AŞIRI ÖĞRENME MAKİNELERİ İLE HİSSE SENEDİ FİYAT TAHMİNİ. , 67 - 89. |
Chicago | ÖZÇALICI Mehmet AŞIRI ÖĞRENME MAKİNELERİ İLE HİSSE SENEDİ FİYAT TAHMİNİ. (2017): 67 - 89. |
MLA | ÖZÇALICI Mehmet AŞIRI ÖĞRENME MAKİNELERİ İLE HİSSE SENEDİ FİYAT TAHMİNİ. , 2017, ss.67 - 89. |
AMA | ÖZÇALICI M AŞIRI ÖĞRENME MAKİNELERİ İLE HİSSE SENEDİ FİYAT TAHMİNİ. . 2017; 67 - 89. |
Vancouver | ÖZÇALICI M AŞIRI ÖĞRENME MAKİNELERİ İLE HİSSE SENEDİ FİYAT TAHMİNİ. . 2017; 67 - 89. |
IEEE | ÖZÇALICI M "AŞIRI ÖĞRENME MAKİNELERİ İLE HİSSE SENEDİ FİYAT TAHMİNİ." , ss.67 - 89, 2017. |
ISNAD | ÖZÇALICI, Mehmet. "AŞIRI ÖĞRENME MAKİNELERİ İLE HİSSE SENEDİ FİYAT TAHMİNİ". (2017), 67-89. |
APA | ÖZÇALICI M (2017). AŞIRI ÖĞRENME MAKİNELERİ İLE HİSSE SENEDİ FİYAT TAHMİNİ. Hacettepe Üniversitesi İktisadi ve İdari Bilimler Fakültesi Dergisi, 35(1), 67 - 89. |
Chicago | ÖZÇALICI Mehmet AŞIRI ÖĞRENME MAKİNELERİ İLE HİSSE SENEDİ FİYAT TAHMİNİ. Hacettepe Üniversitesi İktisadi ve İdari Bilimler Fakültesi Dergisi 35, no.1 (2017): 67 - 89. |
MLA | ÖZÇALICI Mehmet AŞIRI ÖĞRENME MAKİNELERİ İLE HİSSE SENEDİ FİYAT TAHMİNİ. Hacettepe Üniversitesi İktisadi ve İdari Bilimler Fakültesi Dergisi, vol.35, no.1, 2017, ss.67 - 89. |
AMA | ÖZÇALICI M AŞIRI ÖĞRENME MAKİNELERİ İLE HİSSE SENEDİ FİYAT TAHMİNİ. Hacettepe Üniversitesi İktisadi ve İdari Bilimler Fakültesi Dergisi. 2017; 35(1): 67 - 89. |
Vancouver | ÖZÇALICI M AŞIRI ÖĞRENME MAKİNELERİ İLE HİSSE SENEDİ FİYAT TAHMİNİ. Hacettepe Üniversitesi İktisadi ve İdari Bilimler Fakültesi Dergisi. 2017; 35(1): 67 - 89. |
IEEE | ÖZÇALICI M "AŞIRI ÖĞRENME MAKİNELERİ İLE HİSSE SENEDİ FİYAT TAHMİNİ." Hacettepe Üniversitesi İktisadi ve İdari Bilimler Fakültesi Dergisi, 35, ss.67 - 89, 2017. |
ISNAD | ÖZÇALICI, Mehmet. "AŞIRI ÖĞRENME MAKİNELERİ İLE HİSSE SENEDİ FİYAT TAHMİNİ". Hacettepe Üniversitesi İktisadi ve İdari Bilimler Fakültesi Dergisi 35/1 (2017), 67-89. |